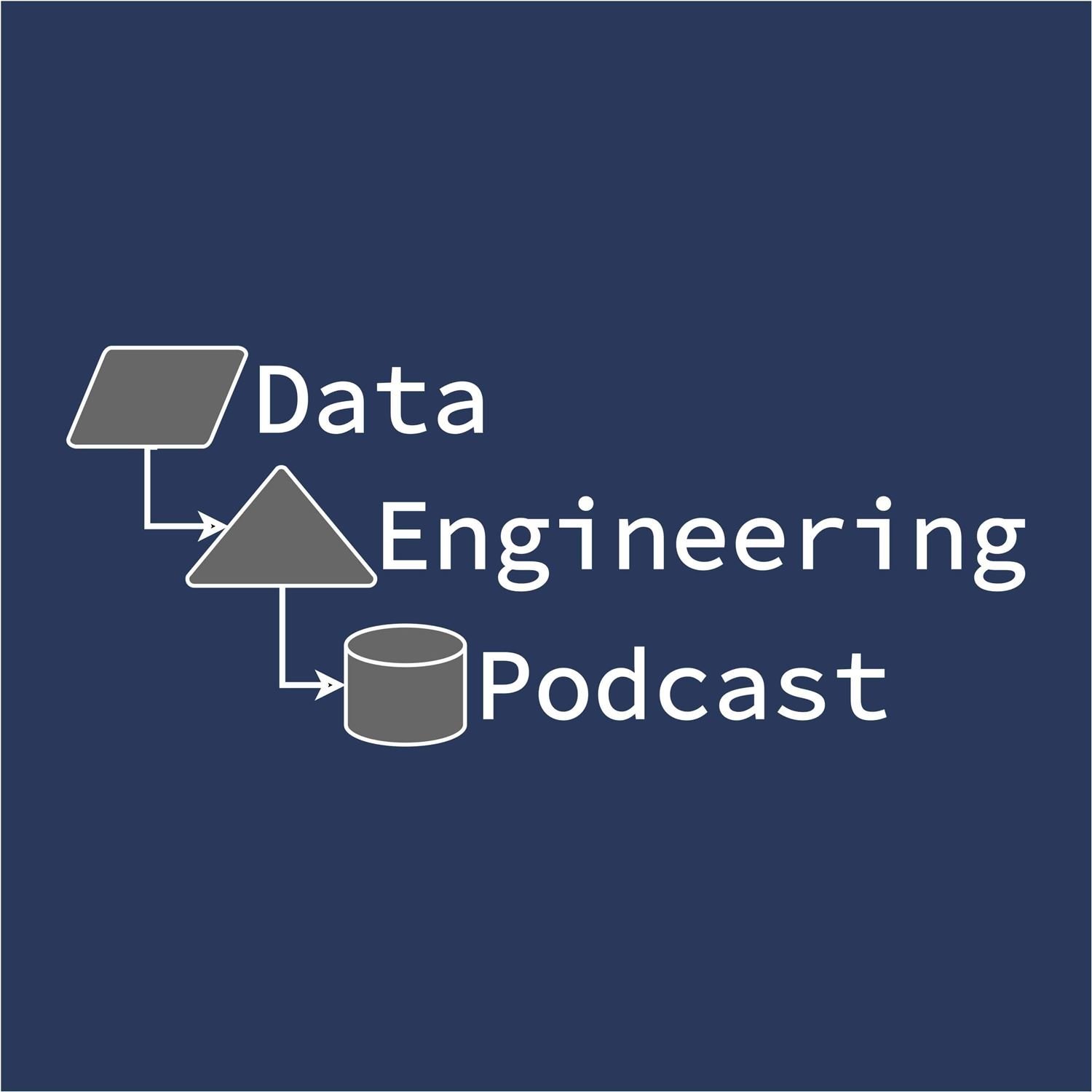
Education
Technology
Tobias Macey
This show goes behind the scenes for the tools, techniques, and difficulties associated with the discipline of data engineering. Databases, workflows, automation, and data manipulation are just some of the topics that you will find here.
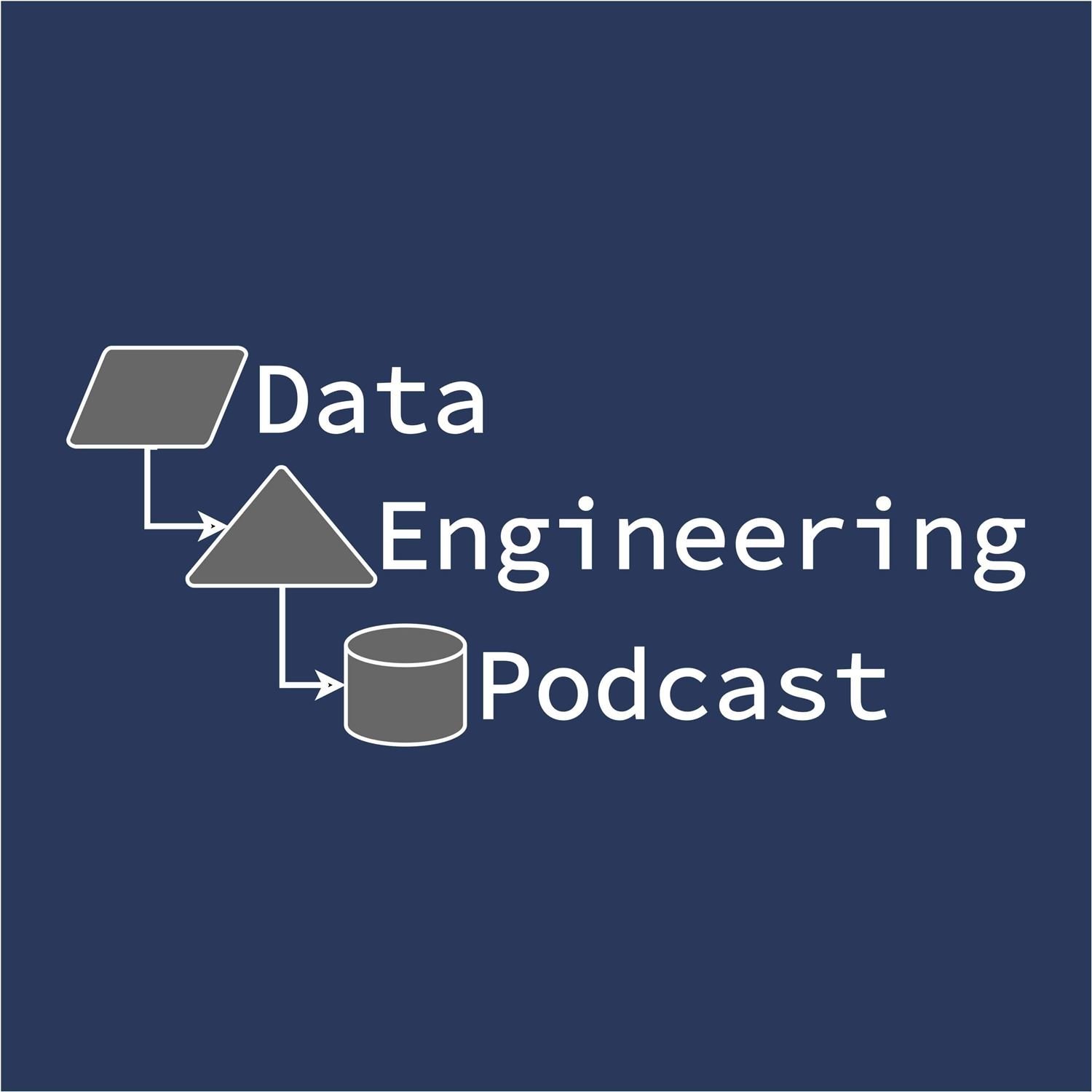
Building A Community For Data Professionals at Data Council
Summary
Data professionals are working in a domain that is rapidly evolving. In order to stay current we need access to deeply technical presentations that aren’t burdened by extraneous marketing. To fulfill that need Pete Soderling and his team have been running the Data Council series of conferences and meetups around the world. In this episode Pete discusses his motivation for starting these events, how they serve to bring the data community together, and the observations that he has made about the direction that we are moving. He also shares his experiences as an investor in developer oriented startups and his views on the importance of empowering engineers to launch their own companies.
Announcements
Hello and welcome to the Data Engineering Podcast, the show about modern data management
When you’re ready to build your next pipeline, or want to test out the projects you hear about on the show, you’ll need somewhere to deploy it, so check out our friends at Linode. With 200Gbit private networking, scalable shared block storage, and a 40Gbit public network, you’ve got everything you need to run a fast, reliable, and bullet-proof data platform. If you need global distribution, they’ve got that covered too with world-wide datacenters including new ones in Toronto and Mumbai. And for your machine learning workloads, they just announced dedicated CPU instances. Go to dataengineeringpodcast.com/linode today to get a $20 credit and launch a new server in under a minute. And don’t forget to thank them for their continued support of this show!
Listen, I’m sure you work for a ‘data driven’ company – who doesn’t these days? Does your company use Amazon Redshift? Have you ever groaned over slow queries or are just afraid that Amazon Redshift is gonna fall over at some point? Well, you’ve got to talk to the folks over at intermix.io. They have built the “missing” Amazon Redshift console – it’s an amazing analytics product for data engineers to find and re-write slow queries and gives actionable recommendations to optimize data pipelines. WeWork, Postmates, and Medium are just a few of their customers. Go to dataengineeringpodcast.com/intermix today and use promo code DEP at sign up to get a $50 discount!
You listen to this show to learn and stay up to date with what’s happening in databases, streaming platforms, big data, and everything else you need to know about modern data management.For even more opportunities to meet, listen, and learn from your peers you don’t want to miss out on this year’s conference season. We have partnered with organizations such as O’Reilly Media, Dataversity, Corinium Global Intelligence, and Data Council. Upcoming events include the O’Reilly AI conference, the Strata Data conference, the combined events of the Data Architecture Summit and Graphorum, and Data Council in Barcelona. Go to dataengineeringpodcast.com/conferences to learn more about these and other events, and take advantage of our partner discounts to save money when you register today.
Your host is Tobias Macey and today I’m interviewing Pete Soderling about his work to build and grow a community for data professionals with the Data Council conferences and meetups, as well as his experiences as an investor in data oriented companies
Interview
Introduction
How did you get involved in the area of data management?
What was your original reason for focusing your efforts on fostering a community of data engineers?
What was the state of recognition in the industry for that role at the time that you began your efforts?
The current manifestation of your community efforts is in the form of the Data Council conferences and meetups. Previously they were known as Data Eng Conf and before that was Hakka Labs. Can you discuss the evolution of your efforts to grow this community?
How has the community itself changed and grown over the past few years?
Communities form around a huge variety of focal points. What are some of the complexities or challenges in building one based on something as nebulous as data?
Where do you draw inspiration and direction for how to manage such a large and distributed community?
What are some of the most interesting/challenging/unexpected aspects of community management that you have encountered?
What are some ways that you have been surprised or delighted in your interactions with the data community?
How do you approach sustainability of the Data Council community and the organization itself?
The tagline that you have focused on for Data Council events is that they are no fluff, juxtaposing them against larger business oriented events. What are your guidelines for fulfilling that promise and why do you think that is an important distinction?
In addition to your community building you are also an investor. How did you get involved in that side of your business and how does it fit into your overall mission?
You also have a stated mission to help engineers build their own companies. In your opinion, how does an engineer led business differ from one that may be founded or run by a business oriented individual and why do you think that we need more of them?
What are the ways that you typically work to empower engineering founders or encourage them to create their own businesses?
What are some of the challenges that engineering founders face and what are some common difficulties or misunderstandings related to business?
What are your opinions on venture-backed vs. "lifestyle" or bootstrapped businesses?
What are the characteristics of a data business that you look at when evaluating a potential investment?
What are some of the current industry trends that you are most excited by?
What are some that you find concerning?
What are your goals and plans for the future of Data Council?
Contact Info
@petesoder on Twitter
LinkedIn
@petesoder on Medium
Parting Question
From your perspective, what is the biggest gap in the tooling or technology for data management today?
Closing Announcements
Thank you for listening! Don’t forget to check out our other show, Podcast.__init__ to learn about the Python language, its community, and the innovative ways it is being used.
Visit the site to subscribe to the show, sign up for the mailing list, and read the show notes.
If you’ve learned something or tried out a project from the show then tell us about it! Email [email protected]) with your story.
To help other people find the show please leave a review on iTunes and tell your friends and co-workers
Join the community in the new Zulip chat workspace at dataengineeringpodcast.com/chat
Links
Data Council
Database Design For Mere Mortals
Bloomberg
Garmin
500 Startups
Geeks On A Plane
Data Council NYC 2019 Track Summary
Pete’s Angel List Syndicate
DataOps
Data Kitchen Episode
DataOps Vs DevOps Episode
Great Expectations
Podcast.__init__ Interview
Elementl
Dagster
Data Council Presentation
Data Council Call For Proposals
The intro and outro music is from The Hug by The Freak Fandango Orchestra / CC BY-SA
Support Data Engineering Podcast
52:4602/09/2019
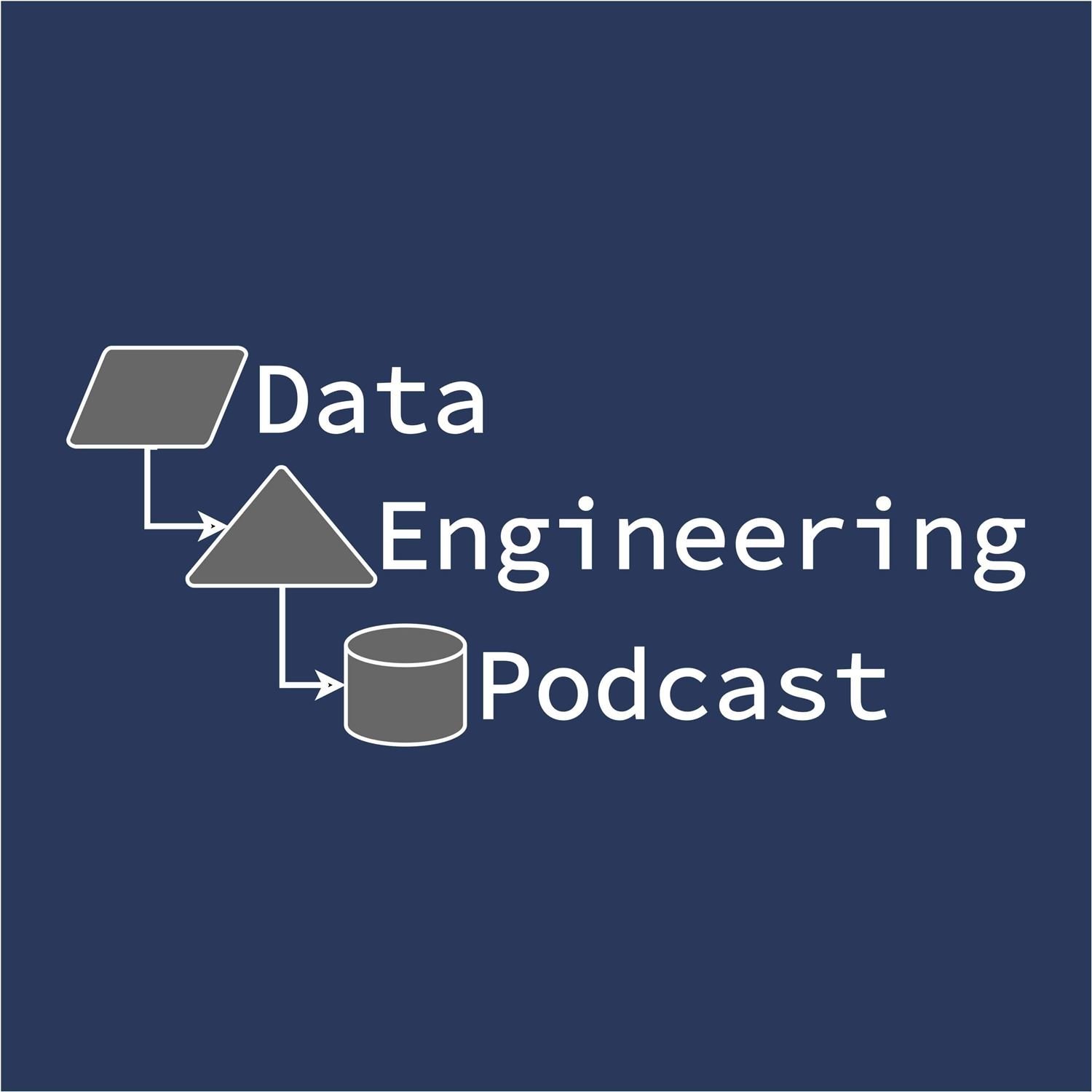
Building Tools And Platforms For Data Analytics
Summary
Data engineers are responsible for building tools and platforms to power the workflows of other members of the business. Each group of users has their own set of requirements for the way that they access and interact with those platforms depending on the insights they are trying to gather. Benn Stancil is the chief analyst at Mode Analytics and in this episode he explains the set of considerations and requirements that data analysts need in their tools and. He also explains useful patterns for collaboration between data engineers and data analysts, and what they can learn from each other.
Announcements
Hello and welcome to the Data Engineering Podcast, the show about modern data management
When you’re ready to build your next pipeline, or want to test out the projects you hear about on the show, you’ll need somewhere to deploy it, so check out our friends at Linode. With 200Gbit private networking, scalable shared block storage, and a 40Gbit public network, you’ve got everything you need to run a fast, reliable, and bullet-proof data platform. If you need global distribution, they’ve got that covered too with world-wide datacenters including new ones in Toronto and Mumbai. And for your machine learning workloads, they just announced dedicated CPU instances. Go to dataengineeringpodcast.com/linode today to get a $20 credit and launch a new server in under a minute. And don’t forget to thank them for their continued support of this show!
You listen to this show to learn and stay up to date with what’s happening in databases, streaming platforms, big data, and everything else you need to know about modern data management.For even more opportunities to meet, listen, and learn from your peers you don’t want to miss out on this year’s conference season. We have partnered with organizations such as O’Reilly Media, Dataversity, Corinium Global Intelligence, and Data Counsil. Upcoming events include the O’Reilly AI conference, the Strata Data conference, the combined events of the Data Architecture Summit and Graphorum, and Data Council in Barcelona. Go to dataengineeringpodcast.com/conferences to learn more about these and other events, and take advantage of our partner discounts to save money when you register today.
Your host is Tobias Macey and today I’m interviewing Benn Stancil, chief analyst at Mode Analytics, about what data engineers need to know when building tools for analysts
Interview
Introduction
How did you get involved in the area of data management?
Can you start by describing some of the main features that you are looking for in the tools that you use?
What are some of the common shortcomings that you have found in out-of-the-box tools that organizations use to build their data stack?
What should data engineers be considering as they design and implement the foundational data platforms that higher order systems are built on, which are ultimately used by analysts and data scientists?
In terms of mindset, what are the ways that data engineers and analysts can align and where are the points of conflict?
In terms of team and organizational structure, what have you found to be useful patterns for reducing friction in the product lifecycle for data tools (internal or external)?
What are some anti-patterns that data engineers can guard against as they are designing their pipelines?
In your experience as an analyst, what have been the characteristics of the most seamless projects that you have been involved with?
How much understanding of analytics are necessary for data engineers to be successful in their projects and careers?
Conversely, how much understanding of data management should analysts have?
What are the industry trends that you are most excited by as an analyst?
Contact Info
LinkedIn
@bennstancil on Twitter
Website
Parting Question
From your perspective, what is the biggest gap in the tooling or technology for data management today?
Closing Announcements
Thank you for listening! Don’t forget to check out our other show, Podcast.__init__ to learn about the Python language, its community, and the innovative ways it is being used.
Visit the site to subscribe to the show, sign up for the mailing list, and read the show notes.
If you’ve learned something or tried out a project from the show then tell us about it! Email [email protected]) with your story.
To help other people find the show please leave a review on iTunes and tell your friends and co-workers
Join the community in the new Zulip chat workspace at dataengineeringpodcast.com/chat
Links
Mode Analytics
Data Council Presentation
Yammer
StitchFix Blog Post
SnowflakeDB
Re:Dash
Superset
Marquez
Amundsen
Podcast Episode
Elementl
Dagster
Data Council Presentation
DBT
Podcast Episode
Great Expectations
Podcast.__init__ Episode
Delta Lake
Podcast Episode
Stitch
Fivetran
Podcast Episode
The intro and outro music is from The Hug by The Freak Fandango Orchestra / CC BY-SA
Support Data Engineering Podcast
48:0726/08/2019
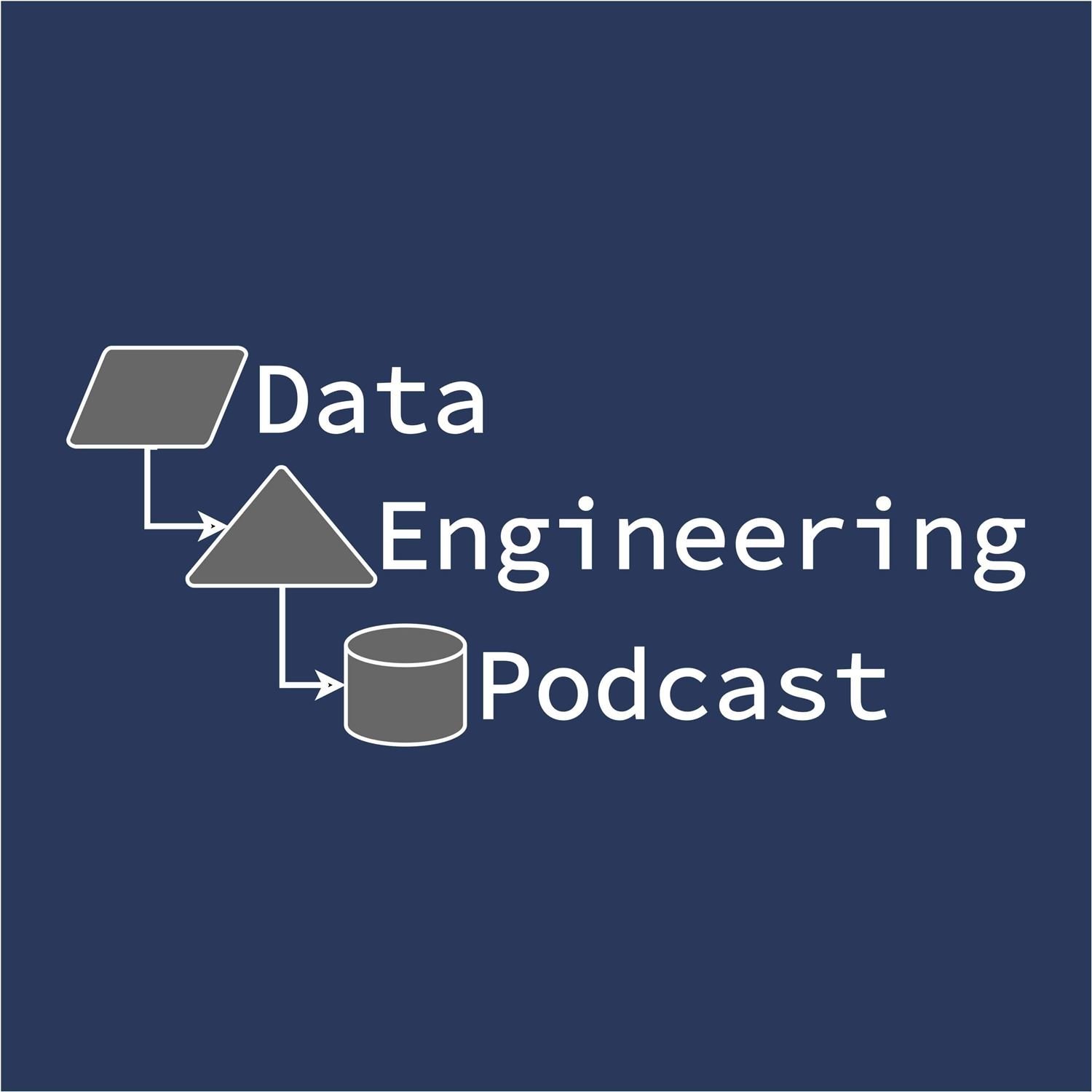
A High Performance Platform For The Full Big Data Lifecycle
Summary
Managing big data projects at scale is a perennial problem, with a wide variety of solutions that have evolved over the past 20 years. One of the early entrants that predates Hadoop and has since been open sourced is the HPCC (High Performance Computing Cluster) system. Designed as a fully integrated platform to meet the needs of enterprise grade analytics it provides a solution for the full lifecycle of data at massive scale. In this episode Flavio Villanustre, VP of infrastructure and products at HPCC Systems, shares the history of the platform, how it is architected for scale and speed, and the unique solutions that it provides for enterprise grade data analytics. He also discusses the motivations for open sourcing the platform, the detailed workflow that it enables, and how you can try it for your own projects. This was an interesting view of how a well engineered product can survive massive evolutionary shifts in the industry while remaining relevant and useful.
Announcements
Hello and welcome to the Data Engineering Podcast, the show about modern data management
When you’re ready to build your next pipeline, or want to test out the projects you hear about on the show, you’ll need somewhere to deploy it, so check out our friends at Linode. With 200Gbit private networking, scalable shared block storage, and a 40Gbit public network, you’ve got everything you need to run a fast, reliable, and bullet-proof data platform. If you need global distribution, they’ve got that covered too with world-wide datacenters including new ones in Toronto and Mumbai. And for your machine learning workloads, they just announced dedicated CPU instances. Go to dataengineeringpodcast.com/linode today to get a $20 credit and launch a new server in under a minute. And don’t forget to thank them for their continued support of this show!
To connect with the startups that are shaping the future and take advantage of the opportunities that they provide, check out Angel List where you can invest in innovative business, find a job, or post a position of your own. Sign up today at dataengineeringpodcast.com/angel and help support this show.
You listen to this show to learn and stay up to date with what’s happening in databases, streaming platforms, big data, and everything else you need to know about modern data management.For even more opportunities to meet, listen, and learn from your peers you don’t want to miss out on this year’s conference season. We have partnered with organizations such as O’Reilly Media, Dataversity, Corinium Global Intelligence, and Data Counsil. Upcoming events include the O’Reilly AI conference, the Strata Data conference, the combined events of the Data Architecture Summit and Graphorum, and Data Council in Barcelona. Go to dataengineeringpodcast.com/conferences to learn more about these and other events, and take advantage of our partner discounts to save money when you register today.
Go to dataengineeringpodcast.com to subscribe to the show, sign up for the mailing list, read the show notes, and get in touch.
To help other people find the show please leave a review on iTunes and tell your friends and co-workers
Join the community in the new Zulip chat workspace at dataengineeringpodcast.com/chat
Your host is Tobias Macey and today I’m interviewing Flavio Villanustre about the HPCC Systems project and his work at LexisNexis Risk Solutions
Interview
Introduction
How did you get involved in the area of data management?
Can you start by describing what the HPCC system is and the problems that you were facing at LexisNexis Risk Solutions which led to its creation?
What was the overall state of the data landscape at the time and what was the motivation for releasing it as open source?
Can you describe the high level architecture of the HPCC Systems platform and some of the ways that the design has changed over the years that it has been maintained?
Given how long the project has been in use, can you talk about some of the ways that it has had to evolve to accomodate changing trends in usage and technologies for big data and advanced analytics?
For someone who is using HPCC Systems, can you talk through a common workflow and the ways that the data traverses the various components?
How does HPCC Systems manage persistence and scalability?
What are the integration points available for extending and enhancing the HPCC Systems platform?
What is involved in deploying and managing a production installation of HPCC Systems?
The ECL language is an intriguing element of the overall system. What are some of the features that it provides which simplify processing and management of data?
How does the Thor engine manage data transformation and manipulation?
What are some of the unique features of Thor and how does it compare to other approaches for ETL and data integration?
For extraction and analysis of data can you talk through the capabilities of the Roxie engine?
How are you using the HPCC Systems platform in your work at LexisNexis?
Despite being older than the Hadoop platform it doesn’t seem that HPCC Systems has seen the same level of growth and popularity. Can you share your perspective on the community for HPCC Systems and how it compares to that of Hadoop over the past decade?
How is the HPCC Systems project governed, and what is your approach to sustainability?
What are some of the additional capabilities that are only available in the enterprise distribution?
When is the HPCC Systems platform the wrong choice, and what are some systems that you might use instead?
What have been some of the most interesting/unexpected/novel ways that you have seen HPCC Systems used?
What are some of the challenges that you have faced and lessons that you have learned while building and maintaining the HPCC Systems platform and community?
What do you have planned for the future of HPCC Systems?
Contact Info
LinkedIn
@fvillanustre on Twitter
Parting Question
From your perspective, what is the biggest gap in the tooling or technology for data management today?
Links
HPCC Systems
LexisNexis Risk Solutions
Risk Management
Hadoop
MapReduce
Sybase
Oracle DB
AbInitio
Data Lake
SQL
ECL
DataFlow
TensorFlow
ECL IDE
The intro and outro music is from The Hug by The Freak Fandango Orchestra / CC BY-SA
Support Data Engineering Podcast
01:13:4619/08/2019
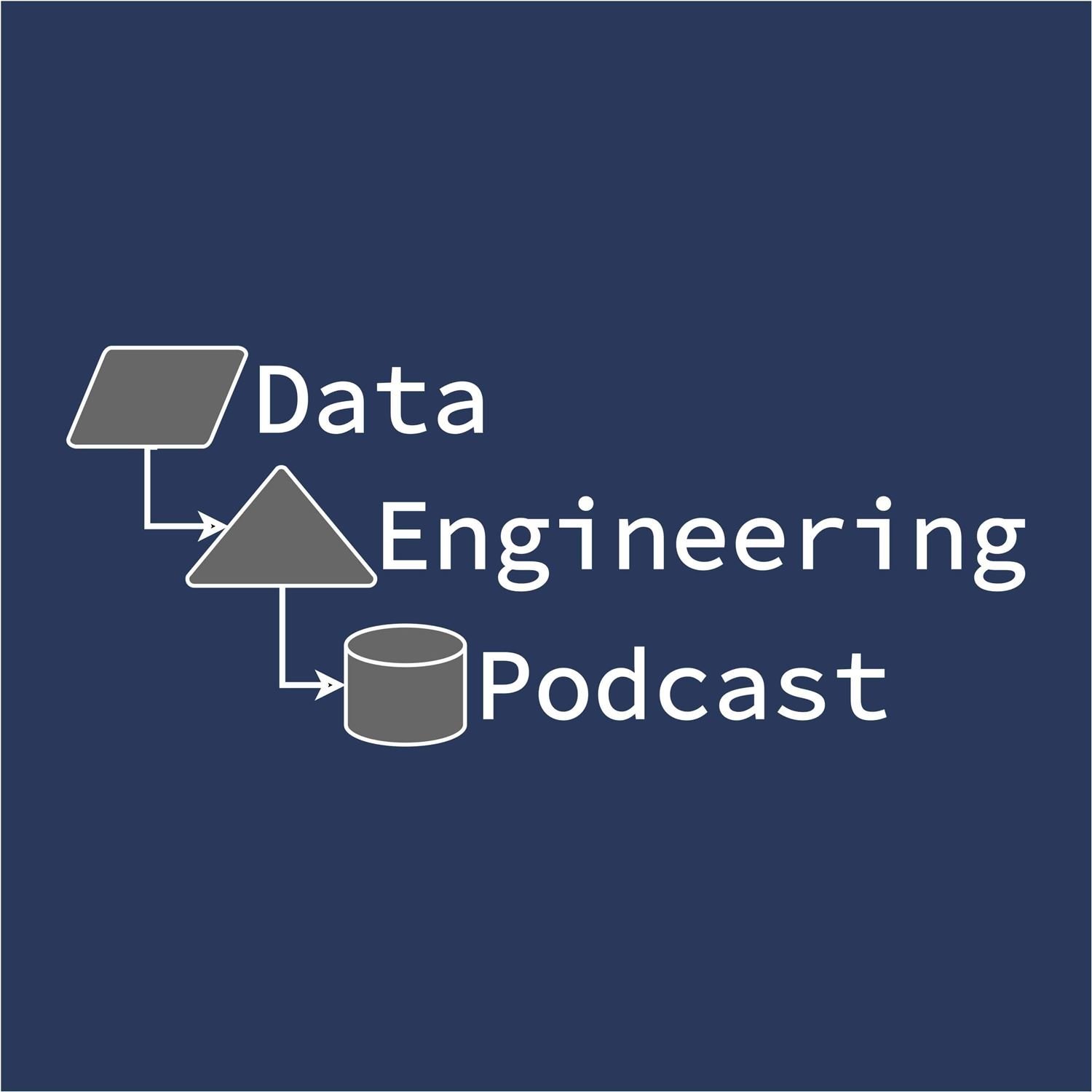
Digging Into Data Replication At Fivetran
Summary
The extract and load pattern of data replication is the most commonly needed process in data engineering workflows. Because of the myriad sources and destinations that are available, it is also among the most difficult tasks that we encounter. Fivetran is a platform that does the hard work for you and replicates information from your source systems into whichever data warehouse you use. In this episode CEO and co-founder George Fraser explains how it is built, how it got started, and the challenges that creep in at the edges when dealing with so many disparate systems that need to be made to work together. This is a great conversation to listen to for a better understanding of the challenges inherent in synchronizing your data.
Announcements
Hello and welcome to the Data Engineering Podcast, the show about modern data management
When you’re ready to build your next pipeline, or want to test out the projects you hear about on the show, you’ll need somewhere to deploy it, so check out our friends at Linode. With 200Gbit private networking, scalable shared block storage, and a 40Gbit public network, you’ve got everything you need to run a fast, reliable, and bullet-proof data platform. If you need global distribution, they’ve got that covered too with world-wide datacenters including new ones in Toronto and Mumbai. And for your machine learning workloads, they just announced dedicated CPU instances. Go to dataengineeringpodcast.com/linode today to get a $20 credit and launch a new server in under a minute. And don’t forget to thank them for their continued support of this show!
You listen to this show to learn and stay up to date with what’s happening in databases, streaming platforms, big data, and everything else you need to know about modern data management.For even more opportunities to meet, listen, and learn from your peers you don’t want to miss out on this year’s conference season. We have partnered with organizations such as O’Reilly Media, Dataversity, and Corinium Global Intelligence. Upcoming events include the O’Reilly AI Conference, the Strata Data Conference, and the combined events of the Data Architecture Summit and Graphorum. Go to dataengineeringpodcast.com/conferences to learn more and take advantage of our partner discounts when you register.
Go to dataengineeringpodcast.com to subscribe to the show, sign up for the mailing list, read the show notes, and get in touch.
To help other people find the show please leave a review on iTunes and tell your friends and co-workers
Join the community in the new Zulip chat workspace at dataengineeringpodcast.com/chat
Your host is Tobias Macey and today I’m interviewing George Fraser about FiveTran, a hosted platform for replicating your data from source to destination
Interview
Introduction
How did you get involved in the area of data management?
Can you start by describing the problem that Fivetran solves and the story of how it got started?
Integration of multiple data sources (e.g. entity resolution)
How is Fivetran architected and how has the overall system design changed since you first began working on it?
monitoring and alerting
Automated schema normalization. How does it work for customized data sources?
Managing schema drift while avoiding data loss
Change data capture
What have you found to be the most complex or challenging data sources to work with reliably?
Workflow for users getting started with Fivetran
When is Fivetran the wrong choice for collecting and analyzing your data?
What have you found to be the most challenging aspects of working in the space of data integrations?}}
What have been the most interesting/unexpected/useful lessons that you have learned while building and growing Fivetran?
What do you have planned for the future of Fivetran?
Contact Info
LinkedIn
@frasergeorgew on Twitter
Parting Question
From your perspective, what is the biggest gap in the tooling or technology for data management today?
Links
Fivetran
Ralph Kimball
DBT (Data Build Tool)
Podcast Interview
Looker
Podcast Interview
Cron
Kubernetes
Postgres
Podcast Episode
Oracle DB
Salesforce
Netsuite
Marketo
Jira
Asana
Cloudwatch
Stackdriver
The intro and outro music is from The Hug by The Freak Fandango Orchestra / CC BY-SA
Support Data Engineering Podcast
44:4112/08/2019
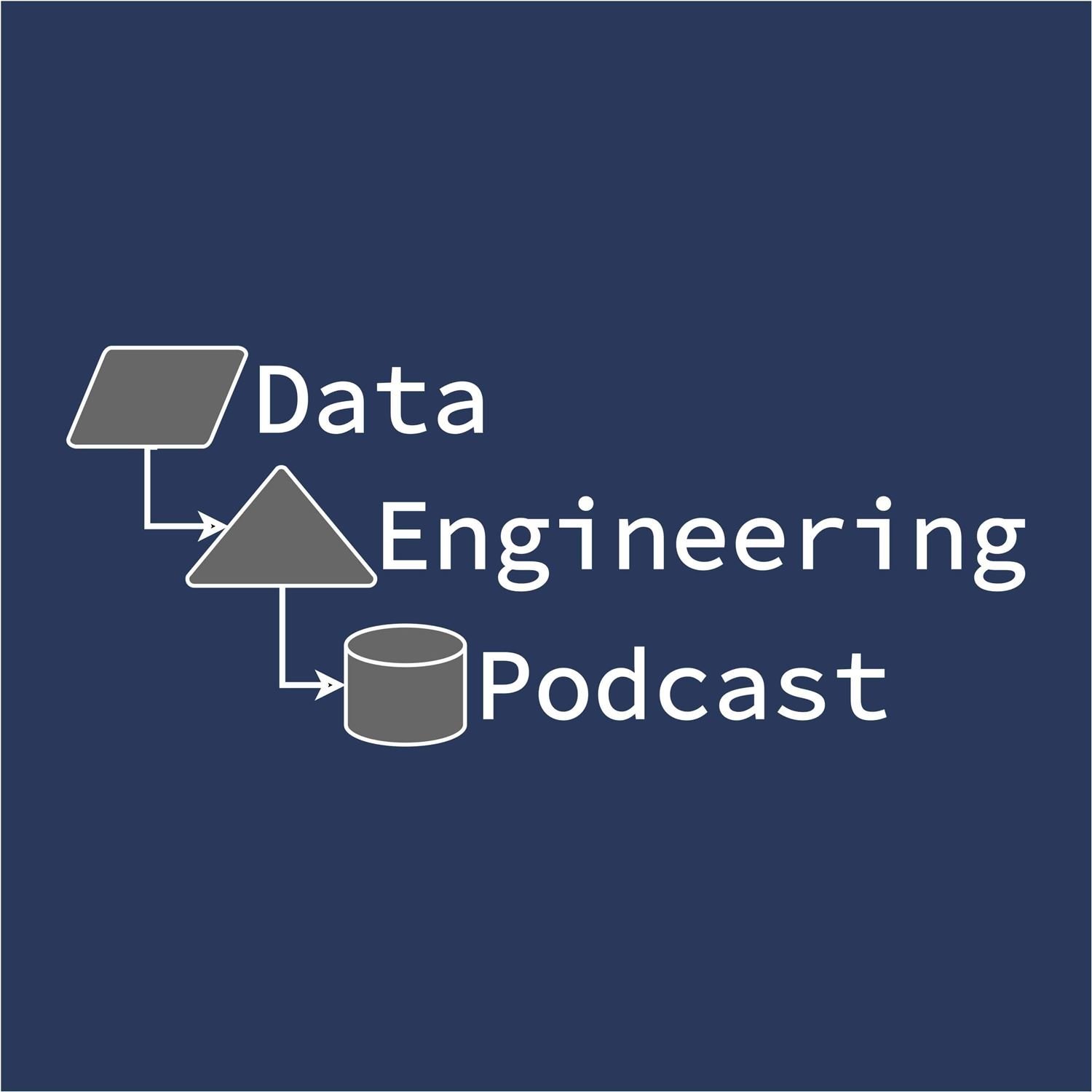
Solving Data Discovery At Lyft
Summary
Data is only valuable if you use it for something, and the first step is knowing that it is available. As organizations grow and data sources proliferate it becomes difficult to keep track of everything, particularly for analysts and data scientists who are not involved with the collection and management of that information. Lyft has build the Amundsen platform to address the problem of data discovery and in this episode Tao Feng and Mark Grover explain how it works, why they built it, and how it has impacted the workflow of data professionals in their organization. If you are struggling to realize the value of your information because you don’t know what you have or where it is then give this a listen and then try out Amundsen for yourself.
Announcements
Welcome to the Data Engineering Podcast, the show about modern data management
When you’re ready to build your next pipeline, or want to test out the projects you hear about on the show, you’ll need somewhere to deploy it, so check out our friends at Linode. With 200Gbit private networking, scalable shared block storage, and a 40Gbit public network, you’ve got everything you need to run a fast, reliable, and bullet-proof data platform. If you need global distribution, they’ve got that covered too with world-wide datacenters including new ones in Toronto and Mumbai. And for your machine learning workloads, they just announced dedicated CPU instances. Go to dataengineeringpodcast.com/linode today to get a $20 credit and launch a new server in under a minute. And don’t forget to thank them for their continued support of this show!
Finding the data that you need is tricky, and Amundsen will help you solve that problem. And as your data grows in volume and complexity, there are foundational principles that you can follow to keep data workflows streamlined. Mode – the advanced analytics platform that Lyft trusts – has compiled 3 reasons to rethink data discovery. Read them at dataengineeringpodcast.com/mode-lyft.
You listen to this show to learn and stay up to date with what’s happening in databases, streaming platforms, big data, and everything else you need to know about modern data management.For even more opportunities to meet, listen, and learn from your peers you don’t want to miss out on this year’s conference season. We have partnered with organizations such as O’Reilly Media, Dataversity, the Open Data Science Conference, and Corinium Intelligence. Upcoming events include the O’Reilly AI Conference, the Strata Data Conference, and the combined events of the Data Architecture Summit and Graphorum. Go to dataengineeringpodcast.com/conferences to learn more and take advantage of our partner discounts when you register.
Go to dataengineeringpodcast.com to subscribe to the show, sign up for the mailing list, read the show notes, and get in touch.
To help other people find the show please leave a review on iTunes and tell your friends and co-workers
Join the community in the new Zulip chat workspace at dataengineeringpodcast.com/chat
Your host is Tobias Macey and today I’m interviewing Mark Grover and Tao Feng about Amundsen, the data discovery platform and metadata engine that powers self service data access at Lyft
Interview
Introduction
How did you get involved in the area of data management?
Can you start by explaining what Amundsen is and the problems that it was designed to address?
What was lacking in the existing projects at the time that led you to building a new platform from the ground up?
How does Amundsen fit in the larger ecosystem of data tools?
How does it compare to what WeWork is building with Marquez?
Can you describe the overall architecture of Amundsen and how it has evolved since you began working on it?
What were the main assumptions that you had going into this project and how have they been challenged or updated in the process of building and using it?
What has been the impact of Amundsen on the workflows of data teams at Lyft?
Can you talk through an example workflow for someone using Amundsen?
Once a dataset has been located, how does Amundsen simplify the process of accessing that data for analysis or further processing?
How does the information in Amundsen get populated and what is the process for keeping it up to date?
What was your motivation for releasing it as open source and how much effort was involved in cleaning up the code for the public?
What are some of the capabilities that you have intentionally decided not to implement yet?
For someone who wants to run their own instance of Amundsen what is involved in getting it deployed and integrated?
What have you found to be the most challenging aspects of building, using and maintaining Amundsen?
What do you have planned for the future of Amundsen?
Contact Info
Tao
LinkedIn
feng-tao on GitHub
Mark
LinkedIn
Website
Parting Question
From your perspective, what is the biggest gap in the tooling or technology for data management today?
Links
Amundsen
Data Council Presentation
Strata Presentation
Blog Post
Lyft
Airflow
Podcast.__init__ Episode
LinkedIn
Slack
Marquez
S3
Hive
Presto
Podcast Episode
Spark
PostgreSQL
Google BigQuery
Neo4J
Apache Atlas
Tableau
Superset
Alation
Cloudera Navigator
DynamoDB
MongoDB
Druid
The intro and outro music is from The Hug by The Freak Fandango Orchestra / CC BY-SA
Support Data Engineering Podcast
51:4805/08/2019
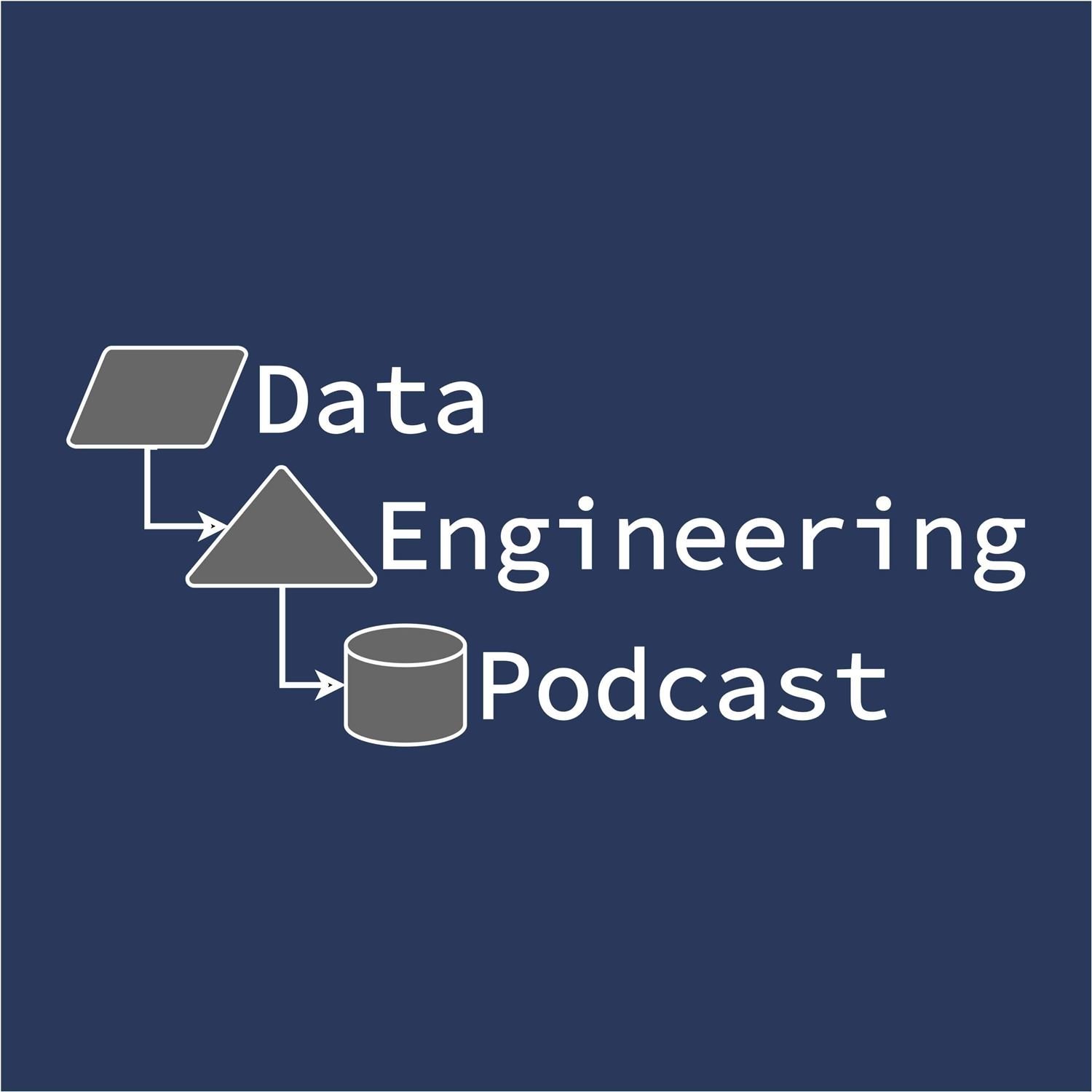
Simplifying Data Integration Through Eventual Connectivity
Summary
The ETL pattern that has become commonplace for integrating data from multiple sources has proven useful, but complex to maintain. For a small number of sources it is a tractable problem, but as the overall complexity of the data ecosystem continues to expand it may be time to identify new ways to tame the deluge of information. In this episode Tim Ward, CEO of CluedIn, explains the idea of eventual connectivity as a new paradigm for data integration. Rather than manually defining all of the mappings ahead of time, we can rely on the power of graph databases and some strategic metadata to allow connections to occur as the data becomes available. If you are struggling to maintain a tangle of data pipelines then you might find some new ideas for reducing your workload.
Announcements
Hello and welcome to the Data Engineering Podcast, the show about modern data management
When you’re ready to build your next pipeline, or want to test out the projects you hear about on the show, you’ll need somewhere to deploy it, so check out our friends at Linode. With 200Gbit private networking, scalable shared block storage, and a 40Gbit public network, you’ve got everything you need to run a fast, reliable, and bullet-proof data platform. If you need global distribution, they’ve got that covered too with world-wide datacenters including new ones in Toronto and Mumbai. And for your machine learning workloads, they just announced dedicated CPU instances. Go to dataengineeringpodcast.com/linode today to get a $20 credit and launch a new server in under a minute. And don’t forget to thank them for their continued support of this show!
