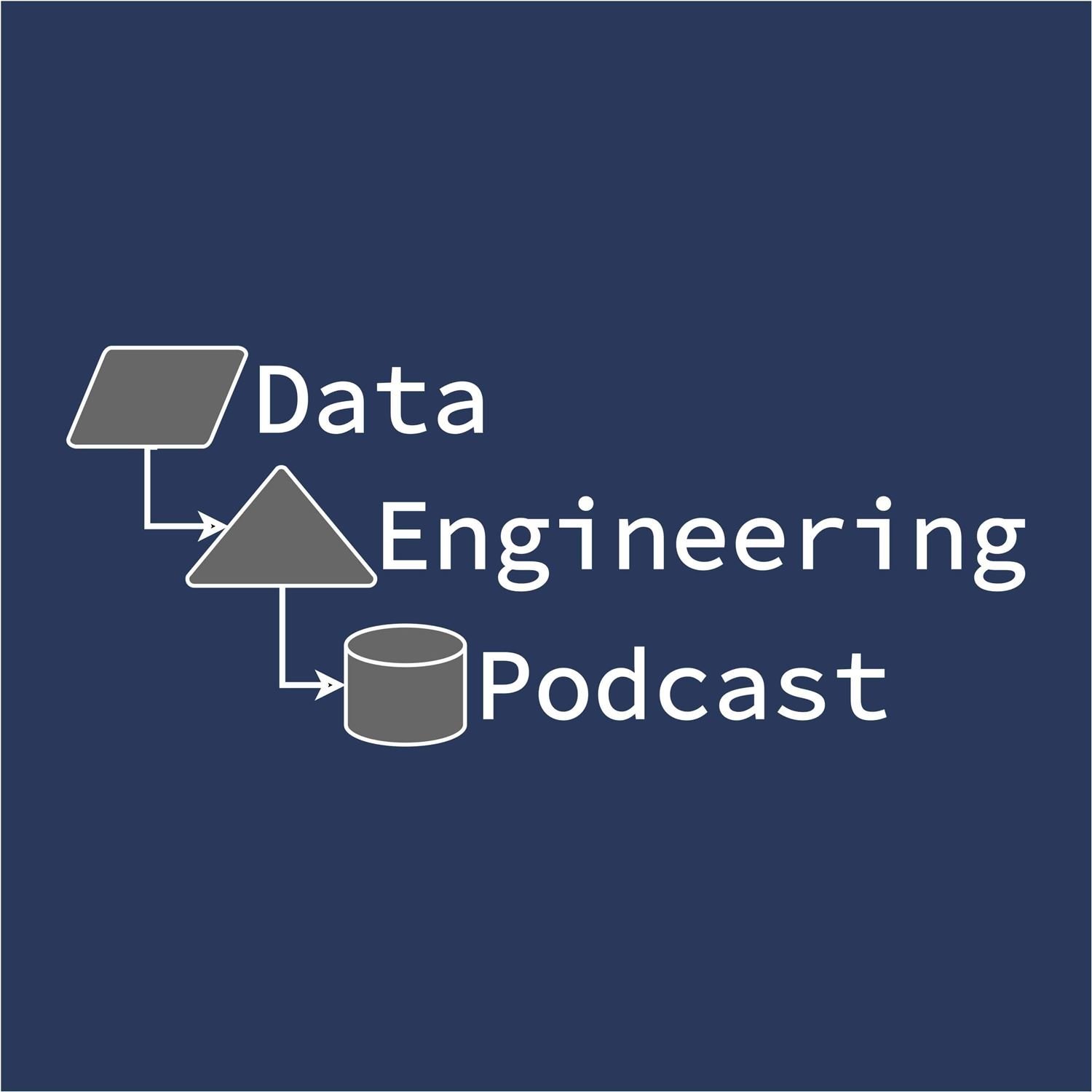
Education
Technology
Tobias Macey
This show goes behind the scenes for the tools, techniques, and difficulties associated with the discipline of data engineering. Databases, workflows, automation, and data manipulation are just some of the topics that you will find here.
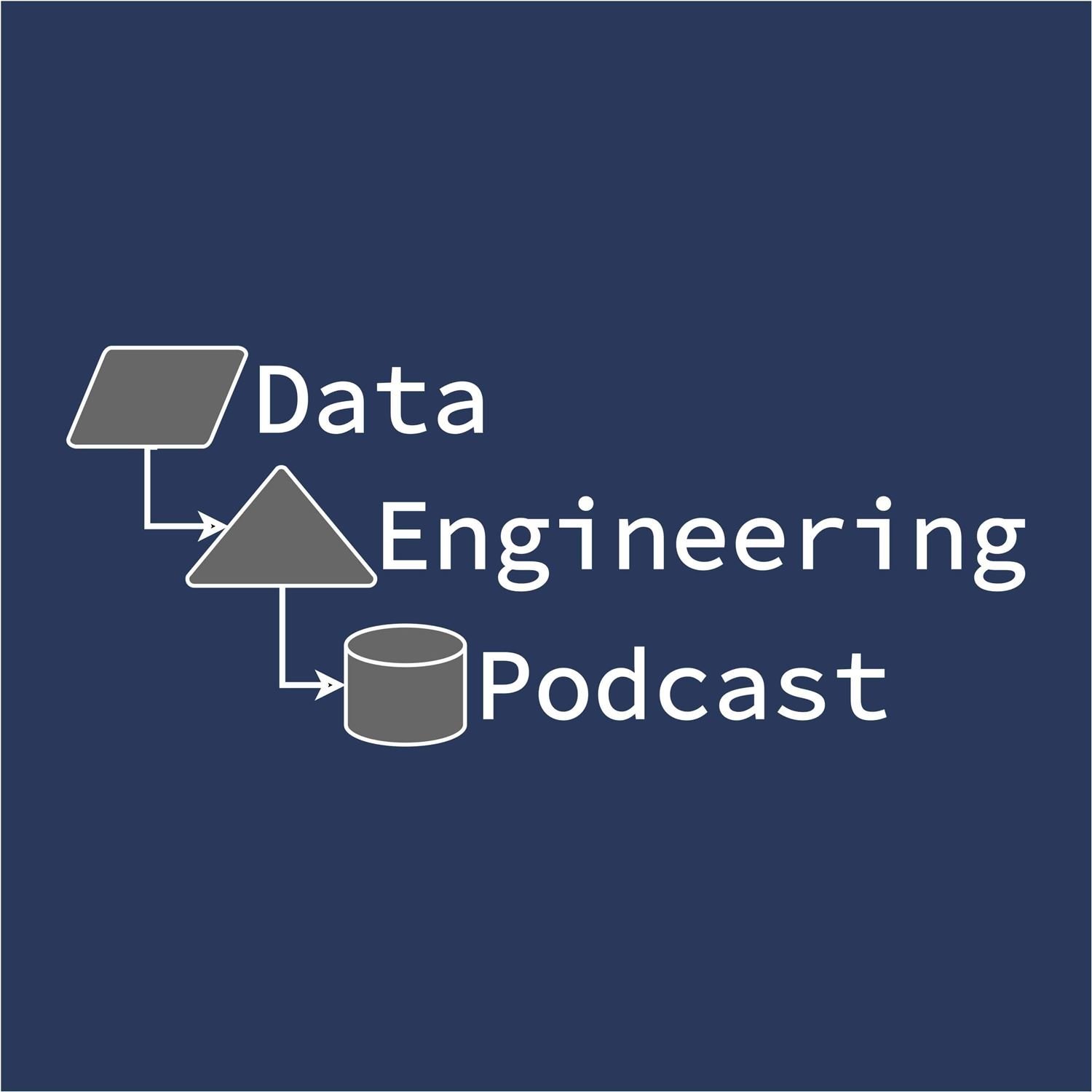
Streaming Data Into The Lakehouse With Iceberg And Trino At Going
In this episode, I had the pleasure of speaking with Ken Pickering, VP of Engineering at Going, about the intricacies of streaming data into a Trino and Iceberg lakehouse. Ken shared his journey from product engineering to becoming deeply involved in data-centric roles, highlighting his experiences in ecommerce and InsurTech. At Going, Ken leads the data platform team, focusing on finding travel deals for consumers, a task that involves handling massive volumes of flight data and event stream information.Ken explained the dual approach of passive and active search strategies used by Going to manage the vast data landscape. Passive search involves aggregating data from global distribution systems, while active search is more transactional, querying specific flight prices. This approach helps Going sift through approximately 50 petabytes of data annually to identify the best travel deals.We delved into the technical architecture supporting these operations, including the use of Confluent for data streaming, Starburst Galaxy for transformation, and Databricks for modeling. Ken emphasized the importance of an open lakehouse architecture, which allows for flexibility and scalability as the business grows.Ken also discussed the composition of Going's engineering and data teams, highlighting the collaborative nature of their work and the reliance on vendor tooling to streamline operations. He shared insights into the challenges and strategies of managing data life cycles, ensuring data quality, and maintaining uptime for consumer-facing applications.Throughout our conversation, Ken provided a glimpse into the future of Going's data architecture, including potential expansions into other travel modes and the integration of large language models for enhanced customer interaction. This episode offers a comprehensive look at the complexities and innovations in building a data-driven travel advisory service.
39:4918/11/2024
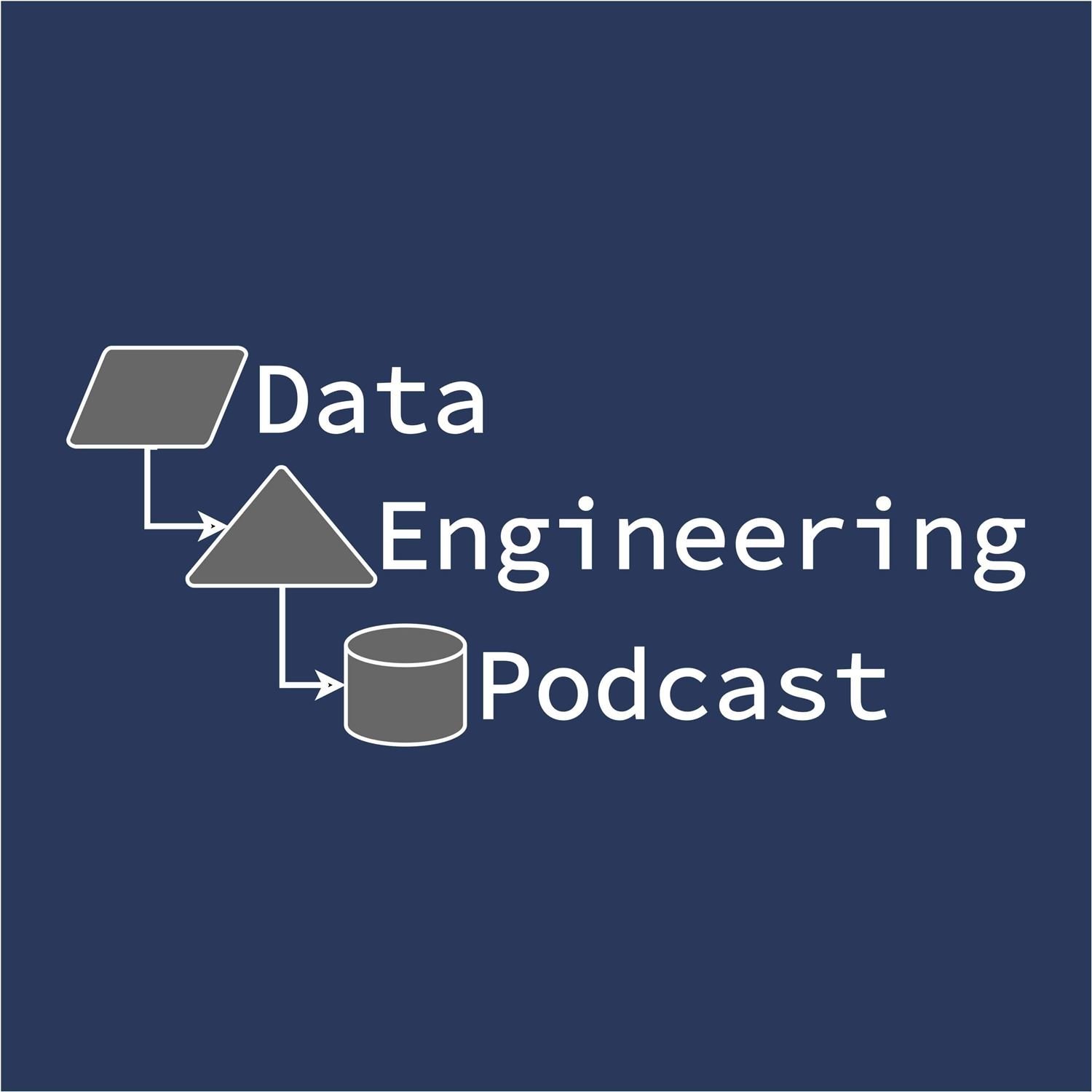
An Opinionated Look At End-to-end Code Only Analytical Workflows With Bruin
SummaryThe challenges of integrating all of the tools in the modern data stack has led to a new generation of tools that focus on a fully integrated workflow. At the same time, there have been many approaches to how much of the workflow is driven by code vs. not. Burak Karakan is of the opinion that a fully integrated workflow that is driven entirely by code offers a beneficial and productive means of generating useful analytical outcomes. In this episode he shares how Bruin builds on those opinions and how you can use it to build your own analytics without having to cobble together a suite of tools with conflicting abstractions.AnnouncementsHello and welcome to the Data Engineering Podcast, the show about modern data managementImagine catching data issues before they snowball into bigger problems. That’s what Datafold’s new Monitors do. With automatic monitoring for cross-database data diffs, schema changes, key metrics, and custom data tests, you can catch discrepancies and anomalies in real time, right at the source. Whether it’s maintaining data integrity or preventing costly mistakes, Datafold Monitors give you the visibility and control you need to keep your entire data stack running smoothly. Want to stop issues before they hit production? Learn more at dataengineeringpodcast.com/datafold today!Your host is Tobias Macey and today I'm interviewing Burak Karakan about the benefits of building code-only data systemsInterviewIntroductionHow did you get involved in the area of data management?Can you describe what Bruin is and the story behind it?Who is your target audience?There are numerous tools that address the ETL workflow for analytical data. What are the pain points that you are focused on for your target users?How does a code-only approach to data pipelines help in addressing the pain points of analytical workflows?How might it act as a limiting factor for organizational involvement?Can you describe how Bruin is designed?How have the design and scope of Bruin evolved since you first started working on it?You call out the ability to mix SQL and Python for transformation pipelines. What are the components that allow for that functionality?What are some of the ways that the combination of Python and SQL improves ergonomics of transformation workflows?What are the key features of Bruin that help to streamline the efforts of organizations building analytical systems?Can you describe the workflow of someone going from source data to warehouse and dashboard using Bruin and Ingestr?What are the opportunities for contributions to Bruin and Ingestr to expand their capabilities?What are the most interesting, innovative, or unexpected ways that you have seen Bruin and Ingestr used?What are the most interesting, unexpected, or challenging lessons that you have learned while working on Bruin?When is Bruin the wrong choice?What do you have planned for the future of Bruin?Contact InfoLinkedInParting QuestionFrom your perspective, what is the biggest gap in the tooling or technology for data management today?Closing AnnouncementsThank you for listening! Don't forget to check out our other shows. Podcast.__init__ covers the Python language, its community, and the innovative ways it is being used. The AI Engineering Podcast is your guide to the fast-moving world of building AI systems.Visit the site to subscribe to the show, sign up for the mailing list, and read the show notes.If you've learned something or tried out a project from the show then tell us about it! Email [email protected] with your story.LinksBruinFivetranStitchIngestrBruin CLIMeltanoSQLGlotdbtSQLMeshPodcast EpisodeSDFPodcast EpisodeAirflowDagsterSnowparkAtlanEvidenceThe intro and outro music is from The Hug by The Freak Fandango Orchestra / CC BY-SA
56:1111/11/2024
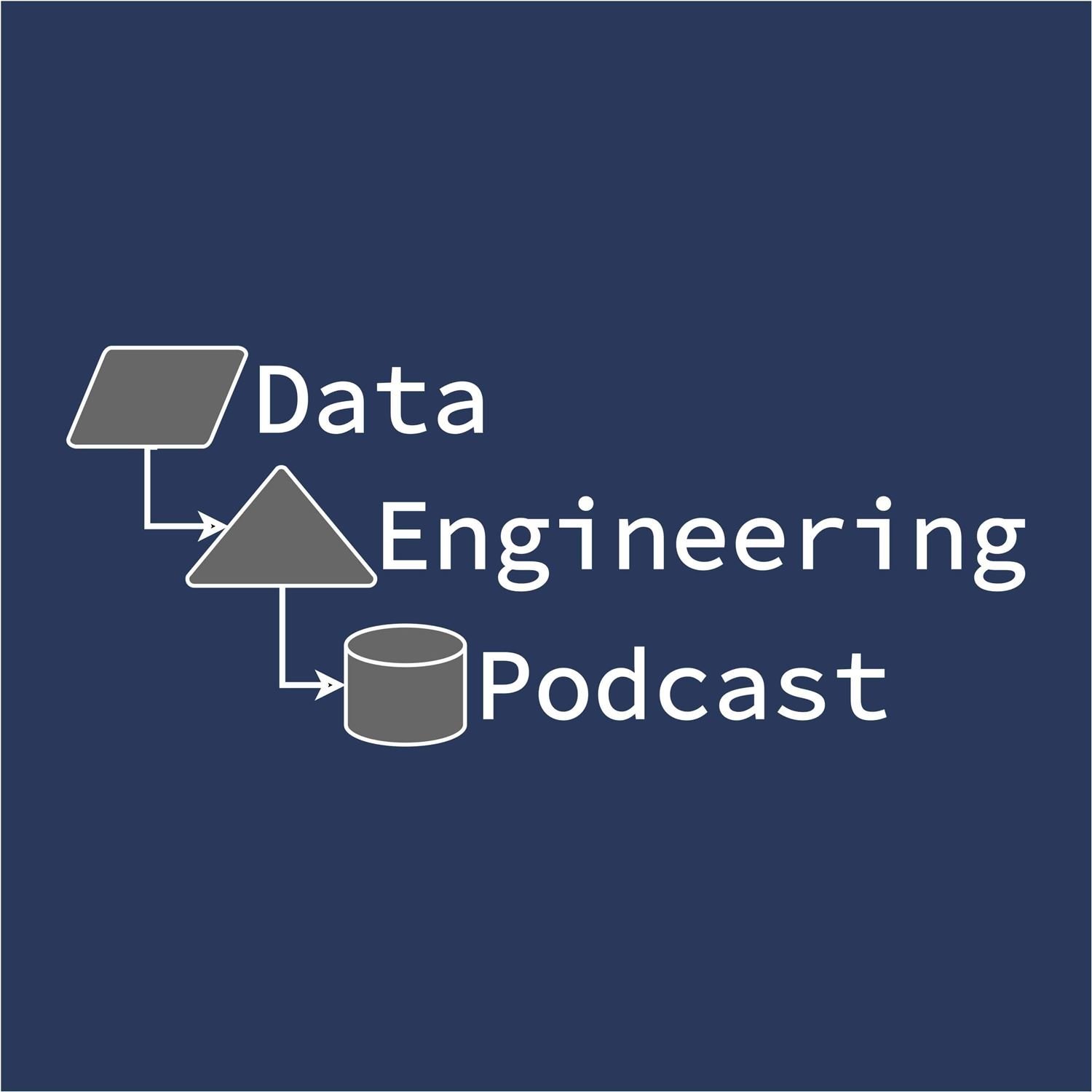
Feldera: Bridging Batch and Streaming with Incremental Computation
SummaryIn this episode of the Data Engineering Podcast, the creators of Feldera talk about their incremental compute engine designed for continuous computation of data, machine learning, and AI workloads. The discussion covers the concept of incremental computation, the origins of Feldera, and its unique ability to handle both streaming and batch data seamlessly. The guests explore Feldera's architecture, applications in real-time machine learning and AI, and challenges in educating users about incremental computation. They also discuss the balance between open-source and enterprise offerings, and the broader implications of incremental computation for the future of data management, predicting a shift towards unified systems that handle both batch and streaming data efficiently.AnnouncementsHello and welcome to the Data Engineering Podcast, the show about modern data managementImagine catching data issues before they snowball into bigger problems. That’s what Datafold’s new Monitors do. With automatic monitoring for cross-database data diffs, schema changes, key metrics, and custom data tests, you can catch discrepancies and anomalies in real time, right at the source. Whether it’s maintaining data integrity or preventing costly mistakes, Datafold Monitors give you the visibility and control you need to keep your entire data stack running smoothly. Want to stop issues before they hit production? Learn more at dataengineeringpodcast.com/datafold today!As a listener of the Data Engineering Podcast you clearly care about data and how it affects your organization and the world. For even more perspective on the ways that data impacts everything around us you should listen to Data Citizens® Dialogues, the forward-thinking podcast from the folks at Collibra. You'll get further insights from industry leaders, innovators, and executives in the world's largest companies on the topics that