To connect with the startups that are shaping the future and take advantage of the opportunities that they provide, check out Angel List where you can invest in innovative business, find a job, or post a position of your own. Sign up today at dataengineeringpodcast.com/angel and help support this show.
You listen to this show to learn and stay up to date with what’s happening in databases, streaming platforms, big data, and everything else you need to know about modern data management.For even more opportunities to meet, listen, and learn from your peers you don’t want to miss out on this year’s conference season. We have partnered with organizations such as O’Reilly Media, Dataversity, and the Open Data Science Conference. Upcoming events include the O’Reilly AI Conference, the Strata Data Conference, and the combined events of the Data Architecture Summit and Graphorum. Go to dataengineeringpodcast.com/conferences to learn more and take advantage of our partner discounts when you register.
Go to dataengineeringpodcast.com to subscribe to the show, sign up for the mailing list, read the show notes, and get in touch.
To help other people find the show please leave a review on iTunes and tell your friends and co-workers
Join the community in the new Zulip chat workspace at dataengineeringpodcast.com/chat
Your host is Tobias Macey and today I’m interviewing Tim Ward about his thoughts on eventual connectivity as a new pattern to replace traditional ETL
Interview
Introduction
How did you get involved in the area of data management?
Can you start by discussing the challenges and shortcomings that you perceive in the existing practices of ETL?
What is eventual connectivity and how does it address the problems with ETL in the current data landscape?
In your white paper you mention the benefits of graph technology and how it solves the problem of data integration. Can you talk through an example use case?
How do different implementations of graph databases impact their viability for this use case?
Can you talk through the overall system architecture and data flow for an example implementation of eventual connectivity?
How much up-front modeling is necessary to make this a viable approach to data integration?
How do the volume and format of the source data impact the technology and architecture decisions that you would make?
What are the limitations or edge cases that you have found when using this pattern?
In modern ETL architectures there has been a lot of time and work put into workflow management systems for orchestrating data flows. Is there still a place for those tools when using the eventual connectivity pattern?
What resources do you recommend for someone who wants to learn more about this approach and start using it in their organization?
Contact Info
Email
LinkedIn
@jerrong on Twitter
Parting Question
From your perspective, what is the biggest gap in the tooling or technology for data management today?
Links
Eventual Connectivity White Paper
CluedIn
Podcast Episode
Copenhagen
Ewok
Multivariate Testing
CRM
ERP
ETL
ELT
DAG
Graph Database
Apache NiFi
Podcast Episode
Apache Airflow
Podcast.init Episode
BigQuery
RedShift
CosmosDB
SAP HANA
IOT == Internet of Things
The intro and outro music is from The Hug by The Freak Fandango Orchestra / CC BY-SA
Support Data Engineering Podcast
53:4729/07/2019
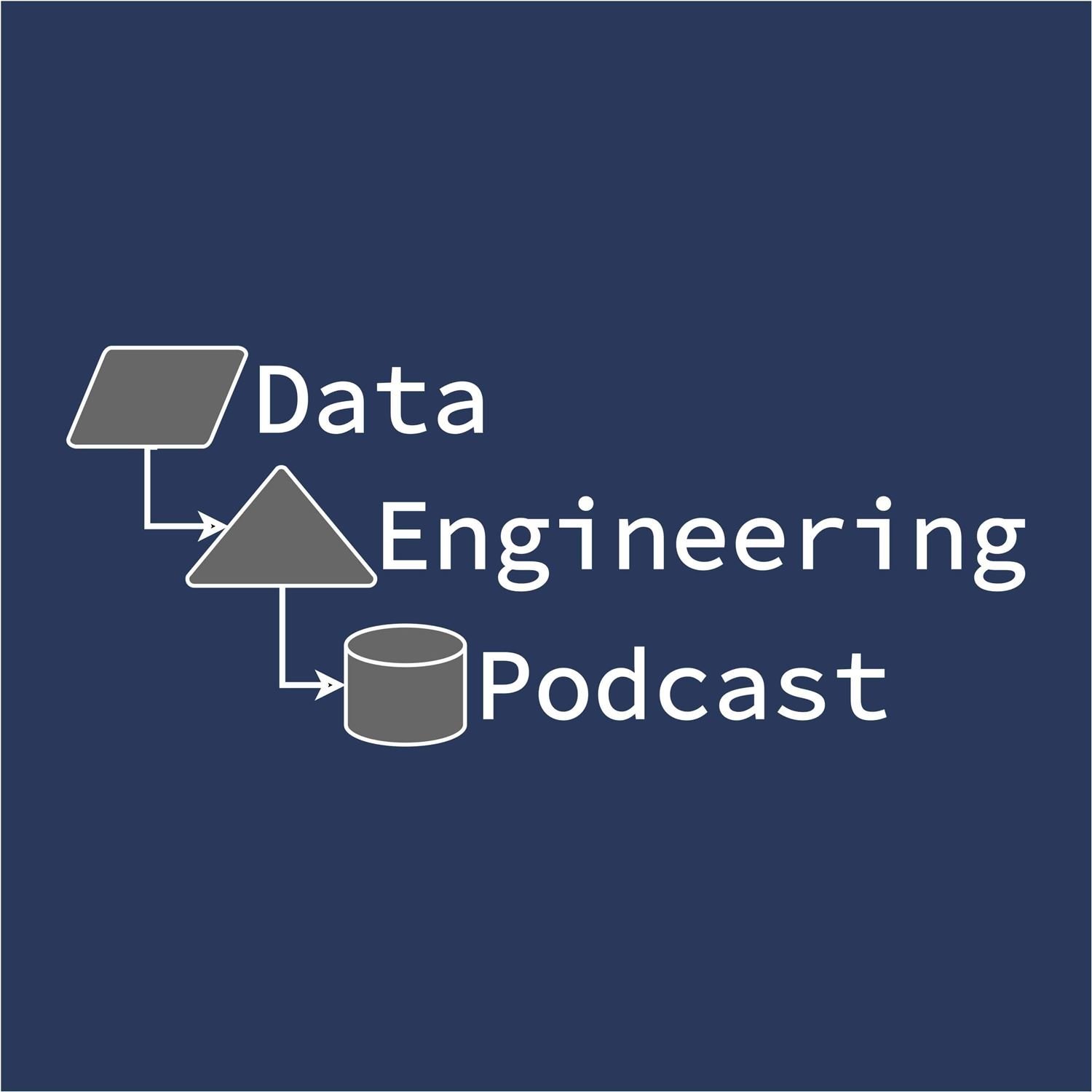
Straining Your Data Lake Through A Data Mesh
Summary
The current trend in data management is to centralize the responsibilities of storing and curating the organization’s information to a data engineering team. This organizational pattern is reinforced by the architectural pattern of data lakes as a solution for managing storage and access. In this episode Zhamak Dehghani shares an alternative approach in the form of a data mesh. Rather than connecting all of your data flows to one destination, empower your individual business units to create data products that can be consumed by other teams. This was an interesting exploration of a different way to think about the relationship between how your data is produced, how it is used, and how to build a technical platform that supports the organizational needs of your business.
Announcements
Hello and welcome to the Data Engineering Podcast, the show about modern data management
When you’re ready to build your next pipeline, or want to test out the projects you hear about on the show, you’ll need somewhere to deploy it, so check out our friends at Linode. With 200Gbit private networking, scalable shared block storage, and a 40Gbit public network, you’ve got everything you need to run a fast, reliable, and bullet-proof data platform. If you need global distribution, they’ve got that covered too with world-wide datacenters including new ones in Toronto and Mumbai. And for your machine learning workloads, they just announced dedicated CPU instances. Go to dataengineeringpodcast.com/linode today to get a $20 credit and launch a new server in under a minute. And don’t forget to thank them for their continued support of this show!
And to grow your professional network and find opportunities with the startups that are changing the world then Angel List is the place to go. Go to dataengineeringpodcast.com/angel to sign up today.
You listen to this show to learn and stay up to date with what’s happening in databases, streaming platforms, big data, and everything else you need to know about modern data management.For even more opportunities to meet, listen, and learn from your peers you don’t want to miss out on this year’s conference season. We have partnered with organizations such as O’Reilly Media, Dataversity, and the Open Data Science Conference. Upcoming events include the O’Reilly AI Conference, the Strata Data Conference, and the combined events of the Data Architecture Summit and Graphorum. Go to dataengineeringpodcast.com/conferences to learn more and take advantage of our partner discounts when you register.
Go to dataengineeringpodcast.com to subscribe to the show, sign up for the mailing list, read the show notes, and get in touch.
To help other people find the show please leave a review on iTunes and tell your friends and co-workers
Join the community in the new Zulip chat workspace at dataengineeringpodcast.com/chat
Your host is Tobias Macey and today I’m interviewing Zhamak Dehghani about building a distributed data mesh for a domain oriented approach to data management
Interview
Introduction
How did you get involved in the area of data management?
Can you start by providing your definition of a "data lake" and discussing some of the problems and challenges that they pose?
What are some of the organizational and industry trends that tend to lead to this solution?
You have written a detailed post outlining the concept of a "data mesh" as an alternative to data lakes. Can you give a summary of what you mean by that phrase?
In a domain oriented data model, what are some useful methods for determining appropriate boundaries for the various data products?
What are some of the challenges that arise in this data mesh approach and how do they compare to those of a data lake?
One of the primary complications of any data platform, whether distributed or monolithic, is that of discoverability. How do you approach that in a data mesh scenario?
A corollary to the issue of discovery is that of access and governance. What are some strategies to making that scalable and maintainable across different data products within an organization?
Who is responsible for implementing and enforcing compliance regimes?
One of the intended benefits of data lakes is the idea that data integration becomes easier by having everything in one place. What has been your experience in that regard?
How do you approach the challenge of data integration in a domain oriented approach, particularly as it applies to aspects such as data freshness, semantic consistency, and schema evolution?
Has latency of data retrieval proven to be an issue in your work?
When it comes to the actual implementation of a data mesh, can you describe the technical and organizational approach that you recommend?
How do team structures and dynamics shift in this scenario?
What are the necessary skills for each team?
Who is responsible for the overall lifecycle of the data in each domain, including modeling considerations and application design for how the source data is generated and captured?
Is there a general scale of organization or problem domain where this approach would generate too much overhead and maintenance burden?
For an organization that has an existing monolothic architecture, how do you suggest they approach decomposing their data into separately managed domains?
Are there any other architectural considerations that data professionals should be considering that aren’t yet widespread?
Contact Info
LinkedIn
@zhamakd on Twitter
Parting Question
From your perspective, what is the biggest gap in the tooling or technology for data management today?
Links
How to Move Beyond a Monolithic Data Lake to a Distributed Data Mesh
Thoughtworks
Technology Radar
Data Lake
Data Warehouse
James Dixon
Azure Data Lake
"Big Ball Of Mud" Anti-Pattern
ETL
ELT
Hadoop
Spark
Kafka
Event Sourcing
Airflow
Podcast.__init__ Episode
Data Engineering Episode
Data Catalog
Master Data Management
Podcast Episode
Polyseme
REST
CNCF (Cloud Native Computing Foundation)
Cloud Events Standard
The intro and outro music is from The Hug by The Freak Fandango Orchestra / CC BY-SA
Support Data Engineering Podcast
01:04:2822/07/2019
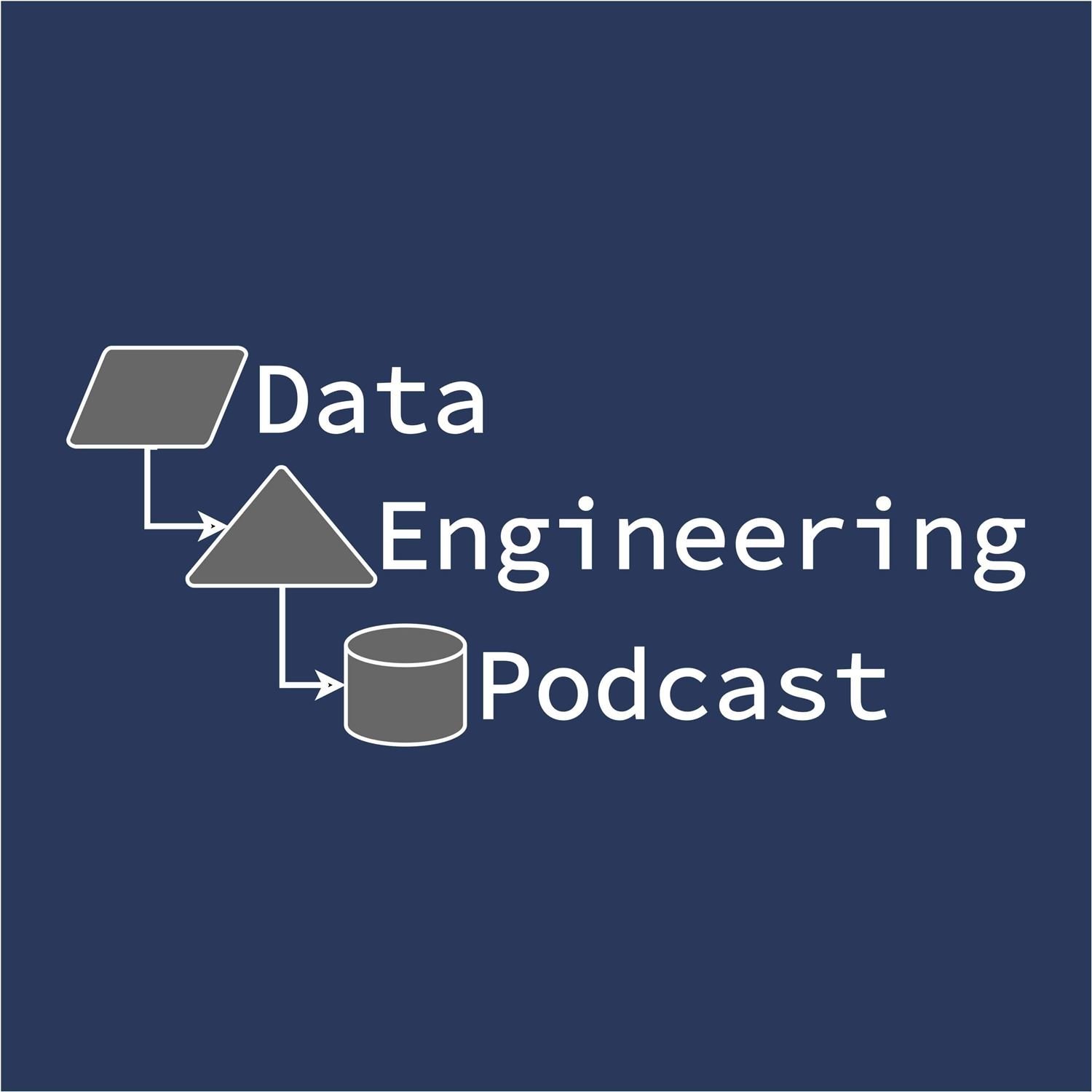
Data Labeling That You Can Feel Good About With CloudFactory
Summary
Successful machine learning and artificial intelligence projects require large volumes of data that is properly labelled. The challenge is that most data is not clean and well annotated, requiring a scalable data labeling process. Ideally this process can be done using the tools and systems that already power your analytics, rather than sending data into a black box. In this episode Mark Sears, CEO of CloudFactory, explains how he and his team built a platform that provides valuable service to businesses and meaningful work to developing nations. He shares the lessons learned in the early years of growing the business, the strategies that have allowed them to scale and train their workforce, and the benefits of working within their customer’s existing platforms. He also shares some valuable insights into the current state of the art for machine learning in the real world.
Announcements
Hello and welcome to the Data Engineering Podcast, the show about modern data management
When you’re ready to build your next pipeline, or want to test out the projects you hear about on the show, you’ll need somewhere to deploy it, so check out our friends at Linode. With 200Gbit private networking, scalable shared block storage, and a 40Gbit public network, you’ve got everything you need to run a fast, reliable, and bullet-proof data platform. If you need global distribution, they’ve got that covered too with world-wide datacenters including new ones in Toronto and Mumbai. And for your machine learning workloads, they just announced dedicated CPU instances. Go to dataengineeringpodcast.com/linode today to get a $20 credit and launch a new server in under a minute. And don’t forget to thank them for their continued support of this show!
Integrating data across the enterprise has been around for decades – so have the techniques to do it. But, a new way of integrating data and improving streams has evolved. By integrating each silo independently – data is able to integrate without any direct relation. At CluedIn they call it “eventual connectivity”. If you want to learn more on how to deliver fast access to your data across the enterprise leveraging this new method, and the technologies that make it possible, get a demo or presentation of the CluedIn Data Hub by visiting dataengineeringpodcast.com/cluedin. And don’t forget to thank them for supporting the show!
You listen to this show to learn and stay up to date with what’s happening in databases, streaming platforms, big data, and everything else you need to know about modern data management.For even more opportunities to meet, listen, and learn from your peers you don’t want to miss out on this year’s conference season. We have partnered with organizations such as O’Reilly Media, Dataversity, and the Open Data Science Conference. Coming up this fall is the combined events of Graphorum and the Data Architecture Summit. The agendas have been announced and super early bird registration for up to $300 off is available until July 26th, with early bird pricing for up to $200 off through August 30th. Use the code BNLLC to get an additional 10% off any pass when you register. Go to dataengineeringpodcast.com/conferences to learn more and take advantage of our partner discounts when you register.
Go to dataengineeringpodcast.com to subscribe to the show, sign up for the mailing list, read the show notes, and get in touch.
To help other people find the show please leave a review on iTunes and tell your friends and co-workers
Join the community in the new Zulip chat workspace at dataengineeringpodcast.com/chat
Your host is Tobias Macey and today I’m interviewing Mark Sears about Cloud Factory, masters of the art and science of labeling data for Machine Learning and more
Interview
Introduction
How did you get involved in the area of data management?
Can you start by explaining what CloudFactory is and the story behind it?
What are some of the common requirements for feature extraction and data labelling that your customers contact you for?
What integration points do you provide to your customers and what is your strategy for ensuring broad compatibility with their existing tools and workflows?
Can you describe the workflow for a sample request from a customer, how that fans out to your cloud workers, and the interface or platform that they are working with to deliver the labelled data?
What protocols do you have in place to ensure data quality and identify potential sources of bias?
What role do humans play in the lifecycle for AI and ML projects?
I understand that you provide skills development and community building for your cloud workers. Can you talk through your relationship with those employees and how that relates to your business goals?
How do you manage and plan for elasticity in customer needs given the workforce requirements that you are dealing with?
Can you share some stories of cloud workers who have benefited from their experience working with your company?
What are some of the assumptions that you made early in the founding of your business which have been challenged or updated in the process of building and scaling CloudFactory?
What have been some of the most interesting/unexpected ways that you have seen customers using your platform?
What lessons have you learned in the process of building and growing CloudFactory that were most interesting/unexpected/useful?
What are your thoughts on the future of work as AI and other digital technologies continue to disrupt existing industries and jobs?
How does that tie into your plans for CloudFactory in the medium to long term?
Contact Info
@marktsears on Twitter
LinkedIn
Parting Question
From your perspective, what is the biggest gap in the tooling or technology for data management today?
Links
CloudFactory
Reading, UK
Nepal
Kenya
Ruby on Rails
Kathmandu
Natural Language Processing (NLP)
Computer Vision
The intro and outro music is from The Hug by The Freak Fandango Orchestra / CC BY-SA
Support Data Engineering Podcast
57:5015/07/2019
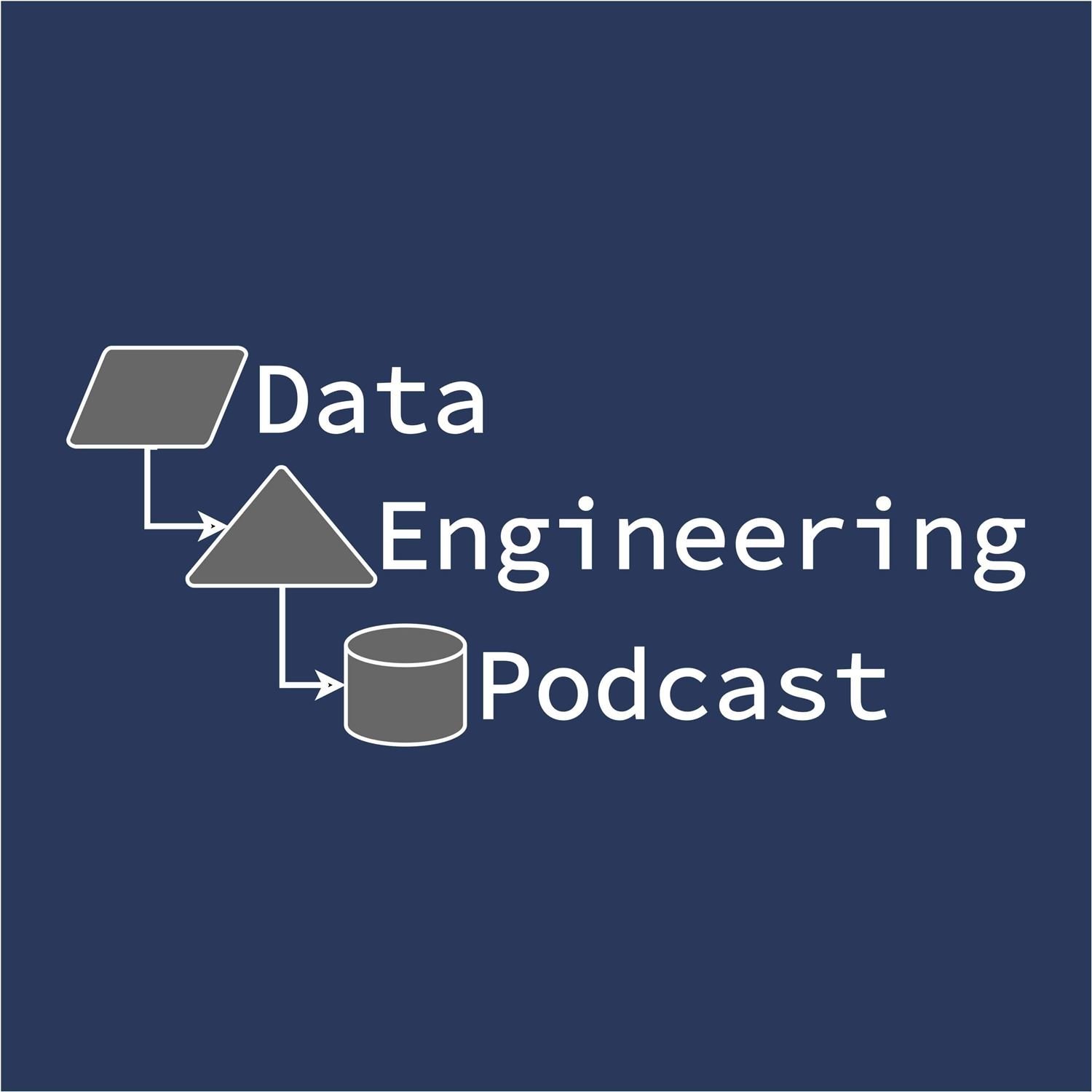
Scale Your Analytics On The Clickhouse Data Warehouse
Summary
The market for data warehouse platforms is large and varied, with options for every use case. ClickHouse is an open source, column-oriented database engine built for interactive analytics with linear scalability. In this episode Robert Hodges and Alexander Zaitsev explain how it is architected to provide these features, the various unique capabilities that it provides, and how to run it in production. It was interesting to learn about some of the custom data types and performance optimizations that are included.
Announcements
Hello and welcome to the Data Engineering Podcast, the show about modern data management
When you’re ready to build your next pipeline, or want to test out the projects you hear about on the show, you’ll need somewhere to deploy it, so check out our friends at Linode. With 200Gbit private networking, scalable shared block storage, and a 40Gbit public network, you’ve got everything you need to run a fast, reliable, and bullet-proof data platform. If you need global distribution, they’ve got that covered too with world-wide datacenters including new ones in Toronto and Mumbai. And for your machine learning workloads, they just announced dedicated CPU instances. Go to dataengineeringpodcast.com/linode today to get a $20 credit and launch a new server in under a minute. And don’t forget to thank them for their continued support of this show!
Integrating data across the enterprise has been around for decades – so have the techniques to do it. But, a new way of integrating data and improving streams has evolved. By integrating each silo independently – data is able to integrate without any direct relation. At CluedIn they call it “eventual connectivity”. If you want to learn more on how to deliver fast access to your data across the enterprise leveraging this new method, and the technologies that make it possible, get a demo or presentation of the CluedIn Data Hub by visiting dataengineeringpodcast.com/cluedin. And don’t forget to thank them for supporting the show!
You listen to this show to learn and stay up to date with what’s happening in databases, streaming platforms, big data, and everything else you need to know about modern data management.For even more opportunities to meet, listen, and learn from your peers you don’t want to miss out on this year’s conference season. We have partnered with organizations such as O’Reilly Media, Dataversity, and the Open Data Science Conference. Coming up this fall is the combined events of Graphorum and the Data Architecture Summit. The agendas have been announced and super early bird registration for up to $300 off is available until July 26th, with early bird pricing for up to $200 off through August 30th. Use the code BNLLC to get an additional 10% off any pass when you register. Go to dataengineeringpodcast.com/conferences to learn more and take advantage of our partner discounts when you register.
Go to dataengineeringpodcast.com to subscribe to the show, sign up for the mailing list, read the show notes, and get in touch.
To help other people find the show please leave a review on iTunes and tell your friends and co-workers
Join the community in the new Zulip chat workspace at dataengineeringpodcast.com/chat
Your host is Tobias Macey and today I’m interviewing Robert Hodges and Alexander Zaitsev about Clickhouse, an open source, column-oriented database for fast and scalable OLAP queries
Interview
Introduction
How did you get involved in the area of data management?
Can you start by explaining what Clickhouse is and how you each got involved with it?
What are the primary use cases that Clickhouse is targeting?
Where does it fit in the database market and how does it compare to other column stores, both open source and commercial?
Can you describe how Clickhouse is architected?
Can you talk through the lifecycle of a given record or set of records from when they first get inserted into Clickhouse, through the engine and storage layer, and then the lookup process at query time?
I noticed that Clickhouse has a feature for implementing data safeguards (deletion protection, etc.). Can you talk through how that factors into different use cases for Clickhouse?
Aside from directly inserting a record via the client APIs can you talk through the options for loading data into Clickhouse?
For the MySQL/Postgres replication functionality how do you maintain schema evolution from the source DB to Clickhouse?
What are some of the advanced capabilities, such as SQL extensions, supported data types, etc. that are unique to Clickhouse?
For someone getting started with Clickhouse can you describe how they should be thinking about data modeling?
Recent entrants to the data warehouse market are encouraging users to insert raw, unprocessed records and then do their transformations with the database engine, as opposed to using a data lake as the staging ground for transformations prior to loading into the warehouse. Where does Clickhouse fall along that spectrum?
How is scaling in Clickhouse implemented and what are the edge cases that users should be aware of?
How is data replication and consistency managed?
What is involved in deploying and maintaining an installation of Clickhouse?
I noticed that Altinity is providing a Kubernetes operator for Clickhouse. What are the opportunities and tradeoffs presented by that platform for Clickhouse?
What are some of the most interesting/unexpected/innovative ways that you have seen Clickhouse used?
What are some of the most challenging aspects of working on Clickhouse itself, and or implementing systems on top of it?
What are the shortcomings of Clickhouse and how do you address them at Altinity?
When is Clickhouse the wrong choice?
Contact Info
Robert
LinkedIn
hodgesrm on GitHub
Alexander
alex-zaitsev on GitHub
LinkedIn
Parting Question
From your perspective, what is the biggest gap in the tooling or technology for data management today?
Links
Clickhouse
Altinity
OLAP
M204
Sybase
MySQL
Vertica
Yandex
Yandex Metrica
Google Analytics
SQL
Greenplum
InfoBright
InfiniDB
MariaDB
Spark
SIMD (Single Instruction, Multiple Data)
Mergesort
ETL
Change Data Capture
MapReduce
KDB
OLTP
Cassandra
InfluxDB
Prometheus
SnowflakeDB
Hive
Hadoop
The intro and outro music is from The Hug by The Freak Fandango Orchestra / CC BY-SA
Support Data Engineering Podcast
01:11:1908/07/2019
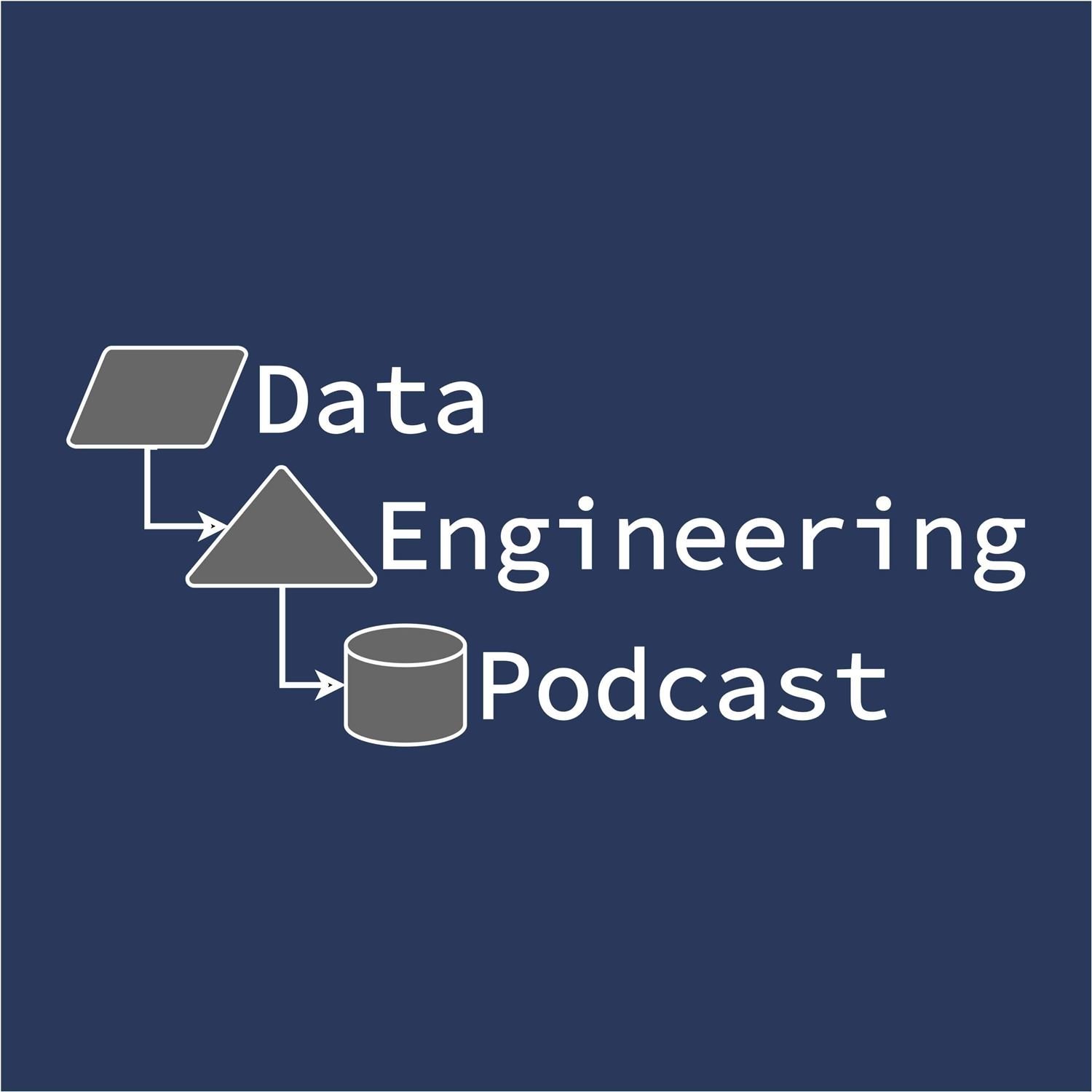
Stress Testing Kafka And Cassandra For Real-Time Anomaly Detection
Summary
Anomaly detection is a capability that is useful in a variety of problem domains, including finance, internet of things, and systems monitoring. Scaling the volume of events that can be processed in real-time can be challenging, so Paul Brebner from Instaclustr set out to see how far he could push Kafka and Cassandra for this use case. In this interview he explains the system design that he tested, his findings for how these tools were able to work together, and how they behaved at different orders of scale. It was an interesting conversation about how he stress tested the Instaclustr managed service for benchmarking an application that has real-world utility.
Announcements
Hello and welcome to the Data Engineering Podcast, the show about modern data management
When you’re ready to build your next pipeline, or want to test out the projects you hear about on the show, you’ll need somewhere to deploy it, so check out our friends at Linode. With 200Gbit private networking, scalable shared block storage, and a 40Gbit public network, you’ve got everything you need to run a fast, reliable, and bullet-proof data platform. If you need global distribution, they’ve got that covered too with world-wide datacenters including new ones in Toronto and Mumbai. And for your machine learning workloads, they just announced dedicated CPU instances. Go to dataengineeringpodcast.com/linode today to get a $20 credit and launch a new server in under a minute. And don’t forget to thank them for their continued support of this show!
Integrating data across the enterprise has been around for decades – so have the techniques to do it. But, a new way of integrating data and improving streams has evolved. By integrating each silo independently – data is able to integrate without any direct relation. At CluedIn they call it “eventual connectivity”. If you want to learn more on how to deliver fast access to your data across the enterprise leveraging this new method, and the technologies that make it possible, get a demo or presentation of the CluedIn Data Hub by visiting dataengineeringpodcast.com/cluedin. And don’t forget to thank them for supporting the show!
You listen to this show to learn and stay up to date with what’s happening in databases, streaming platforms, big data, and everything else you need to know about modern data management.For even more opportunities to meet, listen, and learn from your peers you don’t want to miss out on this year’s conference season. We have partnered with organizations such as O’Reilly Media, Dataversity, and the Open Data Science Conference. Coming up this fall is the combined events of Graphorum and the Data Architecture Summit. The agendas have been announced and super early bird registration for up to $300 off is available until July 26th, with early bird pricing for up to $200 off through August 30th. Use the code BNLLC to get an additional 10% off any pass when you register. Go to dataengineeringpodcast.com/conferences to learn more and take advantage of our partner discounts when you register.
Go to dataengineeringpodcast.com to subscribe to the show, sign up for the mailing list, read the show notes, and get in touch.
To help other people find the show please leave a review on iTunes and tell your friends and co-workers
Join the community in the new Zulip chat workspace at dataengineeringpodcast.com/chat
Your host is Tobias Macey and today I’m interviewing Paul Brebner about his experience designing and building a scalable, real-time anomaly detection system using Kafka and Cassandra
Interview
Introduction
How did you get involved in the area of data management?
Can you start by describing the problem that you were trying to solve and the requirements that you were aiming for?
What are some example cases where anomaly detection is useful or necessary?
Once you had established the requirements in terms of functionality and data volume, what was your approach for determining the target architecture?
What was your selection criteria for the various components of your system design?
What tools and technologies did you consider in your initial assessment and which did you ultimately converge on?
If you were to start over today would you do any of it differently?
Can you talk through the algorithm that you used for detecting anomalous activity?
What is the size/duration of the window within which you can effectively characterize trends and how do you collapse it down to a tractable search space?
What were you using as a data source, and if it was synthetic how did you handle introducing anomalies in a realistic fashion?
What were the main scalability bottlenecks that you encountered as you began ramping up the volume of data and the number of instances?
How did those bottlenecks differ as you moved through different levels of scale?
What were your assumptions going into this project and how accurate were they as you began testing and scaling the system that you built?
What were some of the most interesting or unexpected lessons that you learned in the process of building this anomaly detection system?
How have those lessons fed back to your work at Instaclustr?
Contact Info
LinkedIn
@paulbrebner_ on Twitter
Parting Question
From your perspective, what is the biggest gap in the tooling or technology for data management today?
Links
Instaclustr
Kafka
Cassandra
Canberra, Australia
Spark
Anomaly Detection
Kubernetes
Prometheus
OpenTracing
Jaeger
The intro and outro music is from The Hug by The Freak Fandango Orchestra / CC BY-SA
Support Data Engineering Podcast
38:0302/07/2019
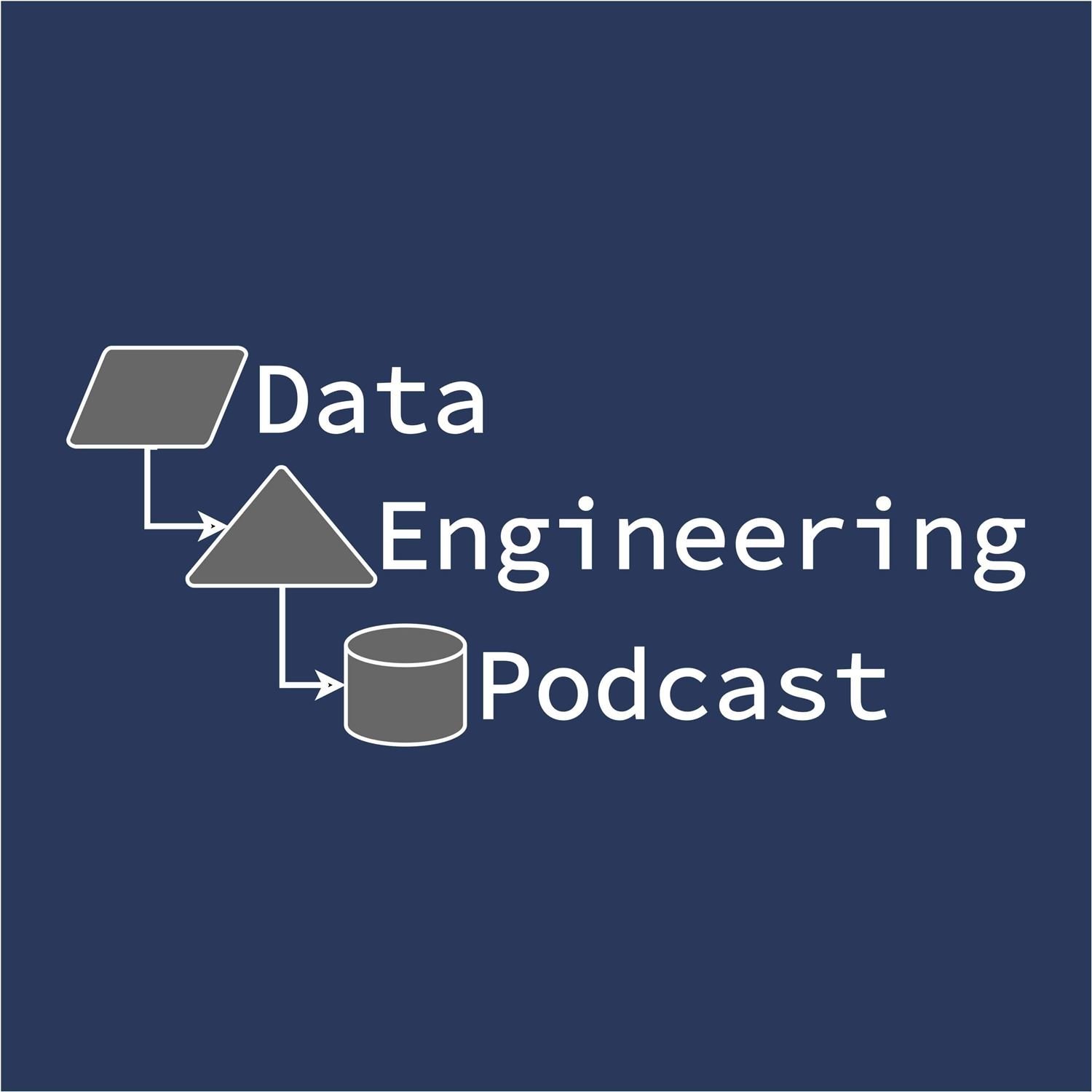
The Workflow Engine For Data Engineers And Data Scientists
Summary
Building a data platform that works equally well for data engineering and data science is a task that requires familiarity with the needs of both roles. Data engineering platforms have a strong focus on stateful execution and tasks that are strictly ordered based on dependency graphs. Data science platforms provide an environment that is conducive to rapid experimentation and iteration, with data flowing directly between stages. Jeremiah Lowin has gained experience in both styles of working, leading him to be frustrated with all of the available tools. In this episode he explains his motivation for creating a new workflow engine that marries the needs of data engineers and data scientists, how it helps to smooth the handoffs between teams working on data projects, and how the design lets you focus on what you care about while it handles the failure cases for you. It is exciting to see a new generation of workflow engine that is learning from the benefits and failures of previous tools for processing your data pipelines.
Announcements
Hello and welcome to the Data Engineering Podcast, the show about modern data management
When you’re ready to build your next pipeline, or want to test out the projects you hear about on the show, you’ll need somewhere to deploy it, so check out our friends at Linode. With 200Gbit private networking, scalable shared block storage, and a 40Gbit public network, you’ve got everything you need to run a fast, reliable, and bullet-proof data platform. If you need global distribution, they’ve got that covered too with world-wide datacenters including new ones in Toronto and Mumbai. And for your machine learning workloads, they just announced dedicated CPU instances. Go to dataengineeringpodcast.com/linode today to get a $20 credit and launch a new server in under a minute. And don’t forget to thank them for their continued support of this show!
You listen to this show to learn and stay up to date with what’s happening in databases, streaming platforms, big data, and everything else you need to know about modern data management.For even more opportunities to meet, listen, and learn from your peers you don’t want to miss out on this year’s conference season. We have partnered with organizations such as O’Reilly Media, Dataversity, and the Open Data Science Conference. Coming up this fall is the combined events of Graphorum and the Data Architecture Summit. The agendas have been announced and super early bird registration for up to $300 off is available until July 26th, with early bird pricing for up to $200 off through August 30th. Use the code BNLLC to get an additional 10% off any pass when you register. Go to dataengineeringpodcast.com/conferences to learn more and take advantage of our partner discounts when you register.
Go to dataengineeringpodcast.com to subscribe to the show, sign up for the mailing list, read the show notes, and get in touch.
To help other people find the show please leave a review on iTunes and tell your friends and co-workers
Join the community in the new Zulip chat workspace at dataengineeringpodcast.com/chat
Your host is Tobias Macey and today I’m interviewing Jeremiah Lowin about Prefect, a workflow platform for data engineering
Interview
Introduction
How did you get involved in the area of data management?
Can you start by explaining what Prefect is and your motivation for creating it?
What are the axes along which a workflow engine can differentiate itself, and which of those have you focused on for Prefect?
In some of your blog posts and your PyData presentation you discuss the concept of negative vs. positive engineering. Can you briefly outline what you mean by that and the ways that Prefect handles the negative cases for you?
How is Prefect itself implemented and what tools or systems have you relied on most heavily for inspiration?
How do you manage passing data between stages in a pipeline when they are running across distributed nodes?
What was your decision making process when deciding to use Dask as your supported execution engine?
For tasks that require specific resources or dependencies how do you approach the idea of task affinity?
Does Prefect support managing tasks that bridge network boundaries?
What are some of the features or capabilities of Prefect that are misunderstood or overlooked by users which you think should be exercised more often?
What are the limitations of the open source core as compared to the cloud offering that you are building?
What were your assumptions going into this project and how have they been challenged or updated as you dug deeper into the problem domain and received feedback from users?
What are some of the most interesting/innovative/unexpected ways that you have seen Prefect used?
When is Prefect the wrong choice?
In your experience working on Airflow and Prefect, what are some of the common challenges and anti-patterns that arise in data engineering projects?
What are some best practices and industry trends that you are most excited by?
What do you have planned for the future of the Prefect project and company?
Contact Info
LinkedIn
@jlowin on Twitter
Parting Question
From your perspective, what is the biggest gap in the tooling or technology for data management today?
Links
Prefect
Airflow
Dask
Podcast Episode
Prefect Blog
PyData Presentation
Tensorflow
Workflow Engine
The intro and outro music is from The Hug by The Freak Fandango Orchestra / CC BY-SA
Support Data Engineering Podcast
01:08:2625/06/2019
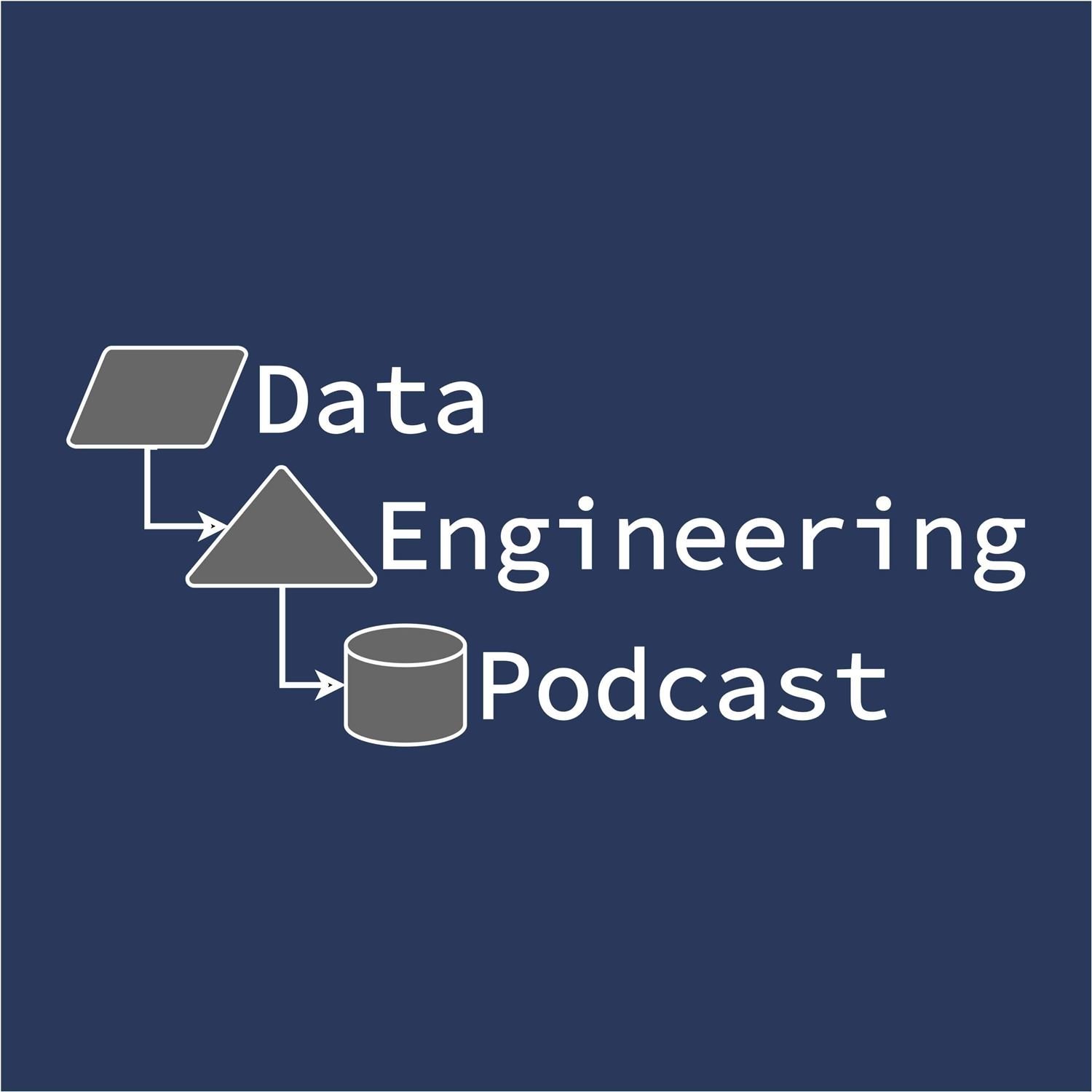
Maintaining Your Data Lake At Scale With Spark
Summary
Building and maintaining a data lake is a choose your own adventure of tools, services, and evolving best practices. The flexibility and freedom that data lakes provide allows for generating significant value, but it can also lead to anti-patterns and inconsistent quality in your analytics. Delta Lake is an open source, opinionated framework built on top of Spark for interacting with and maintaining data lake platforms that incorporates the lessons learned at DataBricks from countless customer use cases. In this episode Michael Armbrust, the lead architect of Delta Lake, explains how the project is designed, how you can use it for building a maintainable data lake, and some useful patterns for progressively refining the data in your lake. This conversation was useful for getting a better idea of the challenges that exist in large scale data analytics, and the current state of the tradeoffs between data lakes and data warehouses in the cloud.
Announcements
Hello and welcome to the Data Engineering Podcast, the show about modern data management
When you’re ready to build your next pipeline, or want to test out the projects you hear about on the show, you’ll need somewhere to deploy it, so check out our friends at Linode. With 200Gbit private networking, scalable shared block storage, and a 40Gbit public network, you’ve got everything you need to run a fast, reliable, and bullet-proof data platform. If you need global distribution, they’ve got that covered too with world-wide datacenters including new ones in Toronto and Mumbai. And for your machine learning workloads, they just announced dedicated CPU instances. Go to dataengineeringpodcast.com/linode today to get a $20 credit and launch a new server in under a minute. And don’t forget to thank them for their continued support of this show!