are top of mind for everyone. They address questions around AI governance, data sharing, and working at global scale. In particular I appreciate the ability to hear about the challenges that enterprise scale businesses are tackling in this fast-moving field. While data is shaping our world, Data Citizens Dialogues is shaping the conversation. Subscribe to Data Citizens Dialogues on Apple, Spotify, Youtube, or wherever you get your podcasts.Your host is Tobias Macey and today I'm interviewing Leonid Ryzhyk, Lalith Suresh, and Mihai Budiu about Feldera, an incremental compute engine for continous computation of data, ML, and AI workloadsInterviewIntroductionCan you describe what Feldera is and the story behind it?DBSP (the theory behind Feldera) has won multiple awards from the database research community. Can you explain what it is and how it solves the incremental computation problem?Depending on which angle you look at it, Feldera has attributes of data warehouses, federated query engines, and stream processors. What are the unique use cases that Feldera is designed to address?In what situations would you replace another technology with Feldera?When is it an additive technology?Can you describe the architecture of Feldera?How have the design and scope evolved since you first started working on it?What are the state storage interfaces available in Feldera?What are the opportunities for integrating with or building on top of open table formats like Iceberg, Lance, Hudi, etc.?Can you describe a typical workflow for an engineer building with Feldera?You advertise Feldera's utility in ML and AI use cases in addition to data management. What are the features that make it conducive to those applications?What is your philosophy toward the community growth and engagement with the open source aspects of Feldera and how you're balancing that with sustainability of the project and business?What are the most interesting, innovative, or unexpected ways that you have seen Feldera used?What are the most interesting, unexpected, or challenging lessons that you have learned while working on Feldera?When is Feldera the wrong choice?What do you have planned for the future of Feldera?Contact InfoLeonidWebsiteGitHubLinkedInLalithLinkedInWebsiteMihaiWebsiteGitHubParting QuestionFrom your perspective, what is the biggest gap in the tooling or technology for data management today?Closing AnnouncementsThank you for listening! Don't forget to check out our other shows. Podcast.__init__ covers the Python language, its community, and the innovative ways it is being used. The AI Engineering Podcast is your guide to the fast-moving world of building AI systems.Visit the site to subscribe to the show, sign up for the mailing list, and read the show notes.If you've learned something or tried out a project from the show then tell us about it! Email [email protected] with your story.LinksFelderaGitHubDBSP paperRust CrateDifferential DataflowTrinoFlinkSparkMaterializeClickhousePodcast EpisodeDuckDBPodcast EpisodeSnowflakeArrowSubstraitDataFusionDSP == Digital Signal ProcessingCDC == Change Data CapturePRQLLSM (Log-Structured Merge) TreeIcebergPodcast EpisodeDelta LakePodcast EpisodeOpen VSwitchFeature EngineeringCalciteThe intro and outro music is from The Hug by The Freak Fandango Orchestra / CC BY-SA
47:3604/11/2024
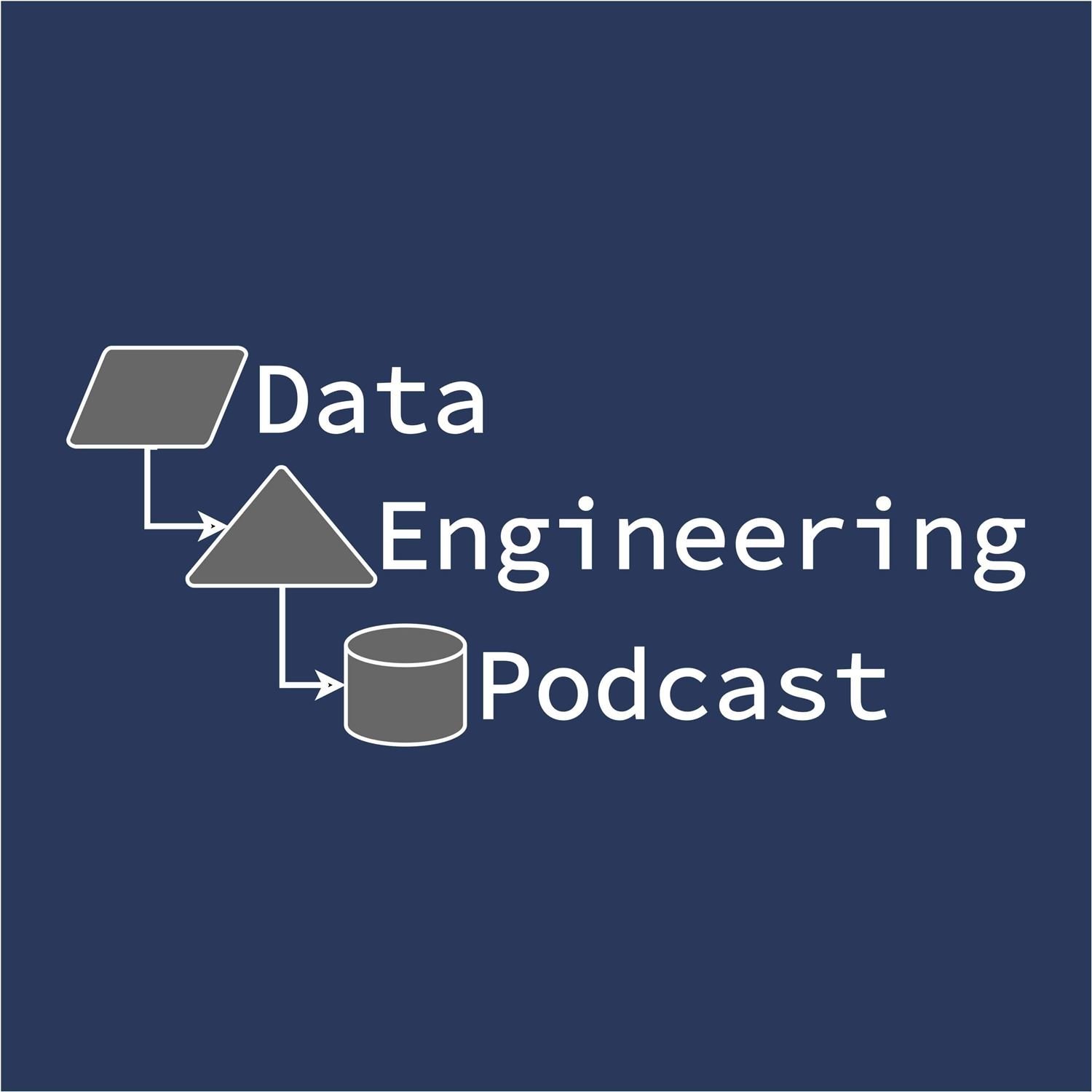
Accelerate Migration Of Your Data Warehouse with Datafold's AI Powered Migration Agent
SummaryGleb Mezhanskiy, CEO and co-founder of DataFold, joins Tobias Macey to discuss the challenges and innovations in data migrations. Gleb shares his experiences building and scaling data platforms at companies like Autodesk and Lyft, and how these experiences inspired the creation of DataFold to address data quality issues across teams. He outlines the complexities of data migrations, including common pitfalls such as technical debt and the importance of achieving parity between old and new systems. Gleb also discusses DataFold's innovative use of AI and large language models (LLMs) to automate translation and reconciliation processes in data migrations, reducing time and effort required for migrations.AnnouncementsHello and welcome to the Data Engineering Podcast, the show about modern data managementImagine catching data issues before they snowball into bigger problems. That’s what Datafold’s new Monitors do. With automatic monitoring for cross-database data diffs, schema changes, key metrics, and custom data tests, you can catch discrepancies and anomalies in real time, right at the source. Whether it’s maintaining data integrity or preventing costly mistakes, Datafold Monitors give you the visibility and control you need to keep your entire data stack running smoothly. Want to stop issues before they hit production? Learn more at dataengineeringpodcast.com/datafold today!Your host is Tobias Macey and today I'm welcoming back Gleb Mezhanskiy to talk about Datafold's experience bringing AI to bear on the problem of migrating your data stackInterviewIntroductionHow did you get involved in the area of data management?Can you describe what the Data Migration Agent is and the story behind it?What is the core problem that you are targeting with the agent?What are the biggest time sinks in the process of database and tooling migration that teams run into?Can you describe the architecture of your agent?What was your selection and evaluation process for the LLM that you are using?What were some of the main unknowns that you had to discover going into the project?What are some of the evolutions in the ecosystem that occurred either during the development process or since your initial launch that have caused you to second-guess elements of the design?In terms of SQL translation there are libraries such as SQLGlot and the work being done with SDF that aim to address that through AST parsing and subsequent dialect generation. What are the ways that approach is insufficient in the context of a platform migration?How does the approach you are taking with the combination of data-diffing and automated translation help build confidence in the migration target?What are the most interesting, innovative, or unexpected ways that you have seen the Data Migration Agent used?What are the most interesting, unexpected, or challenging lessons that you have learned while working on building an AI powered migration assistant?When is the data migration agent the wrong choice?What do you have planned for the future of applications of AI at Datafold?Contact InfoLinkedInParting QuestionFrom your perspective, what is the biggest gap in the tooling or technology for data management today?Closing AnnouncementsThank you for listening! Don't forget to check out our other shows. Podcast.__init__ covers the Python language, its community, and the innovative ways it is being used. The AI Engineering Podcast is your guide to the fast-moving world of building AI systems.Visit the site to subscribe to the show, sign up for the mailing list, and read the show notes.If you've learned something or tried out a project from the show then tell us about it! Email [email protected] with your story.LinksDatafoldDatafold Migration AgentDatafold data-diffDatafold Reconciliation Podcast EpisodeSQLGlotLark parserClaude 3.5 SonnetLookerPodcast EpisodeThe intro and outro music is from The Hug by The Freak Fandango Orchestra / CC BY-SA
48:5027/10/2024
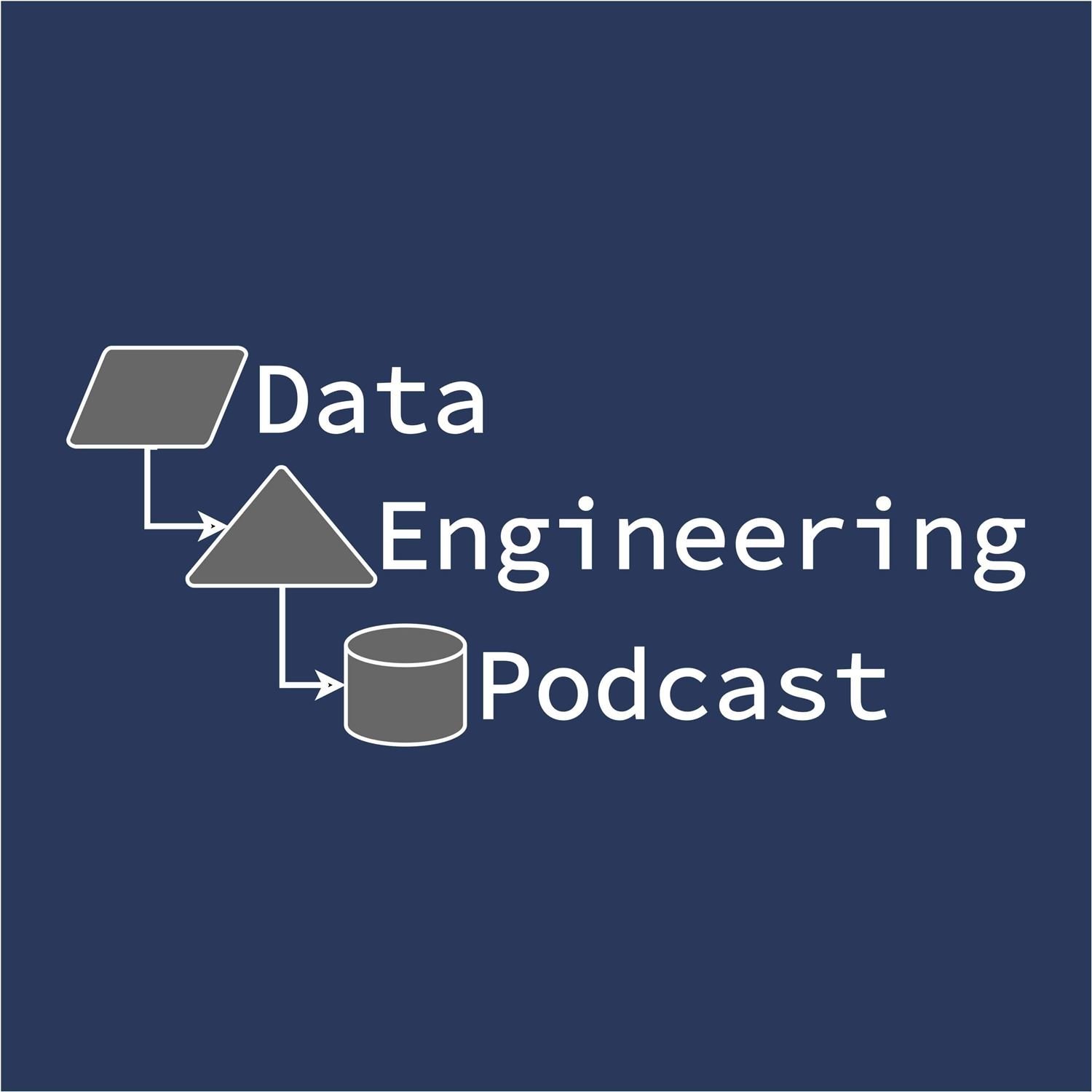
Bring Vector Search And Storage To The Data Lake With Lance
SummaryThe rapid growth of generative AI applications has prompted a surge of investment in vector databases. While there are numerous engines available now, Lance is designed to integrate with data lake and lakehouse architectures. In this episode Weston Pace explains the inner workings of the Lance format for table definitions and file storage, and the optimizations that they have made to allow for fast random access and efficient schema evolution. In addition to integrating well with data lakes, Lance is also a first-class participant in the Arrow ecosystem, making it easy to use with your existing ML and AI toolchains. This is a fascinating conversation about a technology that is focused on expanding the range of options for working with vector data.AnnouncementsHello and welcome to the Data Engineering Podcast, the show about modern data managementImagine catching data issues before they snowball into bigger problems. That’s what Datafold’s new Monitors do. With automatic monitoring for cross-database data diffs, schema changes, key metrics, and custom data tests, you can catch discrepancies and anomalies in real time, right at the source. Whether it’s maintaining data integrity or preventing costly mistakes, Datafold Monitors give you the visibility and control you need to keep your entire data stack running smoothly. Want to stop issues before they hit production? Learn more at dataengineeringpodcast.com/datafold today!Your host is Tobias Macey and today I'm interviewing Weston Pace about the Lance file and table format for column-oriented vector storageInterviewIntroductionHow did you get involved in the area of data management?Can you describe what Lance is and the story behind it?What are the core problems that Lance is designed to solve?What is explicitly out of scope?The README mentions that it is straightforward to convert to Lance from Parquet. What is the motivation for this compatibility/conversion support?What formats does Lance replace or obviate?In terms of data modeling Lance obviously adds a vector type, what are the features and constraints that engineers should be aware of when modeling their embeddings or arbitrary vectors?Are there any practical or hard limitations on vector dimensionality?When generating Lance files/datasets, what are some considerations to be aware of for balancing file/chunk sizes for I/O efficiency and random access in cloud storage?I noticed that the file specification has space for feature flags. How has that aided in enabling experimentation in new capabilities and optimizations?What are some of the engineering and design decisions that were most challenging and/or had the biggest impact on the performance and utility of Lance?The most obvious interface for reading and writing Lance files is through LanceDB. Can you describe the use cases that it focuses on and its notable features?What are the other main integrations for Lance?What are the opportunities or roadblocks in adding support for Lance and vector storage/indexes in e.g. Iceberg or Delta to enable its use in data lake environments?What are the most interesting, innovative, or unexpected ways that you have seen Lance used?What are the most interesting, unexpected, or challenging lessons that you have learned while working on the Lance format?When is Lance the wrong choice?What do you have planned for the future of Lance?Contact InfoLinkedInGitHubParting QuestionFrom your perspective, what is the biggest gap in the tooling or technology for data management today?LinksLance FormatLanceDBSubstraitPyArrowFAISSPineconePodcast EpisodeParquetIcebergPodcast EpisodeDelta LakePodcast EpisodePyLanceHilbert CurvesSIFT VectorsS3 ExpressWekaDataFusionRay DataTorch Data LoaderHNSW == Hierarchical Navigable Small Worlds vector indexIVFPQ vector indexGeoJSONPolarsThe intro and outro music is from The Hug by The Freak Fandango Orchestra / CC BY-SA
58:0120/10/2024
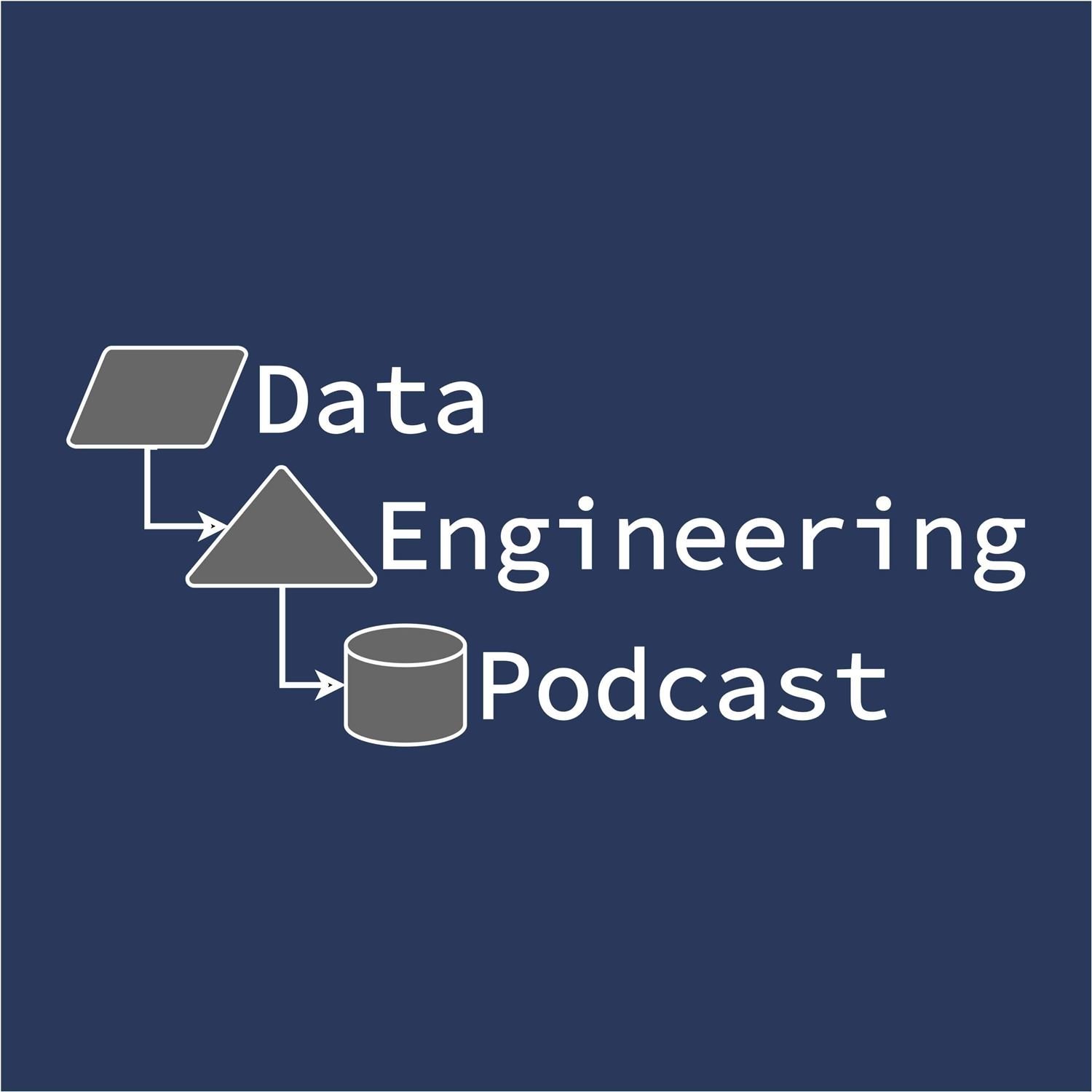
The Role of Python in Shaping the Future of Data Platforms with DLT