And to keep track of how your team is progressing on building new pipelines and tuning their workflows, you need a project management system designed by engineers, for engineers. Clubhouse lets you craft a workflow that fits your style, including per-team tasks, cross-project epics, a large suite of pre-built integrations, and a simple API for crafting your own. With such an intuitive tool it’s easy to make sure that everyone in the business is on the same page. Data Engineering Podcast listeners get 2 months free on any plan by going to dataengineeringpodcast.com/clubhouse today and signing up for a free trial. Support the show and get your data projects in order!
You listen to this show to learn and stay up to date with what’s happening in databases, streaming platforms, big data, and everything else you need to know about modern data management. For even more opportunities to meet, listen, and learn from your peers you don’t want to miss out on this year’s conference season. We have partnered with organizations such as O’Reilly Media, Dataversity, and the Open Data Science Conference. Coming up this fall is the combined events of Graphorum and the Data Architecture Summit. The agendas have been announced and super early bird registration for up to $300 off is available until July 26th, with early bird pricing for up to $200 off through August 30th. Use the code BNLLC to get an additional 10% off any pass when you register. Go to dataengineeringpodcast.com/conferences to learn more and take advantage of our partner discounts when you register.
Go to dataengineeringpodcast.com to subscribe to the show, sign up for the mailing list, read the show notes, and get in touch.
To help other people find the show please leave a review on iTunes and tell your friends and co-workers
Join the community in the new Zulip chat workspace at dataengineeringpodcast.com/chat
Your host is Tobias Macey and today I’m interviewing Michael Armbrust about Delta Lake, an open source storage layer that brings ACID transactions to Apache Spark and big data workloads.
Interview
Introduction
How did you get involved in the area of data management?
Can you start by explaining what Delta Lake is and the motivation for creating it?
What are some of the common antipatterns in data lake implementations and how does Delta Lake address them?
What are the benefits of a data lake over a data warehouse?
How has that equation changed in recent years with the availability of modern cloud data warehouses?
How is Delta lake implemented and how has the design evolved since you first began working on it?
What assumptions did you have going into the project and how have they been challenged as it has gained users?
One of the compelling features is the option for enforcing data quality constraints. Can you talk through how those are defined and tested?
In your experience, how do you manage schema evolution when working with large volumes of data? (e.g. rewriting all of the old files, or just eliding the missing columns/populating default values, etc.)
Can you talk through how Delta Lake manages transactionality and data ownership? (e.g. what if you have other services interacting with the data store)
Are there limits in terms of the volume of data that can be managed within a single transaction?
How does unifying the interface for Spark to interact with batch and streaming data sets simplify the workflow for an end user?
The Lambda architecture was popular in the early days of Hadoop but seems to have fallen out of favor. How does this unified interface resolve the shortcomings and complexities of that approach?
What have been the most difficult/complex/challenging aspects of building Delta Lake?
How is the data versioning in Delta Lake implemented?
By keeping a copy of all iterations of a data set there is the opportunity for a great deal of additional cost. What are some options for mitigating that impact, either in Delta Lake itself or as a separate mechanism or process?
What are the reasons for standardizing on Parquet as the storage format?
What are some of the cases where that has led to greater complications?
In addition to the transactionality and data validation that Delta Lake provides, can you also explain how indexing is implemented and highlight the challenges of keeping them up to date?
When is Delta Lake the wrong choice?
What problems did you consciously decide not to address?
What is in store for the future of Delta Lake?
Contact Info
LinkedIn
@michaelarmbrust on Twitter
marmbrus on GitHub
Parting Question
From your perspective, what is the biggest gap in the tooling or technology for data management today?
Links
Delta Lake
DataBricks
Spark SQL
Microsoft SQL Server
Databricks Delta
Spark Summit
Apache Spark
Enterprise Data Curation Episode
Data Lake
Data Warehouse
SnowflakeDB
BigQuery
Parquet
Data Serialization Episode
Hive Metastore
Great Expectations
Podcast.__init__ Interview
Optimistic Concurrency/Optimistic Locking
Presto
Starburst Labs
Podcast Interview
Apache NiFi
Podcast Interview
Tensorflow
Tableau
Change Data Capture
Apache Pulsar
Podcast Interview
Pravega
Podcast Interview
Multi-Version Concurrency Control
MLFlow
Avro
ORC
The intro and outro music is from The Hug by The Freak Fandango Orchestra / CC BY-SA
Support Data Engineering Podcast
50:5017/06/2019
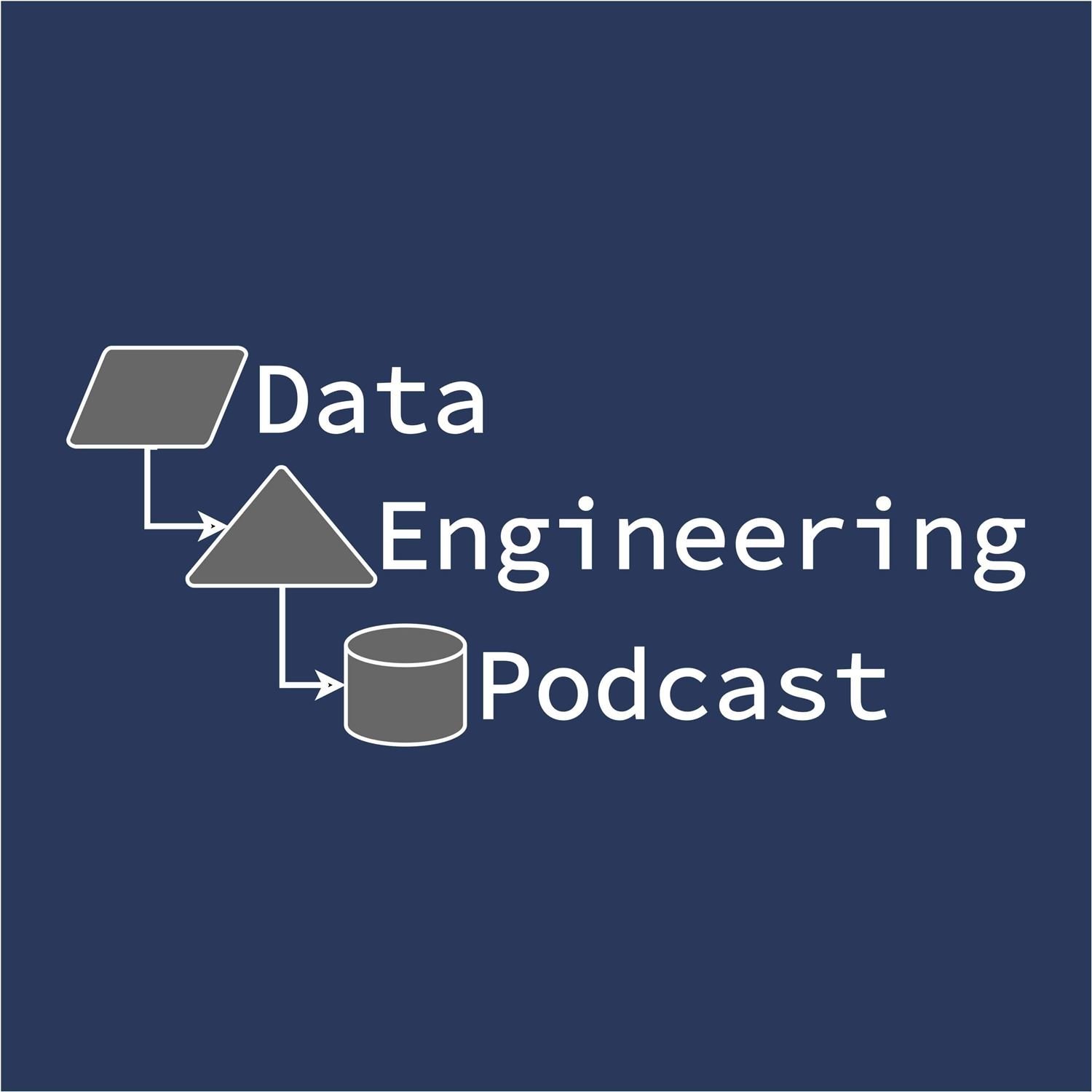
Managing The Machine Learning Lifecycle
Summary
Building a machine learning model can be difficult, but that is only half of the battle. Having a perfect model is only useful if you are able to get it into production. In this episode Stepan Pushkarev, founder of Hydrosphere, explains why deploying and maintaining machine learning projects in production is different from regular software projects and the challenges that they bring. He also describes the Hydrosphere platform, and how the different components work together to manage the full machine learning lifecycle of model deployment and retraining. This was a useful conversation to get a better understanding of the unique difficulties that exist for machine learning projects.
Announcements
Hello and welcome to the Data Engineering Podcast, the show about modern data management
When you’re ready to build your next pipeline, or want to test out the projects you hear about on the show, you’ll need somewhere to deploy it, so check out our friends at Linode. With 200Gbit private networking, scalable shared block storage, and a 40Gbit public network, you’ve got everything you need to run a fast, reliable, and bullet-proof data platform. If you need global distribution, they’ve got that covered too with world-wide datacenters including new ones in Toronto and Mumbai. And for your machine learning workloads, they just announced dedicated CPU instances. Go to dataengineeringpodcast.com/linode today to get a $20 credit and launch a new server in under a minute. And don’t forget to thank them for their continued support of this show!
And to keep track of how your team is progressing on building new pipelines and tuning their workflows, you need a project management system designed by engineers, for engineers. Clubhouse lets you craft a workflow that fits your style, including per-team tasks, cross-project epics, a large suite of pre-built integrations, and a simple API for crafting your own. With such an intuitive tool it’s easy to make sure that everyone in the business is on the same page. Data Engineering Podcast listeners get 2 months free on any plan by going to dataengineeringpodcast.com/clubhouse today and signing up for a free trial. Support the show and get your data projects in order!
You listen to this show to learn and stay up to date with what’s happening in databases, streaming platforms, big data, and everything else you need to know about modern data management. For even more opportunities to meet, listen, and learn from your peers you don’t want to miss out on this year’s conference season. We have partnered with organizations such as O’Reilly Media, Dataversity, and the Open Data Science Conference. Coming up this fall is the combined events of Graphorum and the Data Architecture Summit. The agendas have been announced and super early bird registration for up to $300 off is available until July 26th, with early bird pricing for up to $200 off through August 30th. Use the code BNLLC to get an additional 10% off any pass when you register. Go to dataengineeringpodcast.com/conferences to learn more and take advantage of our partner discounts when you register.
Go to dataengineeringpodcast.com to subscribe to the show, sign up for the mailing list, read the show notes, and get in touch.
To help other people find the show please leave a review on iTunes and tell your friends and co-workers
Join the community in the new Zulip chat workspace at dataengineeringpodcast.com/chat
Your host is Tobias Macey and today I’m interviewing Stepan Pushkarev about Hydrosphere, the first open source platform for Data Science and Machine Learning Management automation
Interview
Introduction
How did you get involved in the area of data management?
Can you start by explaining what Hydrosphere is and share its origin story?
In your experience, what are the most challenging or complicated aspects of managing machine learning models in a production context?
How does it differ from deployment and maintenance of a regular software application?
Can you describe how Hydrosphere is architected and how the different components of the stack fit together?
For someone who is using Hydrosphere in their production workflow, what would that look like?
What is the difference in interaction with Hydrosphere for different roles within a data team?
What are some of the types of metrics that you monitor to determine when and how to retrain deployed models?
Which metrics do you track for testing and verifying the health of the data?
What are the factors that contribute to model degradation in production and how do you incorporate contextual feedback into the training cycle to counteract them?
How has the landscape and sophistication for real world usability of machine learning changed since you first began working on Hydrosphere?
How has that influenced the design and direction of Hydrosphere, both as a project and a business?
How has the design of Hydrosphere evolved since you first began working on it?
What assumptions did you have when you began working on Hydrosphere and how have they been challenged or modified through growing the platform?
What have been some of the most challenging or complex aspects of building and maintaining Hydrosphere?
What do you have in store for the future of Hydrosphere?
Contact Info
LinkedIn
spushkarev on GitHub
Parting Question
From your perspective, what is the biggest gap in the tooling or technology for data management today?
Links
Hydrosphere
GitHub
Data Engineering Podcast at ODSC
KD Nuggets
Big Data Science: Expectation vs. Reality
The Open Data Science Conference
Scala
InfluxDB
RocksDB
Docker
Kubernetes
Akka
Python Pickle
Protocol Buffers
Kubeflow
MLFlow
TensorFlow Extended
Kubeflow Pipelines
Argo
Airflow
Podcast.__init__ Interview
Envoy
Istio
DVC
Podcast.__init__ Interview
Generative Adversarial Networks
The intro and outro music is from The Hug by The Freak Fandango Orchestra / CC BY-SA
Support Data Engineering Podcast
01:02:4010/06/2019
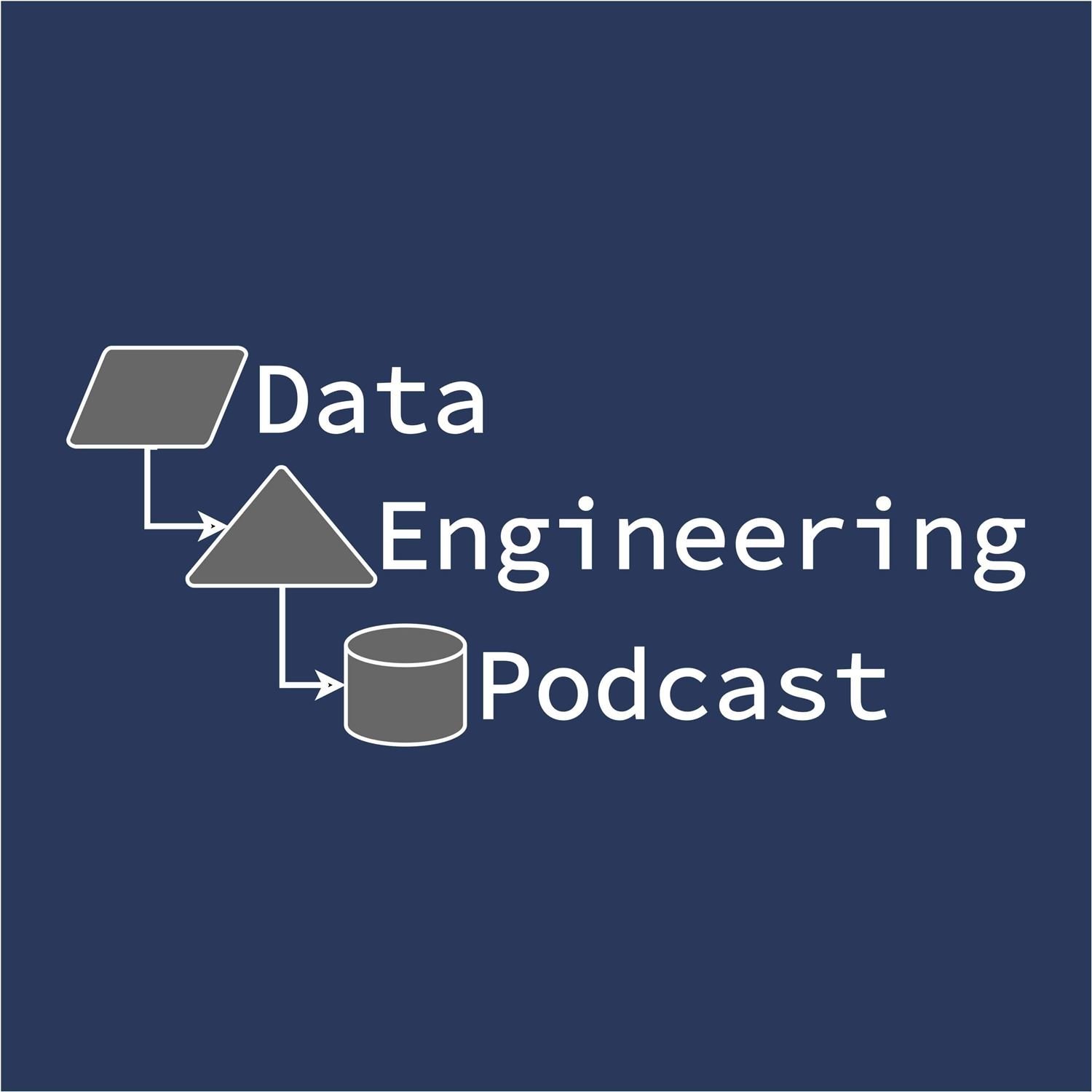
Evolving An ETL Pipeline For Better Productivity
Summary
Building an ETL pipeline can be a significant undertaking, and sometimes it needs to be rebuilt when a better option becomes available. In this episode Aaron Gibralter, director of engineering at Greenhouse, joins Raghu Murthy, founder and CEO of DataCoral, to discuss the journey that he and his team took from an in-house ETL pipeline built out of open source components onto a paid service. He explains how their original implementation was built, why they decided to migrate to a paid service, and how they made that transition. He also discusses how the abstractions provided by DataCoral allows his data scientists to remain productive without requiring dedicated data engineers. If you are either considering how to build a data pipeline or debating whether to migrate your existing ETL to a service this is definitely worth listening to for some perspective.
Announcements
Hello and welcome to the Data Engineering Podcast, the show about modern data management
When you’re ready to build your next pipeline, or want to test out the projects you hear about on the show, you’ll need somewhere to deploy it, so check out our friends at Linode. With 200Gbit private networking, scalable shared block storage, and a 40Gbit public network, you’ve got everything you need to run a fast, reliable, and bullet-proof data platform. If you need global distribution, they’ve got that covered too with world-wide datacenters including new ones in Toronto and Mumbai. And for your machine learning workloads, they just announced dedicated CPU instances. Go to dataengineeringpodcast.com/linode today to get a $20 credit and launch a new server in under a minute. And don’t forget to thank them for their continued support of this show!
And to keep track of how your team is progressing on building new pipelines and tuning their workflows, you need a project management system designed by engineers, for engineers. Clubhouse lets you craft a workflow that fits your style, including per-team tasks, cross-project epics, a large suite of pre-built integrations, and a simple API for crafting your own. With such an intuitive tool it’s easy to make sure that everyone in the business is on the same page. Data Engineering Podcast listeners get 2 months free on any plan by going to dataengineeringpodcast.com/clubhouse today and signing up for a free trial. Support the show and get your data projects in order!
You listen to this show to learn and stay up to date with the ways that Python is being used, including the latest in machine learning and data analysis. For even more opportunities to meet, listen, and learn from your peers you don’t want to miss out on this year’s conference season. We have partnered with organizations such as O’Reilly Media, Dataversity, and the Open Data Science Conference. Coming up this fall is the combined events of Graphorum and the Data Architecture Summit. The agendas have been announced and super early bird registration for up to $300 off is available until July 26th, with early bird pricing for up to $200 off through August 30th. Use the code BNLLC to get an additional 10% off any pass when you register. Go to dataengineeringpodcast.com/conferences to learn more and take advantage of our partner discounts when you register.
You listen to this show to learn and stay up to date with what’s happening in databases, streaming platforms, big data, and everything else you need to know about modern data management. For even more opportunities to meet, listen, and learn from your peers you don’t want to miss out on this year’s conference season. We have partnered with organizations such as O’Reilly Media, Dataversity, and the Open Data Science Conference. Go to dataengineeringpodcast.com/conferences to learn more and take advantage of our partner discounts when you register.
Go to dataengineeringpodcast.com to subscribe to the show, sign up for the mailing list, read the show notes, and get in touch.
To help other people find the show please leave a review on iTunes and tell your friends and co-workers
Join the community in the new Zulip chat workspace at dataengineeringpodcast.com/chat
Your host is Tobias Macey and today I’m interviewing Aaron Gibralter and Raghu Murthy about the experience of Greenhouse migrating their data pipeline to DataCoral
Interview
Introduction
How did you get involved in the area of data management?
Aaron, can you start by describing what Greenhouse is and some of the ways that you use data?
Can you describe your overall data infrastructure and the state of your data pipeline before migrating to DataCoral?
What are your primary sources of data and what are the targets that you are loading them into?
What were your biggest pain points and what motivated you to re-evaluate your approach to ETL?
What were your criteria for your replacement technology and how did you gather and evaluate your options?
Once you made the decision to use DataCoral can you talk through the transition and cut-over process?
What were some of the unexpected edge cases or shortcomings that you experienced when moving to DataCoral?
What were the big wins?
What was your evaluation framework for determining whether your re-engineering was successful?
Now that you are using DataCoral how would you characterize the experiences of yourself and your team?
If you have freed up time for your engineers, how are you allocating that spare capacity?
What do you hope to see from DataCoral in the future?
What advice do you have for anyone else who is either evaluating a re-architecture of their existing data platform or planning out a greenfield project?
Contact Info
Aaron
agribralter on GitHub
LinkedIn
Raghu
LinkedIn
Medium
Parting Question
From your perspective, what is the biggest gap in the tooling or technology for data management today?
Links
Greenhouse
We’re hiring Data Scientists and Software Engineers!
Datacoral
Airflow
Podcast.init Interview
Data Engineering Interview about running Airflow in production
Periscope Data
Mode Analytics
Data Warehouse
ETL
Salesforce
Zendesk
Jira
DataDog
Asana
GDPR
Metabase
Podcast Interview
The intro and outro music is from The Hug by The Freak Fandango Orchestra / CC BY-SA
Support Data Engineering Podcast
01:02:2204/06/2019
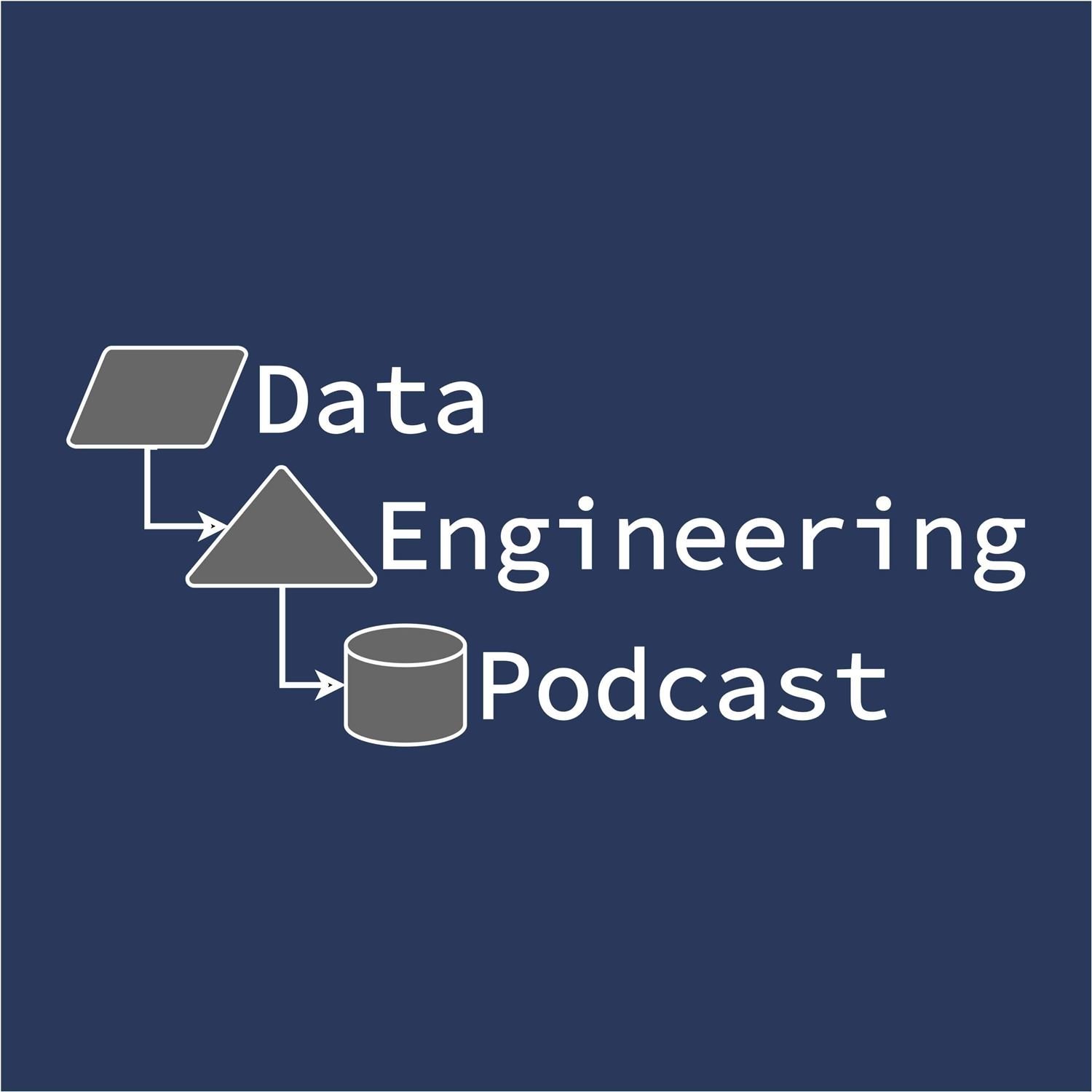
Data Lineage For Your Pipelines
Summary
Some problems in data are well defined and benefit from a ready-made set of tools. For everything else, there’s Pachyderm, the platform for data science that is built to scale. In this episode Joe Doliner, CEO and co-founder, explains how Pachyderm started as an attempt to make data provenance easier to track, how the platform is architected and used today, and examples of how the underlying principles manifest in the workflows of data engineers and data scientists as they collaborate on data projects. In addition to all of that he also shares his thoughts on their recent round of fund-raising and where the future will take them. If you are looking for a set of tools for building your data science workflows then Pachyderm is a solid choice, featuring data versioning, first class tracking of data lineage, and language agnostic data pipelines.
Announcements
Hello and welcome to the Data Engineering Podcast, the show about modern data management
When you’re ready to build your next pipeline, or want to test out the projects you hear about on the show, you’ll need somewhere to deploy it, so check out our friends at Linode. With 200Gbit private networking, scalable shared block storage, and a 40Gbit public network, you’ve got everything you need to run a fast, reliable, and bullet-proof data platform. If you need global distribution, they’ve got that covered too with world-wide datacenters including new ones in Toronto and Mumbai. And for your machine learning workloads, they just announced dedicated CPU instances. Go to dataengineeringpodcast.com/linode today to get a $20 credit and launch a new server in under a minute. And don’t forget to thank them for their continued support of this show!
Alluxio is an open source, distributed data orchestration layer that makes it easier to scale your compute and your storage independently. By transparently pulling data from underlying silos, Alluxio unlocks the value of your data and allows for modern computation-intensive workloads to become truly elastic and flexible for the cloud. With Alluxio, companies like Barclays, JD.com, Tencent, and Two Sigma can manage data efficiently, accelerate business analytics, and ease the adoption of any cloud. Go to dataengineeringpodcast.com/alluxio today to learn more and thank them for their support.
Understanding how your customers are using your product is critical for businesses of any size. To make it easier for startups to focus on delivering useful features Segment offers a flexible and reliable data infrastructure for your customer analytics and custom events. You only need to maintain one integration to instrument your code and get a future-proof way to send data to over 250 services with the flip of a switch. Not only does it free up your engineers’ time, it lets your business users decide what data they want where. Go to dataengineeringpodcast.com/segmentio today to sign up for their startup plan and get $25,000 in Segment credits and $1 million in free software from marketing and analytics companies like AWS, Google, and Intercom. On top of that you’ll get access to Analytics Academy for the educational resources you need to become an expert in data analytics for measuring product-market fit.
You listen to this show to learn and stay up to date with what’s happening in databases, streaming platforms, big data, and everything else you need to know about modern data management. For even more opportunities to meet, listen, and learn from your peers you don’t want to miss out on this year’s conference season. We have partnered with organizations such as O’Reilly Media, Dataversity, and the Open Data Science Conference. Go to dataengineeringpodcast.com/conferences to learn more and take advantage of our partner discounts when you register.
Go to dataengineeringpodcast.com to subscribe to the show, sign up for the mailing list, read the show notes, and get in touch.
To help other people find the show please leave a review on iTunes and tell your friends and co-workers
Join the community in the new Zulip chat workspace at dataengineeringpodcast.com/chat
Your host is Tobias Macey and today I’m interviewing Joe Doliner about Pachyderm, a platform that lets you deploy and manage multi-stage, language-agnostic data pipelines while maintaining complete reproducibility and provenance
Interview
Introduction
How did you get involved in the area of data management?
Can you start by explaining what Pachyderm is and how it got started?
What is new in the last two years since I talked to Dan Whitenack in episode 1?
How have the changes and additional features in Kubernetes impacted your work on Pachyderm?
A recent development in the Kubernetes space is the Kubeflow project. How do its capabilities compare with or complement what you are doing in Pachyderm?
Can you walk through the overall workflow for someone building an analysis pipeline in Pachyderm?
How does that break down across different roles and responsibilities (e.g. data scientist vs data engineer)?
There are a lot of concepts and moving parts in Pachyderm, from getting a Kubernetes cluster set up, to understanding the file system and processing pipeline, to understanding best practices. What are some of the common challenges or points of confusion that new users encounter?
Data provenance is critical for understanding the end results of an analysis or ML model. Can you explain how the tracking in Pachyderm is implemented?
What is the interface for exposing and exploring that provenance data?
What are some of the advanced capabilities of Pachyderm that you would like to call out?
With your recent round of fundraising I’m assuming there is new pressure to grow and scale your product and business. How are you approaching that and what are some of the challenges you are facing?
What have been some of the most challenging/useful/unexpected lessons that you have learned in the process of building, maintaining, and growing the Pachyderm project and company?
What do you have planned for the future of Pachyderm?
Contact Info
@jdoliner on Twitter
LinkedIn
jdoliner on GitHub
Parting Question
From your perspective, what is the biggest gap in the tooling or technology for data management today?
Links
Pachyderm
RethinkDB
AirBnB
Data Provenance
Kubeflow
Stateful Sets
EtcD
Airflow
Kafka
GitHub
GitLab
Docker
Kubernetes
CI == Continuous Integration
CD == Continuous Delivery
Ceph
Podcast Interview
Object Storage
MiniKube
FUSE == File System In User Space
The intro and outro music is from The Hug by The Freak Fandango Orchestra / CC BY-SA
Support Data Engineering Podcast
49:0127/05/2019
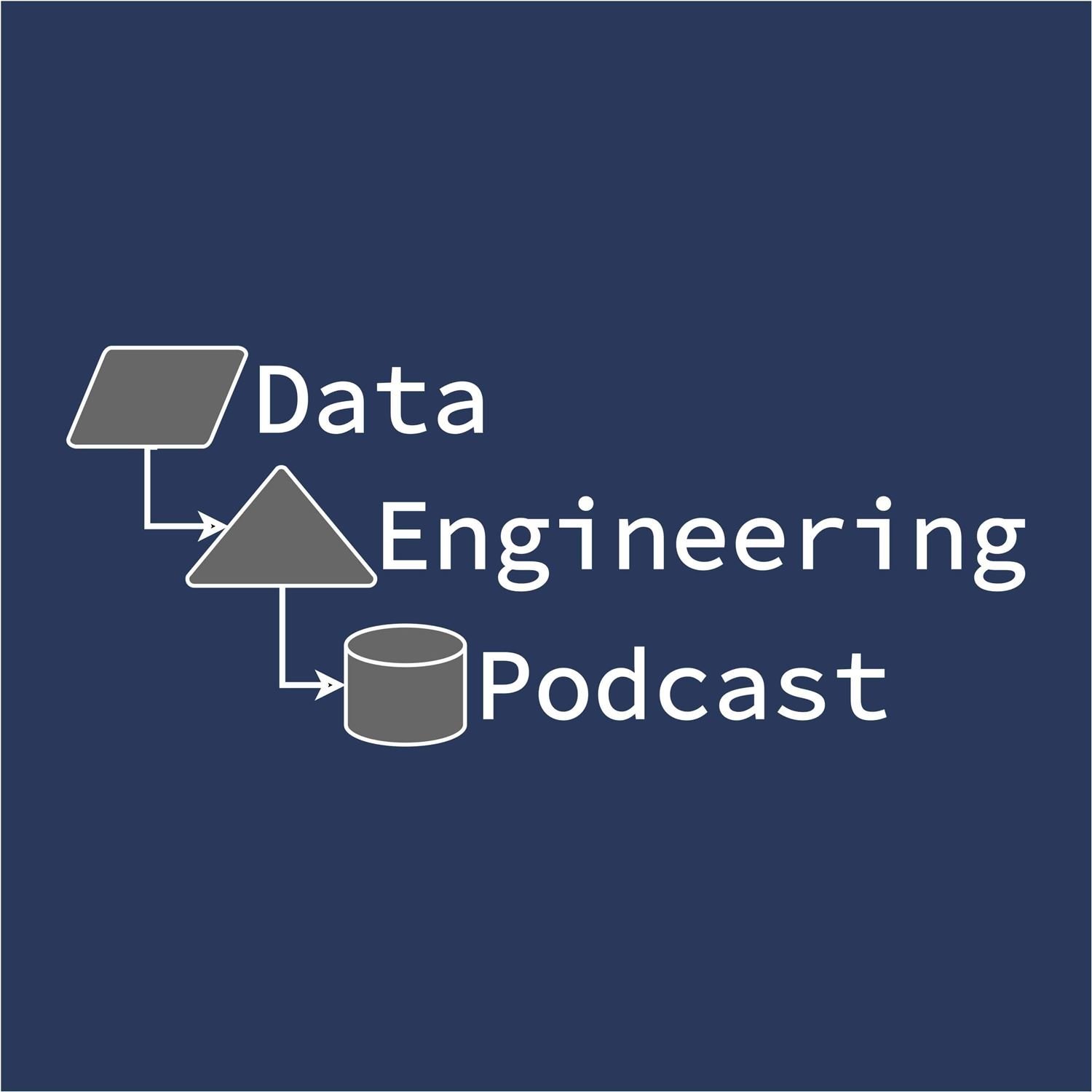
Build Your Data Analytics Like An Engineer With DBT
Summary
In recent years the traditional approach to building data warehouses has shifted from transforming records before loading, to transforming them afterwards. As a result, the tooling for those transformations needs to be reimagined. The data build tool (dbt) is designed to bring battle tested engineering practices to your analytics pipelines. By providing an opinionated set of best practices it simplifies collaboration and boosts confidence in your data teams. In this episode Drew Banin, creator of dbt, explains how it got started, how it is designed, and how you can start using it today to create reliable and well-tested reports in your favorite data warehouse.
Announcements
Hello and welcome to the Data Engineering Podcast, the show about modern data management
When you’re ready to build your next pipeline, or want to test out the projects you hear about on the show, you’ll need somewhere to deploy it, so check out our friends at Linode. With 200Gbit private networking, scalable shared block storage, and a 40Gbit public network, you’ve got everything you need to run a fast, reliable, and bullet-proof data platform. If you need global distribution, they’ve got that covered too with world-wide datacenters including new ones in Toronto and Mumbai. And for your machine learning workloads, they just announced dedicated CPU instances. Go to dataengineeringpodcast.com/linode today to get a $20 credit and launch a new server in under a minute. And don’t forget to thank them for their continued support of this show!
Understanding how your customers are using your product is critical for businesses of any size. To make it easier for startups to focus on delivering useful features Segment offers a flexible and reliable data infrastructure for your customer analytics and custom events. You only need to maintain one integration to instrument your code and get a future-proof way to send data to over 250 services with the flip of a switch. Not only does it free up your engineers’ time, it lets your business users decide what data they want where. Go to dataengineeringpodcast.com/segmentio today to sign up for their startup plan and get $25,000 in Segment credits and $1 million in free software from marketing and analytics companies like AWS, Google, and Intercom. On top of that you’ll get access to Analytics Academy for the educational resources you need to become an expert in data analytics for measuring product-market fit.
You listen to this show to learn and stay up to date with what’s happening in databases, streaming platforms, big data, and everything else you need to know about modern data management. For even more opportunities to meet, listen, and learn from your peers you don’t want to miss out on this year’s conference season. We have partnered with organizations such as O’Reilly Media, Dataversity, and the Open Data Science Conference. Go to dataengineeringpodcast.com/conferences to learn more and take advantage of our partner discounts when you register.
Go to dataengineeringpodcast.com to subscribe to the show, sign up for the mailing list, read the show notes, and get in touch.
To help other people find the show please leave a review on iTunes and tell your friends and co-workers
Join the community in the new Zulip chat workspace at dataengineeringpodcast.com/chat
Your host is Tobias Macey and today I’m interviewing Drew Banin about DBT, the Data Build Tool, a toolkit for building analytics the way that developers build applications
Interview
Introduction
How did you get involved in the area of data management?
Can you start by explaining what DBT is and your motivation for creating it?
Where does it fit in the overall landscape of data tools and the lifecycle of data in an analytics pipeline?
Can you talk through the workflow for someone using DBT?
One of the useful features of DBT for stability of analytics is the ability to write and execute tests. Can you explain how those are implemented?
The packaging capabilities are beneficial for enabling collaboration. Can you talk through how the packaging system is implemented?
Are these packages driven by Fishtown Analytics or the dbt community?
What are the limitations of modeling everything as a SELECT statement?
Making SQL code reusable is notoriously difficult. How does the Jinja templating of DBT address this issue and what are the shortcomings?
What are your thoughts on higher level approaches to SQL that compile down to the specific statements?
Can you explain how DBT is implemented and how the design has evolved since you first began working on it?
What are some of the features of DBT that are often overlooked which you find particularly useful?
What are some of the most interesting/unexpected/innovative ways that you have seen DBT used?
What are the additional features that the commercial version of DBT provides?
What are some of the most useful or challenging lessons that you have learned in the process of building and maintaining DBT?
When is it the wrong choice?
What do you have planned for the future of DBT?
Contact Info
Email
@drebanin on Twitter
drebanin on GitHub
Parting Question
From your perspective, what is the biggest gap in the tooling or technology for data management today?
Links
DBT
Fishtown Analytics
8Tracks Internet Radio
Redshift
Magento
Stitch Data
Fivetran
Airflow
Business Intelligence
Jinja template language
BigQuery
Snowflake
Version Control
Git
Continuous Integration
Test Driven Development
Snowplow Analytics
Podcast Episode
dbt-utils
We Can Do Better Than SQL blog post from EdgeDB
EdgeDB
Looker LookML
Podcast Interview
Presto DB
Podcast Interview
Spark SQL
Hive
Azure SQL Data Warehouse
Data Warehouse
Data Lake
Data Council Conference
Slowly Changing Dimensions
dbt Archival
Mode Analytics
Periscope BI
dbt docs
dbt repository
The intro and outro music is from The Hug by The Freak Fandango Orchestra / CC BY-SA
Support Data Engineering Podcast
56:4620/05/2019
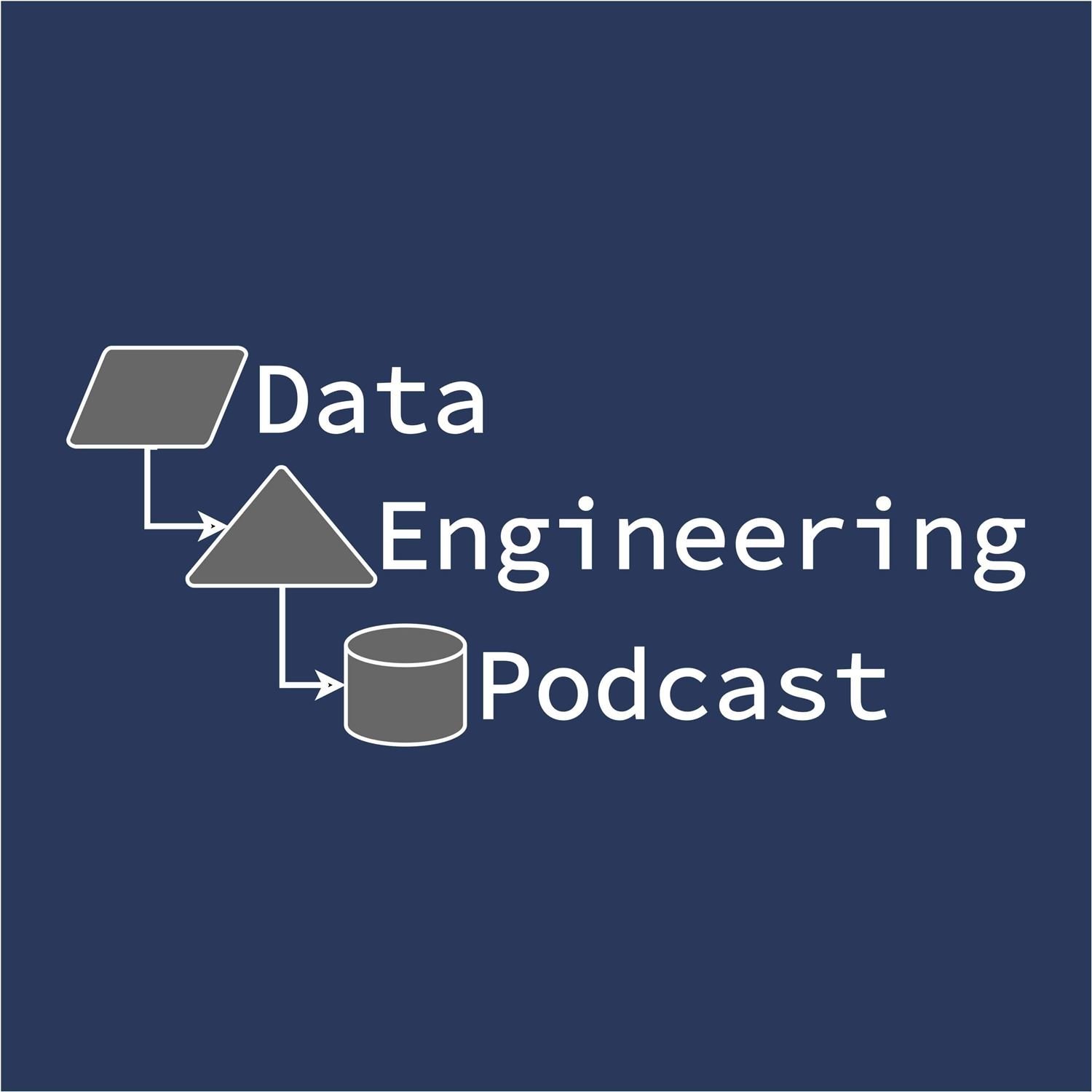
Using FoundationDB As The Bedrock For Your Distributed Systems
Summary
The database market continues to expand, offering systems that are suited to virtually every use case. But what happens if you need something customized to your application? FoundationDB is a distributed key-value store that provides the primitives that you need to build a custom database platform. In this episode Ryan Worl explains how it is architected, how to use it for your applications, and provides examples of system design patterns that can be built on top of it. If you need a foundation for your distributed systems, then FoundationDB is definitely worth a closer look.
Announcements
Hello and welcome to the Data Engineering Podcast, the show about modern data management
When you’re ready to build your next pipeline, or want to test out the projects you hear about on the show, you’ll need somewhere to deploy it, so check out our friends at Linode. With 200Gbit private networking, scalable shared block storage, and a 40Gbit public network, you’ve got everything you need to run a fast, reliable, and bullet-proof data platform. If you need global distribution, they’ve got that covered too with world-wide datacenters including new ones in Toronto and Mumbai. And for your machine learning workloads, they just announced dedicated CPU instances. Go to dataengineeringpodcast.com/linode today to get a $20 credit and launch a new server in under a minute. And don’t forget to thank them for their continued support of this show!
Alluxio is an open source, distributed data orchestration layer that makes it easier to scale your compute and your storage independently. By transparently pulling data from underlying silos, Alluxio unlocks the value of your data and allows for modern computation-intensive workloads to become truly elastic and flexible for the cloud. With Alluxio, companies like Barclays, JD.com, Tencent, and Two Sigma can manage data efficiently, accelerate business analytics, and ease the adoption of any cloud. Go to dataengineeringpodcast.com/alluxio today to learn more and thank them for their support.
Understanding how your customers are using your product is critical for businesses of any size. To make it easier for startups to focus on delivering useful features Segment offers a flexible and reliable data infrastructure for your customer analytics and custom events. You only need to maintain one integration to instrument your code and get a future-proof way to send data to over 250 services with the flip of a switch. Not only does it free up your engineers’ time, it lets your business users decide what data they want where. Go to dataengineeringpodcast.com/segmentio today to sign up for their startup plan and get $25,000 in Segment credits and $1 million in free software from marketing and analytics companies like AWS, Google, and Intercom. On top of that you’ll get access to Analytics Academy for the educational resources you need to become an expert in data analytics for measuring product-market fit.
You listen to this show to learn and stay up to date with what’s happening in databases, streaming platforms, big data, and everything else you need to know about modern data management. For even more opportunities to meet, listen, and learn from your peers you don’t want to miss out on this year’s conference season. We have partnered with organizations such as O’Reilly Media, Dataversity, and the Open Data Science Conference. Go to dataengineeringpodcast.com/conferences to learn more and take advantage of our partner discounts when you register.
Go to dataengineeringpodcast.com to subscribe to the show, sign up for the mailing list, read the show notes, and get in touch.
To help other people find the show please leave a review on iTunes and tell your friends and co-workers
Join the community in the new Zulip chat workspace at dataengineeringpodcast.com/chat
Your host is Tobias Macey and today I’m interviewing Ryan Worl about FoundationDB, a distributed key/value store that gives you the power of ACID transactions in a NoSQL database
Interview
Introduction
How did you get involved in the area of data management?
Can you explain what FoundationDB is and how you got involved with the project?
What are some of the unique use cases that FoundationDB enables?
Can you describe how FoundationDB is architected?
How is the ACID compliance implemented at the cluster level?
What are some of the mechanisms built into FoundationDB that contribute to its fault tolerance?
How are conflicts managed?
FoundationDB has an interesting feature in the form of Layers that provide different semantics on the underlying storage. Can you describe how that is implemented and some of the interesting layers that are available?
Is it possible to apply different layers, such as relational and document, to the same underlying objects in storage?
One of the aspects of FoundationDB that is called out in the documentation and which I have heard about elsewhere is the performance that it provides. Can you describe some of the implementation mechanics of FoundationDB that allow it to provide such high throughput?
For someone who wants to run FoundationDB can you describe a typical deployment topology?
What are the scaling factors for the underlying storage and for the Layers that are operating on the cluster?
Once you have a cluster deployed, what are some of the edge cases that users should watch out for?
How are version upgrades managed in a cluster?
What are some of the ways that FoundationDB impacts the way that an application developer or data engineer would architect their software as compared to working with something like Postgres or MongoDB?
What are some of the more interesting/unusual/unexpected ways that you have seen FoundationDB used?
When is FoundationDB the wrong choice?
What is in store for the future of FoundationDB?
Contact Info
LinkedIn
@ryanworl on Twitter
Website
Parting Question
From your perspective, what is the biggest gap in the tooling or technology for data management today?
Links
FoundationDB
Jepsen
Andy Pavlo
Archive.org – The Internet Archive
FoundationDB Summit
Flow Language
C++
Actor Model
Erlang
Zookeeper
Podcast Episode
PAXOS consensus algorithm
Multi-Version Concurrency Control (MVCC) AKA Optimistic Locking
ACID
CAP Theorem
Redis
Record Layer
CloudKit
Document Layer
Segment
Podcast Episode
NVMe
SnowflakeDB
FlatBuffers
Protocol Buffers
Ryan Worl FoundationDB Summit Presentation
Google F1
Google Spanner
WaveFront
EtcD
B+ Tree
Michael Stonebraker
Three Vs
Confluent
The intro and outro music is from The Hug by The Freak Fandango Orchestra / CC BY-SA
Support Data Engineering Podcast
01:06:0207/05/2019
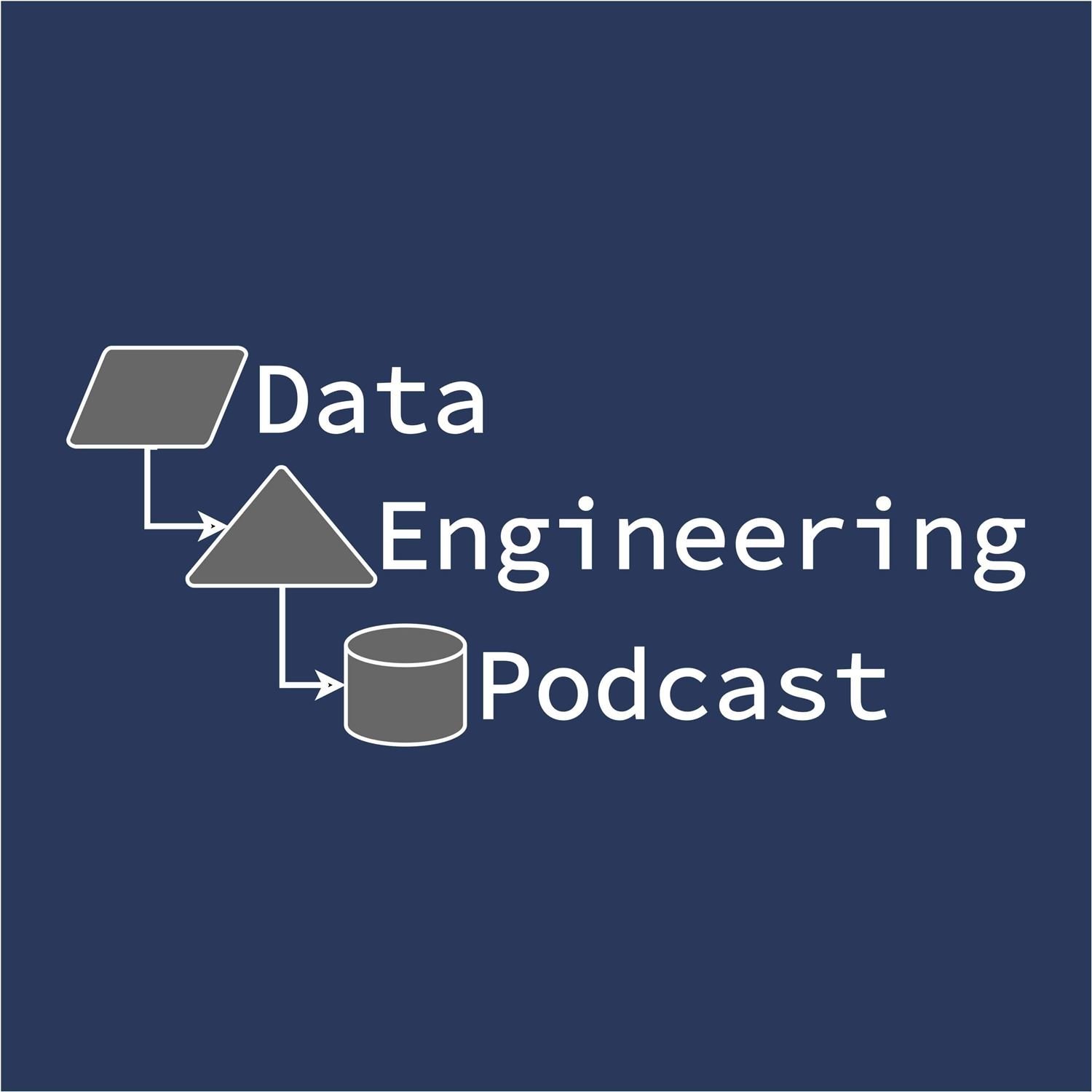
Running Your Database On Kubernetes With KubeDB
Summary
Kubernetes is a driving force in the renaissance around deploying and running applications. However, managing the database layer is still a separate concern. The KubeDB project was created as a way of providing a simple mechanism for running your storage system in the same platform as your application. In this episode Tamal Saha explains how the KubeDB project got started, why you might want to run your database with Kubernetes, and how to get started. He also covers some of the challenges of managing stateful services in Kubernetes and how the fast pace of the community has contributed to the evolution of KubeDB. If you are at any stage of a Kubernetes implementation, or just thinking about it, this is definitely worth a listen to get some perspective on how to leverage it for your entire application stack.
Announcements
Hello and welcome to the Data Engineering Podcast, the show about modern data management
When you’re ready to build your next pipeline, or want to test out the projects you hear about on the show, you’ll need somewhere to deploy it, so check out our friends at Linode. With 200Gbit private networking, scalable shared block storage, and a 40Gbit public network, you’ve got everything you need to run a fast, reliable, and bullet-proof data platform. If you need global distribution, they’ve got that covered too with world-wide datacenters including new ones in Toronto and Mumbai. And for your machine learning workloads, they just announced dedicated CPU instances. Go to dataengineeringpodcast.com/linode today to get a $20 credit and launch a new server in under a minute. And don’t forget to thank them for their continued support of this show!
Alluxio is an open source, distributed data orchestration layer that makes it easier to scale your compute and your storage independently. By transparently pulling data from underlying silos, Alluxio unlocks the value of your data and allows for modern computation-intensive workloads to become truly elastic and flexible for the cloud. With Alluxio, companies like Barclays, JD.com, Tencent, and Two Sigma can manage data efficiently, accelerate business analytics, and ease the adoption of any cloud. Go to dataengineeringpodcast.com/alluxio today to learn more and thank them for their support.
Understanding how your customers are using your product is critical for businesses of any size. To make it easier for startups to focus on delivering useful features Segment offers a flexible and reliable data infrastructure for your customer analytics and custom events. You only need to maintain one integration to instrument your code and get a future-proof way to send data to over 250 services with the flip of a switch. Not only does it free up your engineers’ time, it lets your business users decide what data they want where. Go to dataengineeringpodcast.com/segmentio today to sign up for their startup plan and get $25,000 in Segment credits and $1 million in free software from marketing and analytics companies like AWS, Google, and Intercom. On top of that you’ll get access to Analytics Academy for the educational resources you need to become an expert in data analytics for measuring product-market fit.
You listen to this show to learn and stay up to date with what’s happening in databases, streaming platforms, big data, and everything else you need to know about modern data management. For even more opportunities to meet, listen, and learn from your peers you don’t want to miss out on this year’s conference season. We have partnered with organizations such as O’Reilly Media, Dataversity, and the Open Data Science Conference. Go to dataengineeringpodcast.com/conferences to learn more and take advantage of our partner discounts when you register.
Go to dataengineeringpodcast.com to subscribe to the show, sign up for the mailing list, read the show notes, and get in touch.
To help other people find the show please leave a review on iTunes and tell your friends and co-workers
Join the community in the new Zulip chat workspace at dataengineeringpodcast.com/chat
Your host is Tobias Macey and today I’m interviewing Tamal Saha about KubeDB, a project focused on making running production-grade databases easy on Kubernetes
Interview
Introduction
How did you get involved in the area of data management?
Can you start by explaining what KubeDB is and how the project got started?
What are the main challenges associated with running a stateful system on top of Kubernetes?
Why would someone want to run their database on a container platform rather than on a dedicated instance or with a hosted service?
Can you describe how KubeDB is implemented and how that has evolved since you first started working on it?
Can you talk through how KubeDB simplifies the process of deploying and maintaining databases?
What is involved in adding support for a new database?
How do the requirements change for systems that are natively clustered?
How does KubeDB help with maintenance processes around upgrading existing databases to newer versions?
How does the work that you are doing on KubeDB compare to what is available in StorageOS?
Are there any other projects that are targeting similar goals?
What have you found to be the most interesting/challenging/unexpected aspects of building KubeDB?
What do you have planned for the future of the project?
Contact Info
LinkedIn
@tsaha on Twitter
Email
tamalsaha on GitHub
Parting Question
From your perspective, what is the biggest gap in the tooling or technology for data management today?
Links
KubeDB
AppsCode
Kubernetes
Kubernetes CRD (Custom Resource Definition)
Kubernetes Operator
Kubernetes Stateful Sets
PostgreSQL
Podcast Interview
Hashicorp Vault
Redis
Elasticsearch
Podcast Interview
MySQL
Memcached
MongoDB
Docker
Rook Storage Orchestration for Kubernetes
Ceph
Podcast Interview
EBS
StorageOS
GlusterFS
OpenEBS
CloudFoundry
AppsCode Service Broker
The intro and outro music is from The Hug by The Freak Fandango Orchestra / CC BY-SA
Support Data Engineering Podcast
50:5529/04/2019
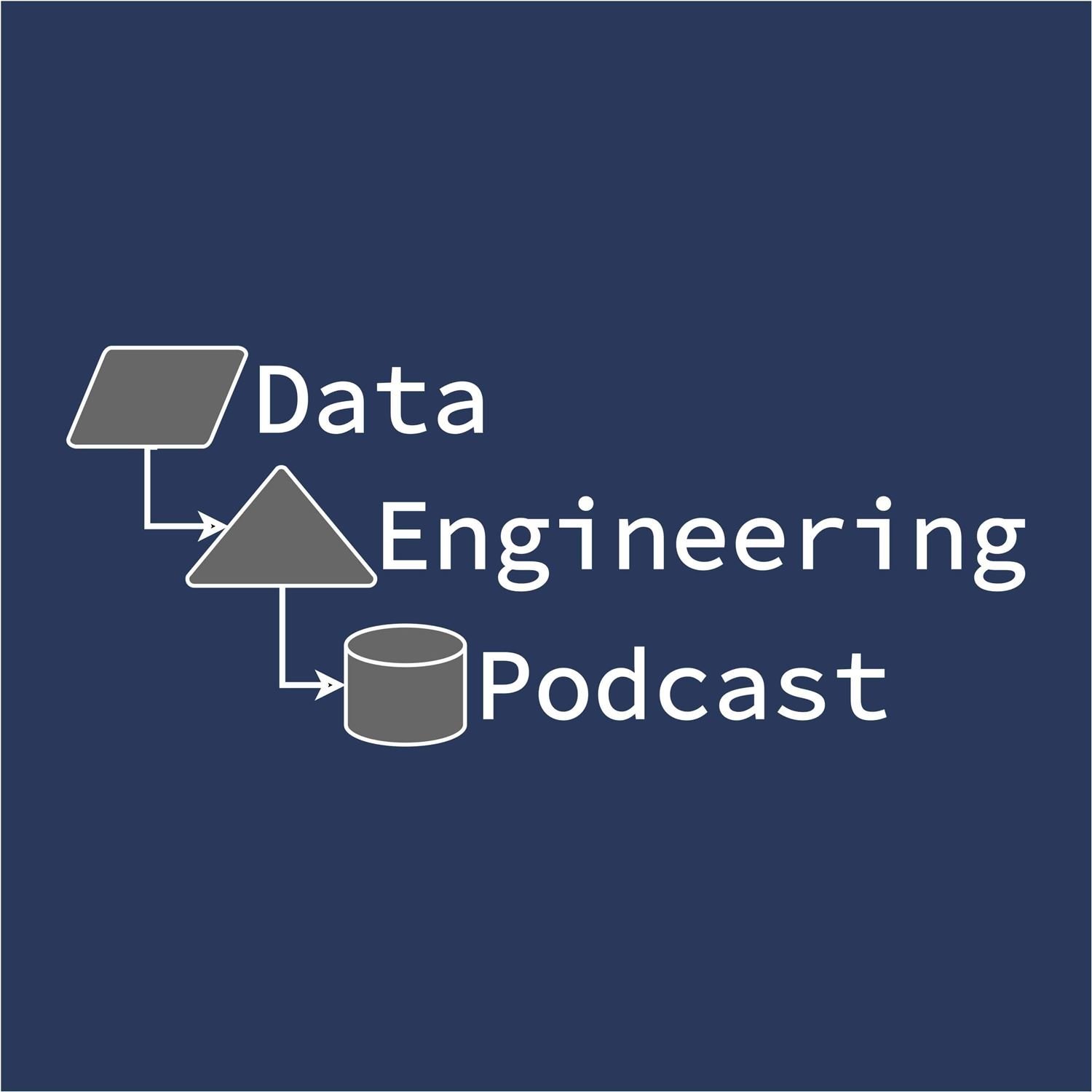
Unpacking Fauna: A Global Scale Cloud Native Database
Summary
One of the biggest challenges for any business trying to grow and reach customers globally is how to scale their data storage. FaunaDB is a cloud native database built by the engineers behind Twitter’s infrastructure and designed to serve the needs of modern systems. Evan Weaver is the co-founder and CEO of Fauna and in this episode he explains the unique capabilities of Fauna, compares the consensus and transaction algorithm to that used in other NewSQL systems, and describes the ways that it allows for new application design patterns. One of the unique aspects of Fauna that is worth drawing attention to is the first class support for temporality that simplifies querying of historical states of the data. It is definitely worth a good look for anyone building a platform that needs a simple to manage data layer that will scale with your business.
Announcements
Hello and welcome to the Data Engineering Podcast, the show about modern data management
When you’re ready to build your next pipeline, or want to test out the projects you hear about on the show, you’ll need somewhere to deploy it, so check out our friends at Linode. With 200Gbit private networking, scalable shared block storage, and a 40Gbit public network, you’ve got everything you need to run a fast, reliable, and bullet-proof data platform. If you need global distribution, they’ve got that covered too with world-wide datacenters including new ones in Toronto and Mumbai. And for your machine learning workloads, they just announced dedicated CPU instances. Go to dataengineeringpodcast.com/linode today to get a $20 credit and launch a new server in under a minute. And don’t forget to thank them for their continued support of this show!
Alluxio is an open source, distributed data orchestration layer that makes it easier to scale your compute and your storage independently. By transparently pulling data from underlying silos, Alluxio unlocks the value of your data and allows for modern computation-intensive workloads to become truly elastic and flexible for the cloud. With Alluxio, companies like Barclays, JD.com, Tencent, and Two Sigma can manage data efficiently, accelerate business analytics, and ease the adoption of any cloud. Go to dataengineeringpodcast.com/alluxio today to learn more and thank them for their support.
Understanding how your customers are using your product is critical for businesses of any size. To make it easier for startups to focus on delivering useful features Segment offers a flexible and reliable data infrastructure for your customer analytics and custom events. You only need to maintain one integration to instrument your code and get a future-proof way to send data to over 250 services with the flip of a switch. Not only does it free up your engineers’ time, it lets your business users decide what data they want where. Go to dataengineeringpodcast.com/segmentio today to sign up for their startup plan and get $25,000 in Segment credits and $1 million in free software from marketing and analytics companies like AWS, Google, and Intercom. On top of that you’ll get access to Analytics Academy for the educational resources you need to become an expert in data analytics for measuring product-market fit.
You listen to this show to learn and stay up to date with what’s happening in databases, streaming platforms, big data, and everything else you need to know about modern data management. For even more opportunities to meet, listen, and learn from your peers you don’t want to miss out on this year’s conference season. We have partnered with organizations such as O’Reilly Media, Dataversity, and the Open Data Science Conference. Go to dataengineeringpodcast.com/conferences to learn more and take advantage of our partner discounts when you register.
Go to dataengineeringpodcast.com to subscribe to the show, sign up for the mailing list, read the show notes, and get in touch.
To help other people find the show please leave a review on iTunes and tell your friends and co-workers
Join the community in the new Zulip chat workspace at dataengineeringpodcast.com/chat
Your host is Tobias Macey and today I’m interviewing Evan Weaver about FaunaDB, a modern operational data platform built for your cloud
Interview
Introduction
How did you get involved in the area of data management?
Can you start by explaining what FaunaDB is and how it got started?
What are some of the main use cases that FaunaDB is targeting?
How does it compare to some of the other global scale databases that have been built in recent years such as CockroachDB?
Can you describe the architecture of FaunaDB and how it has evolved?
The consensus and replication protocol in Fauna is intriguing. Can you talk through how it works?
What are some of the edge cases that users should be aware of?
How are conflicts managed in Fauna?
What is the underlying storage layer?
How is the query layer designed to allow for different query patterns and model representations?
How does data modeling in Fauna compare to that of relational or document databases?
Can you describe the query format?
What are some of the common difficulties or points of confusion around interacting with data in Fauna?
What are some application design patterns that are enabled by using Fauna as the storage layer?
Given the ability to replicate globally, how do you mitigate latency when interacting with the database?
What are some of the most interesting or unexpected ways that you have seen Fauna used?
When is it the wrong choice?
What have been some of the most interesting/unexpected/challenging aspects of building the Fauna database and company?
What do you have in store for the future of Fauna?
Contact Info
@evan on Twitter
LinkedIn
Parting Question
From your perspective, what is the biggest gap in the tooling or technology for data management today?
Links
Fauna
Ruby on Rails
CNET
GitHub
Twitter
NoSQL
Cassandra
InnoDB
Redis
Memcached
Timeseries
Spanner Paper
DynamoDB Paper
Percolator
ACID
Calvin Protocol
Daniel Abadi
LINQ
LSM Tree (Log-structured Merge-tree)
Scala
Change Data Capture
GraphQL
Podcast.init Interview About Graphene
Fauna Query Language (FQL)
CQL == Cassandra Query Language
Object-Relational Databases
LDAP == Lightweight Directory Access Protocol
Auth0
OLAP == Online Analytical Processing
Jepsen distributed systems safety research
The intro and outro music is from The Hug by The Freak Fandango Orchestra / CC BY-SA
Support Data Engineering Podcast
53:5122/04/2019
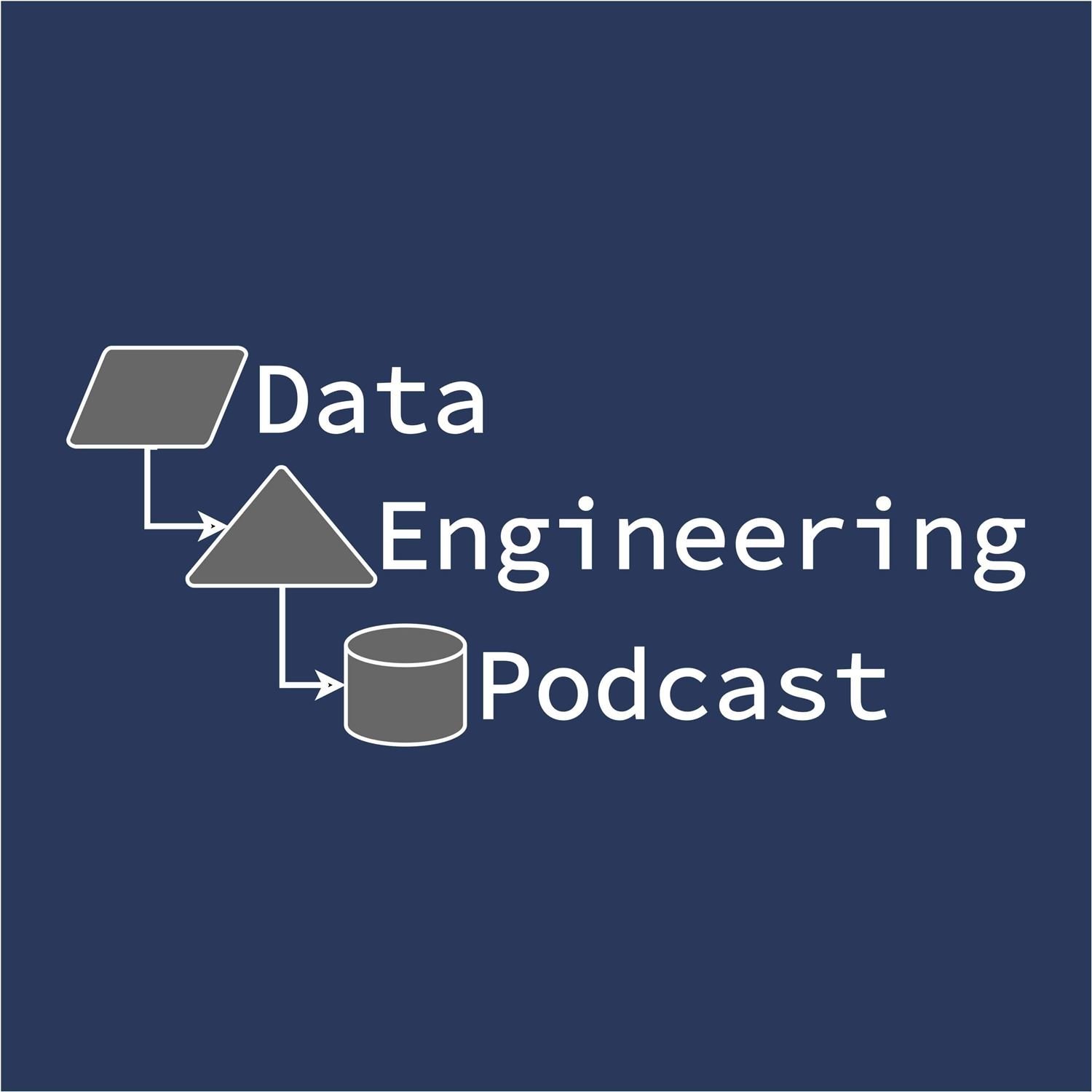
Index Your Big Data With Pilosa For Faster Analytics
Summary
Database indexes are critical to ensure fast lookups of your data, but they are inherently tied to the database engine. Pilosa is rewriting that equation by providing a flexible, scalable, performant engine for building an index of your data to enable high-speed aggregate analysis. In this episode Seebs explains how Pilosa fits in the broader data landscape, how it is architected, and how you can start using it for your own analysis. This was an interesting exploration of a different way to look at what a database can be.
Announcements
Hello and welcome to the Data Engineering Podcast, the show about modern data management
When you’re ready to build your next pipeline, or want to test out the projects you hear about on the show, you’ll need somewhere to deploy it, so check out our friends at Linode. With 200Gbit private networking, scalable shared block storage, and a 40Gbit public network, you’ve got everything you need to run a fast, reliable, and bullet-proof data platform. If you need global distribution, they’ve got that covered too with world-wide datacenters including new ones in Toronto and Mumbai. And for your machine learning workloads, they just announced dedicated CPU instances. Go to dataengineeringpodcast.com/linode today to get a $20 credit and launch a new server in under a minute. And don’t forget to thank them for their continued support of this show!
Alluxio is an open source, distributed data orchestration layer that makes it easier to scale your compute and your storage independently. By transparently pulling data from underlying silos, Alluxio unlocks the value of your data and allows for modern computation-intensive workloads to become truly elastic and flexible for the cloud. With Alluxio, companies like Barclays, JD.com, Tencent, and Two Sigma can manage data efficiently, accelerate business analytics, and ease the adoption of any cloud. Go to dataengineeringpodcast.com/alluxio today to learn more and thank them for their support.
Understanding how your customers are using your product is critical for businesses of any size. To make it easier for startups to focus on delivering useful features Segment offers a flexible and reliable data infrastructure for your customer analytics and custom events. You only need to maintain one integration to instrument your code and get a future-proof way to send data to over 250 services with the flip of a switch. Not only does it free up your engineers’ time, it lets your business users decide what data they want where. Go to dataengineeringpodcast.com/segmentio today to sign up for their startup plan and get $25,000 in Segment credits and $1 million in free software from marketing and analytics companies like AWS, Google, and Intercom. On top of that you’ll get access to Analytics Academy for the educational resources you need to become an expert in data analytics for measuring product-market fit.
You listen to this show to learn and stay up to date with what’s happening in databases, streaming platforms, big data, and everything else you need to know about modern data management. For even more opportunities to meet, listen, and learn from your peers you don’t want to miss out on this year’s conference season. We have partnered with organizations such as O’Reilly Media, Dataversity, and the Open Data Science Conference. Go to dataengineeringpodcast.com/conferences to learn more and take advantage of our partner discounts when you register.
Go to dataengineeringpodcast.com to subscribe to the show, sign up for the mailing list, read the show notes, and get in touch.
To help other people find the show please leave a review on iTunes and tell your friends and co-workers
Join the community in the new Zulip chat workspace at dataengineeringpodcast.com/chat
Your host is Tobias Macey and today I’m interviewing Seebs about Pilosa, an open source, distributed bitmap index
Interview
Introduction
How did you get involved in the area of data management?
Can you start by describing what Pilosa is and how the project got started?
Where does Pilosa fit into the overall data ecosystem and how does it integrate into an existing stack?
What types of use cases is Pilosa uniquely well suited for?
The Pilosa data model is fairly unique. Can you talk through how it is represented and implemented?
What are some approaches to modeling data that might be coming from a relational database or some structured flat files?
How do you handle highly dimensional data?
What are some of the decisions that need to be made early in the modeling process which could have ramifications later on in the lifecycle of the project?
What are the scaling factors of Pilosa?
What are some of the most interesting/challenging/unexpected lessons that you have learned in the process of building Pilosa?
What is in store for the future of Pilosa?
Contact Info
Pilosa
Website
Email
@slothware on Twitter
Seebs
seebs on GitHub
Website
Parting Question
From your perspective, what is the biggest gap in the tooling or technology for data management today?
Links
PQL (Pilosa Query Language)
Roaring Bitmap
Whitepaper
The intro and outro music is from The Hug by The Freak Fandango Orchestra / CC BY-SA
Support Data Engineering Podcast
43:4215/04/2019
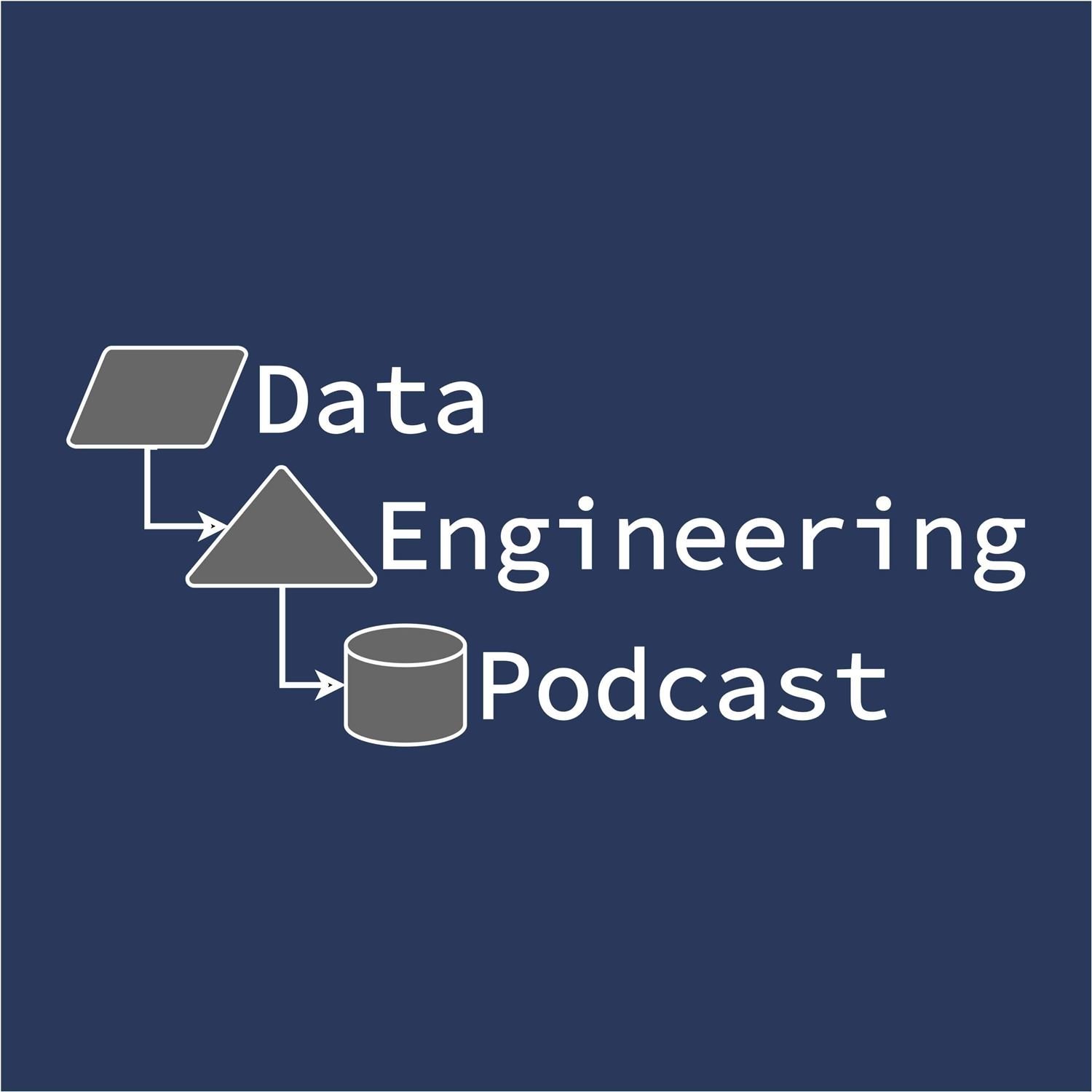
Serverless Data Pipelines On DataCoral
Summary
How much time do you spend maintaining your data pipeline? How much end user value does that provide? Raghu Murthy founded DataCoral as a way to abstract the low level details of ETL so that you can focus on the actual problem that you are trying to solve. In this episode he explains his motivation for building the DataCoral platform, how it is leveraging serverless computing, the challenges of delivering software as a service to customer environments, and the architecture that he has designed to make batch data management easier to work with. This was a fascinating conversation with someone who has spent his entire career working on simplifying complex data problems.
Announcements
Hello and welcome to the Data Engineering Podcast, the show about modern data management
When you’re ready to build your next pipeline, or want to test out the projects you hear about on the show, you’ll need somewhere to deploy it, so check out our friends at Linode. With 200Gbit private networking, scalable shared block storage, and a 40Gbit public network, you’ve got everything you need to run a fast, reliable, and bullet-proof data platform. If you need global distribution, they’ve got that covered too with world-wide datacenters including new ones in Toronto and Mumbai. And for your machine learning workloads, they just announced dedicated CPU instances. Go to dataengineeringpodcast.com/linode today to get a $20 credit and launch a new server in under a minute. And don’t forget to thank them for their continued support of this show!
Managing and auditing access to your servers and databases is a problem that grows in difficulty alongside the growth of your teams. If you are tired of wasting your time cobbling together scripts and workarounds to give your developers, data scientists, and managers the permissions that they need then it’s time to talk to our friends at strongDM. They have built an easy to use platform that lets you leverage your company’s single sign on for your data platform. Go to dataengineeringpodcast.com/strongdm today to find out how you can simplify your systems.
Alluxio is an open source, distributed data orchestration layer that makes it easier to scale your compute and your storage independently. By transparently pulling data from underlying silos, Alluxio unlocks the value of your data and allows for modern computation-intensive workloads to become truly elastic and flexible for the cloud. With Alluxio, companies like Barclays, JD.com, Tencent, and Two Sigma can manage data efficiently, accelerate business analytics, and ease the adoption of any cloud. Go to dataengineeringpodcast.com/alluxio today to learn more and thank them for their support.
You listen to this show to learn and stay up to date with what’s happening in databases, streaming platforms, big data, and everything else you need to know about modern data management. For even more opportunities to meet, listen, and learn from your peers you don’t want to miss out on this year’s conference season. We have partnered with organizations such as O’Reilly Media, Dataversity, and the Open Data Science Conference. Go to dataengineeringpodcast.com/conferences to learn more and take advantage of our partner discounts when you register.
Go to dataengineeringpodcast.com to subscribe to the show, sign up for the mailing list, read the show notes, and get in touch.
To help other people find the show please leave a review on iTunes and tell your friends and co-workers
Join the community in the new Zulip chat workspace at dataengineeringpodcast.com/chat
Your host is Tobias Macey and today I’m interviewing Raghu Murthy about DataCoral, a platform that offers a fully managed and secure stack in your own cloud that delivers data to where you need it
Interview
Introduction
How did you get involved in the area of data management?
Can you start by explaining what DataCoral is and your motivation for founding it?
How does the data-centric approach of DataCoral differ from the way that other platforms think about processing information?
Can you describe how the DataCoral platform is designed and implemented, and how it has evolved since you first began working on it?
How does the concept of a data slice play into the overall architecture of your platform?
How do you manage transformations of data schemas and formats as they traverse different slices in your platform?
On your site it mentions that you have the ability to automatically adjust to changes in external APIs, can you discuss how that manifests?
What has been your experience, both positive and negative, in building on top of serverless components?
Can you discuss the customer experience of onboarding onto Datacoral and how it differs between existing data platforms and greenfield projects?
What are some of the slices that have proven to be the most challenging to implement?
Are there any that you are currently building that you are most excited for?
How much effort do you anticipate if and/or when you begin to support other cloud providers?
When is Datacoral the wrong choice?
What do you have planned for the future of Datacoral, both from a technical and business perspective?
Contact Info
LinkedIn
Parting Question
From your perspective, what is the biggest gap in the tooling or technology for data management today?
Links
Datacoral
Yahoo!
Apache Hive
Relational Algebra
Social Capital
EIR == Entrepreneur In Residence
Spark
Kafka
AWS Lambda
DAG == Directed Acyclic Graph
AWS Redshift
AWS Athena
AWS Glue
Noisy Neighbor Problem
CI/CD
SnowflakeDB
DataBricks Delta
AWS Sagemaker
The intro and outro music is from The Hug by The Freak Fandango Orchestra / CC BY-SA
Support Data Engineering Podcast
53:4208/04/2019
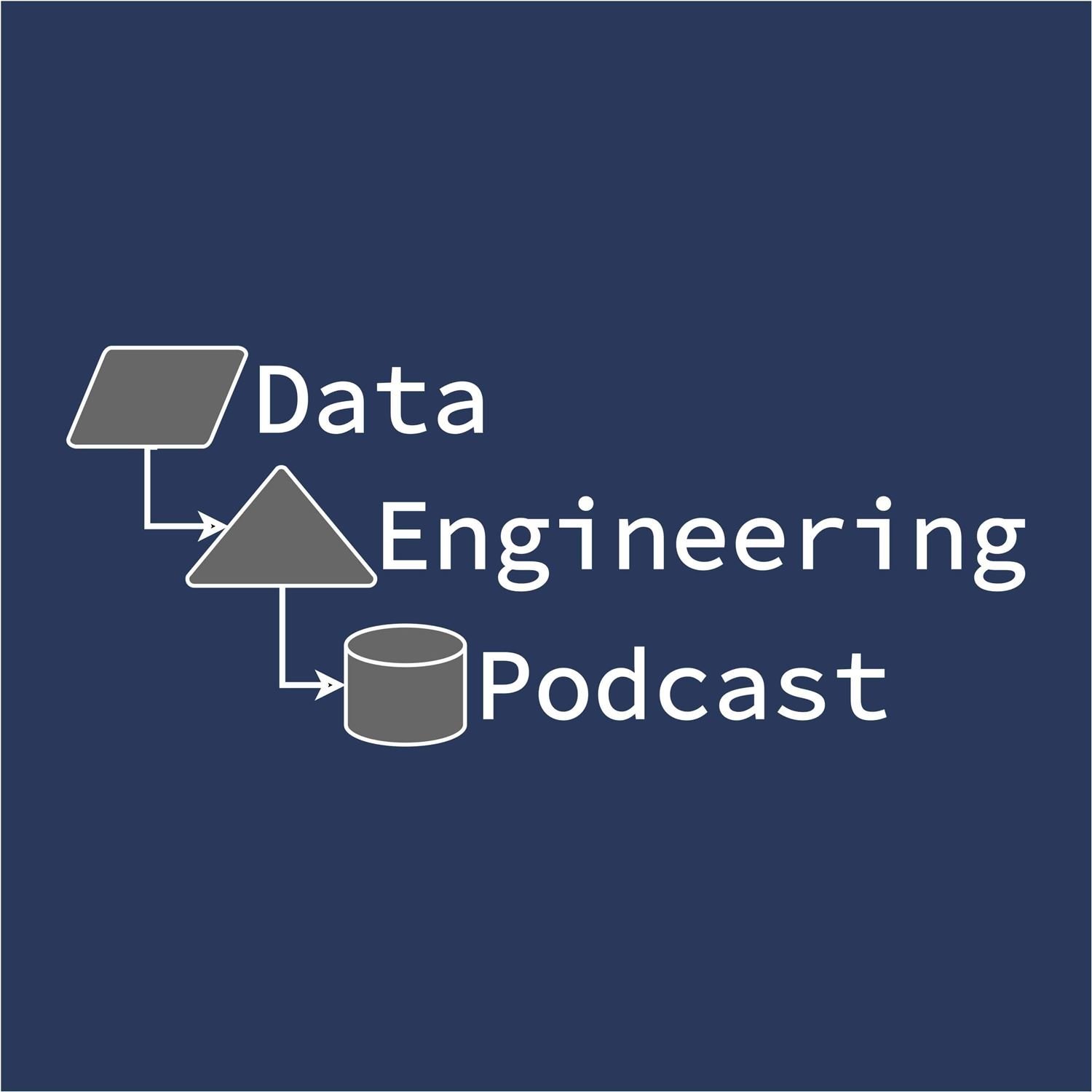
Why Analytics Projects Fail And What To Do About It
Summary
Analytics projects fail all the time, resulting in lost opportunities and wasted resources. There are a number of factors that contribute to that failure and not all of them are under our control. However, many of them are and as data engineers we can help to keep our projects on the path to success. Eugene Khazin is the CEO of PrimeTSR where he is tasked with rescuing floundering analytics efforts and ensuring that they provide value to the business. In this episode he reflects on the ways that data projects can be structured to provide a higher probability of success and utility, how data engineers can get throughout the project lifecycle, and how to salvage a failed project so that some value can be gained from the effort.
Announcements
Hello and welcome to the Data Engineering Podcast, the show about modern data management
When you’re ready to build your next pipeline, or want to test out the projects you hear about on the show, you’ll need somewhere to deploy it, so check out our friends at Linode. With 200Gbit private networking, scalable shared block storage, and a 40Gbit public network, you’ve got everything you need to run a fast, reliable, and bullet-proof data platform. If you need global distribution, they’ve got that covered too with world-wide datacenters including new ones in Toronto and Mumbai. And for your machine learning workloads, they just announced dedicated CPU instances. Go to dataengineeringpodcast.com/linode today to get a $20 credit and launch a new server in under a minute. And don’t forget to thank them for their continued support of this show!
Managing and auditing access to your servers and databases is a problem that grows in difficulty alongside the growth of your teams. If you are tired of wasting your time cobbling together scripts and workarounds to give your developers, data scientists, and managers the permissions that they need then it’s time to talk to our friends at strongDM. They have built an easy to use platform that lets you leverage your company’s single sign on for your data platform. Go to dataengineeringpodcast.com/strongdm today to find out how you can simplify your systems.
Alluxio is an open source, distributed data orchestration layer that makes it easier to scale your compute and your storage independently. By transparently pulling data from underlying silos, Alluxio unlocks the value of your data and allows for modern computation-intensive workloads to become truly elastic and flexible for the cloud. With Alluxio, companies like Barclays, JD.com, Tencent, and Two Sigma can manage data efficiently, accelerate business analytics, and ease the adoption of any cloud. Go to dataengineeringpodcast.com/alluxio today to learn more and thank them for their support.
You listen to this show to learn and stay up to date with what’s happening in databases, streaming platforms, big data, and everything else you need to know about modern data management. For even more opportunities to meet, listen, and learn from your peers you don’t want to miss out on this year’s conference season. We have partnered with organizations such as O’Reilly Media, Dataversity, and the Open Data Science Conference. Go to dataengineeringpodcast.com/conferences to learn more and take advantage of our partner discounts when you register.
Go to dataengineeringpodcast.com to subscribe to the show, sign up for the mailing list, read the show notes, and get in touch.
To help other people find the show please leave a review on iTunes and tell your friends and co-workers
Your host is Tobias Macey and today I’m interviewing Eugene Khazin about the leading causes for failure in analytics projects
Interview
Introduction
How did you get involved in the area of data management?
The term "analytics" has grown to mean many different things to different people, so can you start by sharing your definition of what is in scope for an "analytics project" for the purposes of this discussion?
What are the criteria that you and your customers use to determine the success or failure of a project?
I was recently speaking with someone who quoted a Gartner report stating an estimated failure rate of ~80% for analytics projects. Has your experience reflected this reality, and what have you found to be the leading causes of failure in your experience at PrimeTSR?
As data engineers, what strategies can we pursue to increase the success rate of the projects that we work on?
What are the contributing factors that are beyond our control, which we can help identify and surface early in the lifecycle of a project?
In the event of a failed project, what are the lessons that we can learn and fold into our future work?
How can we salvage a project and derive some value from the efforts that we have put into it?
What are some useful signals to identify when a project is on the road to failure, and steps that can be taken to rescue it?
What advice do you have for data engineers to help them be more active and effective in the lifecycle of an analytics project?
Contact Info
Email
LinkedIn
Parting Question
From your perspective, what is the biggest gap in the tooling or technology for data management today?
Links
Prime TSR
Descriptive, Predictive, and Prescriptive Analytics
Azure Data Factory
Azure Data Warehouse
Mulesoft
SSIS (SQL Server Integration Services)
The intro and outro music is from The Hug by The Freak Fandango Orchestra / CC BY-SA
Support Data Engineering Podcast
36:3001/04/2019
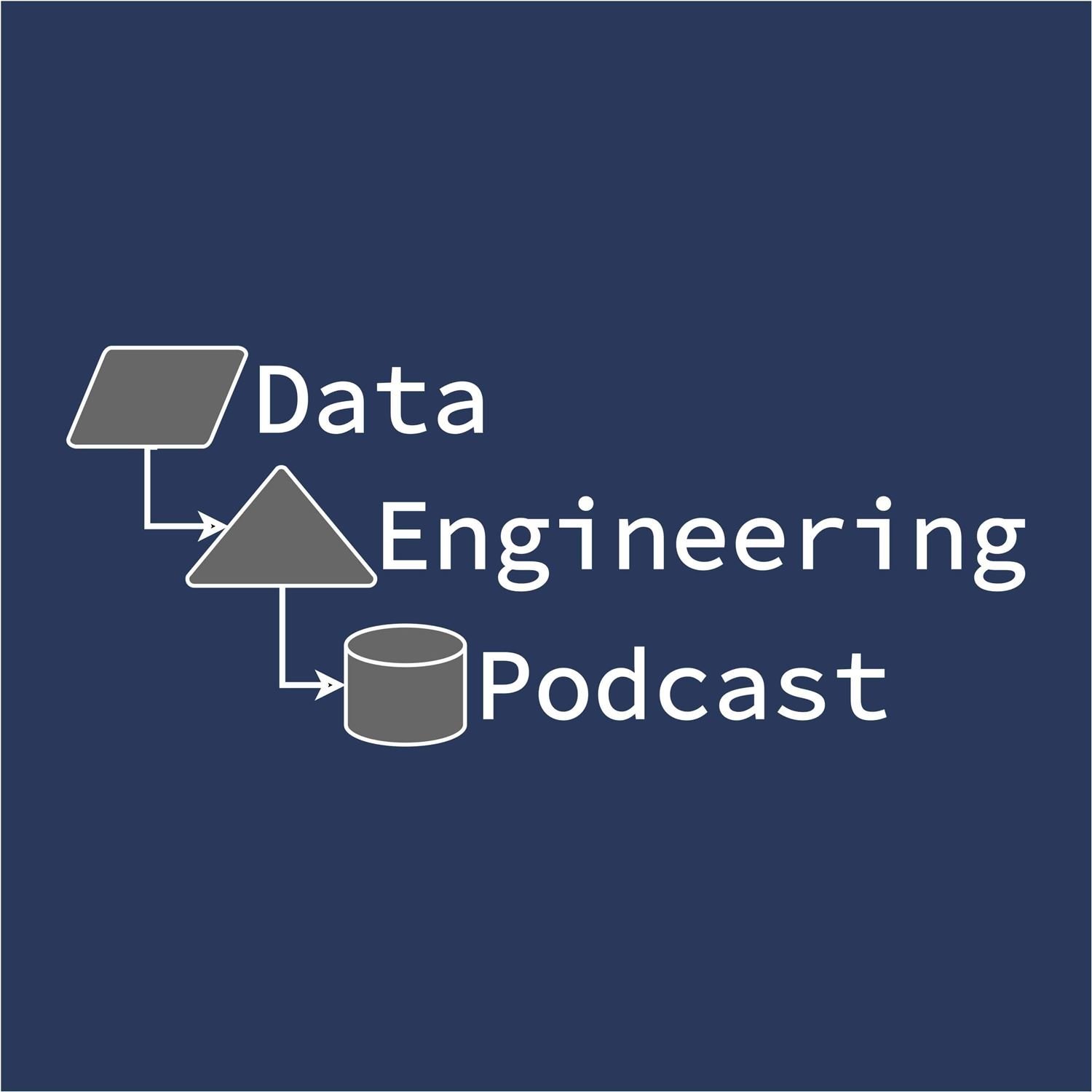
Building An Enterprise Data Fabric At CluedIn
Summary
Data integration is one of the most challenging aspects of any data platform, especially as the variety of data sources and formats grow. Enterprise organizations feel this acutely due to the silos that occur naturally across business units. The CluedIn team experienced this issue first-hand in their previous roles, leading them to build a business aimed at building a managed data fabric for the enterprise. In this episode Tim Ward, CEO of CluedIn, joins me to explain how their platform is architected, how they manage the task of integrating with third-party platforms, automating entity extraction and master data management, and the work of providing multiple views of the same data for different use cases. I highly recommend listening closely to his explanation of how they manage consistency of the data that they process across different storage backends.
Announcements
Hello and welcome to the Data Engineering Podcast, the show about modern data management
When you’re ready to build your next pipeline, or want to test out the projects you hear about on the show, you’ll need somewhere to deploy it, so check out our friends at Linode. With 200Gbit private networking, scalable shared block storage, and a 40Gbit public network, you’ve got everything you need to run a fast, reliable, and bullet-proof data platform. If you need global distribution, they’ve got that covered too with world-wide datacenters including new ones in Toronto and Mumbai. And for your machine learning workloads, they just announced dedicated CPU instances. Go to dataengineeringpodcast.com/linode today to get a $20 credit and launch a new server in under a minute. And don’t forget to thank them for their continued support of this show!
Managing and auditing access to your servers and databases is a problem that grows in difficulty alongside the growth of your teams. If you are tired of wasting your time cobbling together scripts and workarounds to give your developers, data scientists, and managers the permissions that they need then it’s time to talk to our friends at strongDM. They have built an easy to use platform that lets you leverage your company’s single sign on for your data platform. Go to dataengineeringpodcast.com/strongdm today to find out how you can simplify your systems.
Alluxio is an open source, distributed data orchestration layer that makes it easier to scale your compute and your storage independently. By transparently pulling data from underlying silos, Alluxio unlocks the value of your data and allows for modern computation-intensive workloads to become truly elastic and flexible for the cloud. With Alluxio, companies like Barclays, JD.com, Tencent, and Two Sigma can manage data efficiently, accelerate business analytics, and ease the adoption of any cloud. Go to dataengineeringpodcast.com/alluxio today to learn more and thank them for their support.
You listen to this show to learn and stay up to date with what’s happening in databases, streaming platforms, big data, and everything else you need to know about modern data management. For even more opportunities to meet, listen, and learn from your peers you don’t want to miss out on this year’s conference season. We have partnered with organizations such as O’Reilly Media, Dataversity, and the Open Data Science Conference. Go to dataengineeringpodcast.com/conferences to learn more and take advantage of our partner discounts when you register.
Go to dataengineeringpodcast.com to subscribe to the show, sign up for the mailing list, read the show notes, and get in touch.
To help other people find the show please leave a review on iTunes and tell your friends and co-workers
Join the community in the new Zulip chat workspace at dataengineeringpodcast.com/chat
Your host is Tobias Macey and today I’m interviewing Tim Ward about CluedIn, an integration platform for implementing your companies data fabric
Interview
Introduction
How did you get involved in the area of data management?
Before we get started, can you share your definition of what a data fabric is?
Can you explain what CluedIn is and share the story of how it started?
Can you describe your ideal customer?
What are some of the primary ways that organizations are using CluedIn?
Can you give an overview of the system architecture that you have built and how it has evolved since you first began building it?
For a new customer of CluedIn, what is involved in the onboarding process?
What are some of the most challenging aspects of data integration?
What is your approach to managing the process of cleaning the data that you are ingesting?
How much domain knowledge from a business or industry perspective do you incorporate during onboarding and ongoing execution?
How do you preserve and expose data lineage/provenance to your customers?
How do you manage changes or breakage in the interfaces that you use for source or destination systems?
What are some of the signals that you monitor to ensure the continued healthy operation of your platform?
What are some of the most notable customer success stories that you have experienced?
Are there any notable failures that you have experienced, and if so, what were the lessons learned?
What are some cases where CluedIn is not the right choice?
What do you have planned for the future of CluedIn?
Contact Info
Parting Question
From your perspective, what is the biggest gap in the tooling or technology for data management today?