SummaryIn this episode of the Data Engineering Podcast, Adrian Broderieux and Marcin Rudolph, co-founders of DLT Hub, delve into the principles guiding DLT's development, emphasizing its role as a library rather than a platform, and its integration with lakehouse architectures and AI application frameworks. The episode explores the impact of the Python ecosystem's growth on DLT, highlighting integrations with high-performance libraries and the benefits of Arrow and DuckDB. The episode concludes with a discussion on the future of DLT, including plans for a portable data lake and the importance of interoperability in data management tools.AnnouncementsHello and welcome to the Data Engineering Podcast, the show about modern data managementImagine catching data issues before they snowball into bigger problems. That’s what Datafold’s new Monitors do. With automatic monitoring for cross-database data diffs, schema changes, key metrics, and custom data tests, you can catch discrepancies and anomalies in real time, right at the source. Whether it’s maintaining data integrity or preventing costly mistakes, Datafold Monitors give you the visibility and control you need to keep your entire data stack running smoothly. Want to stop issues before they hit production? Learn more at dataengineeringpodcast.com/datafold today!Your host is Tobias Macey and today I'm interviewing Adrian Brudaru and Marcin Rudolf, cofounders at dltHub, about the growth of dlt and the numerous ways that you can use it to address the complexities of data integrationInterviewIntroductionHow did you get involved in the area of data management?Can you describe what dlt is and how it has evolved since we last spoke (September 2023)?What are the core principles that guide your work on dlt and dlthub?You have taken a very opinionated stance against managed extract/load services. What are the shortcomings of those platforms, and when would you argue in their favor?The landscape of data movement has undergone some interesting changes over the past year. Most notably, the growth of PyAirbyte and the rapid shifts around the needs of generative AI stacks (vector stores, unstructured data processing, etc.). How has that informed your product development and positioning?The Python ecosystem, and in particular data-oriented Python, has also undergone substantial evolution. What are the developments in the libraries and frameworks that you have been able to benefit from?What are some of the notable investments that you have made in the developer experience for building dlt pipelines?How have the interfaces for source/destination development improved?You recently published a post about the idea of a portable data lake. What are the missing pieces that would make that possible, and what are the developments/technologies that put that idea within reach?What is your strategy for building a sustainable product on top of dlt?How does that strategy help to form a "virtuous cycle" of improving the open source foundation?What are the most interesting, innovative, or unexpected ways that you have seen dlt used?What are the most interesting, unexpected, or challenging lessons that you have learned while working on dlt?When is dlt the wrong choice?What do you have planned for the future of dlt/dlthub?Contact InfoAdrianLinkedInMarcinLinkedInParting QuestionFrom your perspective, what is the biggest gap in the tooling or technology for data management today?Closing AnnouncementsThank you for listening! Don't forget to check out our other shows. Podcast.__init__ covers the Python language, its community, and the innovative ways it is being used. The AI Engineering Podcast is your guide to the fast-moving world of building AI systems.Visit the site to subscribe to the show, sign up for the mailing list, and read the show notes.If you've learned something or tried out a project from the show then tell us about it! Email [email protected] with your story.LinksdltPodcast EpisodePyArrowPolarsIbisDuckDBPodcast Episodedlt Data ContractsRAG == Retrieval Augmented GenerationAI Engineering Podcast EpisodePyAirbyteOpenAI o1 ModelLanceDBQDrant EmbeddedAirflowGitHub ActionsArrow DataFusionApache ArrowPyIcebergDelta-RSSCD2 == Slowly Changing DimensionsSQLAlchemySQLGlotFSSpecPydanticSpacyEntity RecognitionParquet File FormatPython DecoratorREST API ToolkitOpenAPI Connector GeneratorConnectorXPython no-GILDelta LakePodcast EpisodeSQLMeshPodcast EpisodeHamiltonTabularPostHogPodcast.__init__ EpisodeAsyncIOCursor.AIData MeshPodcast EpisodeFastAPILangChainGraphRAGAI Engineering Podcast EpisodeProperty GraphPython uvThe intro and outro music is from The Hug by The Freak Fandango Orchestra / CC BY-SA
54:0813/10/2024
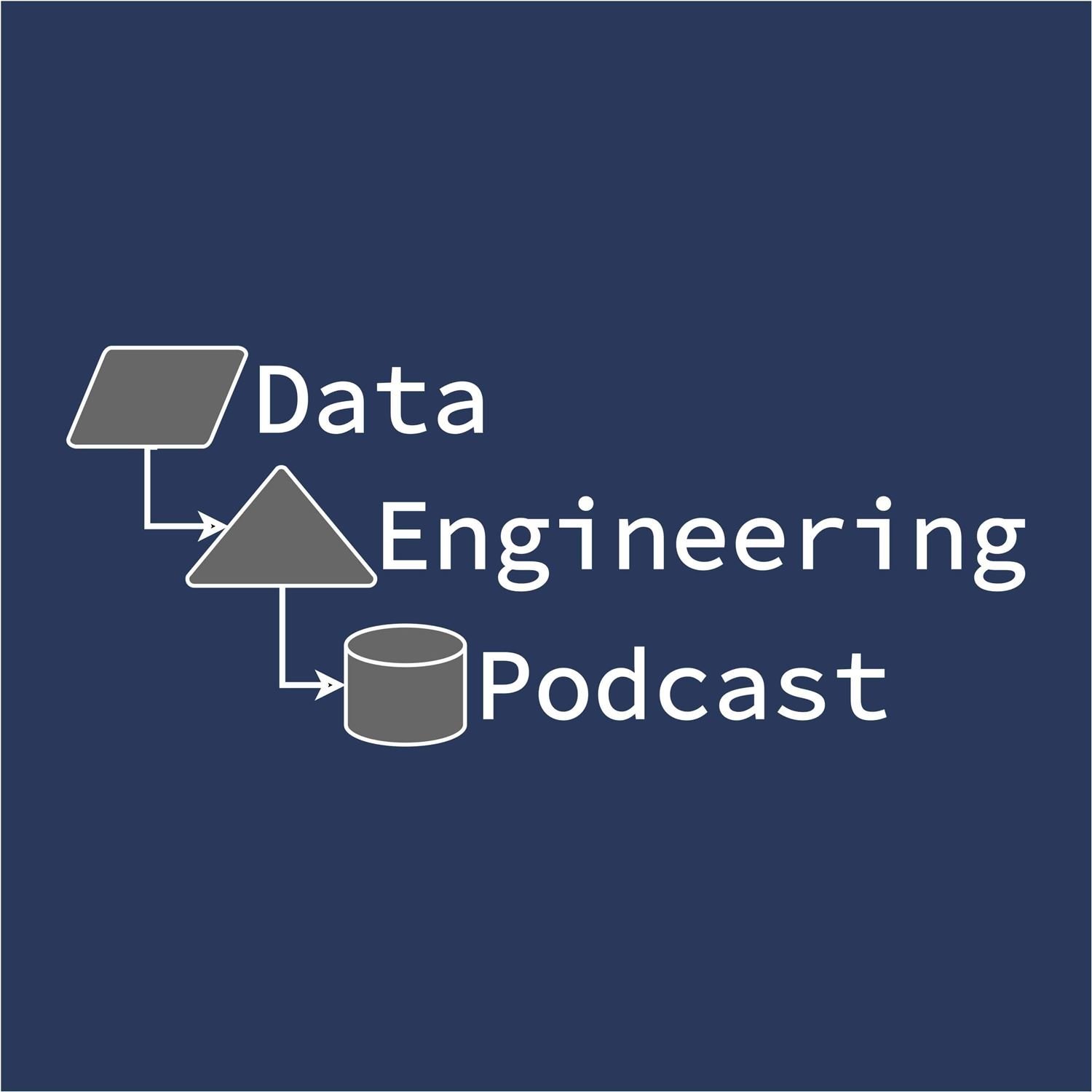
Build Your Data Transformations Faster And Safer With SDF
SummaryIn this episode of the Data Engineering Podcast Lukas Schulte, co-founder and CEO of SDF, explores the development and capabilities of this fast and expressive SQL transformation tool. From its origins as a solution for addressing data privacy, governance, and quality concerns in modern data management, to its unique features like static analysis and type correctness, Lucas dives into what sets SDF apart from other tools like DBT and SQL Mesh. Tune in for insights on building a business around a developer tool, the importance of community and user experience in the data engineering ecosystem, and plans for future development, including supporting Python models and enhancing execution capabilities.AnnouncementsHello and welcome to the Data Engineering Podcast, the show about modern data managementImagine catching data issues before they snowball into bigger problems. That’s what Datafold’s new Monitors do. With automatic monitoring for cross-database data diffs, schema changes, key metrics, and custom data tests, you can catch discrepancies and anomalies in real time, right at the source. Whether it’s maintaining data integrity or preventing costly mistakes, Datafold Monitors give you the visibility and control you need to keep your entire data stack running smoothly. Want to stop issues before they hit production? Learn more at dataengineeringpodcast.com/datafold today!Your host is Tobias Macey and today I'm