Links
CluedIn
Copenhagen, Denmark
A/B Testing
Data Fabric
Dataiku
RapidMiner
Azure Machine Learning Studio
CRM (Customer Relationship Management)
Graph Database
Data Lake
GraphQL
DGraph
Podcast Episode
RabbitMQ
GDPR (General Data Protection Regulation)
Master Data Management
Podcast Interview
OAuth
Docker
Kubernetes
Helm
DevOps
DataOps
DevOps vs DataOps Podcast Interview
Kafka
The intro and outro music is from The Hug by The Freak Fandango Orchestra / CC BY-SA
Support Data Engineering Podcast
57:5025/03/2019
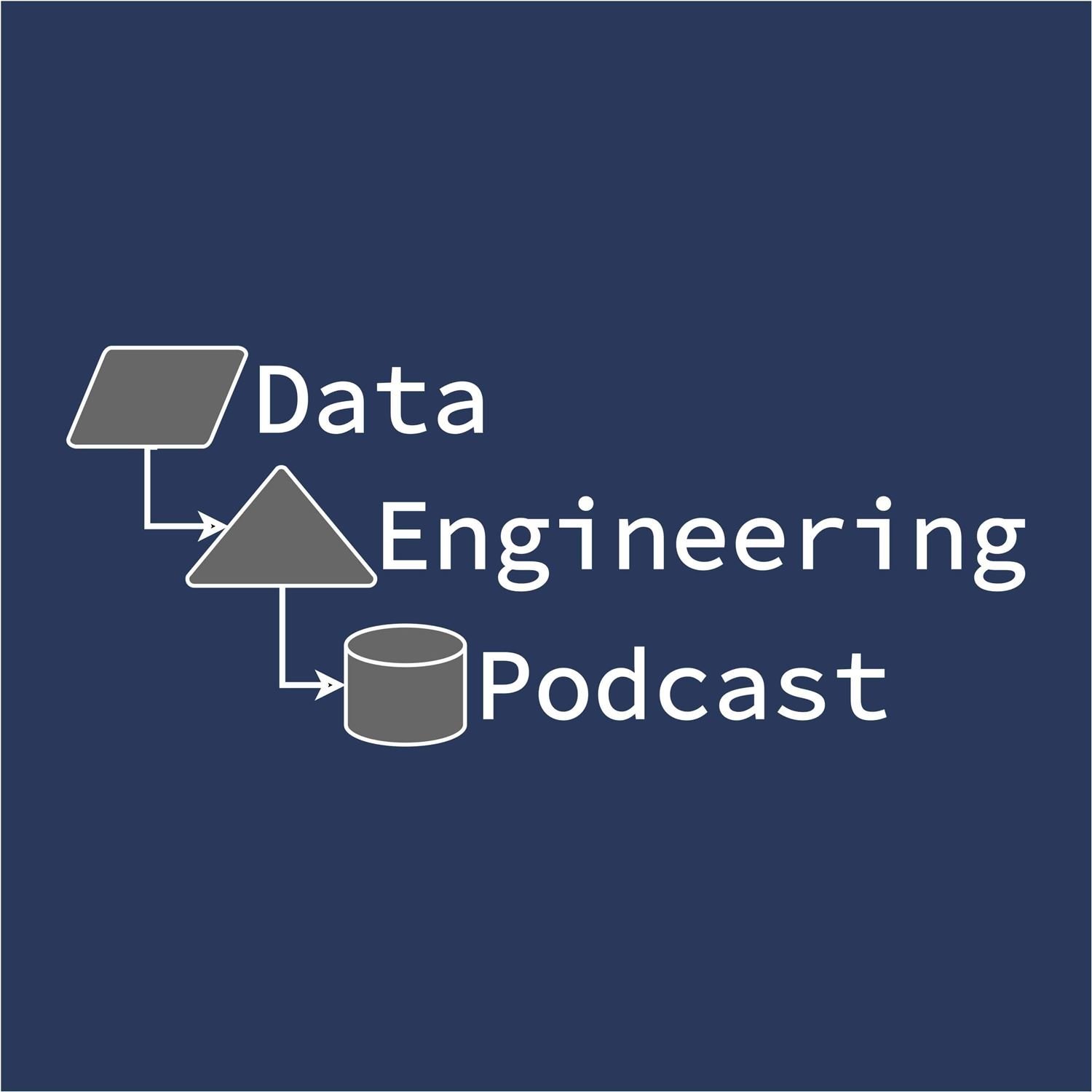
A DataOps vs DevOps Cookoff In The Data Kitchen
Summary
Delivering a data analytics project on time and with accurate information is critical to the success of any business. DataOps is a set of practices to increase the probability of success by creating value early and often, and using feedback loops to keep your project on course. In this episode Chris Bergh, head chef of Data Kitchen, explains how DataOps differs from DevOps, how the industry has begun adopting DataOps, and how to adopt an agile approach to building your data platform.
Announcements
Hello and welcome to the Data Engineering Podcast, the show about modern data management
When you’re ready to build your next pipeline, or want to test out the projects you hear about on the show, you’ll need somewhere to deploy it, so check out our friends at Linode. With 200Gbit private networking, scalable shared block storage, and a 40Gbit public network, you’ve got everything you need to run a fast, reliable, and bullet-proof data platform. If you need global distribution, they’ve got that covered too with world-wide datacenters including new ones in Toronto and Mumbai. And for your machine learning workloads, they just announced dedicated CPU instances. Go to dataengineeringpodcast.com/linode today to get a $20 credit and launch a new server in under a minute. And don’t forget to thank them for their continued support of this show!
Managing and auditing access to your servers and databases is a problem that grows in difficulty alongside the growth of your teams. If you are tired of wasting your time cobbling together scripts and workarounds to give your developers, data scientists, and managers the permissions that they need then it’s time to talk to our friends at strongDM. They have built an easy to use platform that lets you leverage your company’s single sign on for your data platform. Go to dataengineeringpodcast.com/strongdm today to find out how you can simplify your systems.
"There aren’t enough data conferences out there that focus on the community, so that’s why these folks built a better one": Data Council is the premier community powered data platforms & engineering event for software engineers, data engineers, machine learning experts, deep learning researchers & artificial intelligence buffs who want to discover tools & insights to build new products. This year they will host over 50 speakers and 500 attendees (yeah that’s one of the best "Attendee:Speaker" ratios out there) in San Francisco on April 17-18th and are offering a $200 discount to listeners of the Data Engineering Podcast. Use code: DEP-200 at checkout
You listen to this show to learn and stay up to date with what’s happening in databases, streaming platforms, big data, and everything else you need to know about modern data management. For even more opportunities to meet, listen, and learn from your peers you don’t want to miss out on this year’s conference season. We have partnered with organizations such as O’Reilly Media, Dataversity, and the Open Data Science Conference. Go to dataengineeringpodcast.com/conferences to learn more and take advantage of our partner discounts when you register.
Go to dataengineeringpodcast.com to subscribe to the show, sign up for the mailing list, read the show notes, and get in touch.
To help other people find the show please leave a review on iTunes and tell your friends and co-workers
Join the community in the new Zulip chat workspace at dataengineeringpodcast.com/chat
Your host is Tobias Macey and today I’m interviewing Chris Bergh about the current state of DataOps and why it’s more than just DevOps for data
Interview
Introduction
How did you get involved in the area of data management?
We talked last year about what DataOps is, but can you give a quick overview of how the industry has changed or updated the definition since then?
It is easy to draw parallels between DataOps and DevOps, can you provide some clarity as to how they are different?
How has the conversation around DataOps influenced the design decisions of platforms and system components that are targeting the "big data" and data analytics ecosystem?
One of the commonalities is the desire to use collaboration as a means of reducing silos in a business. In the data management space, those silos are often in the form of distinct storage systems, whether application databases, corporate file shares, CRM systems, etc. What are some techniques that are rooted in the principles of DataOps that can help unify those data systems?
Another shared principle is in the desire to create feedback cycles. How do those feedback loops manifest in the lifecycle of an analytics project?
Testing is critical to ensure the continued health and success of a data project. What are some of the current utilities that are available to data engineers for building and executing tests to cover the data lifecycle, from collection through to analysis and delivery?
What are some of the components of a data analytics lifecycle that are resistant to agile or iterative development?
With the continued rise in the use of machine learning in production, how does that change the requirements for delivery and maintenance of an analytics platform?
What are some of the trends that you are most excited for in the analytics and data platform space?
Contact Info
Data Kitchen
Email
Chris
LinkedIn
@ChrisBergh on Twitter
Parting Question
From your perspective, what is the biggest gap in the tooling or technology for data management today?
Links
Download the "DataOps Cookbook"
Data Kitchen
Peace Corps
MIT
NASA
Meyer’s Briggs Personality Test
HBR (Harvard Business Review)
MBA (Master of Business Administration)
W. Edwards Deming
DevOps
Lean Manufacturing
Tableau
Excel
Airflow
Podcast.init Interview
Looker
Podcast Interview
R Language
Alteryx
Data Lake
Data Literacy
Data Governance
Datadog
Kubernetes
Kubeflow
Metis Machine
Gartner Hype Cycle
The intro and outro music is from The Hug by The Freak Fandango Orchestra / CC BY-SA
Support Data Engineering Podcast
54:3118/03/2019
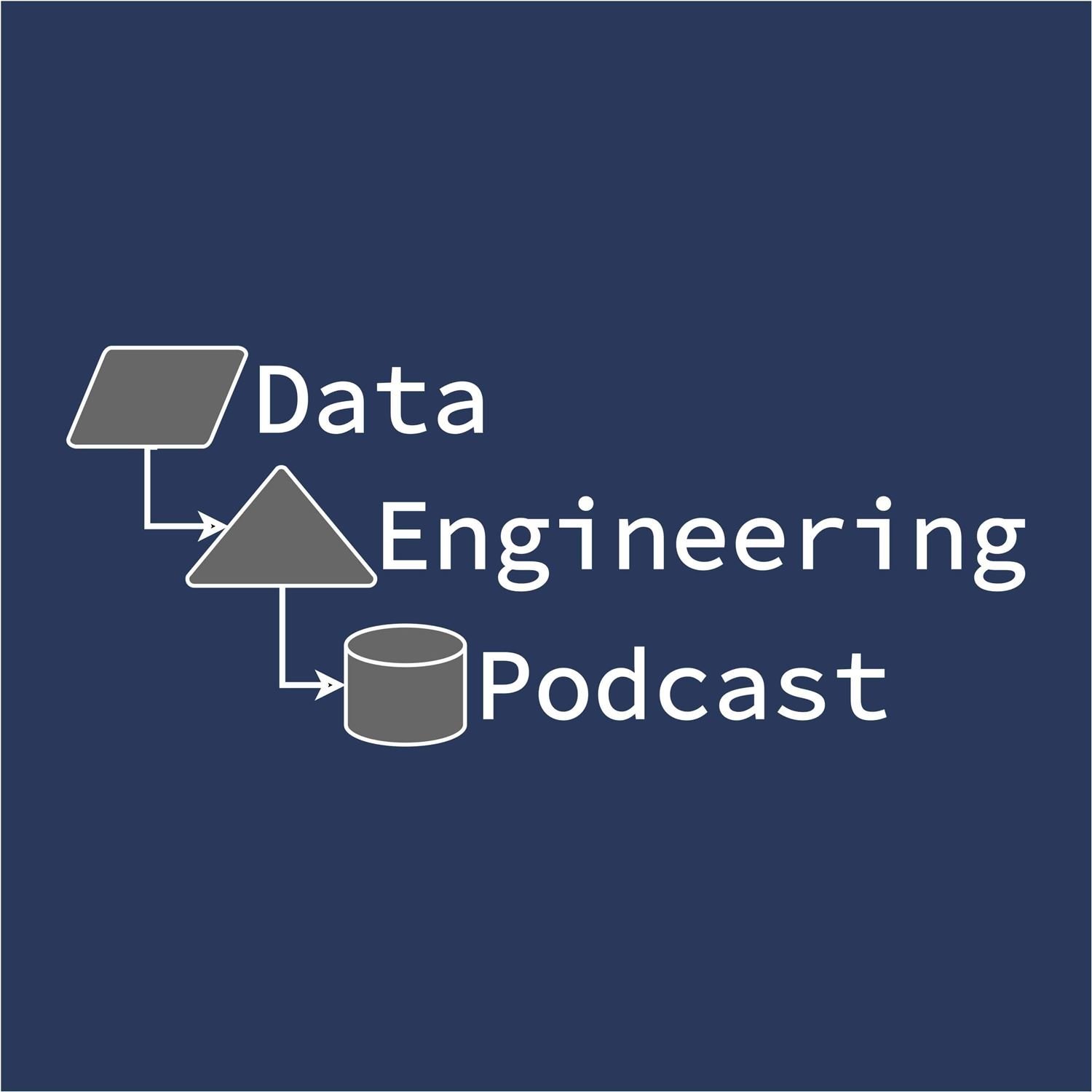
Customer Analytics At Scale With Segment
Summary
Customer analytics is a problem domain that has given rise to its own industry. In order to gain a full understanding of what your users are doing and how best to serve them you may need to send data to multiple services, each with their own tracking code or APIs. To simplify this process and allow your non-engineering employees to gain access to the information they need to do their jobs Segment provides a single interface for capturing data and routing it to all of the places that you need it. In this interview Segment CTO and co-founder Calvin French-Owen explains how the company got started, how it manages to multiplex data streams from multiple sources to multiple destinations, and how it can simplify your work of gaining visibility into how your customers are engaging with your business.
Announcements
Hello and welcome to the Data Engineering Podcast, the show about modern data management
When you’re ready to build your next pipeline, or want to test out the projects you hear about on the show, you’ll need somewhere to deploy it, so check out our friends at Linode. With 200Gbit private networking, scalable shared block storage, and a 40Gbit public network, you’ve got everything you need to run a fast, reliable, and bullet-proof data platform. If you need global distribution, they’ve got that covered too with world-wide datacenters including new ones in Toronto and Mumbai. And for your machine learning workloads, they just announced dedicated CPU instances. Go to dataengineeringpodcast.com/linode today to get a $20 credit and launch a new server in under a minute. And don’t forget to thank them for their continued support of this show!
Managing and auditing access to your servers and databases is a problem that grows in difficulty alongside the growth of your teams. If you are tired of wasting your time cobbling together scripts and workarounds to give your developers, data scientists, and managers the permissions that they need then it’s time to talk to our friends at strongDM. They have built an easy to use platform that lets you leverage your company’s single sign on for your data platform. Go to dataengineeringpodcast.com/strongdm today to find out how you can simplify your systems.
Go to dataengineeringpodcast.com to subscribe to the show, sign up for the mailing list, read the show notes, and get in touch.
To help other people find the show please leave a review on iTunes and tell your friends and co-workers
You listen to this show to learn and stay up to date with what’s happening in databases, streaming platforms, big data, and everything else you need to know about modern data management. For even more opportunities to meet, listen, and learn from your peers you don’t want to miss out on this year’s conference season. We have partnered with O’Reilly Media for the Strata conference in San Francisco on March 25th and the Artificial Intelligence conference in NYC on April 15th. Here in Boston, starting on May 17th, you still have time to grab a ticket to the Enterprise Data World, and from April 30th to May 3rd is the Open Data Science Conference. Go to dataengineeringpodcast.com/conferences to learn more and take advantage of our partner discounts when you register.
Your host is Tobias Macey and today I’m interviewing Calvin French-Owen about the data platform that Segment has built to handle multiplexing continuous streams of data from multiple sources to multiple destinations
Interview
Introduction
How did you get involved in the area of data management?
Can you start by explaining what Segment is and how the business got started?
What are some of the primary ways that your customers are using the Segment platform?
How have the capabilities and use cases of the Segment platform changed since it was first launched?
Layered on top of the data integration platform you have added the concepts of Protocols and Personas. Can you explain how each of those products fit into the overall structure of Segment and the driving force behind their design and use?
What are some of the best practices for structuring custom events in a way that they can be easily integrated with downstream platforms?
How do you manage changes or errors in the events generated by the various sources that you support?
How is the Segment platform architected and how has that architecture evolved over the past few years?
What are some of the unique challenges that you face as a result of being a many-to-many event routing platform?
In addition to the various services that you integrate with for data delivery, you also support populating of data warehouses. What is involved in establishing and maintaining the schema and transformations for a customer?
What have been some of the most interesting, unexpected, and/or challenging lessons that you have learned while building and growing the technical and business aspects of Segment?
What are some of the features and improvements, both technical and business, that you have planned for the future?
Contact Info
LinkedIn
@calvinfo on Twitter
Website
calvinfo on GitHub
Parting Question
From your perspective, what is the biggest gap in the tooling or technology for data management today?
Links
Segment
AWS
ClassMetric
Y Combinator
Amplitude web and mobile analytics
Mixpanel
Kiss Metrics
Hacker News
Segment Connections
User Analytics
SalesForce
Redshift
BigQuery
Kinesis
Google Cloud PubSub
Segment Protocols data governance product
Segment Personas
Heap Analytics
Podcast Episode
Hotel Tonight
Golang
Kafka
GDPR
RocksDB
Dead Letter Queue
Segment Centrifuge
Webhook
Google Analytics
Intercom
Stripe
GRPC
DynamoDB
FoundationDB
Parquet
Podcast Episode
The intro and outro music is from The Hug by The Freak Fandango Orchestra / CC BY-SA
Support Data Engineering Podcast
47:4704/03/2019
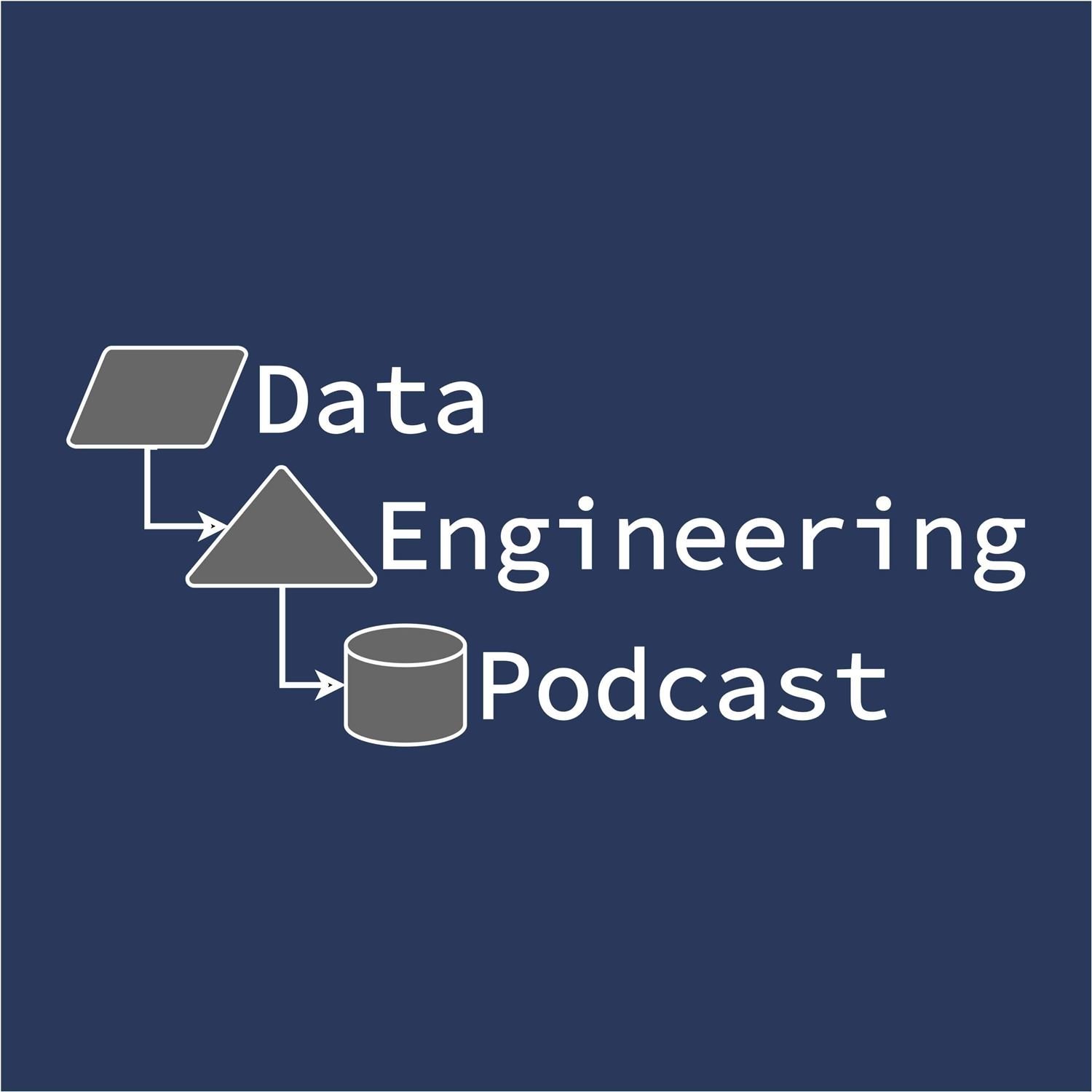
Deep Learning For Data Engineers
Summary
Deep learning is the latest class of technology that is gaining widespread interest. As data engineers we are responsible for building and managing the platforms that power these models. To help us understand what is involved, we are joined this week by Thomas Henson. In this episode he shares his experiences experimenting with deep learning, what data engineers need to know about the infrastructure and data requirements to power the models that your team is building, and how it can be used to supercharge our ETL pipelines.
Announcements
Hello and welcome to the Data Engineering Podcast, the show about modern data management
When you’re ready to build your next pipeline, or want to test out the projects you hear about on the show, you’ll need somewhere to deploy it, so check out our friends at Linode. With 200Gbit private networking, scalable shared block storage, and a 40Gbit public network, you’ve got everything you need to run a fast, reliable, and bullet-proof data platform. If you need global distribution, they’ve got that covered too with world-wide datacenters including new ones in Toronto and Mumbai. Go to dataengineeringpodcast.com/linode today to get a $20 credit and launch a new server in under a minute. And don’t forget to thank them for their continued support of this show!
Managing and auditing access to your servers and databases is a problem that grows in difficulty alongside the growth of your teams. If you are tired of wasting your time cobbling together scripts and workarounds to give your developers, data scientists, and managers the permissions that they need then it’s time to talk to our friends at strongDM. They have built an easy to use platform that lets you leverage your company’s single sign on for your data platform. Go to dataengineeringpodcast.com/strongdm today to find out how you can simplify your systems.
Go to dataengineeringpodcast.com to subscribe to the show, sign up for the mailing list, read the show notes, and get in touch.
To help other people find the show please leave a review on iTunes, or Google Play Music, tell your friends and co-workers, and share it on social media.
Join the community in the new Zulip chat workspace at dataengineeringpodcast.com/chat
You listen to this show to learn and stay up to date with what’s happening in databases, streaming platforms, big data, and everything else you need to know about modern data platforms. For even more opportunities to meet, listen, and learn from your peers you don’t want to miss the Strata conference in San Francisco on March 25th and the Artificial Intelligence conference in NYC on April 15th, both run by our friends at O’Reilly Media. Go to dataengineeringpodcast.com/stratacon and dataengineeringpodcast.com/aicon to register today and get 20% off
Your host is Tobias Macey and today I’m interviewing Thomas Henson about what data engineers need to know about deep learning, including how to use it for their own projects
Interview
Introduction
How did you get involved in the area of data management?
Can you start by giving an overview of what deep learning is for anyone who isn’t familiar with it?
What has been your personal experience with deep learning and what set you down that path?
What is involved in building a data pipeline and production infrastructure for a deep learning product?
How does that differ from other types of analytics projects such as data warehousing or traditional ML?
For anyone who is in the early stages of a deep learning project, what are some of the edge cases or gotchas that they should be aware of?
What are your opinions on the level of involvement/understanding that data engineers should have with the analytical products that are being built with the information we collect and curate?
What are some ways that we can use deep learning as part of the data management process?
How does that shift the infrastructure requirements for our platforms?
Cloud providers have been releasing numerous products to provide deep learning and/or GPUs as a managed platform. What are your thoughts on that layer of the build vs buy decision?
What is your litmus test for whether to use deep learning vs explicit ML algorithms or a basic decision tree?
Deep learning algorithms are often a black box in terms of how decisions are made, however regulations such as GDPR are introducing requirements to explain how a given decision gets made. How does that factor into determining what approach to take for a given project?
For anyone who wants to learn more about deep learning, what are some resources that you recommend?
Contact Info
Website
Pluralsight
@henson_tm on Twitter
Parting Question
From your perspective, what is the biggest gap in the tooling or technology for data management today?
Links
Pluralsight
Dell EMC
Hadoop
DBA (Database Administrator)
Elasticsearch
Podcast Episode
Spark
Podcast Episode
MapReduce
Deep Learning
Machine Learning
Neural Networks
Feature Engineering
SVD (Singular Value Decomposition)
Andrew Ng
Machine Learning Course
Unstructured Data Solutions Team of Dell EMC
Tensorflow
PyTorch
GPU (Graphics Processing Unit)
Nvidia RAPIDS
Project Hydrogen
Submarine
ETL (Extract, Transform, Load)
Supervised Learning
Unsupervised Learning
Apache Kudu
Podcast Episode
CNN (Convolutional Neural Network)
Sentiment Analysis
DataRobot
GDPR
Weapons Of Math Destruction by Cathy O’Neil
Backpropagation
Deep Learning Bootcamps
Thomas Henson Tensorflow Course on Pluralsight
TFLearn
Google ML Bootcamp
Caffe deep learning framework
The intro and outro music is from The Hug by The Freak Fandango Orchestra / CC BY-SA
Support Data Engineering Podcast
42:4625/02/2019
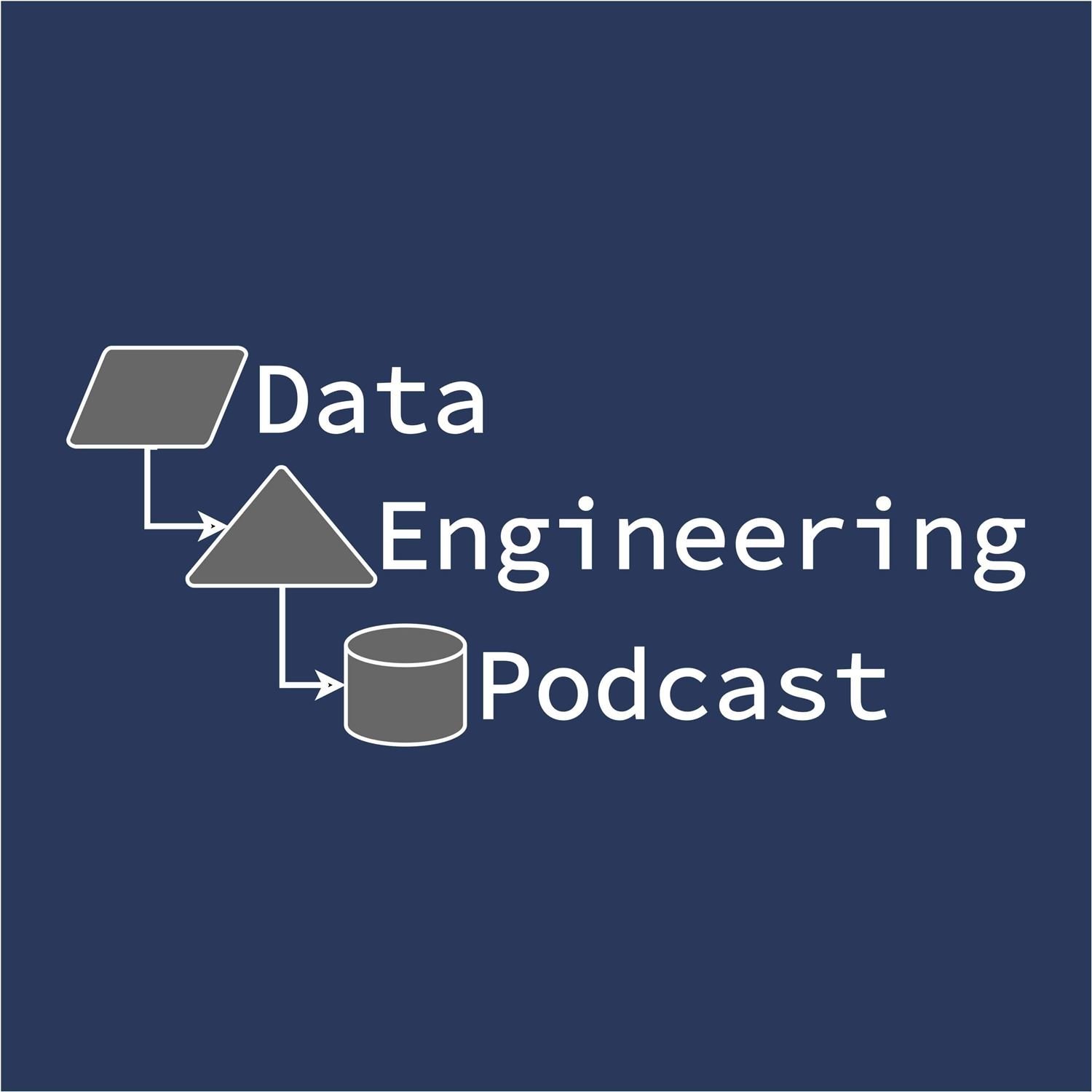
Speed Up Your Analytics With The Alluxio Distributed Storage System
Summary
Distributed storage systems are the foundational layer of any big data stack. There are a variety of implementations which support different specialized use cases and come with associated tradeoffs. Alluxio is a distributed virtual filesystem which integrates with multiple persistent storage systems to provide a scalable, in-memory storage layer for scaling computational workloads independent of the size of your data. In this episode Bin Fan explains how he got involved with the project, how it is implemented, and the use cases that it is particularly well suited for. If your storage and compute layers are too tightly coupled and you want to scale them independently then Alluxio is the tool for the job.
Introduction
Hello and welcome to the Data Engineering Podcast, the show about modern data management
When you’re ready to build your next pipeline, or want to test out the projects you hear about on the show, you’ll need somewhere to deploy it, so check out Linode. With 200Gbit private networking, scalable shared block storage, and a 40Gbit public network, you’ve got everything you need to run a fast, reliable, and bullet-proof data platform. If you need global distribution, they’ve got that covered too with world-wide datacenters including new ones in Toronto and Mumbai. Go to dataengineeringpodcast.com/linode today to get a $20 credit and launch a new server in under a minute.
Go to dataengineeringpodcast.com to subscribe to the show, sign up for the mailing list, read the show notes, and get in touch.
To help other people find the show please leave a review on iTunes, or Google Play Music, tell your friends and co-workers, and share it on social media.
Join the community in the new Zulip chat workspace at dataengineeringpodcast.com/chat
Your host is Tobias Macey and today I’m interviewing Bin Fan about Alluxio, a distributed virtual filesystem for unified access to disparate data sources
Interview
Introduction
How did you get involved in the area of data management?
Can you start by explaining what Alluxio is and the history of the project?
What are some of the use cases that Alluxio enables?
How is Alluxio implemented and how has its architecture evolved over time?
What are some of the techniques that you use to mitigate the impact of latency, particularly when interfacing with storage systems across cloud providers and private data centers?
When dealing with large volumes of data over time it is often necessary to age out older records to cheaper storage. What capabilities does Alluxio provide for that lifecycle management?
What are some of the most complex or challenging aspects of providing a unified abstraction across disparate storage platforms?
What are the tradeoffs that are made to provide a single API across systems with varying capabilities?
Testing and verification of distributed systems is a complex undertaking. Can you describe the approach that you use to ensure proper functionality of Alluxio as part of the development and release process?
In order to allow for this large scale testing with any regularity it must be straightforward to deploy and configure Alluxio. What are some of the mechanisms that you have built into the platform to simplify the operational aspects?
Can you describe a typical system topology that incorporates Alluxio?
For someone planning a deployment of Alluxio, what should they be considering in terms of system requirements and deployment topologies?
What are some edge cases or operational complexities that they should be aware of?
What are some cases where Alluxio is the wrong choice?
What are some projects or products that provide a similar capability to Alluxio?
What do you have planned for the future of the Alluxio project and company?
Contact Info
LinkedIn
@binfan on Twitter
Parting Question
From your perspective, what is the biggest gap in the tooling or technology for data management today?
Links
Alluxio
Project
Company
Carnegie Mellon University
Memcached
Key/Value Storage
UC Berkeley AMPLab
Apache Spark
Podcast Episode
Presto
Podcast Episode
Tensorflow
HDFS
LRU Cache
Hive Metastore
Iceberg Table Format
Podcast Episode
Java
Dependency Hell
Java Class Loader
Apache Zookeeper
Podcast Interview
Raft Consensus Algorithm
Consistent Hashing
Alluxio Testing At Scale Blog Post
S3Guard
The intro and outro music is from The Hug by The Freak Fandango Orchestra / CC BY-SA
Support Data Engineering Podcast
59:4419/02/2019
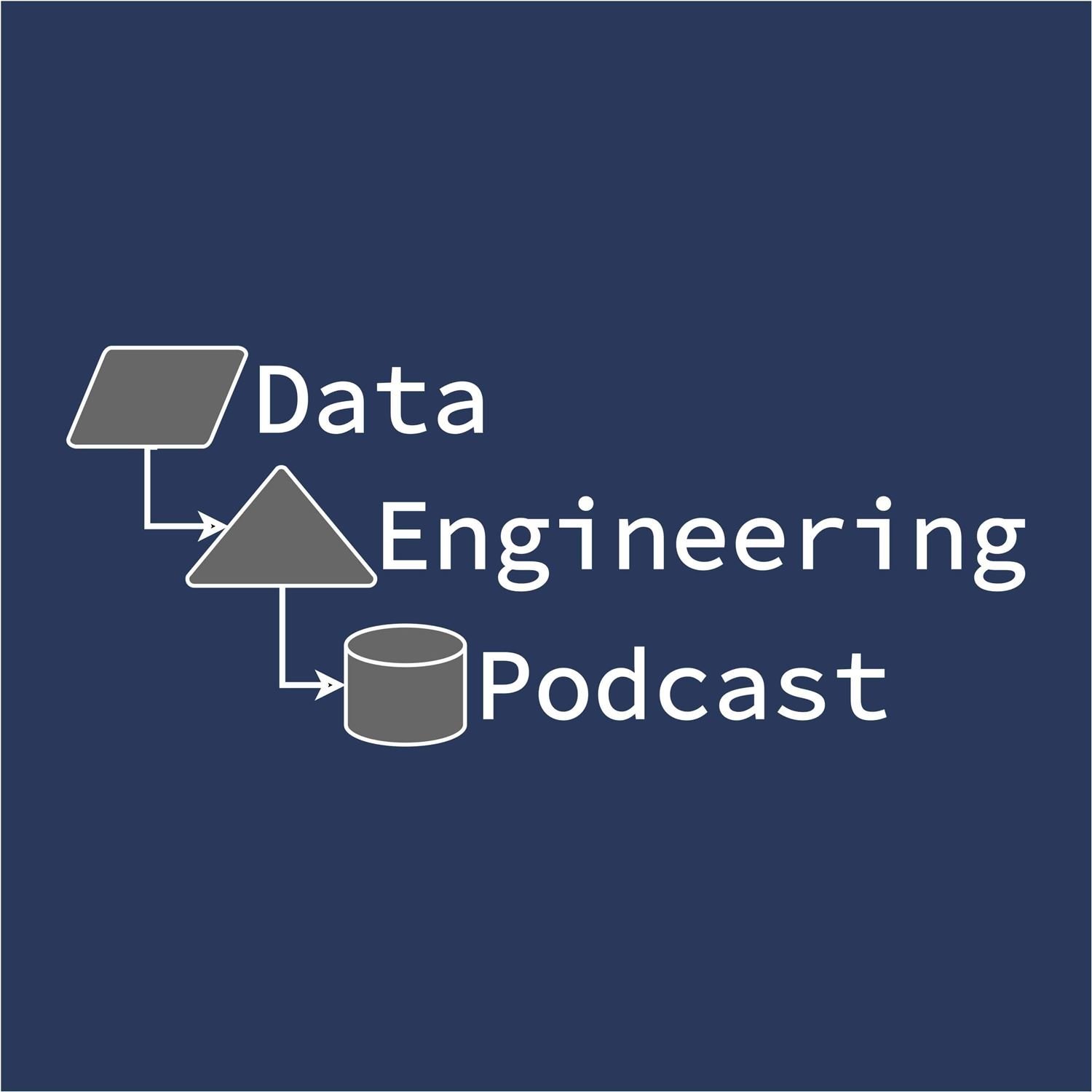
Machine Learning In The Enterprise
Summary
Machine learning is a class of technologies that promise to revolutionize business. Unfortunately, it can be difficult to identify and execute on ways that it can be used in large companies. Kevin Dewalt founded Prolego to help Fortune 500 companies build, launch, and maintain their first machine learning projects so that they can remain competitive in our landscape of constant change. In this episode he discusses why machine learning projects require a new set of capabilities, how to build a team from internal and external candidates, and how an example project progressed through each phase of maturity. This was a great conversation for anyone who wants to understand the benefits and tradeoffs of machine learning for their own projects and how to put it into practice.
Introduction
Hello and welcome to the Data Engineering Podcast, the show about modern data management
When you’re ready to build your next pipeline, or want to test out the projects you hear about on the show, you’ll need somewhere to deploy it, so check out Linode. With 200Gbit private networking, scalable shared block storage, and a 40Gbit public network, you’ve got everything you need to run a fast, reliable, and bullet-proof data platform. If you need global distribution, they’ve got that covered too with world-wide datacenters including new ones in Toronto and Mumbai. Go to dataengineeringpodcast.com/linode today to get a $20 credit and launch a new server in under a minute.
Go to dataengineeringpodcast.com to subscribe to the show, sign up for the mailing list, read the show notes, and get in touch.
To help other people find the show please leave a review on iTunes, or Google Play Music, tell your friends and co-workers, and share it on social media.
Join the community in the new Zulip chat workspace at dataengineeringpodcast.com/chat
Your host is Tobias Macey and today I’m interviewing Kevin Dewalt about his experiences at Prolego, building machine learning projects for Fortune 500 companies
Interview
Introduction
How did you get involved in the area of data management?
For the benefit of software engineers and team leaders who are new to machine learning, can you briefly describe what machine learning is and why is it relevant to them?
What is your primary mission at Prolego and how did you identify, execute on, and establish a presence in your particular market?
How much of your sales process is spent on educating your clients about what AI or ML are and the benefits that these technologies can provide?
What have you found to be the technical skills and capacity necessary for being successful in building and deploying a machine learning project?
When engaging with a client, what have you found to be the most common areas of technical capacity or knowledge that are needed?
Everyone talks about a talent shortage in machine learning. Can you suggest a recruiting or skills development process for companies which need to build out their data engineering practice?
What challenges will teams typically encounter when creating an efficient working relationship between data scientists and data engineers?
Can you briefly describe a successful project of developing a first ML model and putting it into production?
What is the breakdown of how much time was spent on different activities such as data wrangling, model development, and data engineering pipeline development?
When releasing to production, can you share the types of metrics that you track to ensure the health and proper functioning of the models?
What does a deployable artifact for a machine learning/deep learning application look like?
What basic technology stack is necessary for putting the first ML models into production?
How does the build vs. buy debate break down in this space and what products do you typically recommend to your clients?
What are the major risks associated with deploying ML models and how can a team mitigate them?
Suppose a software engineer wants to break into ML. What data engineering skills would you suggest they learn? How should they position themselves for the right opportunity?
Contact Info
Email: Kevin Dewalt [email protected] and Russ Rands [email protected]
Connect on LinkedIn: Kevin Dewalt and Russ Rands
Twitter: @kevindewalt
Parting Question
From your perspective, what is the biggest gap in the tooling or technology for data management today?
Links
Prolego
Download our book: Become an AI Company in 90 Days
Google Rules Of ML
AI Winter
Machine Learning
Supervised Learning
O’Reilly Strata Conference
GE Rebranding Commercials
Jez Humble: Stop Hiring Devops Experts (And Start Growing Them)
SQL
ORM
Django
RoR
Tensorflow
PyTorch
Keras
Data Engineering Podcast Episode About Data Teams
DevOps For Data Teams – DevOps Days Boston Presentation by Tobias
Jupyter Notebook
Data Engineering Podcast: Notebooks at Netflix
Pandas
Podcast Interview
Joel Grus
JupyterCon Presentation
Data Science From Scratch
Expensify
Airflow
James Meickle Interview
Git
Jenkins
Continuous Integration
Practical Deep Learning For Coders Course by Jeremy Howard
Data Carpentry
The intro and outro music is from The Hug by The Freak Fandango Orchestra / CC BY-SA
Support Data Engineering Podcast
48:1911/02/2019
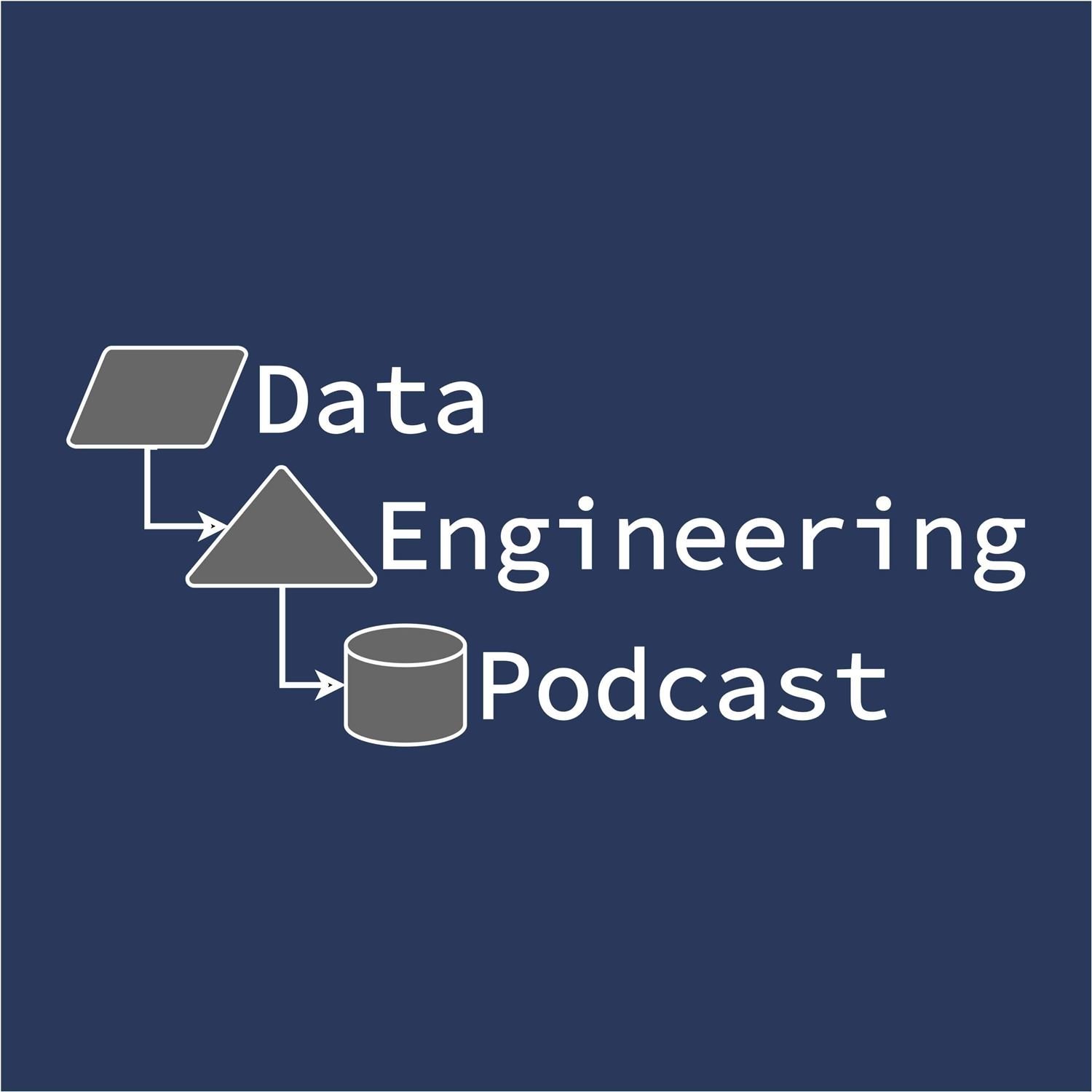
Cleaning And Curating Open Data For Archaeology
Summary
Archaeologists collect and create a variety of data as part of their research and exploration. Open Context is a platform for cleaning, curating, and sharing this data. In this episode Eric Kansa describes how they process, clean, and normalize the data that they host, the challenges that they face with scaling ETL processes which require domain specific knowledge, and how the information contained in connections that they expose is being used for interesting projects.
Introduction
Hello and welcome to the Data Engineering Podcast, the show about modern data management
When you’re ready to build your next pipeline, or want to test out the projects you hear about on the show, you’ll need somewhere to deploy it, so check out Linode. With 200Gbit private networking, scalable shared block storage, and a 40Gbit public network, you’ve got everything you need to run a fast, reliable, and bullet-proof data platform. If you need global distribution, they’ve got that covered too with world-wide datacenters including new ones in Toronto and Mumbai. Go to dataengineeringpodcast.com/linode today to get a $20 credit and launch a new server in under a minute.
Go to dataengineeringpodcast.com to subscribe to the show, sign up for the mailing list, read the show notes, and get in touch.
To help other people find the show please leave a review on iTunes, or Google Play Music, tell your friends and co-workers, and share it on social media.
Join the community in the new Zulip chat workspace at dataengineeringpodcast.com/chat
Your host is Tobias Macey and today I’m interviewing Eric Kansa about Open Context, a platform for publishing, managing, and sharing research data
Interview
Introduction
How did you get involved in the area of data management?
I did some database and GIS work for my dissertation in archaeology, back in the late 1990’s. I got frustrated at the lack of comparative data, and I got frustrated at all the work I put into creating data that nobody would likely use. So I decided to focus my energies in research data management.
Can you start by describing what Open Context is and how it started?
Open Context is an open access data publishing service for archaeology. It started because we need better ways of dissminating structured data and digital media than is possible with conventional articles, books and reports.
What are your protocols for determining which data sets you will work with?
Datasets need to come from research projects that meet the normal standards of professional conduct (laws, ethics, professional norms) articulated by archaeology’s professional societies.
What are some of the challenges unique to research data?
What are some of the unique requirements for processing, publishing, and archiving research data?
You have to work on a shoe-string budget, essentially providing "public goods". Archaeologists typically don’t have much discretionary money available, and publishing and archiving data are not yet very common practices.
Another issues is that it will take a long time to publish enough data to power many "meta-analyses" that draw upon many datasets. The issue is that lots of archaeological data describes very particular places and times. Because datasets can be so particularistic, finding data relevant to your interests can be hard. So, we face a monumental task in supplying enough data to satisfy many, many paricularistic interests.
How much education is necessary around your content licensing for researchers who are interested in publishing their data with you?
We require use of Creative Commons licenses, and greatly encourage the CC-BY license or CC-Zero (public domain) to try to keep things simple and easy to understand.
Can you describe the system architecture that you use for Open Context?
Open Context is a Django Python application, with a Postgres database and an Apache Solr index. It’s running on Google cloud services on a Debian linux.
What is the process for cleaning and formatting the data that you host?
How much domain expertise is necessary to ensure proper conversion of the source data?
That’s one of the bottle necks. We have to do an ETL (extract transform load) on each dataset researchers submit for publication. Each dataset may need lots of cleaning and back and forth conversations with data creators.
Can you discuss the challenges that you face in maintaining a consistent ontology?
What pieces of metadata do you track for a given data set?
Can you speak to the average size of data sets that you manage and any approach that you use to optimize for cost of storage and processing capacity?
Can you walk through the lifecycle of a given data set?
Data archiving is a complicated and difficult endeavor due to issues pertaining to changing data formats and storage media, as well as repeatability of computing environments to generate and/or process them. Can you discuss the technical and procedural approaches that you take to address those challenges?
Once the data is stored you expose it for public use via a set of APIs which support linked data. Can you discuss any complexities that arise from needing to identify and expose interrelations between the data sets?
What are some of the most interesting uses you have seen of the data that is hosted on Open Context?
What have been some of the most interesting/useful/challenging lessons that you have learned while working on Open Context?
What are your goals for the future of Open Context?
Contact Info
@ekansa on Twitter
LinkedIn
ResearchGate
Parting Question
From your perspective, what is the biggest gap in the tooling or technology for data management today?
Links
Open Context
Bronze Age
GIS (Geographic Information System)
Filemaker
Access Database
Excel
Creative Commons
Open Context On Github
Django
PostgreSQL
Apache Solr
GeoJSON
JSON-LD
RDF
OCHRE
SKOS (Simple Knowledge Organization System)
Django Reversion
California Digital Library
Zenodo
CERN
Digital Index of North American Archaeology (DINAA)
Ansible
Docker
OpenRefine
The intro and outro music is from The Hug by The Freak Fandango Orchestra / CC BY-SA
Support Data Engineering Podcast
01:00:5604/02/2019
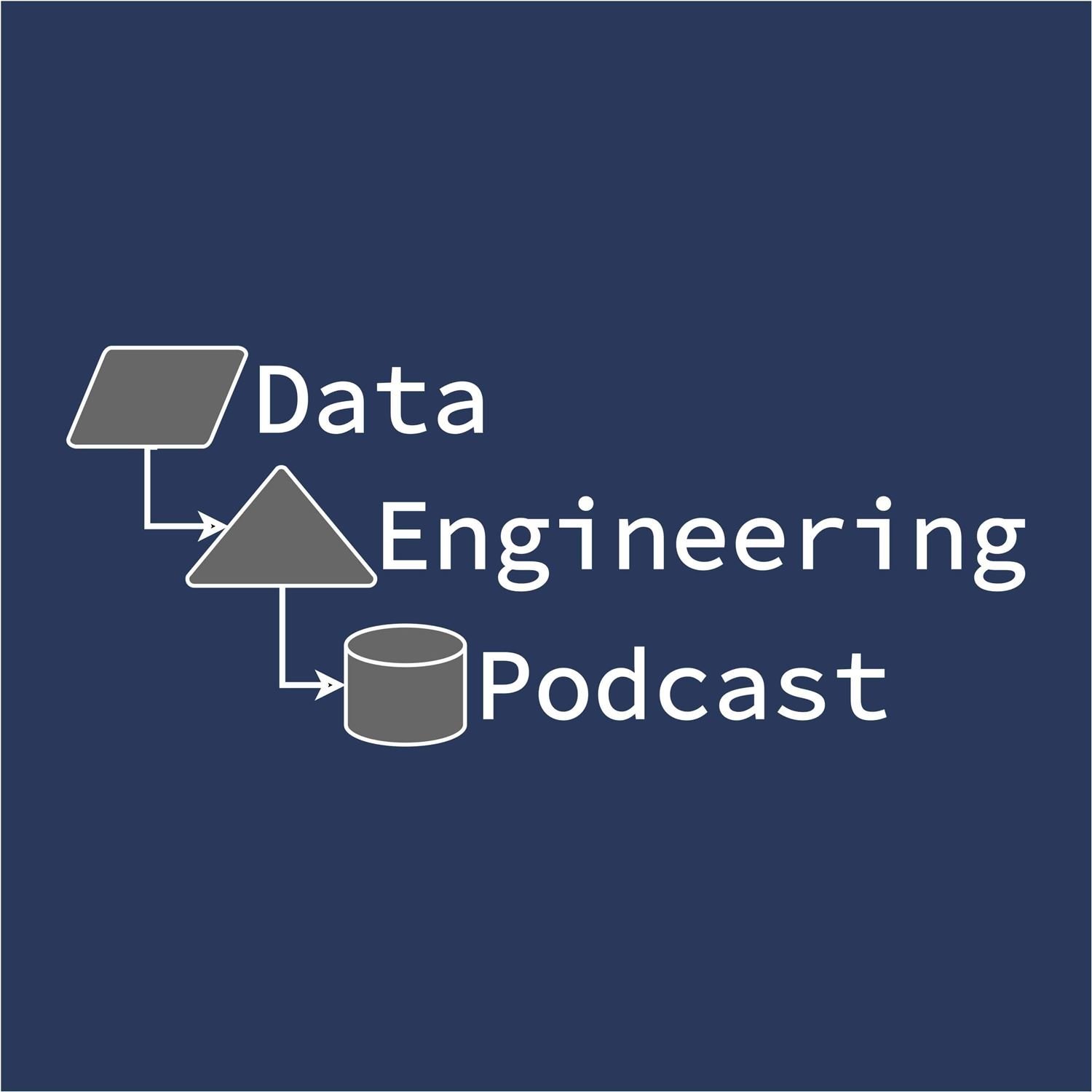
Managing Database Access Control For Teams With strongDM
Summary
Controlling access to a database is a solved problem… right? It can be straightforward for small teams and a small number of storage engines, but once either or both of those start to scale then things quickly become complex and difficult to manage. After years of running across the same issues in numerous companies and even more projects Justin McCarthy built strongDM to solve database access management for everyone. In this episode he explains how the strongDM proxy works to grant and audit access to storage systems and the benefits that it provides to engineers and team leads.
Introduction
Hello and welcome to the Data Engineering Podcast, the show about modern data management
When you’re ready to build your next pipeline, or want to test out the projects you hear about on the show, you’ll need somewhere to deploy it, so check out Linode. With 200Gbit private networking, scalable shared block storage, and a 40Gbit public network, you’ve got everything you need to run a fast, reliable, and bullet-proof data platform. If you need global distribution, they’ve got that covered too with world-wide datacenters including new ones in Toronto and Mumbai. Go to dataengineeringpodcast.com/linode today to get a $20 credit and launch a new server in under a minute.
Go to dataengineeringpodcast.com to subscribe to the show, sign up for the mailing list, read the show notes, and get in touch.
To help other people find the show please leave a review on iTunes, or Google Play Music, tell your friends and co-workers, and share it on social media.
Join the community in the new Zulip chat workspace at dataengineeringpodcast.com/chat
Your host is Tobias Macey and today I’m interviewing Justin McCarthy about StrongDM, a hosted service that simplifies access controls for your data
Interview
Introduction
How did you get involved in the area of data management?
Can you start by explaining the problem that StrongDM is solving and how the company got started?
What are some of the most common challenges around managing access and authentication for data storage systems?
What are some of the most interesting workarounds that you have seen?
Which areas of authentication, authorization, and auditing are most commonly overlooked or misunderstood?
Can you describe the architecture of your system?
What strategies have you used to enable interfacing with such a wide variety of storage systems?
What additional capabilities do you provide beyond what is natively available in the underlying systems?
What are some of the most difficult aspects of managing varying levels of permission for different roles across the diversity of platforms that you support, given that they each have different capabilities natively?
For a customer who is onboarding, what is involved in setting up your platform to integrate with their systems?
What are some of the assumptions that you made about your problem domain and market when you first started which have been disproven?
How do organizations in different industries react to your product and how do their policies around granting access to data differ?
What are some of the most interesting/unexpected/challenging lessons that you have learned in the process of building and growing StrongDM?
Contact Info
LinkedIn
@justinm on Twitter
Parting Question
From your perspective, what is the biggest gap in the tooling or technology for data management today?
Links
StrongDM
Authentication Vs. Authorization
Hashicorp Vault
Configuration Management
Chef
Puppet
SaltStack
Ansible
Okta
SSO (Single Sign On
SOC 2
Two Factor Authentication
SSH (Secure SHell)
RDP
The intro and outro music is from The Hug by The Freak Fandango Orchestra / CC BY-SA
Support Data Engineering Podcast
42:1829/01/2019
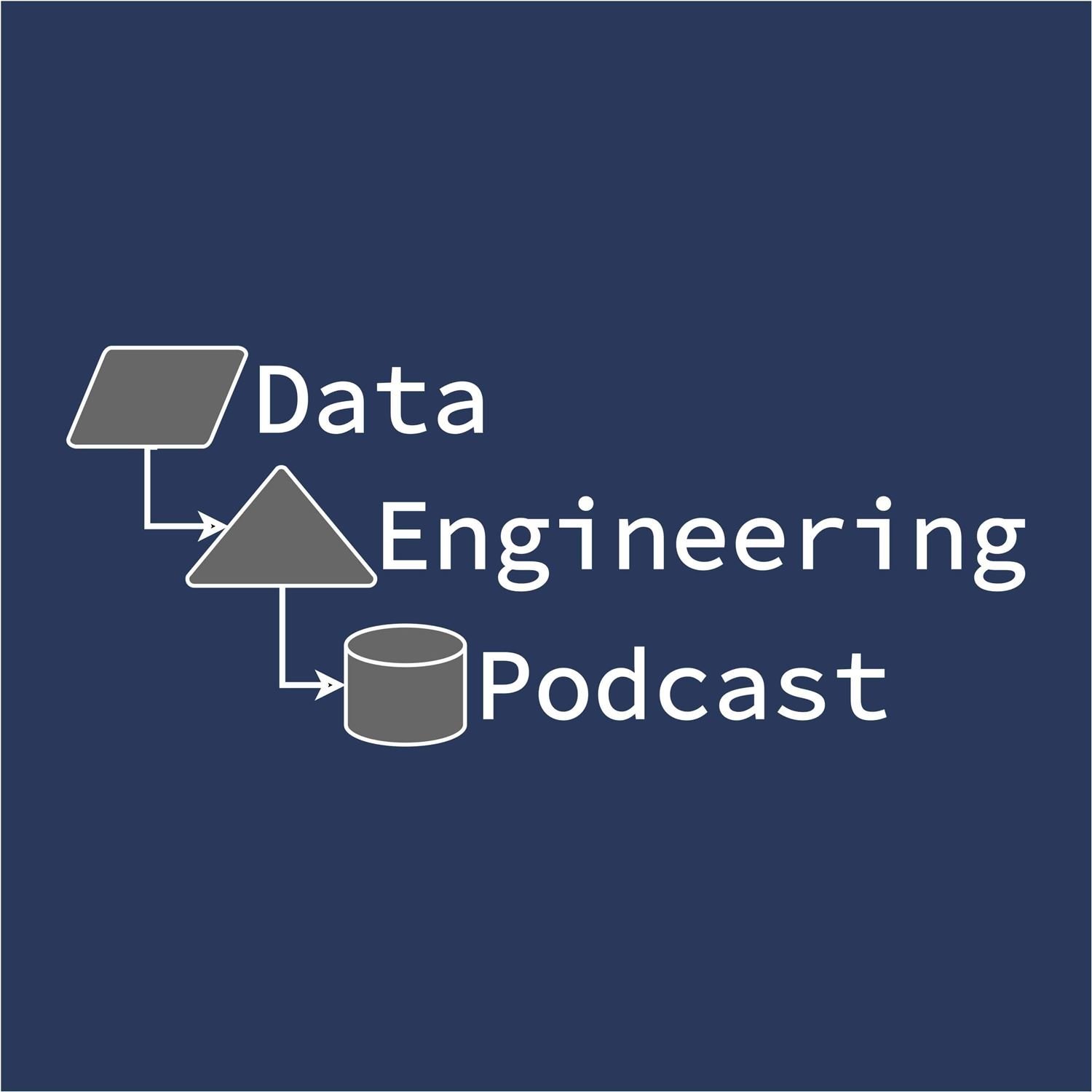
Building Enterprise Big Data Systems At LEGO
Summary
Building internal expertise around big data in a large organization is a major competitive advantage. However, it can be a difficult process due to compliance needs and the need to scale globally on day one. In this episode Jesper Søgaard and Keld Antonsen share the story of starting and growing the big data group at LEGO. They discuss the challenges of being at global scale from the start, hiring and training talented engineers, prototyping and deploying new systems in the cloud, and what they have learned in the process. This is a useful conversation for engineers, managers, and leadership who are interested in building enterprise big data systems.
Preamble
Hello and welcome to the Data Engineering Podcast, the show about modern data management
When you’re ready to build your next pipeline, or want to test out the projects you hear about on the show, you’ll need somewhere to deploy it, so check out Linode. With 200Gbit private networking, scalable shared block storage, and a 40Gbit public network, you’ve got everything you need to run a fast, reliable, and bullet-proof data platform. If you need global distribution, they’ve got that covered too with world-wide datacenters including new ones in Toronto and Mumbai. Go to dataengineeringpodcast.com/linode today to get a $20 credit and launch a new server in under a minute.
Go to dataengineeringpodcast.com to subscribe to the show, sign up for the mailing list, read the show notes, and get in touch.
To help other people find the show please leave a review on iTunes, or Google Play Music, tell your friends and co-workers, and share it on social media.
Join the community in the new Zulip chat workspace at dataengineeringpodcast.com/chat
Your host is Tobias Macey and today I’m interviewing Keld Antonsen and Jesper Soegaard about the data infrastructure and analytics that powers LEGO
Interview
Introduction
How did you get involved in the area of data management?
My understanding is that the big data group at LEGO is a fairly recent development. Can you share the story of how it got started?
What kinds of data practices were in place prior to starting a dedicated group for managing the organization’s data?
What was the transition process like, migrating data silos into a uniformly managed platform?
What are the biggest data challenges that you face at LEGO?
What are some of the most critical sources and types of data that you are managing?
What are the main components of the data infrastructure that you have built to support the organizations analytical needs?
What are some of the technologies that you have found to be most useful?
Which have been the most problematic?
What does the team structure look like for the data services at LEGO?
Does that reflect in the types/numbers of systems that you support?
What types of testing, monitoring, and metrics do you use to ensure the health of the systems you support?
What have been some of the most interesting, challenging, or useful lessons that you have learned while building and maintaining the data platforms at LEGO?
How have the data systems at Lego evolved over recent years as new technologies and techniques have been developed?
How does the global nature of the LEGO business influence the design strategies and technology choices for your platform?
What are you most excited for in the coming year?
Contact Info
Jesper
LinkedIn
Keld
LinkedIn
Parting Question
From your perspective, what is the biggest gap in the tooling or technology for data management today?
Links
LEGO Group
ERP (Enterprise Resource Planning)
Predictive Analytics
Prescriptive Analytics
Hadoop
Center Of Excellence
Continuous Integration
Spark
Podcast Episode
Apache NiFi
Podcast Episode
The intro and outro music is from The Hug by The Freak Fandango Orchestra / CC BY-SA
Support Data Engineering Podcast
48:0421/01/2019
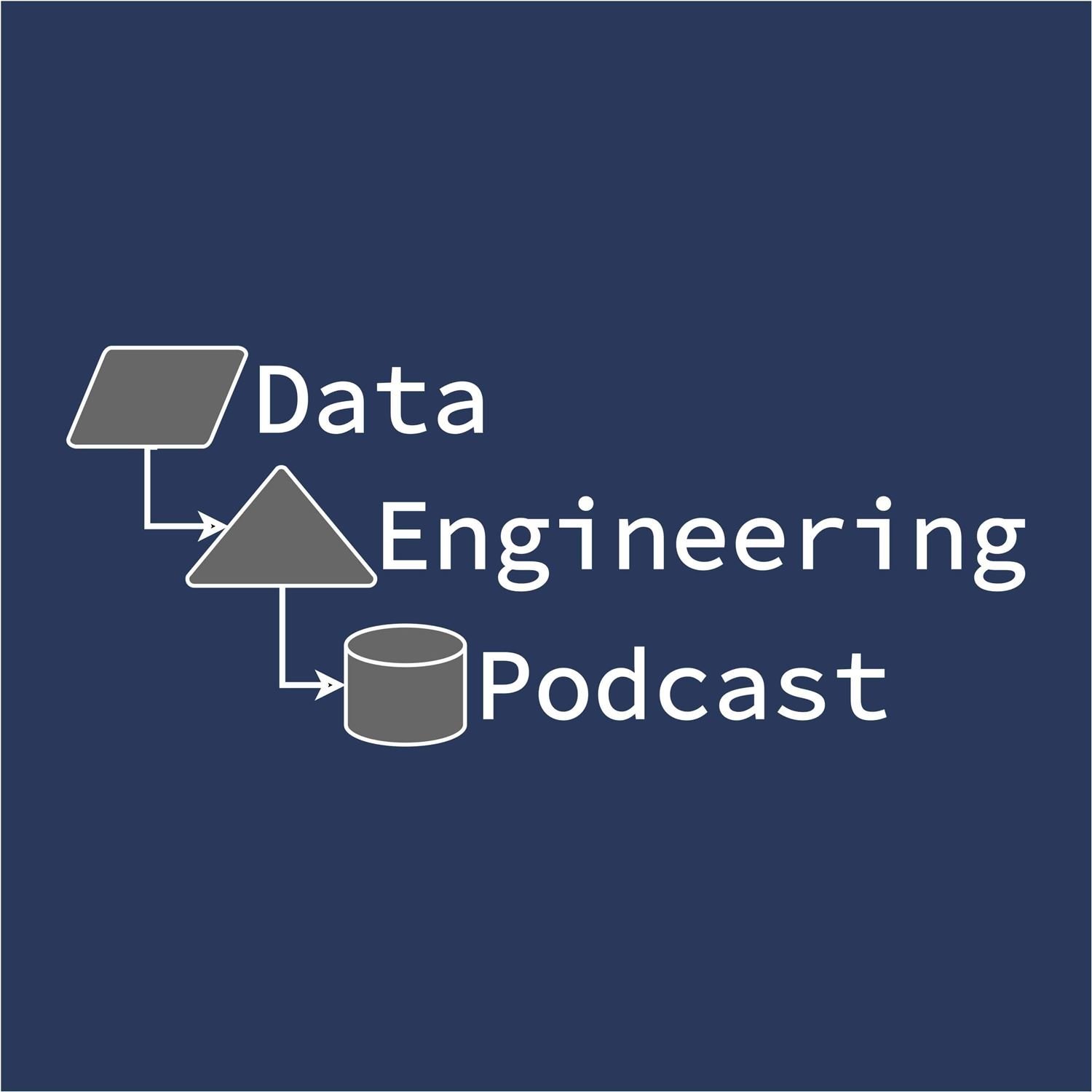
TimescaleDB: The Timeseries Database Built For SQL And Scale - Episode 65
Summary
The past year has been an active one for the timeseries market. New products have been launched, more businesses have moved to streaming analytics, and the team at Timescale has been keeping busy. In this episode the TimescaleDB CEO Ajay Kulkarni and CTO Michael Freedman stop by to talk about their 1.0 release, how the use cases for timeseries data have proliferated, and how they are continuing to simplify the task of processing your time oriented events.
Introduction
Hello and welcome to the Data Engineering Podcast, the show about modern data management
When you’re ready to build your next pipeline, or want to test out the projects you hear about on the show, you’ll need somewhere to deploy it, so check out Linode. With 200Gbit private networking, scalable shared block storage, and a 40Gbit public network, you’ve got everything you need to run a fast, reliable, and bullet-proof data platform. If you need global distribution, they’ve got that covered too with world-wide datacenters including new ones in Toronto and Mumbai. Go to dataengineeringpodcast.com/linode today to get a $20 credit and launch a new server in under a minute.
Go to dataengineeringpodcast.com to subscribe to the show, sign up for the mailing list, read the show notes, and get in touch.
To help other people find the show please leave a review on iTunes, or Google Play Music, tell your friends and co-workers, and share it on social media.
Join the community in the new Zulip chat workspace at dataengineeringpodcast.com/chat
Your host is Tobias Macey and today I’m welcoming Ajay Kulkarni and Mike Freedman back to talk about how TimescaleDB has grown and changed over the past year
Interview
Introduction
How did you get involved in the area of data management?
Can you refresh our memory about what TimescaleDB is?
How has the market for timeseries databases changed since we last spoke?
What has changed in the focus and features of the TimescaleDB project and company?
Toward the end of 2018 you launched the 1.0 release of Timescale. What were your criteria for establishing that milestone?
What were the most challenging aspects of reaching that goal?
In terms of timeseries workloads, what are some of the factors that differ across varying use cases?
How do those differences impact the ways in which Timescale is used by the end user, and built by your team?
What are some of the initial assumptions that you made while first launching Timescale that have held true, and which have been disproven?
How have the improvements and new features in the recent releases of PostgreSQL impacted the Timescale product?
Have you been able to leverage some of the native improvements to simplify your implementation?
Are there any use cases for Timescale that would have been previously impractical in vanilla Postgres that would now be reasonable without the help of Timescale?
What is in store for the future of the Timescale product and organization?
Contact Info
Ajay
@acoustik on Twitter
LinkedIn
Mike
LinkedIn
Website
@michaelfreedman on Twitter
Timescale
Website
Documentation
Careers
timescaledb on GitHub
@timescaledb on Twitter
Parting Question
From your perspective, what is the biggest gap in the tooling or technology for data management today?
Links
TimescaleDB
Original Appearance on the Data Engineering Podcast
1.0 Release Blog Post
PostgreSQL
Podcast Interview
RDS
DB-Engines
MongoDB
IOT (Internet Of Things)
AWS Timestream
Kafka
Pulsar
Podcast Episode
Spark
Podcast Episode
Flink
Podcast Episode
Hadoop
DevOps
PipelineDB
Podcast Interview
Grafana
Tableau
Prometheus
OLTP (Online Transaction Processing)
Oracle DB
Data Lake
The intro and outro music is from The Hug by The Freak Fandango Orchestra / CC BY-SASupport Data Engineering Podcast
41:2614/01/2019
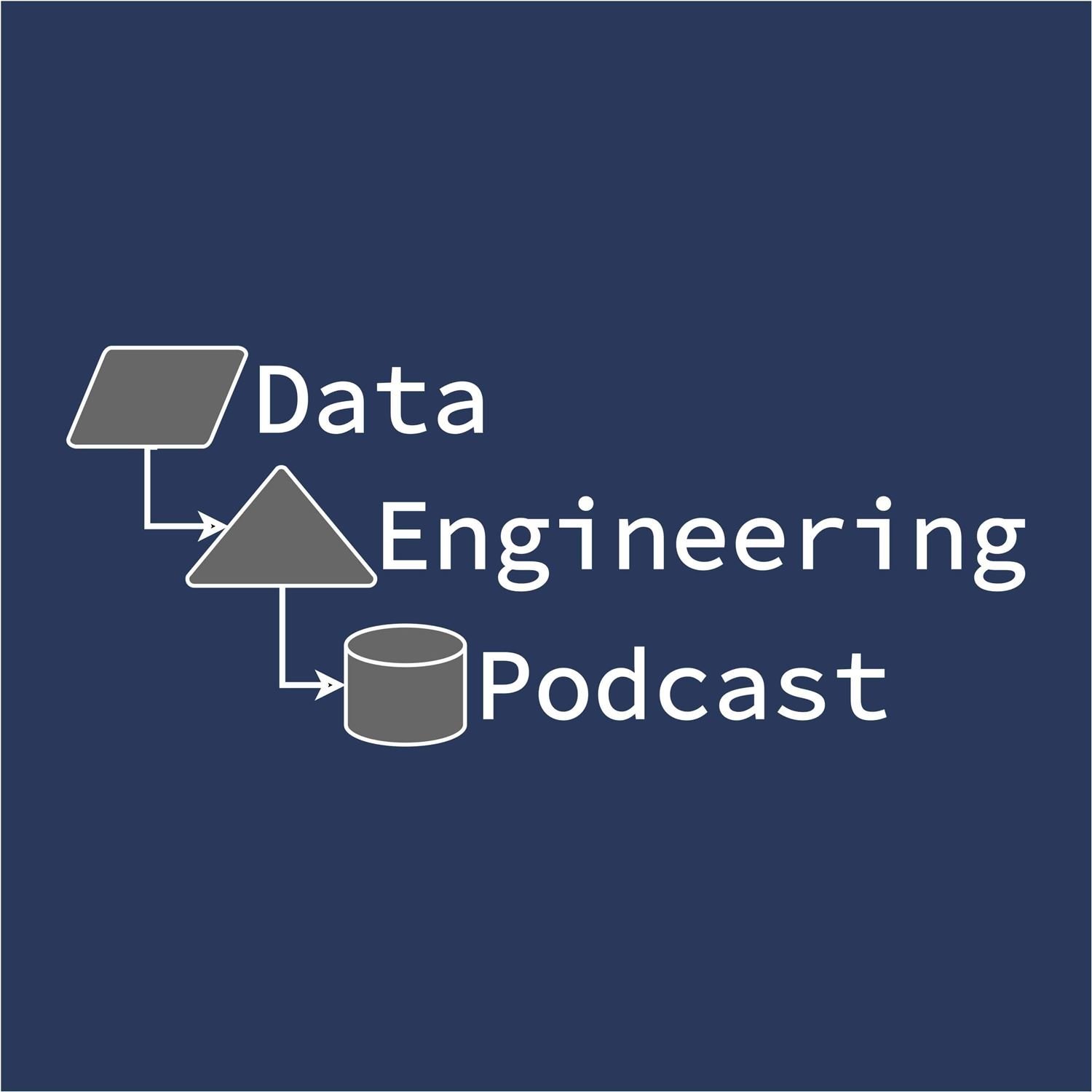
Performing Fast Data Analytics Using Apache Kudu - Episode 64
Summary
The Hadoop platform is purpose built for processing large, slow moving data in long-running batch jobs. As the ecosystem around it has grown, so has the need for fast data analytics on fast moving data. To fill this need the Kudu project was created with a column oriented table format that was tuned for high volumes of writes and rapid query execution across those tables. For a perfect pairing, they made it easy to connect to the Impala SQL engine. In this episode Brock Noland and Jordan Birdsell from PhData explain how Kudu is architected, how it compares to other storage systems in the Hadoop orbit, and how to start integrating it into you analytics pipeline.
Preamble
Hello and welcome to the Data Engineering Podcast, the show about modern data management
When you’re ready to build your next pipeline, or want to test out the projects you hear about on the show, you’ll need somewhere to deploy it, so check out Linode. With 200Gbit private networking, scalable shared block storage, and a 40Gbit public network, you’ve got everything you need to run a fast, reliable, and bullet-proof data platform. If you need global distribution, they’ve got that covered too with world-wide datacenters including new ones in Toronto and Mumbai. Go to dataengineeringpodcast.com/linode today to get a $20 credit and launch a new server in under a minute.
Go to dataengineeringpodcast.com to subscribe to the show, sign up for the mailing list, read the show notes, and get in touch.
To help other people find the show please leave a review on iTunes, or Google Play Music, tell your friends and co-workers, and share it on social media.
Join the community in the new Zulip chat workspace at dataengineeringpodcast.com/chat
Your host is Tobias Macey and today I’m interviewing Brock Noland and Jordan Birdsell about Apache Kudu and how it is able to provide fast analytics on fast data in the Hadoop ecosystem
Interview
Introduction
How did you get involved in the area of data management?
Can you start by explaining what Kudu is and the motivation for building it?
How does it fit into the Hadoop ecosystem?
How does it compare to the work being done on the Iceberg table format?
What are some of the common application and system design patterns that Kudu supports?
How is Kudu architected and how has it evolved over the life of the project?
There are many projects in and around the Hadoop ecosystem that rely on Zookeeper as a building block for consensus. What was the reasoning for using Raft in Kudu?
How does the storage layer in Kudu differ from what would be found in systems like Hive or HBase?
What are the implementation details in the Kudu storage interface that have had the greatest impact on its overall speed and performance?
A number of the projects built for large scale data processing were not initially built with a focus on operational simplicity. What are the features of Kudu that simplify deployment and management of production infrastructure?
What was the motivation for using C++ as the language target for Kudu?
If you were to start the project over today what would you do differently?
What are some situations where you would advise against using Kudu?
What have you found to be the most interesting/unexpected/challenging lessons learned in the process of building and maintaining Kudu?
What are you most excited about for the future of Kudu?
Contact Info
Brock
LinkedIn
@brocknoland on Twitter
Jordan
LinkedIn
@jordanbirdsell
jbirdsell on GitHub
PhData
Website
phdata on GitHub
@phdatainc on Twitter
Parting Question
From your perspective, what is the biggest gap in the tooling or technology for data management today?
Links
Kudu
PhData
Getting Started with Apache Kudu
Thomson Reuters
Hadoop
Oracle Exadata
Slowly Changing Dimensions
HDFS
S3
Azure Blob Storage
State Farm
Stanly Black & Decker
ETL (Extract, Transform, Load)
Parquet
Podcast Episode
ORC
HBase
Spark
Podcast Episode
Impala
Netflix Iceberg
Podcast Episode
Hive ACID
IOT (Internet Of Things)
Streamsets
NiFi
Podcast Episode
Kafka Connect
Moore’s Law
3D XPoint
Raft Consensus Algorithm
STONITH (Shoot The Other Node In The Head)
Yarn
Cython
Podcast.__init__ Episode
Pandas
Podcast.__init__ Episode
Cloudera Manager
Apache Sentry
Collibra
The intro and outro music is from The Hug by The Freak Fandango Orchestra / CC BY-SASupport Data Engineering Podcast
50:4707/01/2019
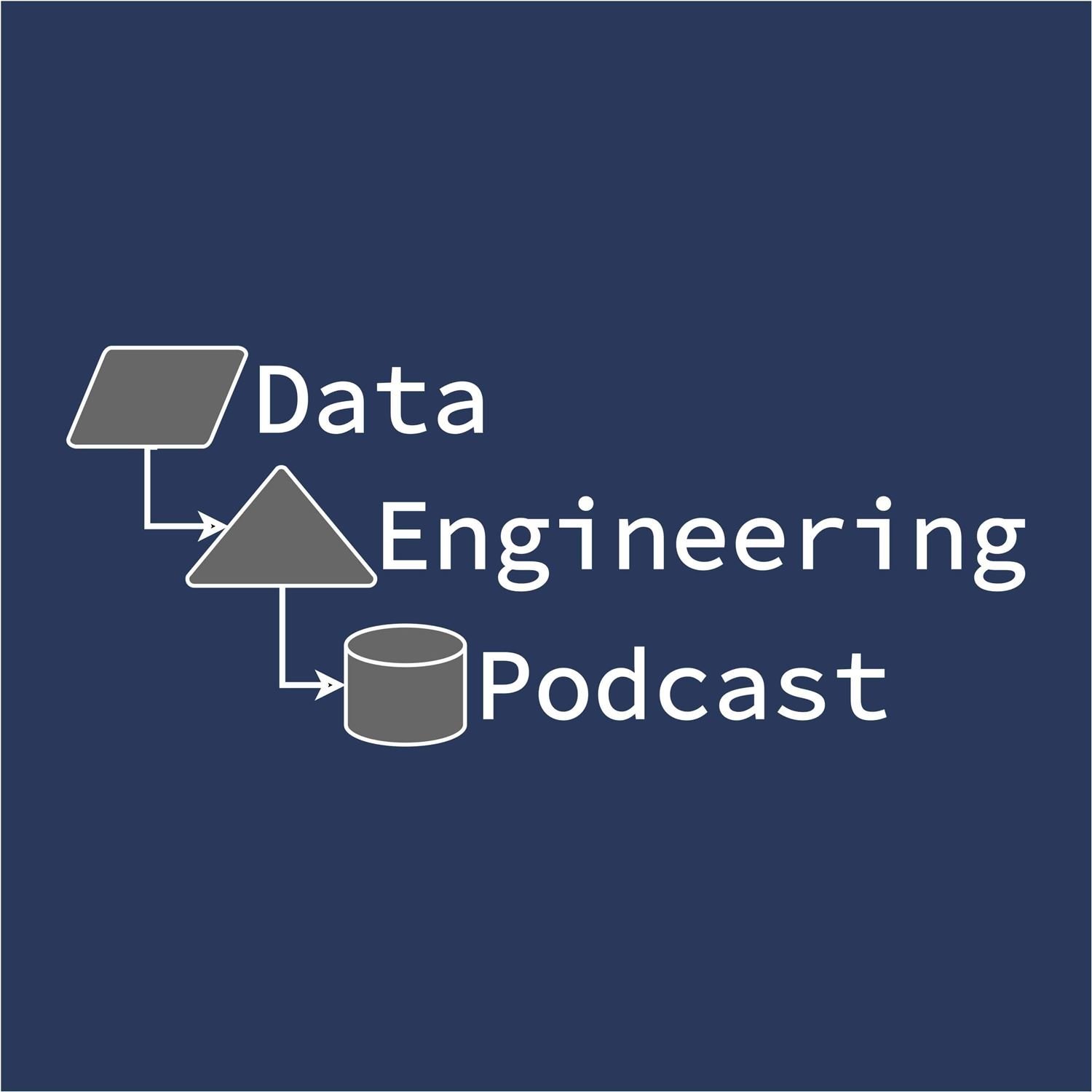
Simplifying Continuous Data Processing Using Stream Native Storage In Pravega with Tom Kaitchuck - Episode 63
Summary
As more companies and organizations are working to gain a real-time view of their business, they are increasingly turning to stream processing technologies to fullfill that need. However, the storage requirements for continuous, unbounded streams of data are markedly different than that of batch oriented workloads. To address this shortcoming the team at Dell EMC has created the open source Pravega project. In this episode Tom Kaitchuk explains how Pravega simplifies storage and processing of data streams, how it integrates with processing engines such as Flink, and the unique capabilities that it provides in the area of exactly once processing and transactions. And if you listen at approximately the half-way mark, you can hear as the hosts mind is blown by the possibilities of treating everything, including schema information, as a stream.
Preamble
Hello and welcome to the Data Engineering Podcast, the show about modern data management
When you’re ready to build your next pipeline, or want to test out the projects you hear about on the show, you’ll need somewhere to deploy it, so check out Linode. With 200Gbit private networking, scalable shared block storage, and a 40Gbit public network, you’ve got everything you need to run a fast, reliable, and bullet-proof data platform. If you need global distribution, they’ve got that covered too with world-wide datacenters including new ones in Toronto and Mumbai. Go to dataengineeringpodcast.com/linode today to get a $20 credit and launch a new server in under a minute.
Go to dataengineeringpodcast.com to subscribe to the show, sign up for the mailing list, read the show notes, and get in touch.
To help other people find the show please leave a review on iTunes, or Google Play Music, tell your friends and co-workers, and share it on social media.
Join the community in the new Zulip chat workspace at dataengineeringpodcast.com/chat
Your host is Tobias Macey and today I’m interviewing Tom Kaitchuck about Pravega, an open source data storage platform optimized for persistent streams
Interview
Introduction
How did you get involved in the area of data management?
Can you start by explaining what Pravega is and the story behind it?
What are the use cases for Pravega and how does it fit into the data ecosystem?
How does it compare with systems such as Kafka and Pulsar for ingesting and persisting unbounded data?
How do you represent a stream on-disk?
What are the benefits of using this format for persisted streams?
One of the compelling aspects of Pravega is the automatic sharding and resource allocation for variations in data patterns. Can you describe how that operates and the benefits that it provides?
I am also intrigued by the automatic tiering of the persisted storage. How does that work and what options exist for managing the lifecycle of the data in the cluster?
For someone who wants to build an application on top of Pravega, what interfaces does it provide and what architectural patterns does it lend itself toward?
What are some of the unique system design patterns that are made possible by Pravega?
How is Pravega architected internally?
What is involved in integrating engines such as Spark, Flink, or Storm with Pravega?
A common challenge for streaming systems is exactly once semantics. How does Pravega approach that problem?
Does it have any special capabilities for simplifying processing of out-of-order events?
For someone planning a deployment of Pravega, what is involved in building and scaling a cluster?
What are some of the operational edge cases that users should be aware of?
What are some of the most interesting, useful, or challenging experiences that you have had while building Pravega?
What are some cases where you would recommend against using Pravega?
What is in store for the future of Pravega?
Contact Info
tkaitchuk on GitHub
LinkedIn
Parting Question
From your perspective, what is the biggest gap in the tooling or technology for data management today?
Links
Pravega
Amazon SQS (Simple Queue Service)
Amazon Simple Workflow Service (SWF)
Azure
EMC
Zookeeper
Podcast Episode
Bookkeeper
Kafka
Pulsar
Podcast Episode
RocksDB
Flink
Podcast Episode
Spark
Podcast Episode
Heron
Lambda Architecture
Kappa Architecture
Erasure Code
Flink Forward Conference
CAP Theorem
The intro and outro music is from The Hug by The Freak Fandango Orchestra / CC BY-SASupport Data Engineering Podcast
44:4231/12/2018
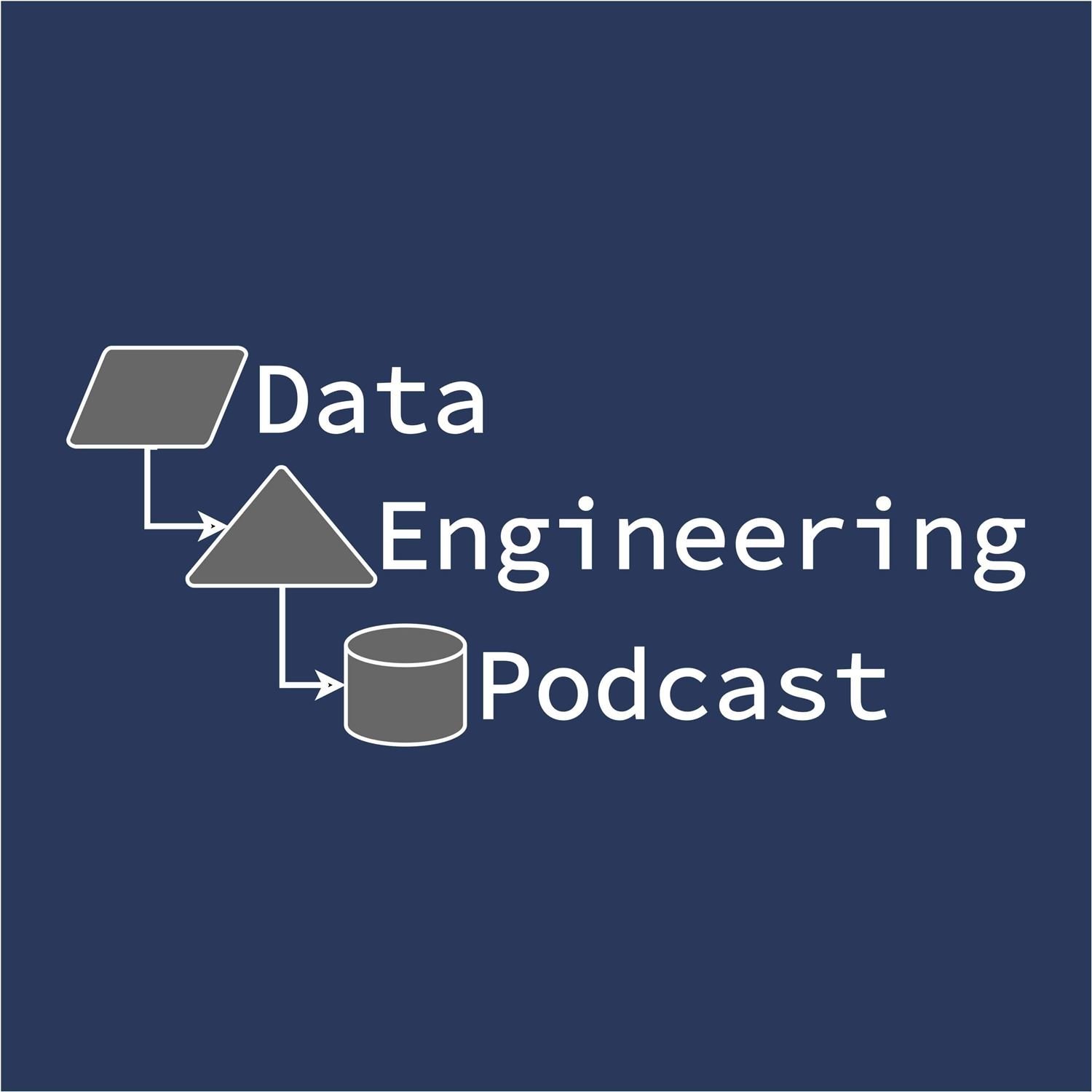
Continuously Query Your Time-Series Data Using PipelineDB with Derek Nelson and Usman Masood - Episode 62
Summary
Processing high velocity time-series data in real-time is a complex challenge. The team at PipelineDB has built a continuous query engine that simplifies the task of computing aggregates across incoming streams of events. In this episode Derek Nelson and Usman Masood explain how it is architected, strategies for designing your data flows, how to scale it up and out, and edge cases to be aware of.
Preamble
Hello and welcome to the Data Engineering Podcast, the show about modern data management
When you’re ready to build your next pipeline, or want to test out the projects you hear about on the show, you’ll need somewhere to deploy it, so check out Linode. With 200Gbit private networking, scalable shared block storage, and a 40Gbit public network, you’ve got everything you need to run a fast, reliable, and bullet-proof data platform. If you need global distribution, they’ve got that covered too with world-wide datacenters including new ones in Toronto and Mumbai. Go to dataengineeringpodcast.com/linode today to get a $20 credit and launch a new server in under a minute.
Go to dataengineeringpodcast.com to subscribe to the show, sign up for the mailing list, read the show notes, and get in touch.
Join the community in the new Zulip chat workspace at dataengineeringpodcast.com/chat
Your host is Tobias Macey and today I’m interviewing Usman Masood and Derek Nelson about PipelineDB, an open source continuous query engine for PostgreSQL
Interview
Introduction
How did you get involved in the area of data management?
Can you start by explaining what PipelineDB is and the motivation for creating it?
What are the major use cases that it enables?
What are some example applications that are uniquely well suited to the capabilities of PipelineDB?
What are the major concepts and components that users of PipelineDB should be familiar with?
Given the fact that it is a plugin for PostgreSQL, what level of compatibility exists between PipelineDB and other plugins such as Timescale and Citus?
What are some of the common patterns for populating data streams?
What are the options for scaling PipelineDB systems, both vertically and horizontally?
How much elasticity does the system support in terms of changing volumes of inbound data?
What are some of the limitations or edge cases that users should be aware of?
Given that inbound data is not persisted to disk, how do you guard against data loss?
Is it possible to archive the data in a stream, unaltered, to a separate destination table or other storage location?
Can a separate table be used as an input stream?
Since the data being processed by the continuous queries is potentially unbounded, how do you approach checkpointing or windowing the data in the continuous views?
What are some of the features that you have found to be the most useful which users might initially overlook?
What would be involved in generating an alert or notification on an aggregate output that was in some way anomalous?
What are some of the most challenging aspects of building continuous aggregates on unbounded data?
What have you found to be some of the most interesting, complex, or challenging aspects of building and maintaining PipelineDB?
What are some of the most interesting or unexpected ways that you have seen PipelineDB used?
When is PipelineDB the wrong choice?
What do you have planned for the future of PipelineDB now that you have hit the 1.0 milestone?
Contact Info
Derek
derekjn on GitHub
LinkedIn
Usman
@usmanm on Twitter
Website
Parting Question
From your perspective, what is the biggest gap in the tooling or technology for data management today?
Links
PipelineDB
Stride
PostgreSQL
Podcast Episode
AdRoll
Probabilistic Data Structures
TimescaleDB
[Podcast Episode](
Hive
Redshift
Kafka
Kinesis
ZeroMQ
Nanomsg
HyperLogLog
Bloom Filter
The intro and outro music is from The Hug by The Freak Fandango Orchestra / CC BY-SASupport Data Engineering Podcast
01:03:5224/12/2018
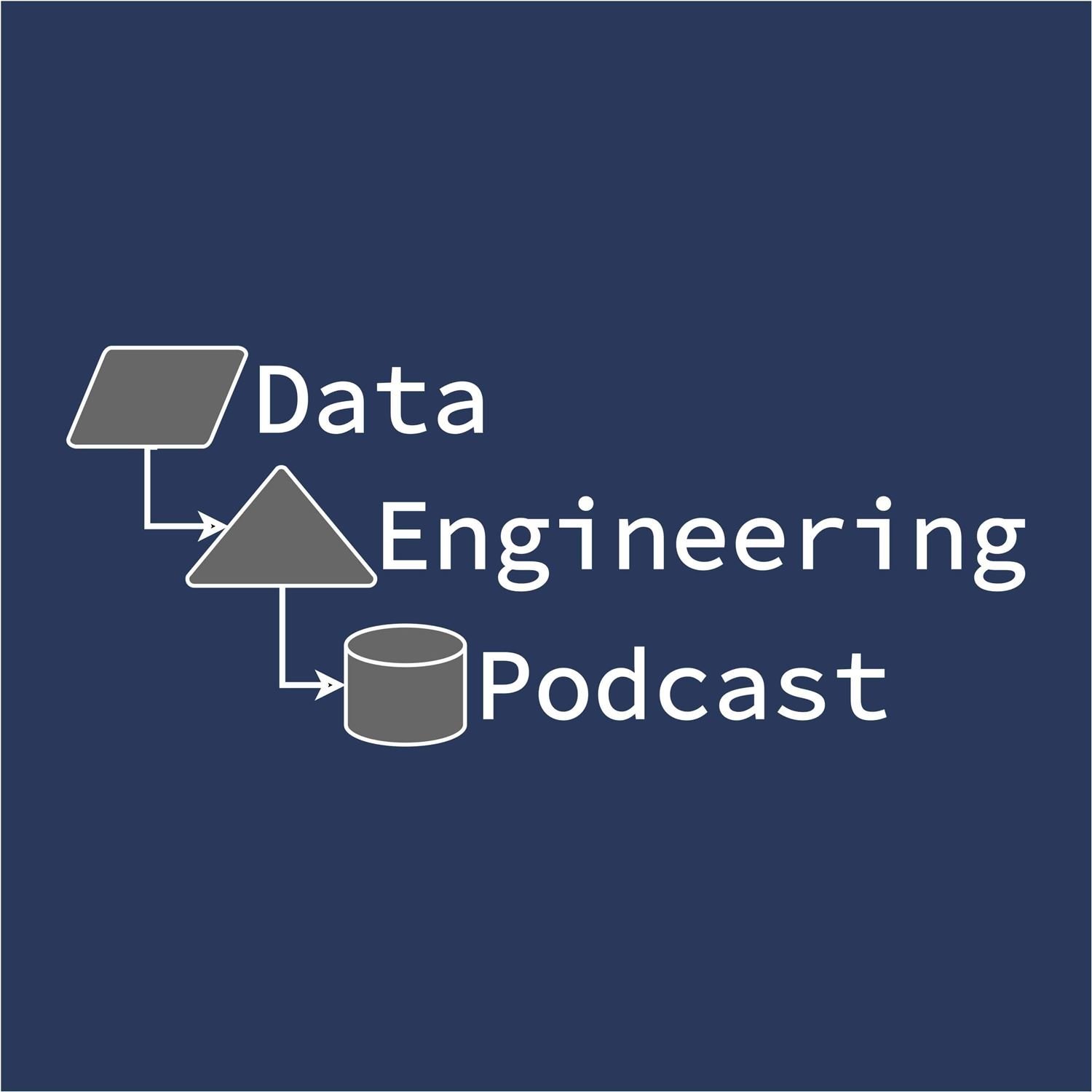
Advice On Scaling Your Data Pipeline Alongside Your Business with Christian Heinzmann - Episode 61
Summary
Every business needs a pipeline for their critical data, even if it is just pasting into a spreadsheet. As the organization grows and gains more customers, the requirements for that pipeline will change. In this episode Christian Heinzmann, Head of Data Warehousing at Grubhub, discusses the various requirements for data pipelines and how the overall system architecture evolves as more data is being processed. He also covers the changes in how the output of the pipelines are used, how that impacts the expectations for accuracy and availability, and some useful advice on build vs. buy for the components of a data platform.
Preamble
Hello and welcome to the Data Engineering Podcast, the show about modern data management
When you’re ready to build your next pipeline, or want to test out the projects you hear about on the show, you’ll need somewhere to deploy it, so check out Linode. With 200Gbit private networking, scalable shared block storage, and a 40Gbit public network, you’ve got everything you need to run a fast, reliable, and bullet-proof data platform. If you need global distribution, they’ve got that covered too with world-wide datacenters including new ones in Toronto and Mumbai. Go to dataengineeringpodcast.com/linode today to get a $20 credit and launch a new server in under a minute.
Go to dataengineeringpodcast.com to subscribe to the show, sign up for the mailing list, read the show notes, and get in touch.
Join the community in the new Zulip chat workspace at dataengineeringpodcast.com/chat
Your host is Tobias Macey and today I’m interviewing Christian Heinzmann about how data pipelines evolve as your business grows
Interview
Introduction
How did you get involved in the area of data management?
Can you start by sharing your definition of a data pipeline?
At what point in the life of a project or organization should you start thinking about building a pipeline?
In the early stages when the scale of the data and business are still small, what are some of the design characteristics that you should be targeting for your pipeline?
What metrics/use cases should you be optimizing for at this point?
What are some of the indicators that you look for to signal that you are reaching the next order of magnitude in terms of scale?
How do the design requirements for a data pipeline change as you reach this stage?
What are some of the challenges and complexities that begin to present themselves as you build and run your pipeline at medium scale?
What are some of the changes that are necessary as you move to a large scale data pipeline?
At each level of scale it is important to minimize the impact of the ETL process on the source systems. What are some strategies that you have employed to avoid degrading the performance of the application systems?
In recent years there has been a shift to using data lakes as a staging ground before performing transformations. What are your thoughts on that approach?
When performing transformations there is a potential for discarding information or losing fidelity. How have you worked to reduce the impact of this effect?
Transformations of the source data can be brittle when the format or volume changes. How do you design the pipeline to be resilient to these types of changes?
What are your selection criteria when determining what workflow or ETL engines to use in your pipeline?
How has your preference of build vs buy changed at different scales of operation and as new/different projects become available?
What are some of the dead ends or edge cases that you have had to deal with in your current role at Grubhub?
What are some of the common mistakes or overlooked aspects of building a data pipeline that you have seen?
What are your plans for improving your current pipeline at Grubhub?
What are some references that you recommend for anyone who is designing a new data platform?
Contact Info
@sirchristian on Twitter
Blog
sirchristian on GitHub
Parting Question
From your perspective, what is the biggest gap in the tooling or technology for data management today?