interviewing Lukas Schulte about SDF, a fast and expressive SQL transformation tool that understands your schemaInterviewIntroductionHow did you get involved in the area of data management?Can you describe what SDF is and the story behind it?What's the story behind the name?What problem are you solving with SDF?dbt has been the dominant player for SQL-based transformations for several years, with other notable competition in the form of SQLMesh. Can you give an overview of the venn diagram for features and functionality across SDF, dbt and SQLMesh?Can you describe the design and implementation of SDF?How have the scope and goals of the project changed since you first started working on it?What does the development experience look like for a team working with SDF?How does that differ between the open and paid versions of the product?What are the features and functionality that SDF offers to address intra- and inter-team collaboration?One of the challenges for any second-mover technology with an established competitor is the adoption/migration path for teams who have already invested in the incumbent (dbt in this case). How are you addressing that barrier for SDF?Beyond the core migration path of the direct functionality of the incumbent product is the amount of tooling and communal knowledge that grows up around that product. How are you thinking about that aspect of the current landscape?What is your governing principle for what capabilities are in the open core and which go in the paid product?What are the most interesting, innovative, or unexpected ways that you have seen SDF used?What are the most interesting, unexpected, or challenging lessons that you have learned while working on SDF?When is SDF the wrong choice?What do you have planned for the future of SDF?Contact InfoLinkedInParting QuestionFrom your perspective, what is the biggest gap in the tooling or technology for data management today?LinksSDFSemantic Data Warehouseasdf-vmdbtSoftware Linting)SQLMeshPodcast EpisodeCoalescePodcast EpisodeApache IcebergPodcast EpisodeDuckDB Podcast Episode SDF Classifiersdbt Semantic Layerdbt expectationsApache DatafusionIbisThe intro and outro music is from The Hug by The Freak Fandango Orchestra / CC BY-SA
42:3606/10/2024
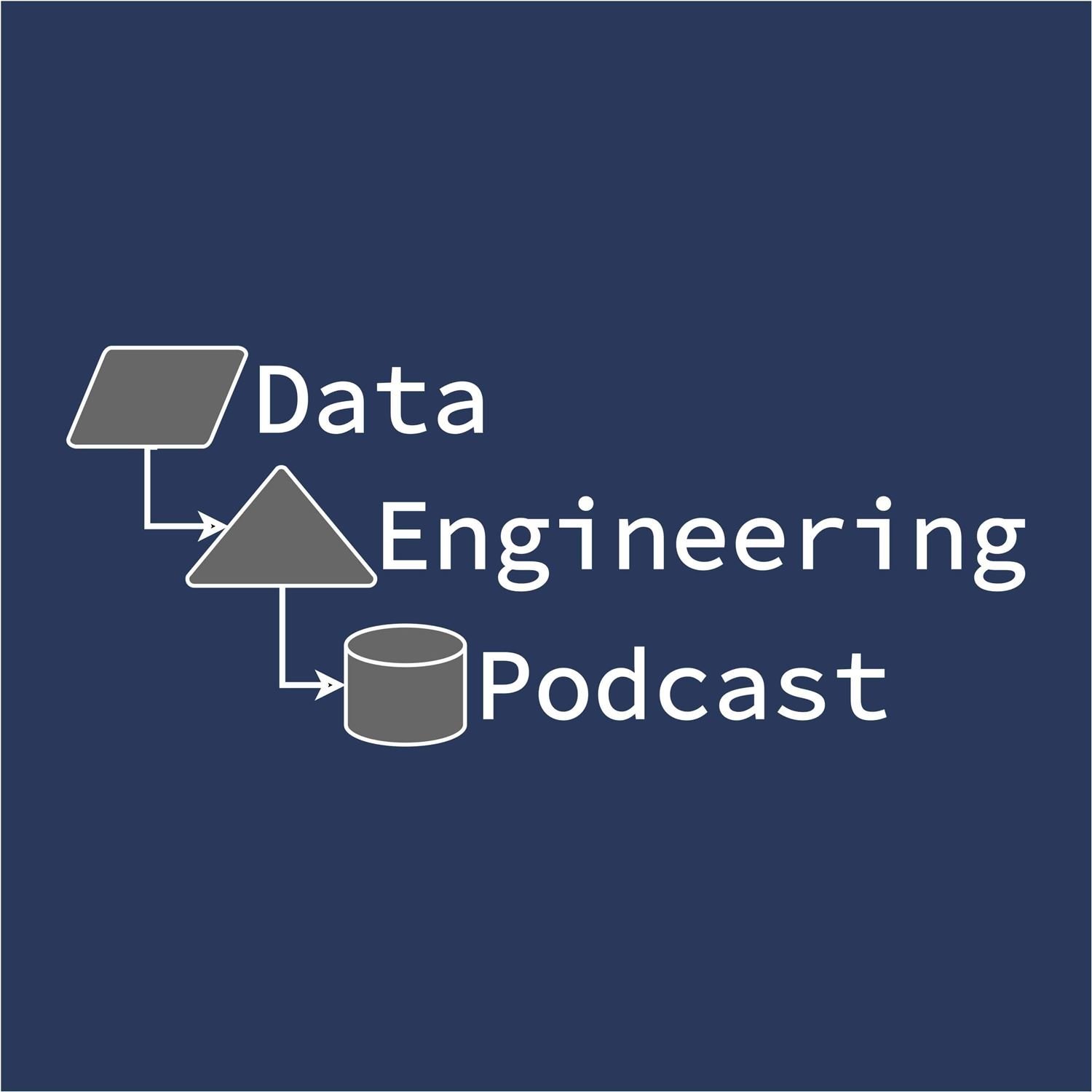
Scaling Airbyte: Challenges and Milestones on the Road to 1.0
SummaryAirbyte is one of the most prominent platforms for data movement. Over the past 4 years they have invested heavily in solutions for scaling the self-hosted and cloud operations, as well as the quality and stability of their connectors. As a result of that hard work, they have declared their commitment to the future of the platform with a 1.0 release. In this episode Michel Tricot shares the highlights of their journey and the exciting new capabilities that are coming next.AnnouncementsHello and welcome to the Data Engineering Podcast, the show about modern data managementYour host is Tobias Macey and today I'm interviewing Michel Tricot about the journey to the 1.0 launch of Airbyte and what that means for the projectInterviewIntroductionHow did you get involved in the area of data management?Can you describe what Airbyte is and the story behind it?What are some of the notable milestones that you have traversed on your path to the 1.0 release?The ecosystem has gone through some significant shifts since you first launched Airbyte. How have trends such as generative AI, the rise and fall of the "modern data stack", and the shifts in investment impacted your overall product and business strategies?What are some of the hard-won lessons that you have learned about the realities of data movement and integration?What are some of the most interesting/challenging/surprising edge cases or performance bottlenecks that you have had to address?What are the core architectural decisions that have proven to be effective?How has the architecture had to change as you progressed to the 1.0 release?A 1.0 version signals a degree of stability and commitment. Can you describe the decision process that you went through in committing to a 1.0 version?What are the most interesting, innovative, or unexpected ways that you have seen Airbyte used?What are the most interesting, unexpected, or challenging lessons that you have learned while working on Airbyte?When is Airbyte the wrong choice?What do you have planned for the future of Airbyte after the 1.0 launch?Contact InfoLinkedInParting QuestionFrom your perspective, what is the biggest gap in the tooling or technology for data management today?Closing AnnouncementsThank you for listening! Don't forget to check out our other shows. Podcast.__init__ covers the Python language, its community, and the innovative ways it is being used. The AI Engineering Podcast is your guide to the fast-moving world of building AI systems.Visit the site to subscribe to the show, sign up for the mailing list, and read the show notes.If you've learned something or tried out a project from the show then tell us about it! Email [email protected] with your story.LinksAirbytePodcast EpisodeAirbyte CloudAirbyte Connector BuilderSinger ProtocolAirbyte ProtocolAirbyte CDKModern Data StackELTVector DatabasedbtFivetranPodcast EpisodeMeltanoPodcast EpisodedltReverse ETLGraphRAGAI Engineering Podcast EpisodeThe intro and outro music is from The Hug by The Freak Fandango Orchestra / CC BY-SA
57:1123/09/2024
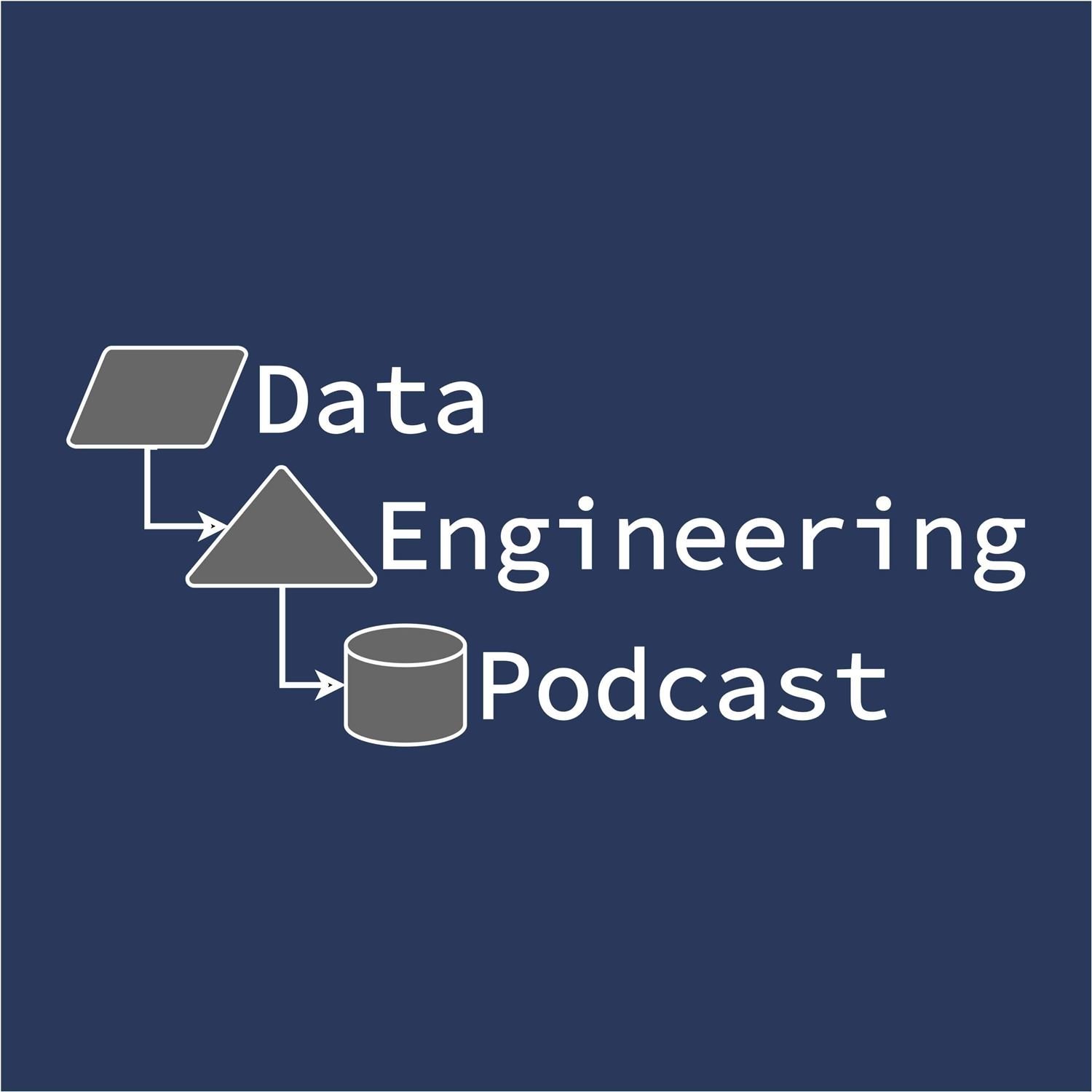
Enhancing Data Accessibility and Governance with Gravitino