Links
Scaling ETL blog post
GrubHub
Data Warehouse
Redshift
Spark
Spark In Action Podcast Episode
Hive
Amazon EMR
Looker
Podcast Episode
Redash
Metabase
Podcast Episode
A Primer on Enterprise Data Curation
Pub/Sub (Publish-Subscribe Pattern)
Change Data Capture
Jenkins
Python
Azkaban
Luigi
Zendesk
Data Lineage
AirBnB Engineering Blog
The intro and outro music is from The Hug by The Freak Fandango Orchestra / CC BY-SASupport Data Engineering Podcast
39:2217/12/2018
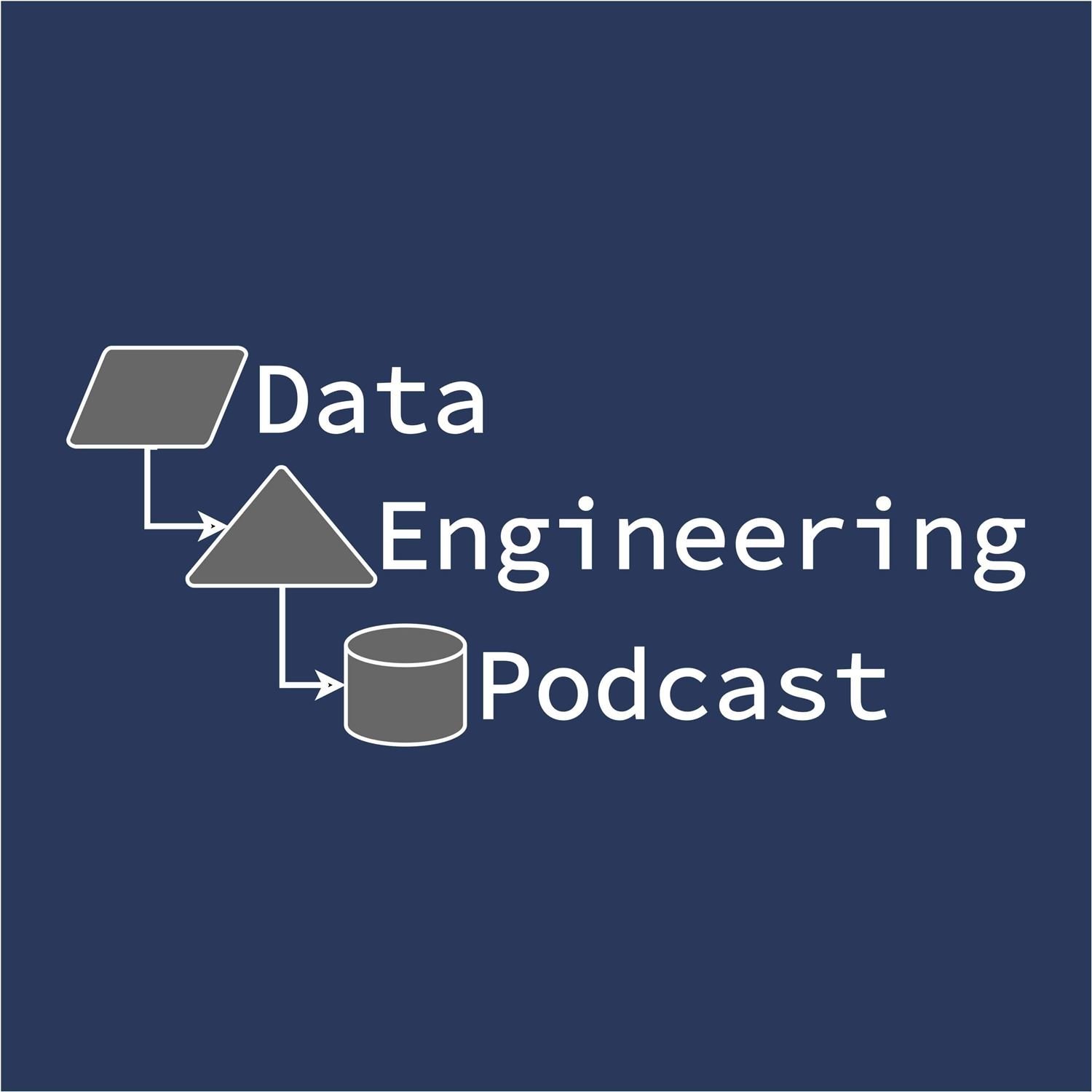
Putting Apache Spark Into Action with Jean Georges Perrin - Episode 60
Summary
Apache Spark is a popular and widely used tool for a variety of data oriented projects. With the large array of capabilities, and the complexity of the underlying system, it can be difficult to understand how to get started using it. Jean George Perrin has been so impressed by the versatility of Spark that he is writing a book for data engineers to hit the ground running. In this episode he helps to make sense of what Spark is, how it works, and the various ways that you can use it. He also discusses what you need to know to get it deployed and keep it running in a production environment and how it fits into the overall data ecosystem.
Preamble
Hello and welcome to the Data Engineering Podcast, the show about modern data management
When you’re ready to build your next pipeline, or want to test out the projects you hear about on the show, you’ll need somewhere to deploy it, so check out Linode. With 200Gbit private networking, scalable shared block storage, and a 40Gbit public network, you’ve got everything you need to run a fast, reliable, and bullet-proof data platform. If you need global distribution, they’ve got that covered too with world-wide datacenters including new ones in Toronto and Mumbai. Go to dataengineeringpodcast.com/linode today to get a $20 credit and launch a new server in under a minute.
Go to dataengineeringpodcast.com to subscribe to the show, sign up for the mailing list, read the show notes, and get in touch.
Join the community in the new Zulip chat workspace at dataengineeringpodcast.com/chat
Your host is Tobias Macey and today I’m interviewing Jean Georges Perrin, author of the upcoming Manning book Spark In Action 2nd Edition, about the ways that Spark is used and how it fits into the data landscape
Interview
Introduction
How did you get involved in the area of data management?
Can you start by explaining what Spark is?
What are some of the main use cases for Spark?
What are some of the problems that Spark is uniquely suited to address?
Who uses Spark?
What are the tools offered to Spark users?
How does it compare to some of the other streaming frameworks such as Flink, Kafka, or Storm?
For someone building on top of Spark what are the main software design paradigms?
How does the design of an application change as you go from a local development environment to a production cluster?
Once your application is written, what is involved in deploying it to a production environment?
What are some of the most useful strategies that you have seen for improving the efficiency and performance of a processing pipeline?
What are some of the edge cases and architectural considerations that engineers should be considering as they begin to scale their deployments?
What are some of the common ways that Spark is deployed, in terms of the cluster topology and the supporting technologies?
What are the limitations of the Spark programming model?
What are the cases where Spark is the wrong choice?
What was your motivation for writing a book about Spark?
Who is the target audience?
What have been some of the most interesting or useful lessons that you have learned in the process of writing a book about Spark?
What advice do you have for anyone who is considering or currently using Spark?
Contact Info
@jgperrin on Twitter
Blog
Parting Question
From your perspective, what is the biggest gap in the tooling or technology for data management today?
Book Discount
Use the code poddataeng18 to get 40% off of all of Manning’s products at manning.com
Links
Apache Spark
Spark In Action
Book code examples in GitHub
Informix
International Informix Users Group
MySQL
Microsoft SQL Server
ETL (Extract, Transform, Load)
Spark SQL and Spark In Action‘s chapter 11
Spark ML and Spark In Action‘s chapter 18
Spark Streaming (structured) and Spark In Action‘s chapter 10
Spark GraphX
Hadoop
Jupyter
Podcast Interview
Zeppelin
Databricks
IBM Watson Studio
Kafka
Flink
Podcast Episode
AWS Kinesis
Yarn
HDFS
Hive
Scala
PySpark
DAG
Spark Catalyst
Spark Tungsten
Spark UDF
AWS EMR
Mesos
DC/OS
Kubernetes
Dataframes
The intro and outro music is from The Hug by The Freak Fandango Orchestra / CC BY-SASupport Data Engineering Podcast
50:3110/12/2018
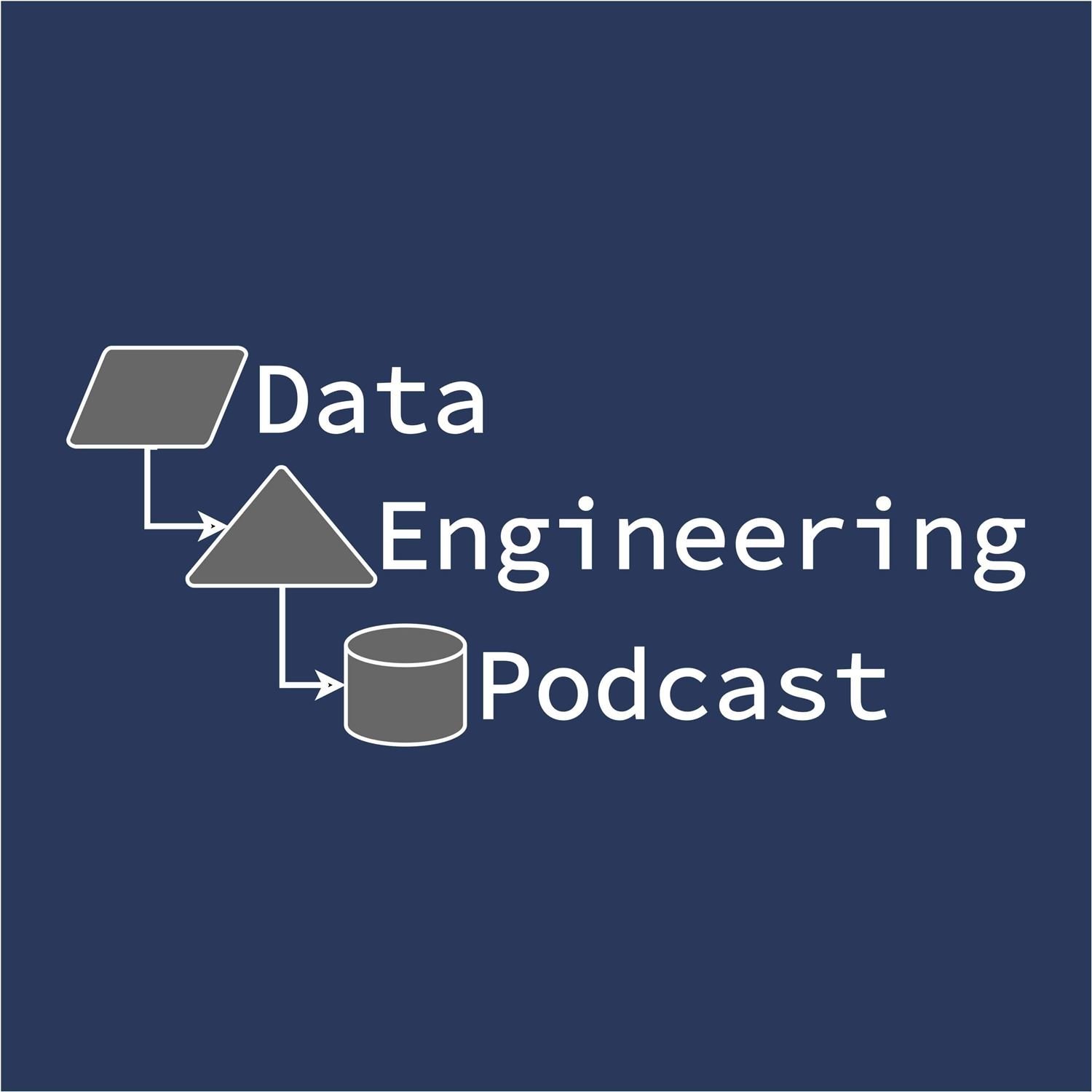
Apache Zookeeper As A Building Block For Distributed Systems with Patrick Hunt - Episode 59
Summary
Distributed systems are complex to build and operate, and there are certain primitives that are common to a majority of them. Rather then re-implement the same capabilities every time, many projects build on top of Apache Zookeeper. In this episode Patrick Hunt explains how the Apache Zookeeper project was started, how it functions, and how it is used as a building block for other distributed systems. He also explains the operational considerations for running your own cluster, how it compares to more recent entrants such as Consul and EtcD, and what is in store for the future.
Preamble
Hello and welcome to the Data Engineering Podcast, the show about modern data management
When you’re ready to build your next pipeline, or want to test out the projects you hear about on the show, you’ll need somewhere to deploy it, so check out Linode. With 200Gbit private networking, scalable shared block storage, and a 40Gbit public network, you’ve got everything you need to run a fast, reliable, and bullet-proof data platform. If you need global distribution, they’ve got that covered too with world-wide datacenters including new ones in Toronto and Mumbai. Go to dataengineeringpodcast.com/linode today to get a $20 credit and launch a new server in under a minute.
Go to dataengineeringpodcast.com to subscribe to the show, sign up for the mailing list, read the show notes, and get in touch.
Join the community in the new Zulip chat workspace at dataengineeringpodcast.com/chat
Your host is Tobias Macey and today I’m interviewing Patrick Hunt about Apache Zookeeper and how it is used as a building block for distributed systems
Interview
Introduction
How did you get involved in the area of data management?
Can you start by explaining what Zookeeper is and how the project got started?
What are the main motivations for using a centralized coordination service for distributed systems?
What are the distributed systems primitives that are built into Zookeeper?
What are some of the higher-order capabilities that Zookeeper provides to users who are building distributed systems on top of Zookeeper?
What are some of the types of system level features that application developers will need which aren’t provided by Zookeeper?
Can you discuss how Zookeeper is architected and how that design has evolved over time?
What have you found to be some of the most complicated or difficult aspects of building and maintaining Zookeeper?
What are the scaling factors for Zookeeper?
What are the edge cases that users should be aware of?
Where does it fall on the axes of the CAP theorem?
What are the main failure modes for Zookeeper?
How much of the recovery logic is left up to the end user of the Zookeeper cluster?
Since there are a number of projects that rely on Zookeeper, many of which are likely to be run in the same environment (e.g. Kafka and Flink), what would be involved in sharing a single Zookeeper cluster among those multiple services?
In recent years we have seen projects such as EtcD which is used by Kubernetes, and Consul. How does Zookeeper compare with those projects?
What are some of the cases where Zookeeper is the wrong choice?
How have the needs of distributed systems engineers changed since you first began working on Zookeeper?
If you were to start the project over today, what would you do differently?
Would you still use Java?
What are some of the most interesting or unexpected ways that you have seen Zookeeper used?
What do you have planned for the future of Zookeeper?
Contact Info
@phunt on Twitter
Parting Question
From your perspective, what is the biggest gap in the tooling or technology for data management today?
Links
Zookeeper
Cloudera
Google Chubby
Sourceforge
HBase
High Availability
Fallacies of distributed computing
Falsehoods programmers believe about networking
Consul
EtcD
Apache Curator
Raft Consensus Algorithm
Zookeeper Atomic Broadcast
SSD Write Cliff
Apache Kafka
Apache Flink
Podcast Episode
HDFS
Kubernetes
Netty
Protocol Buffers
Avro
Rust
The intro and outro music is from The Hug by The Freak Fandango Orchestra / CC BY-SA
Support Data Engineering Podcast
54:2503/12/2018
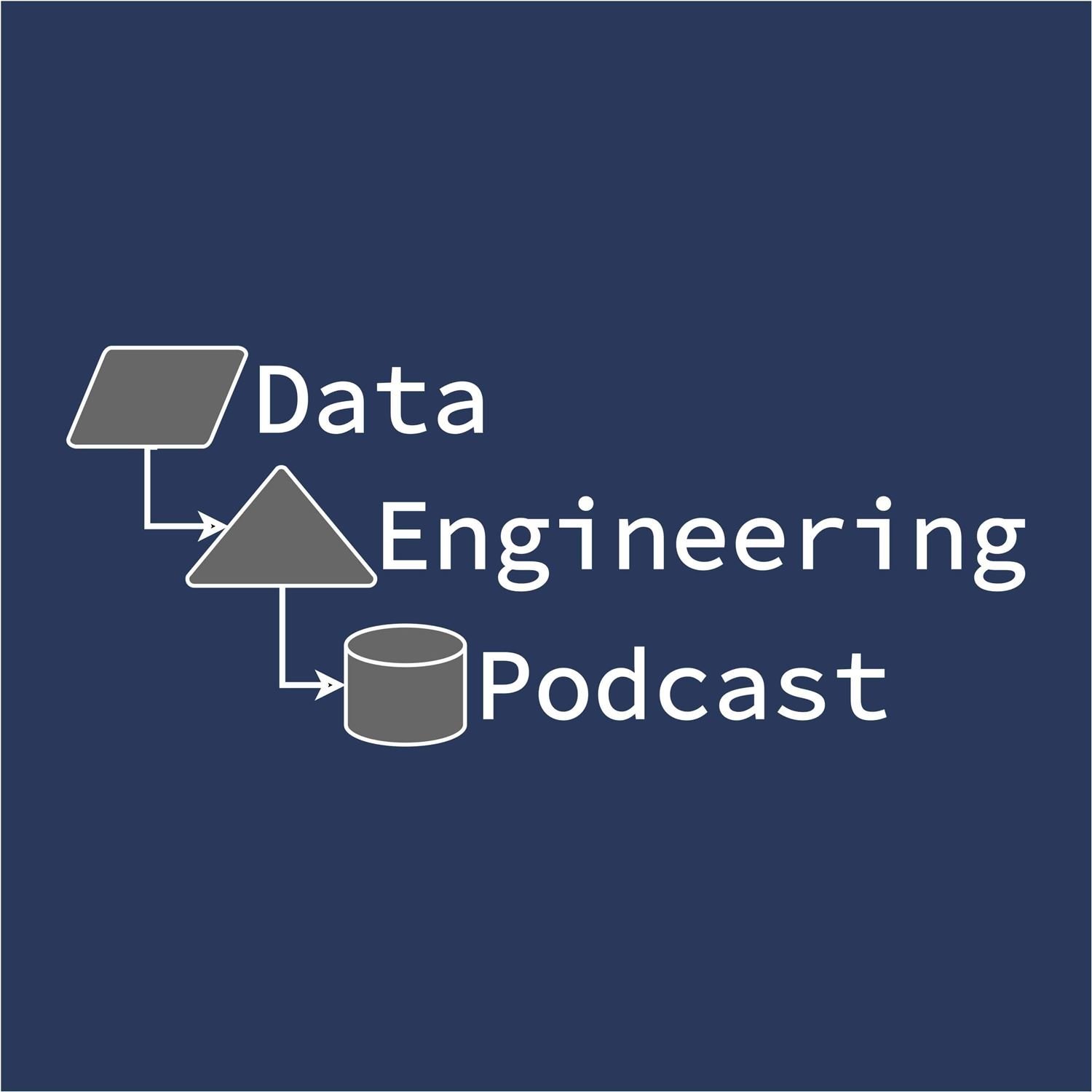
Set Up Your Own Data-as-a-Service Platform On Dremio with Tomer Shiran - Episode 58
Summary
When your data lives in multiple locations, belonging to at least as many applications, it is exceedingly difficult to ask complex questions of it. The default way to manage this situation is by crafting pipelines that will extract the data from source systems and load it into a data lake or data warehouse. In order to make this situation more manageable and allow everyone in the business to gain value from the data the folks at Dremio built a self service data platform. In this episode Tomer Shiran, CEO and co-founder of Dremio, explains how it fits into the modern data landscape, how it works under the hood, and how you can start using it today to make your life easier.
Preamble
Hello and welcome to the Data Engineering Podcast, the show about modern data management
When you’re ready to build your next pipeline, or want to test out the projects you hear about on the show, you’ll need somewhere to deploy it, so check out Linode. With 200Gbit private networking, scalable shared block storage, and a 40Gbit public network, you’ve got everything you need to run a fast, reliable, and bullet-proof data platform. If you need global distribution, they’ve got that covered too with world-wide datacenters including new ones in Toronto and Mumbai. Go to dataengineeringpodcast.com/linode today to get a $20 credit and launch a new server in under a minute.
Go to dataengineeringpodcast.com to subscribe to the show, sign up for the mailing list, read the show notes, and get in touch.
Join the community in the new Zulip chat workspace at dataengineeringpodcast.com/chat
Your host is Tobias Macey and today I’m interviewing Tomer Shiran about Dremio, the open source data as a service platform
Interview
Introduction
How did you get involved in the area of data management?
Can you start by explaining what Dremio is and how the project and business got started?
What was the motivation for keeping your primary product open source?
What is the governance model for the project?
How does Dremio fit in the current landscape of data tools?
What are some use cases that Dremio is uniquely equipped to support?
Do you think that Dremio obviates the need for a data warehouse or large scale data lake?
How is Dremio architected internally?
How has that architecture evolved from when it was first built?
There are a large array of components (e.g. governance, lineage, catalog) built into Dremio that are often found in dedicated products. What are some of the strategies that you have as a business and development team to manage and integrate the complexity of the product?
What are the benefits of integrating all of those capabilities into a single system?
What are the drawbacks?
One of the useful features of Dremio is the granular access controls. Can you discuss how those are implemented and controlled?
For someone who is interested in deploying Dremio to their environment what is involved in getting it installed?
What are the scaling factors?
What are some of the most exciting features that have been added in recent releases?
When is Dremio the wrong choice?
What have been some of the most challenging aspects of building, maintaining, and growing the technical and business platform of Dremio?
What do you have planned for the future of Dremio?
Contact Info
Tomer
@tshiran on Twitter
LinkedIn
Dremio
Website
@dremio on Twitter
dremio on GitHub
Parting Question
From your perspective, what is the biggest gap in the tooling or technology for data management today?
Links
Dremio
MapR
Presto
Business Intelligence
Arrow
Tableau
Power BI
Jupyter
OLAP Cube
Apache Foundation
Hadoop
Nikon DSLR
Spark
ETL (Extract, Transform, Load)
Parquet
Avro
K8s
Helm
Yarn
Gandiva Initiative for Apache Arrow
LLVM
TLS
The intro and outro music is from The Hug by The Freak Fandango Orchestra / CC BY-SASupport Data Engineering Podcast
39:1826/11/2018
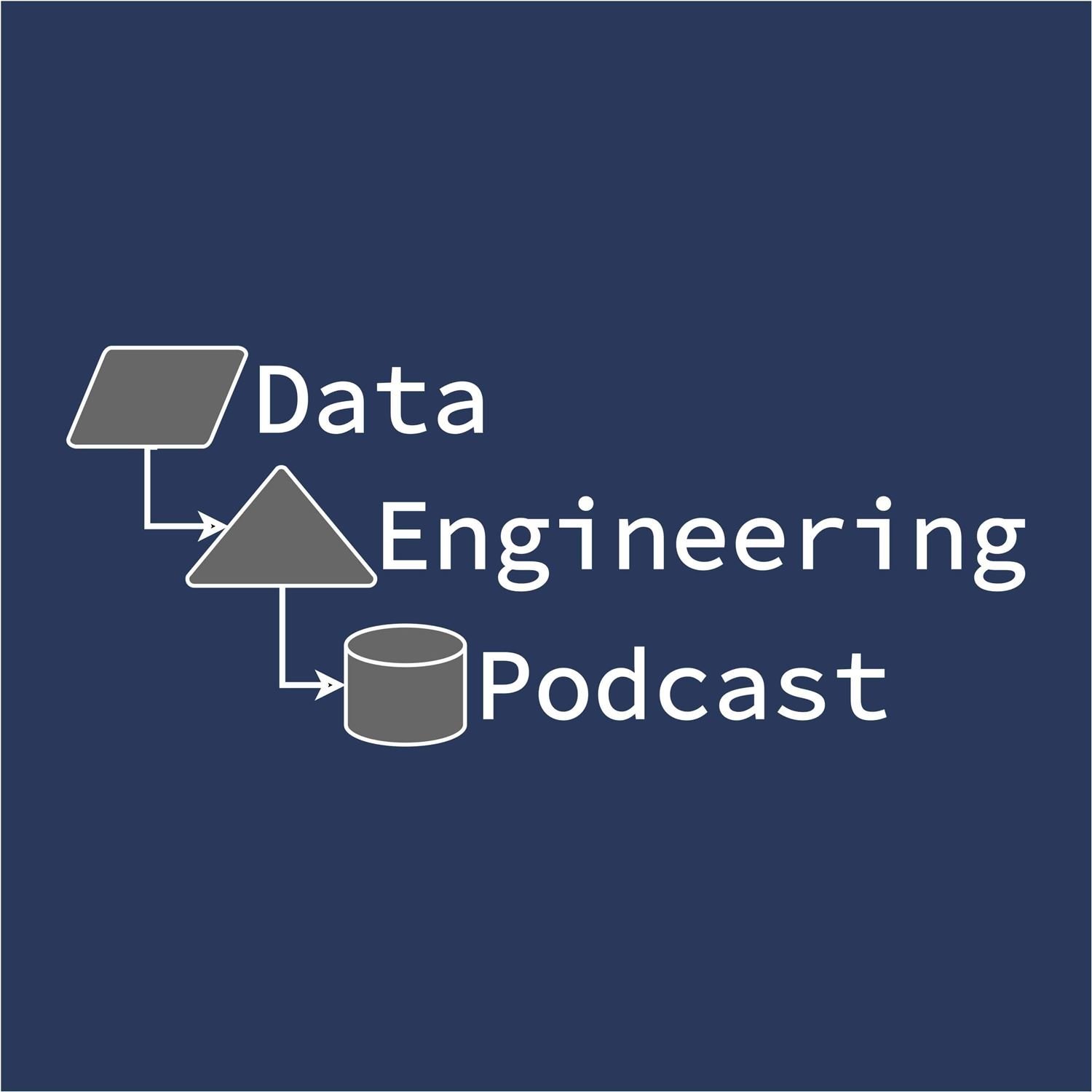
Stateful, Distributed Stream Processing on Flink with Fabian Hueske - Episode 57
Summary
Modern applications and data platforms aspire to process events and data in real time at scale and with low latency. Apache Flink is a true stream processing engine with an impressive set of capabilities for stateful computation at scale. In this episode Fabian Hueske, one of the original authors, explains how Flink is architected, how it is being used to power some of the world’s largest businesses, where it sits in the lanscape of stream processing tools, and how you can start using it today.
Preamble
Hello and welcome to the Data Engineering Podcast, the show about modern data management
When you’re ready to build your next pipeline, or want to test out the projects you hear about on the show, you’ll need somewhere to deploy it, so check out Linode. With 200Gbit private networking, scalable shared block storage, and a 40Gbit public network, you’ve got everything you need to run a fast, reliable, and bullet-proof data platform. If you need global distribution, they’ve got that covered too with world-wide datacenters including new ones in Toronto and Mumbai. Go to dataengineeringpodcast.com/linode today to get a $20 credit and launch a new server in under a minute.
Go to dataengineeringpodcast.com to subscribe to the show, sign up for the mailing list, read the show notes, and get in touch.
Join the community in the new Zulip chat workspace at dataengineeringpodcast.com/chat
Your host is Tobias Macey and today I’m interviewing Fabian Hueske, co-author of the upcoming O’Reilly book Stream Processing With Apache Flink, about his work on Apache Flink, the stateful streaming engine
Interview
Introduction
How did you get involved in the area of data management?
Can you start by describing what Flink is and how the project got started?
What are some of the primary ways that Flink is used?
How does Flink compare to other streaming engines such as Spark, Kafka, Pulsar, and Storm?
What are some use cases that Flink is uniquely qualified to handle?
Where does Flink fit into the current data landscape?
How is Flink architected?
How has that architecture evolved?
Are there any aspects of the current design that you would do differently if you started over today?
How does scaling work in a Flink deployment?
What are the scaling limits?
What are some of the failure modes that users should be aware of?
How is the statefulness of a cluster managed?
What are the mechanisms for managing conflicts?
What are the limiting factors for the volume of state that can be practically handled in a cluster and for a given purpose?
Can state be shared across processes or tasks within a Flink cluster?
What are the comparative challenges of working with bounded vs unbounded streams of data?
How do you handle out of order events in Flink, especially as the delay for a given event increases?
For someone who is using Flink in their environment, what are the primary means of interacting with and developing on top of it?
What are some of the most challenging or complicated aspects of building and maintaining Flink?
What are some of the most interesting or unexpected ways that you have seen Flink used?
What are some of the improvements or new features that are planned for the future of Flink?
What are some features or use cases that you are explicitly not planning to support?
For people who participate in the training sessions that you offer through Data Artisans, what are some of the concepts that they are challenged by?
What do they find most interesting or exciting?
Contact Info
LinkedIn
@fhueske on Twitter
fhueske on GitHub
Parting Question
From your perspective, what is the biggest gap in the tooling or technology for data management today?
Links
Flink
Data Artisans
IBM
DB2
Technische Universität Berlin
Hadoop
Relational Database
Google Cloud Dataflow
Spark
Cascading
Java
RocksDB
Flink Checkpoints
Flink Savepoints
Kafka
Pulsar
Storm
Scala
LINQ (Language INtegrated Query)
SQL
Backpressure
Watermarks
HDFS
S3
Avro
JSON
Hive Metastore
Dell EMC
Pravega
The intro and outro music is from The Hug by The Freak Fandango Orchestra / CC BY-SASupport Data Engineering Podcast
48:0219/11/2018
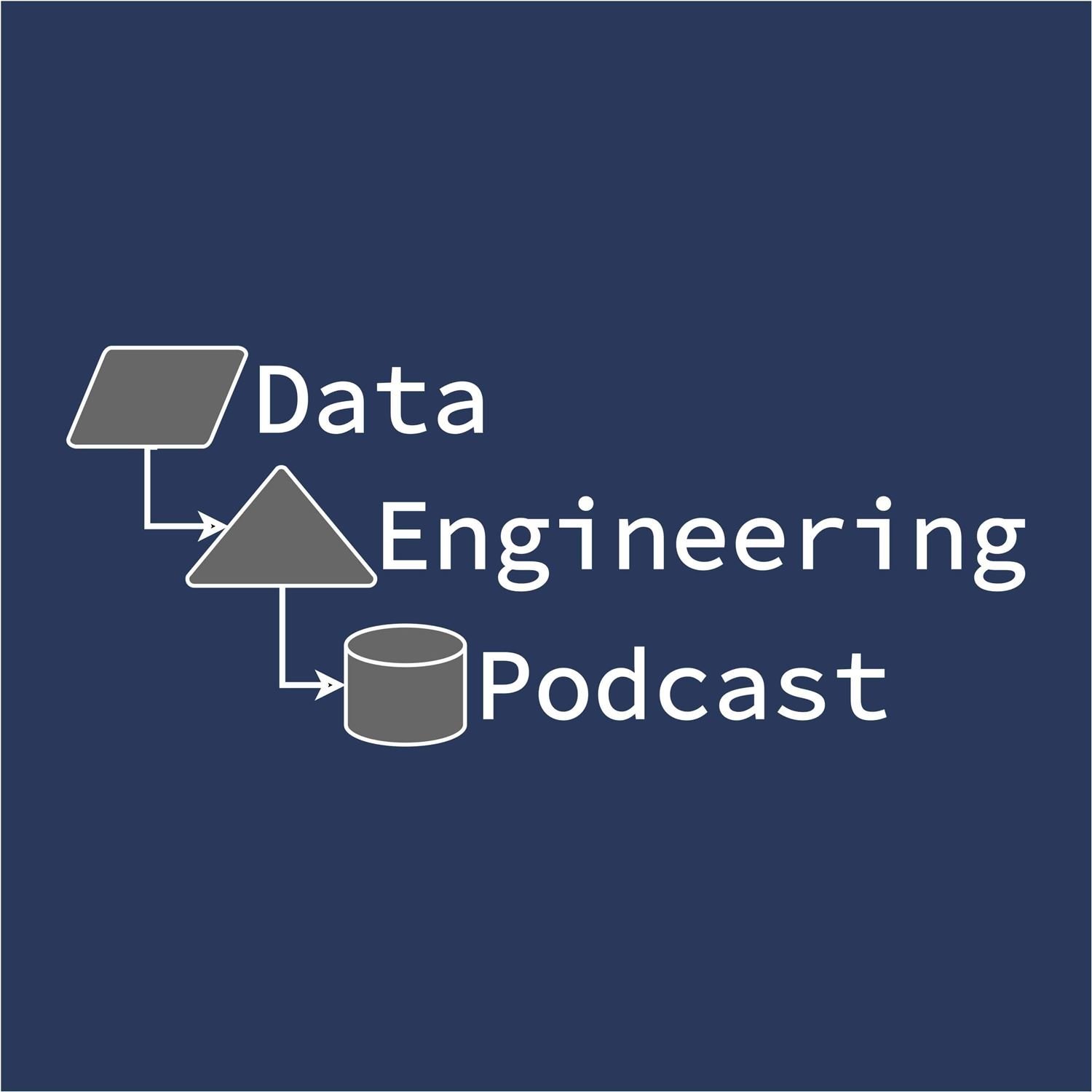
How Upsolver Is Building A Data Lake Platform In The Cloud with Yoni Iny - Episode 56
Summary
A data lake can be a highly valuable resource, as long as it is well built and well managed. Unfortunately, that can be a complex and time-consuming effort, requiring specialized knowledge and diverting resources from your primary business. In this episode Yoni Iny, CTO of Upsolver, discusses the various components that are necessary for a successful data lake project, how the Upsolver platform is architected, and how modern data lakes can benefit your organization.
Preamble
Hello and welcome to the Data Engineering Podcast, the show about modern data management
When you’re ready to build your next pipeline you’ll need somewhere to deploy it, so check out Linode. With private networking, shared block storage, node balancers, and a 40Gbit network, all controlled by a brand new API you’ve got everything you need to run a bullet-proof data platform. Go to dataengineeringpodcast.com/linode to get a $20 credit and launch a new server in under a minute.
Go to dataengineeringpodcast.com to subscribe to the show, sign up for the mailing list, read the show notes, and get in touch.
Join the community in the new Zulip chat workspace at dataengineeringpodcast.com/chat
Your host is Tobias Macey and today I’m interviewing Yoni Iny about Upsolver, a data lake platform that lets developers integrate and analyze streaming data with ease
Interview
Introduction
How did you get involved in the area of data management?
Can you start by describing what Upsolver is and how it got started?
What are your goals for the platform?
There are a lot of opinions on both sides of the data lake argument. When is it the right choice for a data platform?
What are the shortcomings of a data lake architecture?
How is Upsolver architected?
How has that architecture changed over time?
How do you manage schema validation for incoming data?
What would you do differently if you were to start over today?
What are the biggest challenges at each of the major stages of the data lake?
What is the workflow for a user of Upsolver and how does it compare to a self-managed data lake?
When is Upsolver the wrong choice for an organization considering implementation of a data platform?
Is there a particular scale or level of data maturity for an organization at which they would be better served by moving management of their data lake in house?
What features or improvements do you have planned for the future of Upsolver?
Contact Info
Yoni
yoniiny on GitHub
LinkedIn
Upsolver
Website
@upsolver on Twitter
LinkedIn
Facebook
Parting Question
From your perspective, what is the biggest gap in the tooling or technology for data management today?
Links
Upsolver
Data Lake
Israeli Army
Data Warehouse
Data Engineering Podcast Episode About Data Curation
Three Vs
Kafka
Spark
Presto
Drill
Spot Instances
Object Storage
Cassandra
Redis
Latency
Avro
Parquet
ORC
Data Engineering Podcast Episode About Data Serialization Formats
SSTables
Run Length Encoding
CSV (Comma Separated Values)
Protocol Buffers
Kinesis
ETL
DevOps
Prometheus
Cloudwatch
DataDog
InfluxDB
SQL
Pandas
Confluent
KSQL
The intro and outro music is from The Hug by The Freak Fandango Orchestra / CC BY-SASupport Data Engineering Podcast
51:5111/11/2018
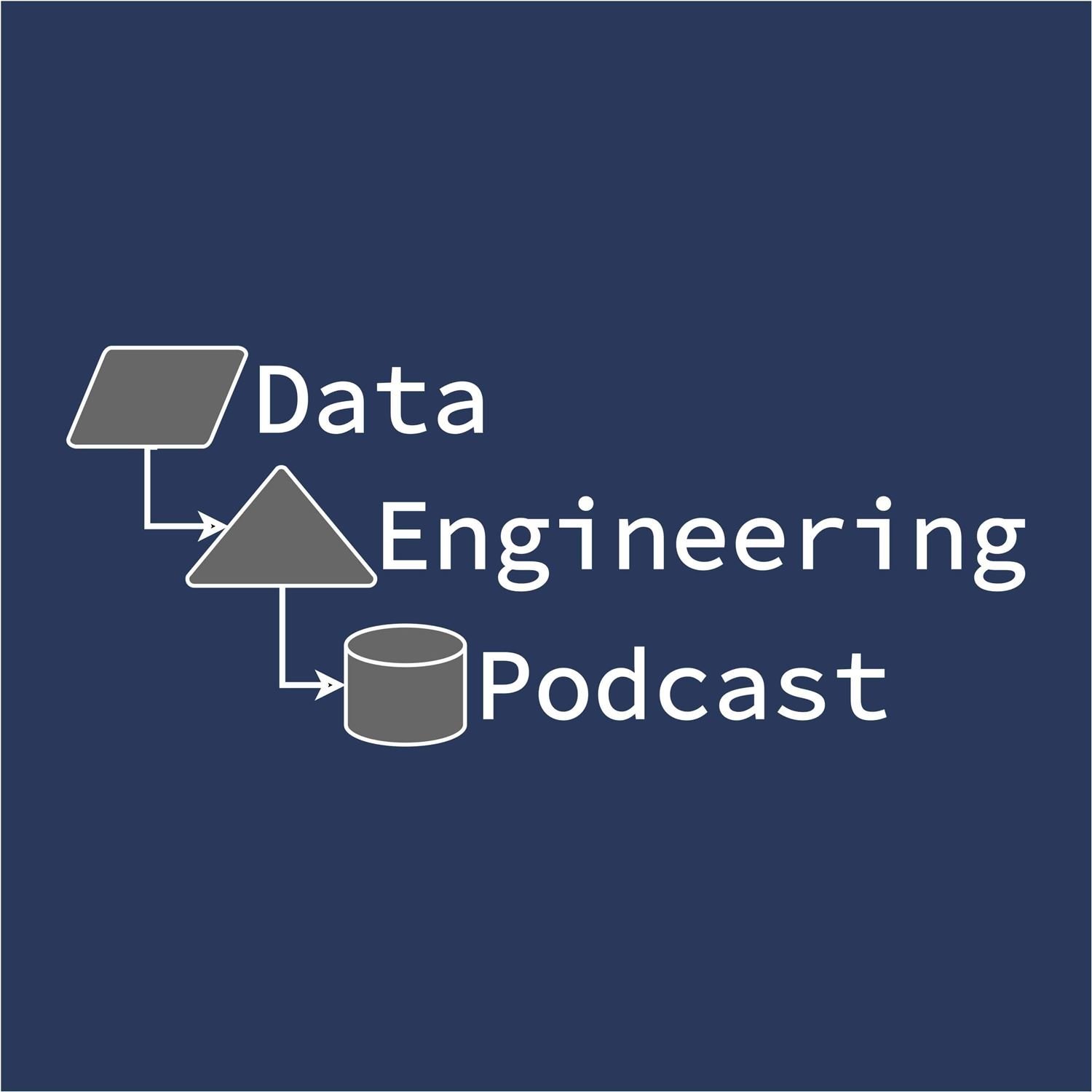
Self Service Business Intelligence And Data Sharing Using Looker with Daniel Mintz - Episode 55
Summary
Business intelligence is a necessity for any organization that wants to be able to make informed decisions based on the data that they collect. Unfortunately, it is common for different portions of the business to build their reports with different assumptions, leading to conflicting views and poor choices. Looker is a modern tool for building and sharing reports that makes it easy to get everyone on the same page. In this episode Daniel Mintz explains how the product is architected, the features that make it easy for any business user to access and explore their reports, and how you can use it for your organization today.
Preamble
Hello and welcome to the Data Engineering Podcast, the show about modern data management
When you’re ready to build your next pipeline you’ll need somewhere to deploy it, so check out Linode. With private networking, shared block storage, node balancers, and a 40Gbit network, all controlled by a brand new API you’ve got everything you need to run a bullet-proof data platform. Go to dataengineeringpodcast.com/linode to get a $20 credit and launch a new server in under a minute.
Go to dataengineeringpodcast.com to subscribe to the show, sign up for the mailing list, read the show notes, and get in touch.
Join the community in the new Zulip chat workspace at dataengineeringpodcast.com/chat
Your host is Tobias Macey and today I’m interviewing Daniel Mintz about Looker, a a modern data platform that can serve the data needs of an entire company
Interview
Introduction
How did you get involved in the area of data management?
Can you start by describing what Looker is and the problem that it is aiming to solve?
How do you define business intelligence?
How is Looker unique from other approaches to business intelligence in the enterprise?
How does it compare to open source platforms for BI?
Can you describe the technical infrastructure that supports Looker?
Given that you are connecting to the customer’s data store, how do you ensure sufficient security?
For someone who is using Looker, what does their workflow look like?
How does that change for different user roles (e.g. data engineer vs sales management)
What are the scaling factors for Looker, both in terms of volume of data for reporting from, and for user concurrency?
What are the most challenging aspects of building a business intelligence tool and company in the modern data ecosystem?
What are the portions of the Looker architecture that you would do differently if you were to start over today?
What are some of the most interesting or unusual uses of Looker that you have seen?
What is in store for the future of Looker?
Contact Info
LinkedIn
Parting Question
From your perspective, what is the biggest gap in the tooling or technology for data management today?
Links
Looker
Upworthy
MoveOn.org
LookML
SQL
Business Intelligence
Data Warehouse
Linux
Hadoop
BigQuery
Snowflake
Redshift
DB2
PostGres
ETL (Extract, Transform, Load)
ELT (Extract, Load, Transform)
Airflow
Luigi
NiFi
Data Curation Episode
Presto
Hive
Athena
DRY (Don’t Repeat Yourself)
Looker Action Hub
Salesforce
Marketo
Twilio
Netscape Navigator
Dynamic Pricing
Survival Analysis
DevOps
BigQuery ML
Snowflake Data Sharehouse
The intro and outro music is from The Hug by The Freak Fandango Orchestra / CC BY-SASupport Data Engineering Podcast
58:0405/11/2018
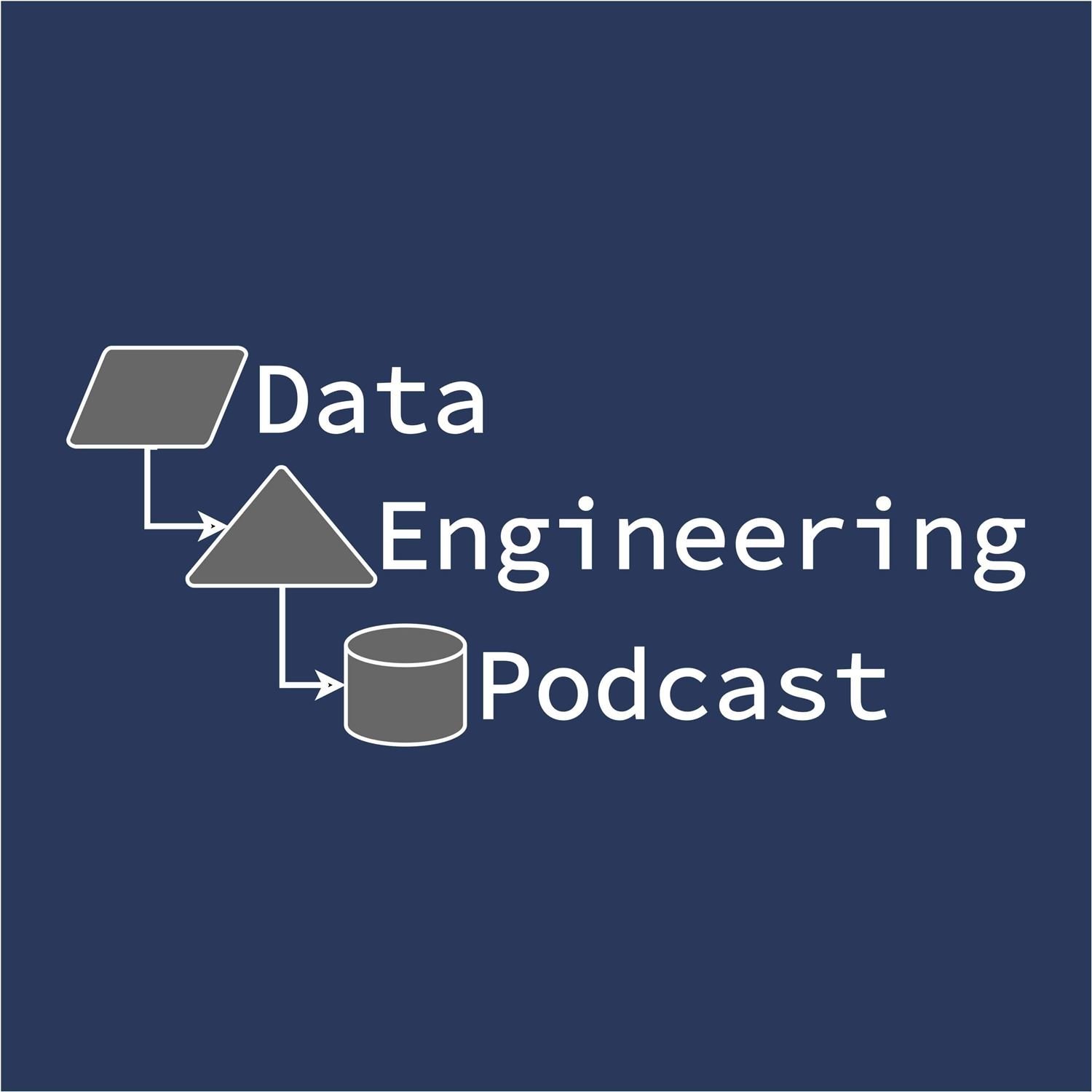
Using Notebooks As The Unifying Layer For Data Roles At Netflix with Matthew Seal - Episode 54
Summary
Jupyter notebooks have gained popularity among data scientists as an easy way to do exploratory analysis and build interactive reports. However, this can cause difficulties when trying to move the work of the data scientist into a more standard production environment, due to the translation efforts that are necessary. At Netflix they had the crazy idea that perhaps that last step isn’t necessary, and the production workflows can just run the notebooks directly. Matthew Seal is one of the primary engineers who has been tasked with building the tools and practices that allow the various data oriented roles to unify their work around notebooks. In this episode he explains the rationale for the effort, the challenges that it has posed, the development that has been done to make it work, and the benefits that it provides to the Netflix data platform teams.
Preamble
Hello and welcome to the Data Engineering Podcast, the show about modern data management
When you’re ready to build your next pipeline you’ll need somewhere to deploy it, so check out Linode. With private networking, shared block storage, node balancers, and a 40Gbit network, all controlled by a brand new API you’ve got everything you need to run a bullet-proof data platform. Go to dataengineeringpodcast.com/linode to get a $20 credit and launch a new server in under a minute.
Go to dataengineeringpodcast.com to subscribe to the show, sign up for the mailing list, read the show notes, and get in touch.
Join the community in the new Zulip chat workspace at dataengineeringpodcast.com/chat
Your host is Tobias Macey and today I’m interviewing Matthew Seal about the ways that Netflix is using Jupyter notebooks to bridge the gap between data roles
Interview
Introduction
How did you get involved in the area of data management?
Can you start by outlining the motivation for choosing Jupyter notebooks as the core interface for your data teams?
Where are you using notebooks and where are you not?
What is the technical infrastructure that you have built to suppport that design choice?
Which team was driving the effort?
Was it difficult to get buy in across teams?
How much shared code have you been able to consolidate or reuse across teams/roles?
Have you investigated the use of any of the other notebook platforms for similar workflows?
What are some of the notebook anti-patterns that you have encountered and what conventions or tooling have you established to discourage them?
What are some of the limitations of the notebook environment for the work that you are doing?
What have been some of the most challenging aspects of building production workflows on top of Jupyter notebooks?
What are some of the projects that are ongoing or planned for the future that you are most excited by?
Contact Info
Matthew Seal
Email
LinkedIn
@codeseal on Twitter
MSeal on GitHub
Parting Question
From your perspective, what is the biggest gap in the tooling or technology for data management today?
Links
Netflix Notebook Blog Posts
Nteract Tooling
OpenGov
Project Jupyter
Zeppelin Notebooks
Papermill
Titus
Commuter
Scala
Python
R
Emacs
NBDime
The intro and outro music is from The Hug by The Freak Fandango Orchestra / CC BY-SASupport Data Engineering Podcast
40:5529/10/2018
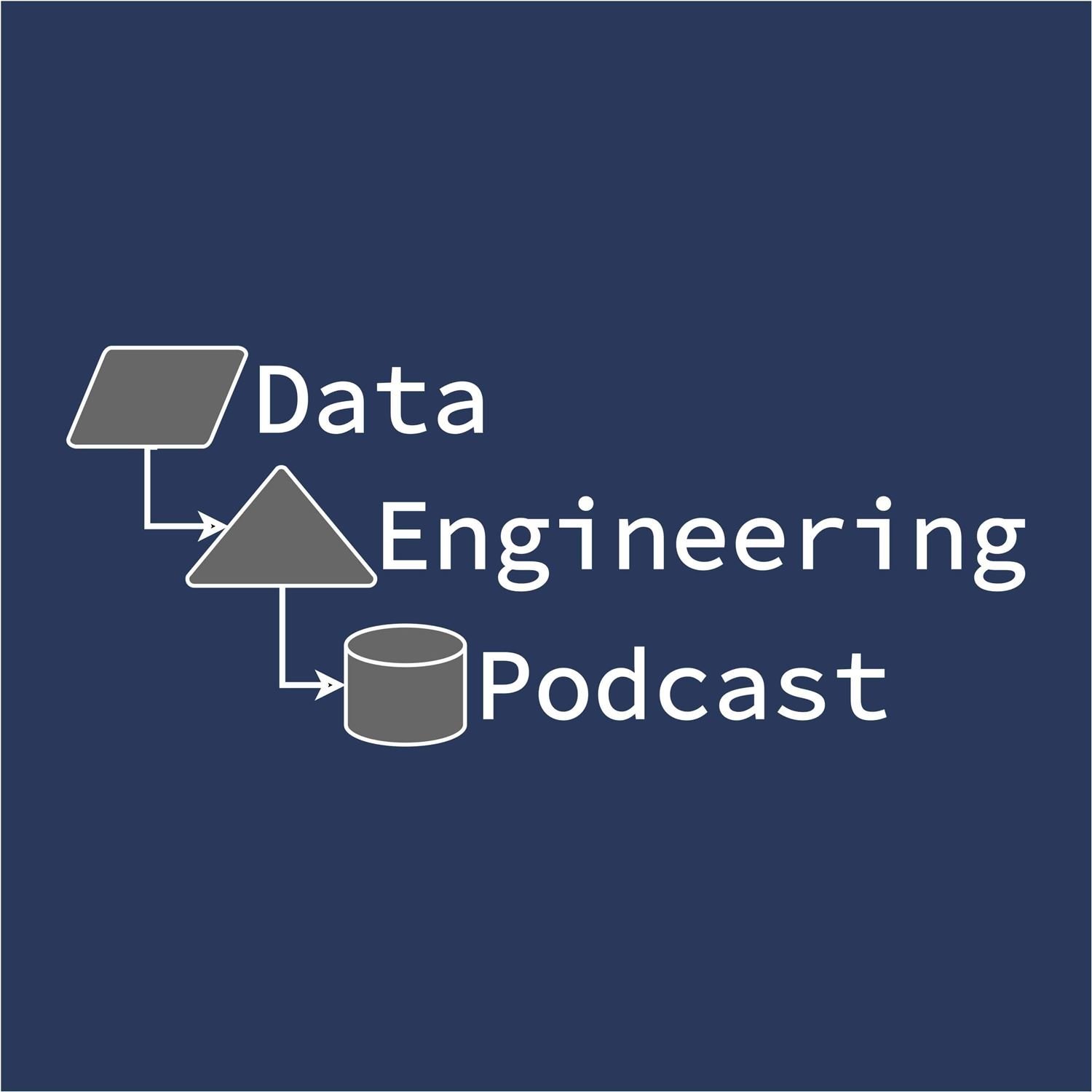
Of Checklists, Ethics, and Data with Emily Miller and Peter Bull (Cross Post from Podcast.__init__) - Episode 53
Summary
As data science becomes more widespread and has a bigger impact on the lives of people, it is important that those projects and products are built with a conscious consideration of ethics. Keeping ethical principles in mind throughout the lifecycle of a data project helps to reduce the overall effort of preventing negative outcomes from the use of the final product. Emily Miller and Peter Bull of Driven Data have created Deon to improve the communication and conversation around ethics among and between data teams. It is a Python project that generates a checklist of common concerns for data oriented projects at the various stages of the lifecycle where they should be considered. In this episode they discuss their motivation for creating the project, the challenges and benefits of maintaining such a checklist, and how you can start using it today.
Preamble
Hello and welcome to the Data Engineering Podcast, the show about modern data management
When you’re ready to build your next pipeline you’ll need somewhere to deploy it, so check out Linode. With private networking, shared block storage, node balancers, and a 40Gbit network, all controlled by a brand new API you’ve got everything you need to run a bullet-proof data platform. Go to dataengineeringpodcast.com/linode to get a $20 credit and launch a new server in under a minute.
Go to dataengineeringpodcast.com to subscribe to the show, sign up for the mailing list, read the show notes, and get in touch.
Join the community in the new Zulip chat workspace at dataengineeringpodcast.com/chat
This is your host Tobias Macey and this week I am sharing an episode from my other show, Podcast.__init__, about a project from Driven Data called Deon. It is a simple tool that generates a checklist of ethical considerations for the various stages of the lifecycle for data oriented projects. This is an important topic for all of the teams involved in the management and creation of projects that leverage data. So give it a listen and if you like what you hear, be sure to check out the other episodes at pythonpodcast.com
Interview
Introductions
How did you get introduced to Python?
Can you start by describing what Deon is and your motivation for creating it?
Why a checklist, specifically? What’s the advantage of this over an oath, for example?
What is unique to data science in terms of the ethical concerns, as compared to traditional software engineering?
What is the typical workflow for a team that is using Deon in their projects?
Deon ships with a default checklist but allows for customization. What are some common addendums that you have seen?
Have you received pushback on any of the default items?
How does Deon simplify communication around ethics across team boundaries?
What are some of the most often overlooked items?
What are some of the most difficult ethical concerns to comply with for a typical data science project?
How has Deon helped you at Driven Data?
What are the customer facing impacts of embedding a discussion of ethics in the product development process?
Some of the items on the default checklist coincide with regulatory requirements. Are there any cases where regulation is in conflict with an ethical concern that you would like to see practiced?
What are your hopes for the future of the Deon project?
Keep In Touch
Emily
LinkedIn
ejm714 on GitHub
Peter
LinkedIn
@pjbull on Twitter
pjbull on GitHub
Driven Data
@drivendataorg on Twitter
drivendataorg on GitHub
Website
Picks
Tobias
Richard Bond Glass Art
Emily
Tandem Coffee in Portland, Maine
Peter
The Model Bakery in Saint Helena and Napa, California
Links
Deon
Driven Data
International Development
Brookings Institution
Stata
Econometrics
Metis Bootcamp
Pandas
Podcast Episode
C#
.NET
Podcast.__init__ Episode On Software Ethics
Jupyter Notebook
Podcast Episode
Word2Vec
cookiecutter data science
Logistic Regression
The intro and outro music is from Requiem for a Fish The Freak Fandango Orchestra / CC BY-SASupport Data Engineering Podcast
45:3222/10/2018
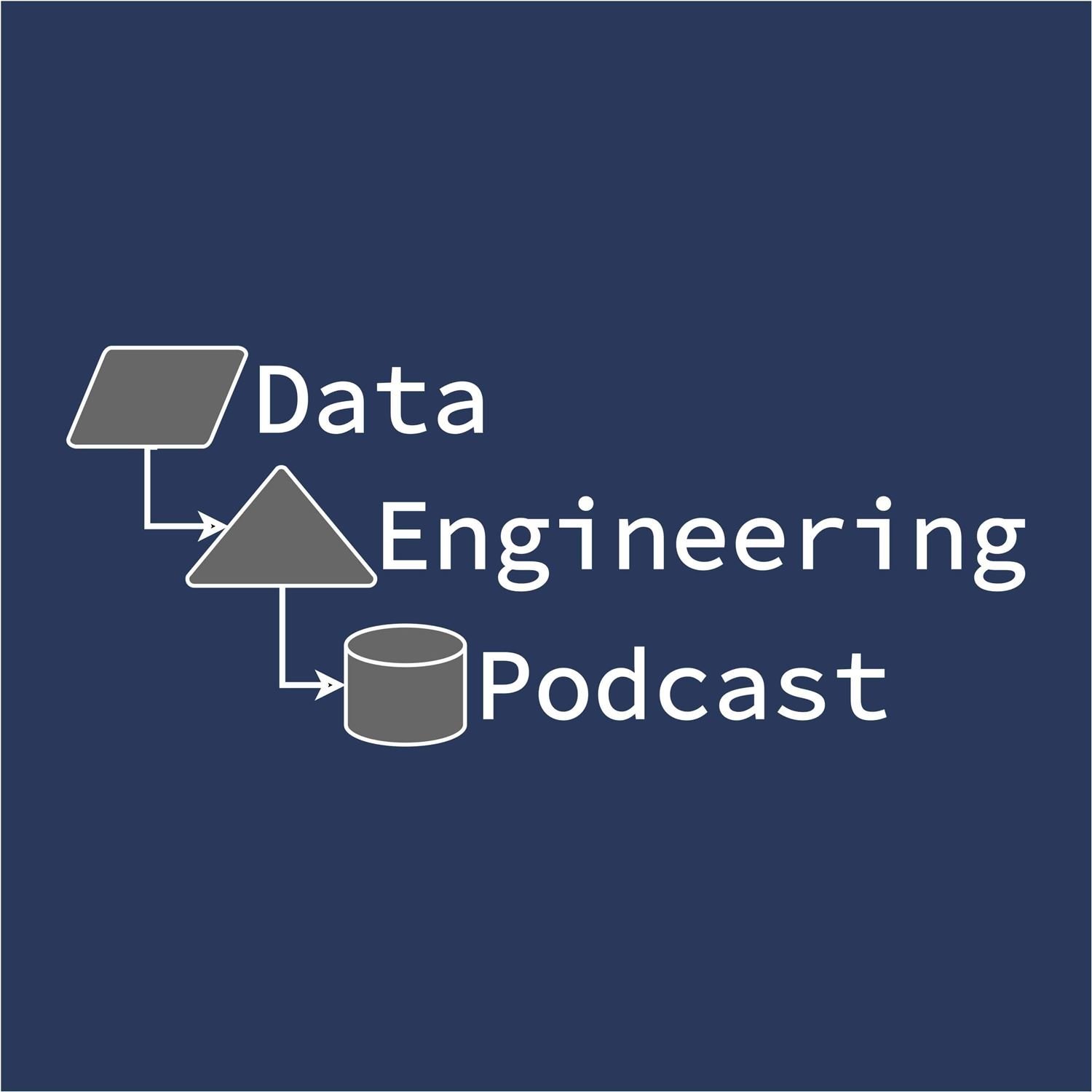
Improving The Performance Of Cloud-Native Big Data At Netflix Using The Iceberg Table Format with Ryan Blue - Episode 52
Summary
With the growth of the Hadoop ecosystem came a proliferation of implementations for the Hive table format. Unfortunately, with no formal specification, each project works slightly different which increases the difficulty of integration across systems. The Hive format is also built with the assumptions of a local filesystem which results in painful edge cases when leveraging cloud object storage for a data lake. In this episode Ryan Blue explains how his work on the Iceberg table format specification and reference implementation has allowed Netflix to improve the performance and simplify operations for their S3 data lake. This is a highly detailed and technical exploration of how a well-engineered metadata layer can improve the speed, accuracy, and utility of large scale, multi-tenant, cloud-native data platforms.
Preamble
Hello and welcome to the Data Engineering Podcast, the show about modern data management
When you’re ready to build your next pipeline you’ll need somewhere to deploy it, so check out Linode. With private networking, shared block storage, node balancers, and a 40Gbit network, all controlled by a brand new API you’ve got everything you need to run a bullet-proof data platform. Go to dataengineeringpodcast.com/linode to get a $20 credit and launch a new server in under a minute.
Go to dataengineeringpodcast.com to subscribe to the show, sign up for the mailing list, read the show notes, and get in touch.
Join the community in the new Zulip chat workspace at dataengineeringpodcast.com/chat
Your host is Tobias Macey and today I’m interviewing Ryan Blue about Iceberg, a Netflix project to implement a high performance table format for batch workloads
Interview
Introduction
How did you get involved in the area of data management?
Can you start by explaining what Iceberg is and the motivation for creating it?
Was the project built with open-source in mind or was it necessary to refactor it from an internal project for public use?
How has the use of Iceberg simplified your work at Netflix?
How is the reference implementation architected and how has it evolved since you first began work on it?
What is involved in deploying it to a user’s environment?
For someone who is interested in using Iceberg within their own environments, what is involved in integrating it with their existing query engine?
Is there a migration path for pre-existing tables into the Iceberg format?
How is schema evolution managed at the file level?
How do you handle files on disk that don’t contain all of the fields specified in a table definition?
One of the complicated problems in data modeling is managing table partitions. How does Iceberg help in that regard?
What are the unique challenges posed by using S3 as the basis for a data lake?
What are the benefits that outweigh the difficulties?
What have been some of the most challenging or contentious details of the specification to define?
What are some things that you have explicitly left out of the specification?
What are your long-term goals for the Iceberg specification?
Do you anticipate the reference implementation continuing to be used and maintained?
Contact Info
rdblue on GitHub
LinkedIn
Parting Question
From your perspective, what is the biggest gap in the tooling or technology for data management today?
Links
Iceberg Reference Implementation
Iceberg Table Specification
Netflix
Hadoop
Cloudera
Avro
Parquet
Spark
S3
HDFS
Hive
ORC
S3mper
Git
Metacat
Presto
Pig
DDL (Data Definition Language)
Cost-Based Optimization
The intro and outro music is from The Hug by The Freak Fandango Orchestra / CC BY-SASupport Data Engineering Podcast
53:4615/10/2018
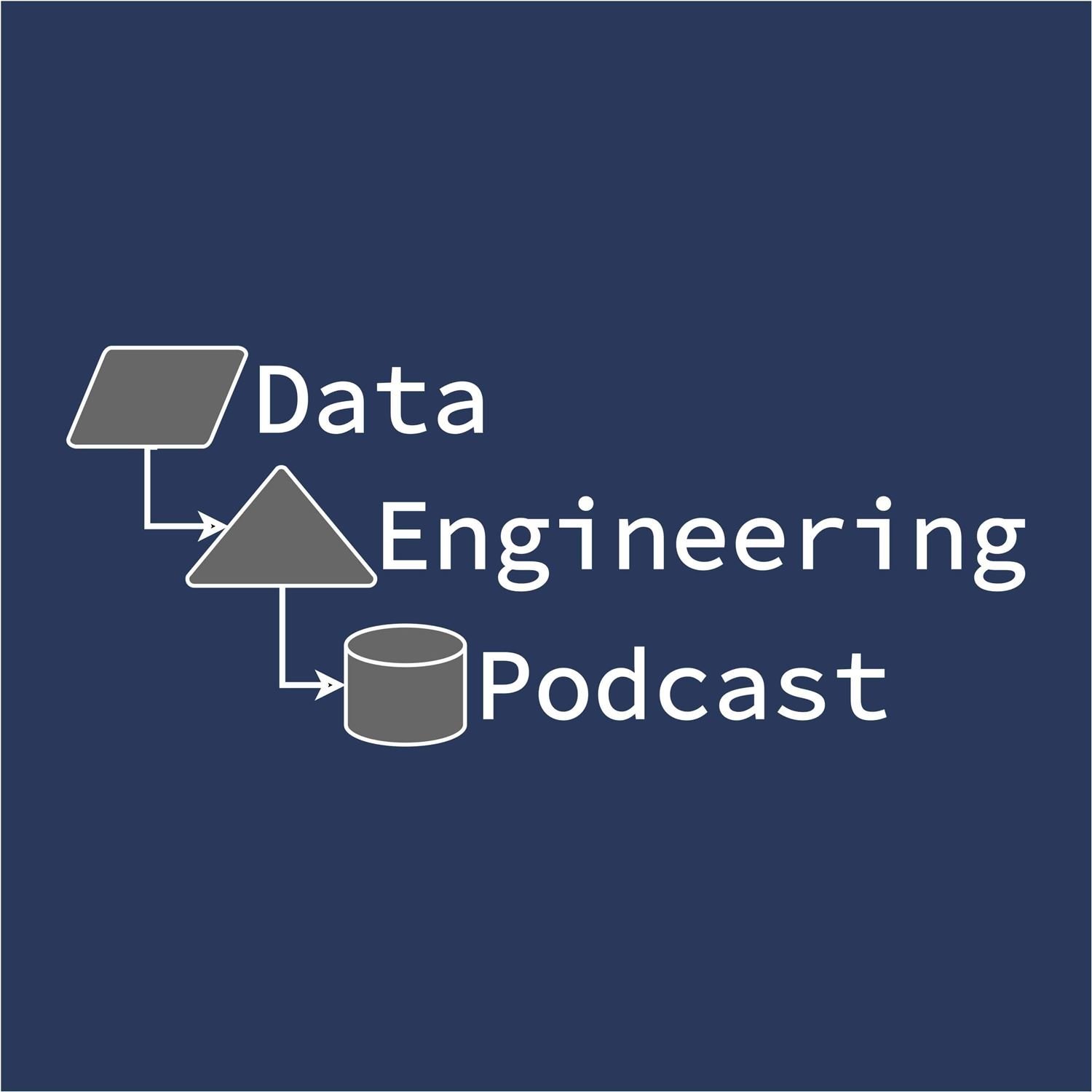
Combining Transactional And Analytical Workloads On MemSQL with Nikita Shamgunov
SummaryOne of the most complex aspects of managing data for analytical workloads is moving it from a transactional database into the data warehouse. What if you didn’t have to do that at all? MemSQL is a distributed database built to support concurrent use by transactional, application oriented, and analytical, high volume, workloads on the same hardware. In this episode the CEO of MemSQL describes how the company and database got started, how it is architected for scale and speed, and how it is being used in production. This was a deep dive on how to build a successful company around a powerful platform, and how that platform simplifies operations for enterprise grade data management.PreambleHello and welcome to the Data Engineering Podcast, the show about modern data managementWhen you’re ready to build your next pipeline you’ll need somewhere to deploy it, so check out Linode. With private networking, shared block storage, node balancers, and a 40Gbit network, all controlled by a brand new API you’ve got everything you need to run a bullet-proof data platform. Go to dataengineeringpodcast.com/linode to get a $20 credit and launch a new server in under a minute.You work hard to make sure that your data is reliable and accurate, but can you say the same about the deployment of your machine learning models? The Skafos platform from Metis Machine was built to give your data scientists the end-to-end support that they need throughout the machine learning lifecycle. Skafos maximizes interoperability with your existing tools and platforms, and offers real-time insights and the ability to be up and running with cloud-based production scale infrastructure instantaneously. Request a demo at dataengineeringpodcast.com/metis-machine to learn more about how Metis Machine is operationalizing data science.And the team at Metis Machine has shipped a proof-of-concept integration between the Skafos machine learning platform and the Tableau business intelligence tool, meaning that your BI team can now run the machine learning models custom built by your data science team. If you think that sounds awesome (and it is) then join the free webinar with Metis Machine on October 11th at 2 PM ET (11 AM PT). Metis Machine will walk through the architecture of the extension, demonstrate its capabilities in real time, and illustrate the use case for empowering your BI team to modify and run machine learning models directly from Tableau. Go to metismachine.com/webinars now to register.Go to dataengineeringpodcast.com to subscribe to the show, sign up for the mailing list, read the show notes, and get in touch.Join the community in the new Zulip chat workspace at dataengineeringpodcast.com/chatYour host is Tobias Macey and today I’m interviewing Nikita Shamgunov about MemSQL, a newSQL database built for simultaneous transactional and analytic workloadsInterviewIntroductionHow did you get involved in the area of data management?Can you start by describing what MemSQL is and how the product and business first got started?What are the typical use cases for customers running MemSQL?What are the benefits of integrating the ingestion pipeline with the database engine? What are some typical ways that the ingest capability is leveraged by customers?How is MemSQL architected and how has the internal design evolved from when you first started working on it?Where does it fall on the axes of the CAP theorem?How much processing overhead is involved in the conversion from the column oriented data stored on disk to the row oriented data stored in memory?Can you describe the lifecycle of a write transaction?Can you discuss the techniques that are used in MemSQL to optimize for speed and overall system performance?How do you mitigate the impact of network latency throughout the cluster during query planning and execution?How much of the implementation of MemSQL is using custom built code vs. open source projects?What are some of the common difficulties that your customers encounter when building on top of or migrating to MemSQL?What have been some of the most challenging aspects of building and growing the technical and business implementation of MemSQL?When is MemSQL the wrong choice for a data platform?What do you have planned for the future of MemSQL?Contact Info@nikitashamgunov on TwitterLinkedInParting QuestionFrom your perspective, what is the biggest gap in the tooling or technology for data management today?LinksMemSQLNewSQLMicrosoft SQL ServerSt. Petersburg University of Fine Mechanics And OpticsCC++In-Memory DatabaseRAM (Random Access Memory)Flash StorageOracle DBPostgreSQLPodcast EpisodeKafkaKinesisWealth ManagementData WarehouseODBCS3HDFSAvroParquetData Serialization Podcast EpisodeBroadcast JoinShuffle JoinCAP TheoremApache ArrowLZ4S2 Geospatial LibrarySybaseSAP HanaKubernetesThe intro and outro music is from The Hug by The Freak Fandango Orchestra / CC BY-SA
56:5509/10/2018
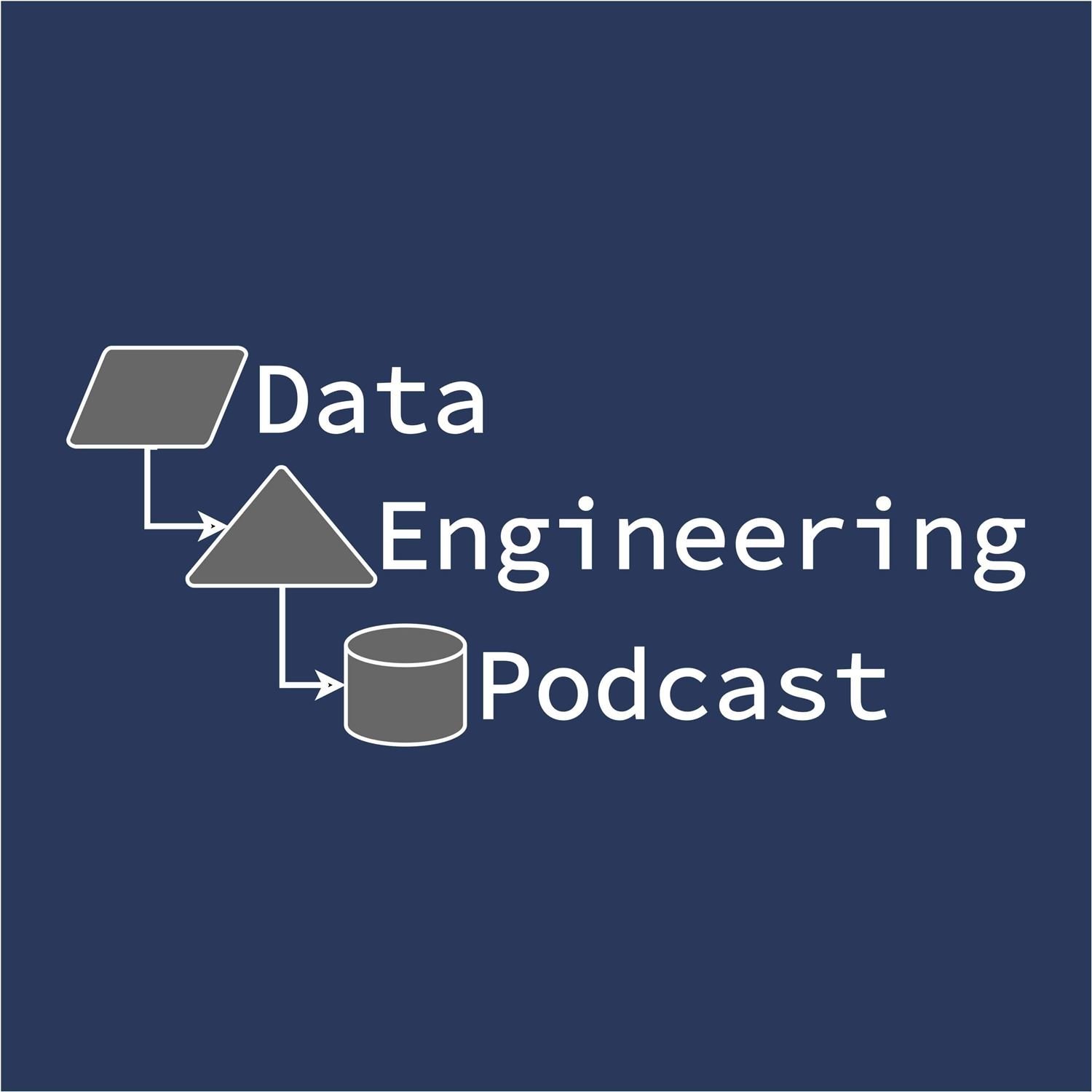
Building A Knowledge Graph From Public Data At Enigma With Chris Groskopf - Episode 50
Summary
There are countless sources of data that are publicly available for use. Unfortunately, combining those sources and making them useful in aggregate is a time consuming and challenging process. The team at Enigma builds a knowledge graph for use in your own data projects. In this episode Chris Groskopf explains the platform they have built to consume large varieties and volumes of public data for constructing a graph for serving to their customers. He discusses the challenges they are facing to scale the platform and engineering processes, as well as the workflow that they have established to enable testing of their ETL jobs. This is a great episode to listen to for ideas on how to organize a data engineering organization.
Preamble
Hello and welcome to the Data Engineering Podcast, the show about modern data management
When you’re ready to build your next pipeline you’ll need somewhere to deploy it, so check out Linode. With private networking, shared block storage, node balancers, and a 40Gbit network, all controlled by a brand new API you’ve got everything you need to run a bullet-proof data platform. Go to dataengineeringpodcast.com/linode to get a $20 credit and launch a new server in under a minute.
You work hard to make sure that your data is reliable and accurate, but can you say the same about the deployment of your machine learning models? The Skafos platform from Metis Machine was built to give your data scientists the end-to-end support that they need throughout the machine learning lifecycle. Skafos maximizes interoperability with your existing tools and platforms, and offers real-time insights and the ability to be up and running with cloud-based production scale infrastructure instantaneously. Request a demo at dataengineeringpodcast.com/metis-machine to learn more about how Metis Machine is operationalizing data science.
Go to dataengineeringpodcast.com to subscribe to the show, sign up for the mailing list, read the show notes, and get in touch.
Join the community in the new Zulip chat workspace at dataengineeringpodcast.com/chat
Your host is Tobias Macey and today I’m interviewing Chris Groskopf about Enigma and how the are using public data sources to build a knowledge graph
Interview
Introduction
How did you get involved in the area of data management?
Can you give a brief overview of what Enigma has built and what the motivation was for starting the company?
How do you define the concept of a knowledge graph?
What are the processes involved in constructing a knowledge graph?
Can you describe the overall architecture of your data platform and the systems that you use for storing and serving your knowledge graph?
What are the most challenging or unexpected aspects of building the knowledge graph that you have encountered?
How do you manage the software lifecycle for your ETL code?
What kinds of unit, integration, or acceptance tests do you run to ensure that you don’t introduce regressions in your processing logic?
What are the current challenges that you are facing in building and scaling your data infrastructure?
How does the fact that your data sources are primarily public influence your pipeline design and what challenges does it pose?
What techniques are you using to manage accuracy and consistency in the data that you ingest?
Can you walk through the lifecycle of the data that you process from acquisition through to delivery to your customers?
What are the weak spots in your platform that you are planning to address in upcoming projects?
If you were to start from scratch today, what would you have done differently?
What are some of the most interesting or unexpected uses of your product that you have seen?
What is in store for the future of Enigma?
Contact Info
Email
Twitter
Parting Question
From your perspective, what is the biggest gap in the tooling or technology for data management today?
Links
Enigma
Chicago Tribune
NPR
Quartz
CSVKit
Agate
Knowledge Graph
Taxonomy
Concourse
Airflow
Docker
S3
Data Lake
Parquet
Podcast Episode
Spark
AWS Neptune
AWS Batch
Money Laundering
Jupyter Notebook
Papermill
Jupytext
Cauldron: The Un-Notebook
Podcast.__init__ Episode
The intro and outro music is from The Hug by The Freak Fandango Orchestra / CC BY-SASupport Data Engineering Podcast
52:5301/10/2018
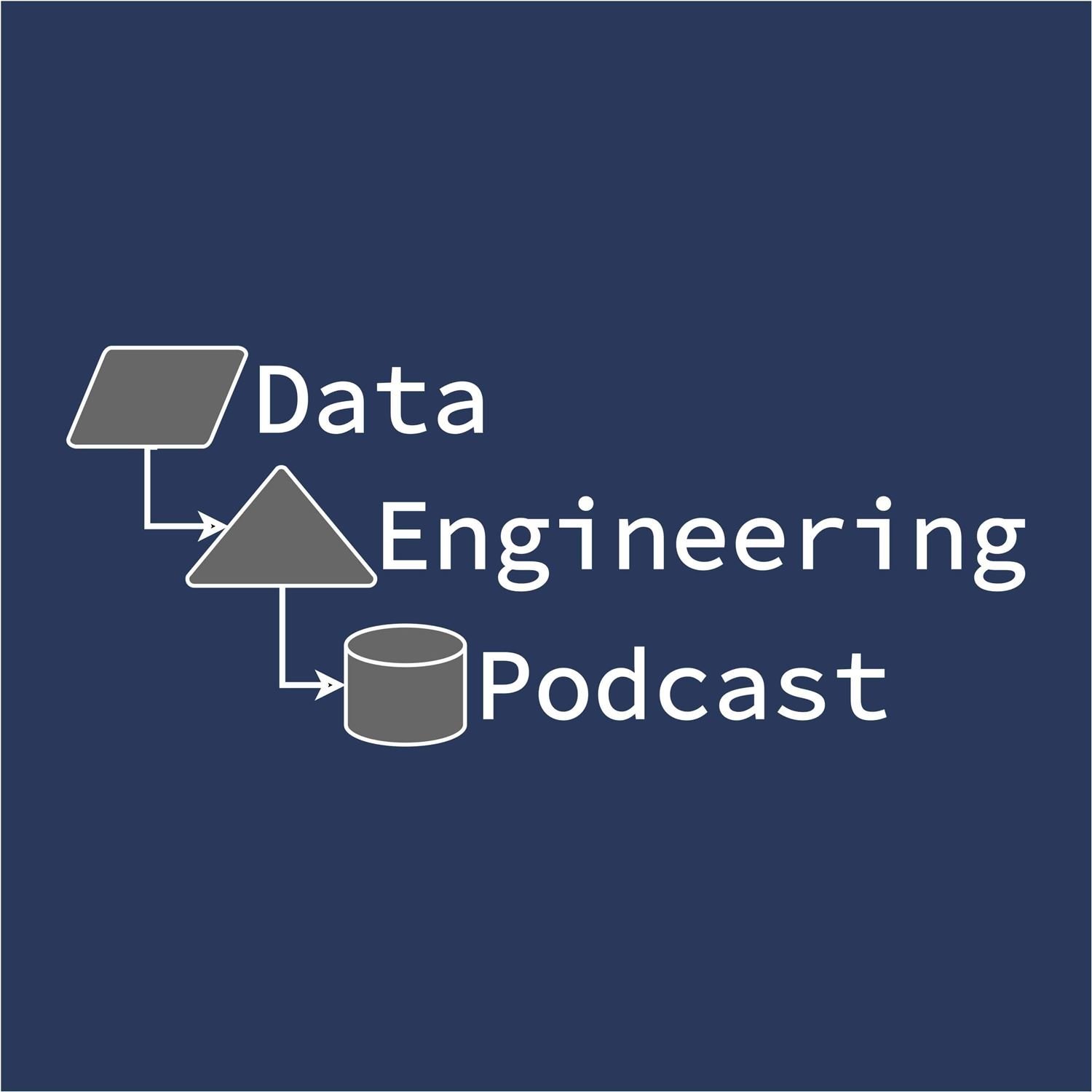
A Primer On Enterprise Data Curation with Todd Walter - Episode 49
Summary
As your data needs scale across an organization the need for a carefully considered approach to collection, storage, organization, and access becomes increasingly critical. In this episode Todd Walter shares his considerable experience in data curation to clarify the many aspects that are necessary for a successful platform for your business. Using the metaphor of a museum curator carefully managing the precious resources on display and in the vaults, he discusses the various layers of an enterprise data strategy. This includes modeling the lifecycle of your information as a pipeline from the raw, messy, loosely structured records in your data lake, through a series of transformations and ultimately to your data warehouse. He also explains which layers are useful for the different members of the business, and which pitfalls to look out for along the path to a mature and flexible data platform.
Preamble
Hello and welcome to the Data Engineering Podcast, the show about modern data management
When you’re ready to build your next pipeline you’ll need somewhere to deploy it, so check out Linode. With private networking, shared block storage, node balancers, and a 40Gbit network, all controlled by a brand new API you’ve got everything you need to run a bullet-proof data platform. Go to dataengineeringpodcast.com/linode to get a $20 credit and launch a new server in under a minute.
You work hard to make sure that your data is reliable and accurate, but can you say the same about the deployment of your machine learning models? The Skafos platform from Metis Machine was built to give your data scientists the end-to-end support that they need throughout the machine learning lifecycle. Skafos maximizes interoperability with your existing tools and platforms, and offers real-time insights and the ability to be up and running with cloud-based production scale infrastructure instantaneously. Request a demo at dataengineeringpodcast.com/metis-machine to learn more about how Metis Machine is operationalizing data science.
Go to dataengineeringpodcast.com to subscribe to the show, sign up for the mailing list, read the show notes, and get in touch.
Join the community in the new Zulip chat workspace at dataengineeringpodcast.com/chat
Your host is Tobias Macey and today I’m interviewing Todd Walter about data curation and how to architect your data systems to support high quality, maintainable intelligence
Interview
Introduction
How did you get involved in the area of data management?
How do you define data curation?
What are some of the high level concerns that are encapsulated in that effort?
How does the size and maturity of a company affect the ways that they architect and interact with their data systems?
Can you walk through the stages of an ideal lifecycle for data within the context of an organizations uses for it?
What are some of the common mistakes that are made when designing a data architecture and how do they lead to failure?
What has changed in terms of complexity and scope for data architecture and curation since you first started working in this space?
As “big data” became more widely discussed the common mantra was to store everything because you never know when you’ll need the data that might get thrown away. As the industry is reaching a greater degree of maturity and more regulations are implemented there has been a shift to being more considerate as to what information gets stored and for how long. What are your views on that evolution and what is your litmus test for determining which data to keep?
In terms of infrastructure, what are the components of a modern data architecture and how has that changed over the years?
What is your opinion on the relative merits of a data warehouse vs a data lake and are they mutually exclusive?
Once an architecture has been established, how do you allow for continued evolution to prevent stagnation and eventual failure?
ETL has long been the default approach for building and enforcing data architecture, but there have been significant shifts in recent years due to the emergence of streaming systems and ELT approaches in new data warehouses. What are your thoughts on the landscape for managing data flows and migration and when to use which approach?
What are some of the areas of data architecture and curation that are most often forgotten or ignored?
What resources do you recommend for anyone who is interested in learning more about the landscape of data architecture and curation?
Contact Info
LinkedIn
Parting Question
From your perspective, what is the biggest gap in the tooling or technology for data management today?
Links
Teradata
Data Architecture
Data Curation
Data Warehouse
Chief Data Officer
ETL (Extract, Transform, Load)
Data Lake
Metadata
Data Lineage
Data Provenance
Strata Conference
ELT (Extract, Load, Transform)
Map-Reduce
Hive
Pig
Spark
Data Governance
The intro and outro music is from The Hug by The Freak Fandango Orchestra / CC BY-SA
Support Data Engineering Podcast
49:3524/09/2018
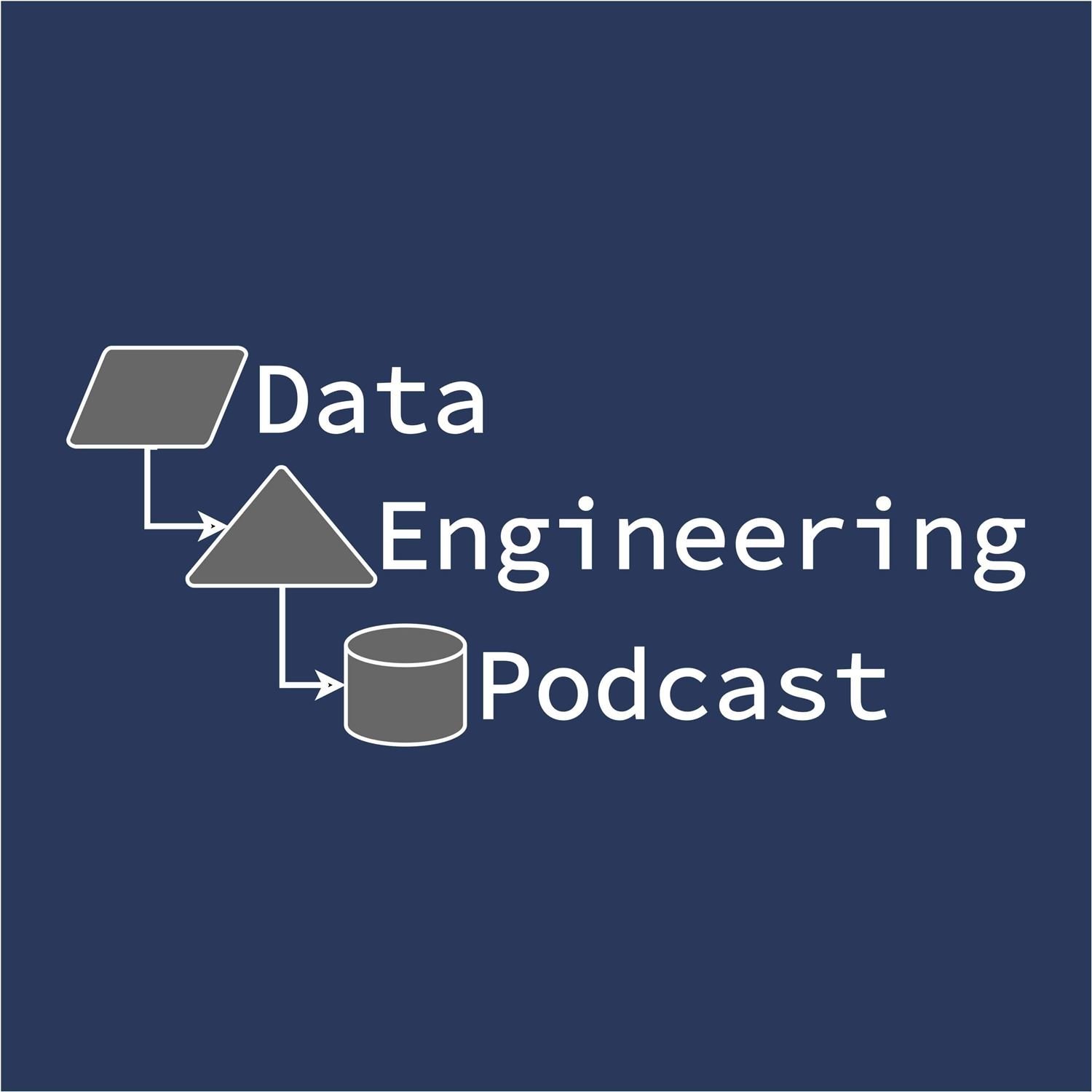
Take Control Of Your Web Analytics Using Snowplow With Alexander Dean - Episode 48
Summary
Every business with a website needs some way to keep track of how much traffic they are getting, where it is coming from, and which actions are being taken. The default in most cases is Google Analytics, but this can be limiting when you wish to perform detailed analysis of the captured data. To address this problem, Alex Dean co-founded Snowplow Analytics to build an open source platform that gives you total control of your website traffic data. In this episode he explains how the project and company got started, how the platform is architected, and how you can start using it today to get a clearer view of how your customers are interacting with your web and mobile applications.
Preamble
Hello and welcome to the Data Engineering Podcast, the show about modern data management
When you’re ready to build your next pipeline you’ll need somewhere to deploy it, so check out Linode. With private networking, shared block storage, node balancers, and a 40Gbit network, all controlled by a brand new API you’ve got everything you need to run a bullet-proof data platform. Go to dataengineeringpodcast.com/linode to get a $20 credit and launch a new server in under a minute.
You work hard to make sure that your data is reliable and accurate, but can you say the same about the deployment of your machine learning models? The Skafos platform from Metis Machine was built to give your data scientists the end-to-end support that they need throughout the machine learning lifecycle. Skafos maximizes interoperability with your existing tools and platforms, and offers real-time insights and the ability to be up and running with cloud-based production scale infrastructure instantaneously. Request a demo at dataengineeringpodcast.com/metis-machine to learn more about how Metis Machine is operationalizing data science.
Go to dataengineeringpodcast.com to subscribe to the show, sign up for the mailing list, read the show notes, and get in touch.
Join the community in the new Zulip chat workspace at dataengineeringpodcast.com/chat
This is your host Tobias Macey and today I’m interviewing Alexander Dean about Snowplow Analytics
Interview
Introductions
How did you get involved in the area of data engineering and data management?
What is Snowplow Analytics and what problem were you trying to solve when you started the company?
What is unique about customer event data from an ingestion and processing perspective?
Challenges with properly matching up data between sources
Data collection is one of the more difficult aspects of an analytics pipeline because of the potential for inconsistency or incorrect information. How is the collection portion of the Snowplow stack designed and how do you validate the correctness of the data?
Cleanliness/accuracy
What kinds of metrics should be tracked in an ingestion pipeline and how do you monitor them to ensure that everything is operating properly?
Can you describe the overall architecture of the ingest pipeline that Snowplow provides?
How has that architecture evolved from when you first started?
What would you do differently if you were to start over today?
Ensuring appropriate use of enrichment sources
What have been some of the biggest challenges encountered while building and evolving Snowplow?
What are some of the most interesting uses of your platform that you are aware of?
Keep In Touch
Alex
@alexcrdean on Twitter
LinkedIn
Snowplow
@snowplowdata on Twitter
Parting Question
From your perspective, what is the biggest gap in the tooling or technology for data management today?
Links
Snowplow
GitHub
Deloitte Consulting
OpenX
Hadoop
AWS
EMR (Elastic Map-Reduce)
Business Intelligence
Data Warehousing
Google Analytics
CRM (Customer Relationship Management)
S3
GDPR (General Data Protection Regulation)
Kinesis
Kafka
Google Cloud Pub-Sub
JSON-Schema
Iglu
IAB Bots And Spiders List
Heap Analytics
Podcast Interview
Redshift
SnowflakeDB
Snowplow Insights
Google Cloud Platform
Azure
GitLab
The intro and outro music is from The Hug by The Freak Fandango Orchestra / CC BY-SASupport Data Engineering Podcast
47:4917/09/2018
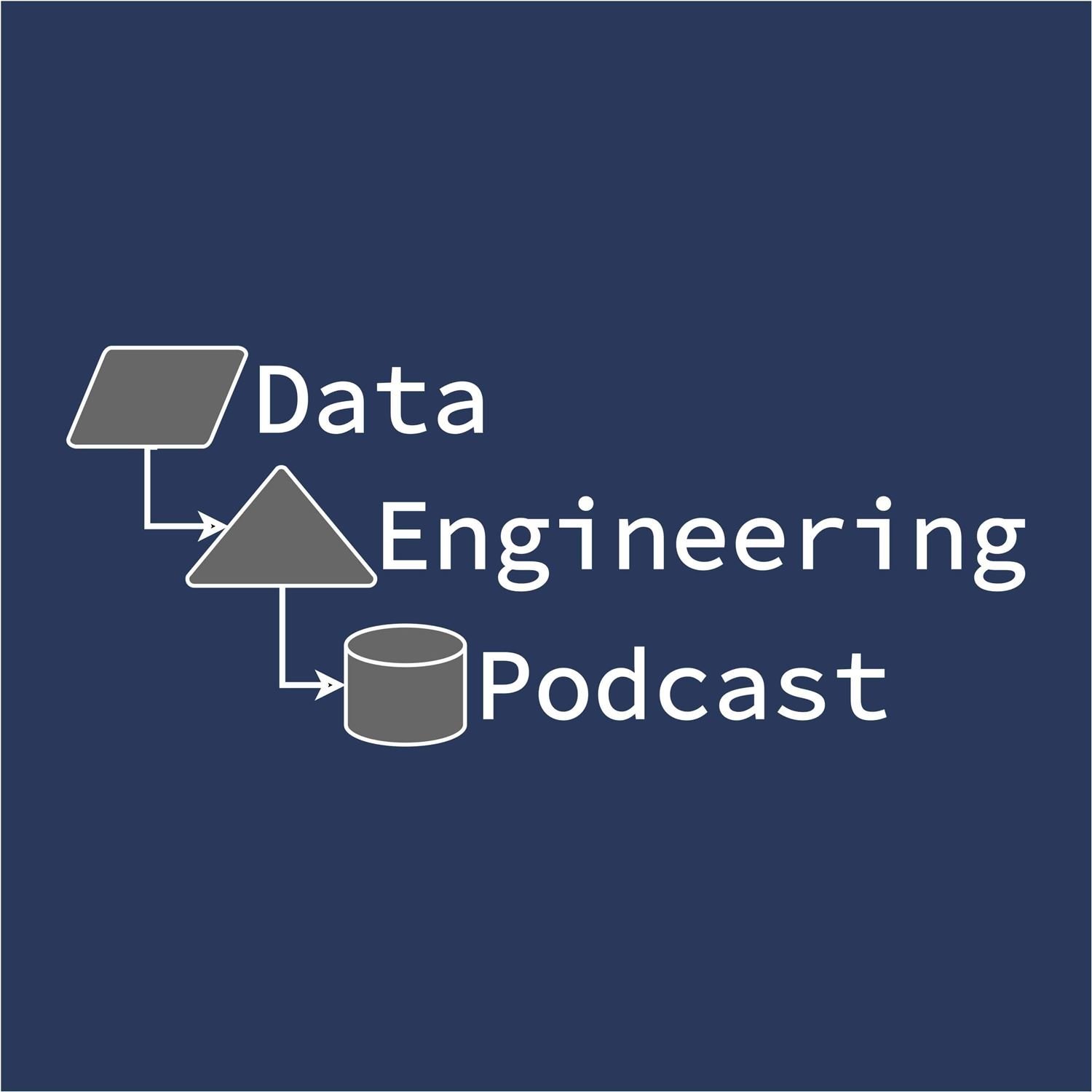
Keep Your Data And Query It Too Using Chaos Search with Thomas Hazel and Pete Cheslock - Episode 47
Summary
Elasticsearch is a powerful tool for storing and analyzing data, but when using it for logs and other time oriented information it can become problematic to keep all of your history. Chaos Search was started to make it easy for you to keep all of your data and make it usable in S3, so that you can have the best of both worlds. In this episode the CTO, Thomas Hazel, and VP of Product, Pete Cheslock, describe how they have built a platform to let you keep all of your history, save money, and reduce your operational overhead. They also explain some of the types of data that you can use with Chaos Search, how to load it into S3, and when you might want to choose it over Amazon Athena for our serverless data analysis.
Preamble
Hello and welcome to the Data Engineering Podcast, the show about modern data management
When you’re ready to build your next pipeline you’ll need somewhere to deploy it, so check out Linode. With private networking, shared block storage, node balancers, and a 40Gbit network, all controlled by a brand new API you’ve got everything you need to run a bullet-proof data platform. Go to dataengineeringpodcast.com/linode to get a $/0 credit and launch a new server in under a minute.
You work hard to make sure that your data is reliable and accurate, but can you say the same about the deployment of your machine learning models? The Skafos platform from Metis Machine was built to give your data scientists the end-to-end support that they need throughout the machine learning lifecycle. Skafos maximizes interoperability with your existing tools and platforms, and offers real-time insights and the ability to be up and running with cloud-based production scale infrastructure instantaneously. Request a demo at dataengineeringpodcast.com/metis-machine to learn more about how Metis Machine is operationalizing data science.
Go to dataengineeringpodcast.com to subscribe to the show, sign up for the mailing list, read the show notes, and get in touch.
Join the community in the new Zulip chat workspace at dataengineeringpodcast.com/chat
Your host is Tobias Macey and today I’m interviewing Pete Cheslock and Thomas Hazel about Chaos Search and their effort to bring historical depth to your Elasticsearch data
Interview
Introduction
How did you get involved in the area of data management?
Can you start by explaining what you have built at Chaos Search and the problems that you are trying to solve with it?
What types of data are you focused on supporting?
What are the challenges inherent to scaling an elasticsearch infrastructure to large volumes of log or metric data?
Is there any need for an Elasticsearch cluster in addition to Chaos Search?
For someone who is using Chaos Search, what mechanisms/formats would they use for loading their data into S3?
What are the benefits of implementing the Elasticsearch API on top of your data in S3 as opposed to using systems such as Presto or Drill to interact with the same information via SQL?
Given that the S3 API has become a de facto standard for many other object storage platforms, what would be involved in running Chaos Search on data stored outside of AWS?
What mechanisms do you use to allow for such drastic space savings of indexed data in S3 versus in an Elasticsearch cluster?
What is the system architecture that you have built to allow for querying terabytes of data in S3?
What are the biggest contributors to query latency and what have you done to mitigate them?
What are the options for access control when running queries against the data stored in S3?
What are some of the most interesting or unexpected uses of Chaos Search and access to large amounts of historical log information that you have seen?
What are your plans for the future of Chaos Search?
Contact Info
Pete Cheslock
@petecheslock on Twitter
Website
Thomas Hazel
@thomashazel on Twitter
LinkedIn
Parting Question
From your perspective, what is the biggest gap in the tooling or technology for data management today?
Links
Chaos Search
AWS S3
Cassandra
Elasticsearch
Podcast Interview
PostgreSQL
Distributed Systems
Information Theory
Lucene
Inverted Index
Kibana
Logstash
NVMe
AWS KMS
Kinesis
FluentD
Parquet
Athena
Presto
Drill
Backblaze
OpenStack Swift
Minio
EMR
DataDog
NewRelic
Elastic Beats
Metricbeat
Graphite
Snappy
Scala
Akka
Elastalert
Tensorflow
X-Pack
Data Lake
The intro and outro music is from The Hug by The Freak Fandango Orchestra / CC BY-SASupport Data Engineering Podcast
48:0910/09/2018