SummaryAs data architectures become more elaborate and the number of applications of data increases, it becomes increasingly challenging to locate and access the underlying data. Gravitino was created to provide a single interface to locate and query your data. In this episode Junping Du explains how Gravitino works, the capabilities that it unlocks, and how it fits into your data platform.AnnouncementsHello and welcome to the Data Engineering Podcast, the show about modern data managementYour host is Tobias Macey and today I'm interviewing Junping Du about Gravitino, an open source metadata service for a unified view of all of your schemasInterviewIntroductionHow did you get involved in the area of data management?Can you describe what Gravitino is and the story behind it?What problems are you solving with Gravitino?What are the methods that teams have relied on in the absence of Gravitino to address those use cases?What led to the Hive Metastore being the default for so long?What are the opportunities for innovation and new functionality in the metadata service?The documentation suggests that Gravitino has overlap with a number of tool categories such as table schema (Hive metastore), metadata repository (Open Metadata), data federation (Trino/Alluxio). What are the capabilities that it can completely replace, and which will require other systems for more comprehensive functionality?What are the capabilities that you are explicitly keeping out of scope for Gravitino?Can you describe the technical architecture of Gravitino?How have the design and scope evolved from when you first started working on it?Can you describe how Gravitino integrates into an overall data platform?In a typical day, what are the different ways that a data engineer or data analyst might interact with Gravitino?One of the features that you highlight is centralized permissions management. Can you describe the access control model that you use for unifying across underlying sources?What are the most interesting, innovative, or unexpected ways that you have seen Gravitino used?What are the most interesting, unexpected, or challenging lessons that you have learned while working on Gravitino?When is Gravitino the wrong choice?What do you have planned for the future of Gravitino?Contact InfoLinkedInGitHubParting QuestionFrom your perspective, what is the biggest gap in the tooling or technology for data management today?Closing AnnouncementsThank you for listening! Don't forget to check out our other shows. Podcast.__init__ covers the Python language, its community, and the innovative ways it is being used. The AI Engineering Podcast is your guide to the fast-moving world of building AI systems.Visit the site to subscribe to the show, sign up for the mailing list, and read the show notes.If you've learned something or tried out a project from the show then tell us about it! Email [email protected] with your story.LinksGravitinoHadoopDatastratoPyTorchRayData FabricHiveIcebergPodcast EpisodeHive MetastoreTrinoOpenMetadataPodcast EpisodeAlluxioAtlanPodcast EpisodeSparkThriftThe intro and outro music is from The Hug by The Freak Fandango Orchestra / CC BY-SA
38:4101/09/2024
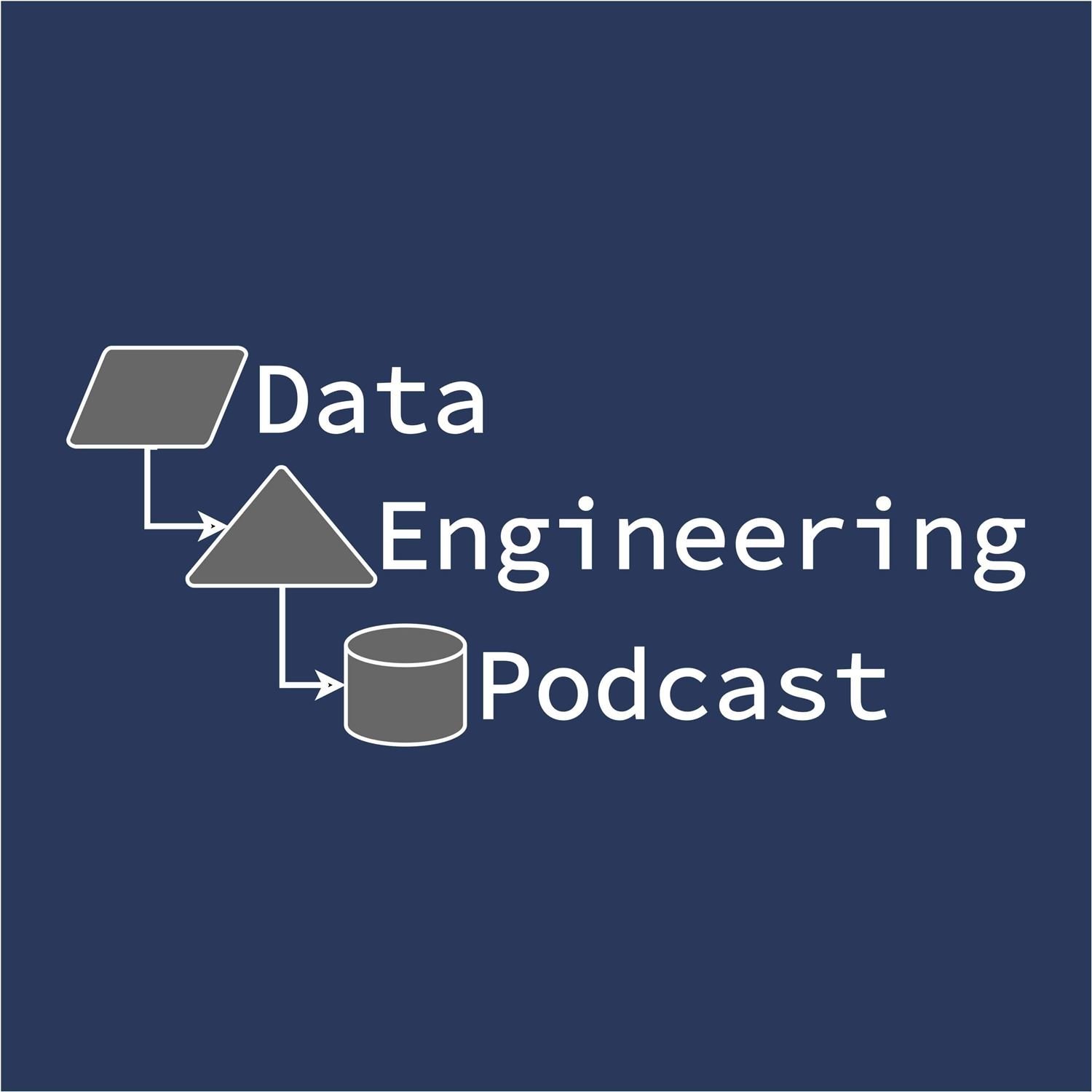
The Evolution of DataOps: Insights from DataKitchen's CEO
SummaryIn this episode of the Data Engineering Podcast, host Tobias Macey welcomes back Chris Berg, CEO of DataKitchen, to discuss his ongoing mission to simplify the lives of data engineers. Chris explains the challenges faced by data engineers, such as constant system failures, the need for rapid changes, and high customer demands. Chris delves into the concept of DataOps, its evolution, and the misappropriation of related terms like data mesh and data observability. He emphasizes the importance of focusing on processes and systems rather than just tools to improve data engineering workflows. Chris also introduces DataKitchen's open-source tools, DataOps TestGen and DataOps Observability, designed to automate data quality validation and monitor data journeys in production.AnnouncementsHello and welcome to the Data Engineering Podcast, the show about modern data managementData lakes are notoriously complex. For data engineers who battle to build and scale high quality data workflows on the data lake, Starburst is an end-to-end data lakehouse platform built on Trino, the query engine Apache Iceberg was designed for, with complete support for all table formats including Apache Iceberg, Hive, and Delta Lake. Trusted by teams of all sizes, including Comcast and Doordash. Want to see Starburst in action? Go to dataengineeringpodcast.com/starburst and get $500 in credits to try Starburst Galaxy today, the easiest and fastest way to get started using Trino.Your host is Tobias Macey and today I'm interviewing Chris Bergh about his tireless quest to simplify the lives of data engineersInterviewIntroductionHow did you get involved in the area of data management?Can you describe what DataKitchen is and the story behind it?You helped to define and popularize "DataOps", which then went through a journey of misappropriation similar to "DevOps", and has since faded in use. What is your view on the realities of "DataOps" today?Out of the popularized wave of "DataOps" tools came subsequent trends in data observability, data reliability engineering, etc. How have those cycles influenced the way that you think about the work that you are doing at DataKitchen?The data ecosystem went through a massive growth period over the past ~7 years, and we are now entering a cycle of consolidation. What are the fundamental shifts that we have gone through as an industry in the management and application of data?What are the challenges that never went away?You recently open sourced the dataops-testgen and dataops-observability tools. What are the outcomes that you are trying to produce with those projects?What are the areas of overlap with existing tools and what are the unique capabilities that you are offering?Can you talk through the technical implementation of your new obserability and quality testing platform?What does the onboarding and integration process look like?Once a team has one or both tools set up, what are the typical points of interaction that they will have over the course of their workday?What are the most interesting, innovative, or unexpected ways that you have seen dataops-observability/testgen used?What are the most interesting, unexpected, or challenging lessons that you have learned while working on promoting DataOps?What do you have planned for the future of your work at DataKitchen?Contact InfoLinkedInParting QuestionFrom your perspective, what is the biggest gap in the tooling or technology for data management today?LinksDataKitchenPodcast EpisodeNASADataOps ManifestoData Reliability EngineeringData ObservabilitydbtDevOps Enterprise SummitBuilding The Data Warehouse by Bill Inmon (affiliate link)dataops-testgen, dataops-observabilityFree Data Quality and Data Observability CertificationDatabricksDORA MetricsDORA for dataThe intro and outro music is from The Hug by The Freak Fandango Orchestra / CC BY-SA
53:3004/08/2024
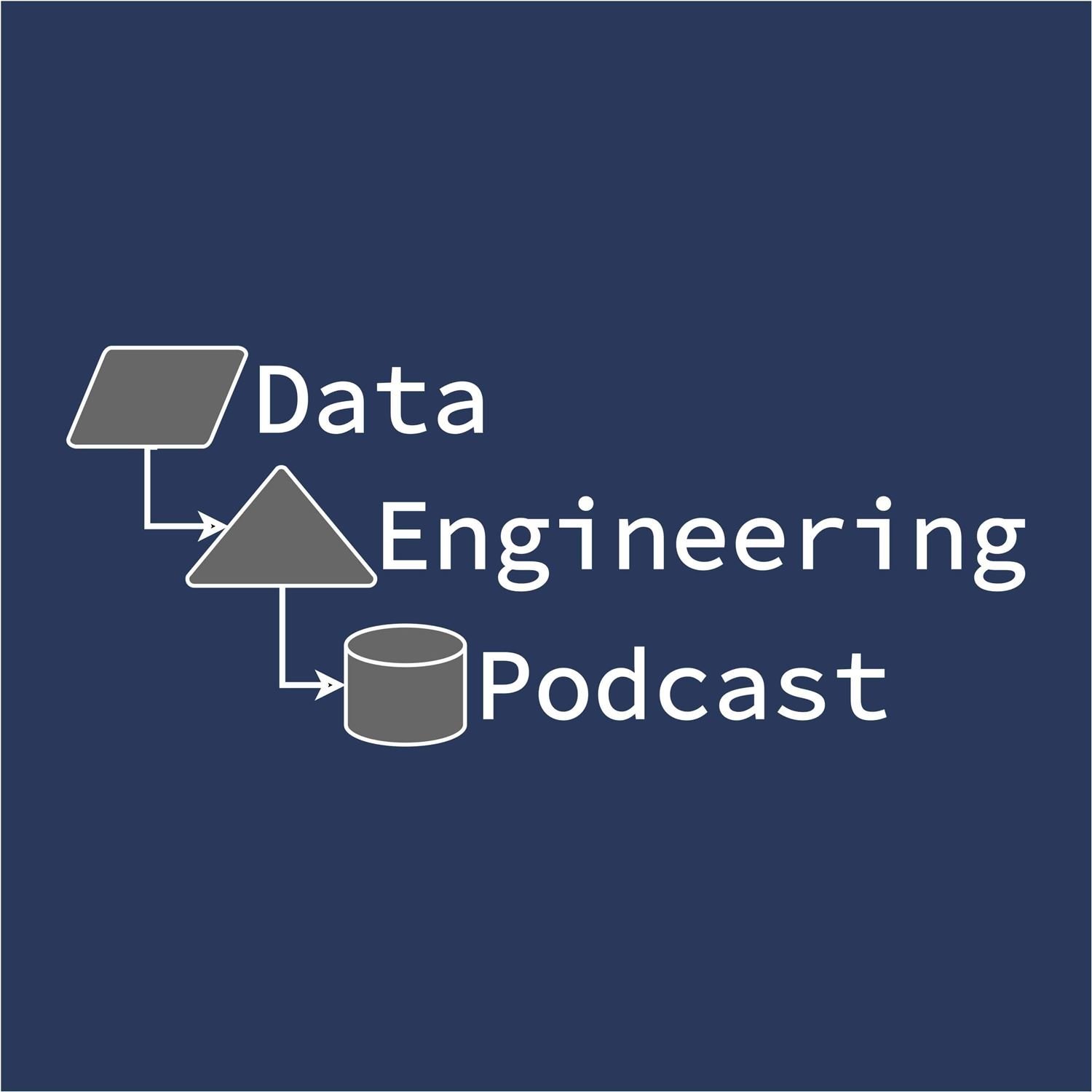
Achieving Data Reliability: The Role of Data Contracts in Modern Data Management
SummaryData contracts are both an enforcement mechanism for data quality, and a promise to downstream consumers. In this episode Tom Baeyens returns to discuss the purpose and scope of data contracts, emphasizing their importance in achieving reliable analytical data and preventing issues before they arise. He explains how data contracts can be used to enforce guarantees and requirements, and how they fit into the broader context of data observability and quality monitoring. The discussion also covers the challenges and benefits of implementing data contracts, the organizational impact, and the potential for standardization in the field.AnnouncementsHello and welcome to the Data Engineering Podcast, the show about modern data managementData lakes are notoriously complex. For data engineers who battle to build and scale high quality data workflows on the data lake, Starburst is an end-to-end data lakehouse platform built on Trino, the query engine Apache Iceberg was designed for, with complete support for all table formats including Apache Iceberg, Hive, and Delta Lake. Trusted by teams of all sizes, including Comcast and Doordash. Want to see Starburst in action? Go to dataengineeringpodcast.com/starburst and get $500 in credits to try Starburst Galaxy today, the easiest and fastest way to get started using Trino.At Outshift, the incubation engine from Cisco, they are driving innovation in AI, cloud, and quantum technologies with the powerful combination of enterprise strength and startup agility. Their latest innovation for the AI ecosystem is Motific, addressing a critical gap in going from prototype to production with generative AI. Motific is your vendor and model-agnostic platform for building safe, trustworthy, and cost-effective generative AI solutions in days instead of months. Motific provides easy integration with your organizational data, combined with advanced, customizable policy controls and observability to help ensure compliance throughout the entire process. Move beyond the constraints of traditional AI implementation and ensure your projects are launched quickly and with a firm foundation of trust and efficiency. Go to motific.ai today to learn more!Your host is Tobias Macey and today I'm interviewing Tom Baeyens about using data contracts to build a clearer API for your dataInterviewIntroductionHow did you get involved in the area of data management?Can you describe the scope and purpose of data contracts in the context of this conversation?In what way(s) do they differ from data quality/data observability?Data contracts are also known as the API for data, can you elaborate on this?What are the types of guarantees and requirements that you can enforce with these data contracts?What are some examples of constraints or guarantees that cannot be represented in these contracts?Are data contracts related to the shift-left?Data contracts are also known as the API for data, can you elaborate on this?The obvious application of data contracts are in the context of pipeline execution flows to prevent failing checks from propagating further in the data flow. What are some of the other ways that these contracts can be integrated into an organization's data ecosystem?How did you approach the design of the syntax and implementation for Soda's data contracts?Guarantees and constraints around data in different contexts have been implemented in numerous tools and systems. What are the areas of overlap in e.g. dbt, great expectations?Are there any emerging standards or design patterns around data contracts/guarantees that will help encourage portability and integration across tooling/platform contexts?What are the most interesting, innovative, or unexpected ways that you have seen data contracts used?What are the most interesting, unexpected, or challenging lessons that you have learned while working on data contracts at Soda?When are data contracts the wrong choice?What do you have planned for the future of data contracts?Contact InfoLinkedInParting QuestionFrom your perspective, what is the biggest gap in the tooling or technology for data management today?Closing AnnouncementsThank you for listening! Don't forget to check out our other shows. Podcast.__init__ covers the Python language, its community, and the innovative ways it is being used. The AI Engineering Podcast is your guide to the fast-moving world of building AI systems.Visit the site to subscribe to the show, sign up for the mailing list, and read the show notes.If you've learned something or tried out a project from the show then tell us about it! Email [email protected] with your story.LinksSodaPodcast EpisodeJBossData ContractAirflowUnit TestingIntegration TestingOpenAPIGraphQLCircuit Breaker PatternSodaCLSoda Data ContractsData MeshGreat Expectationsdbt Unit TestsOpen Data ContractsODCS == Open Data Contract StandardODPS == Open Data Product SpecificationThe intro and outro music is from The Hug by The Freak Fandango Orchestra / CC BY-SA
49:2628/07/2024
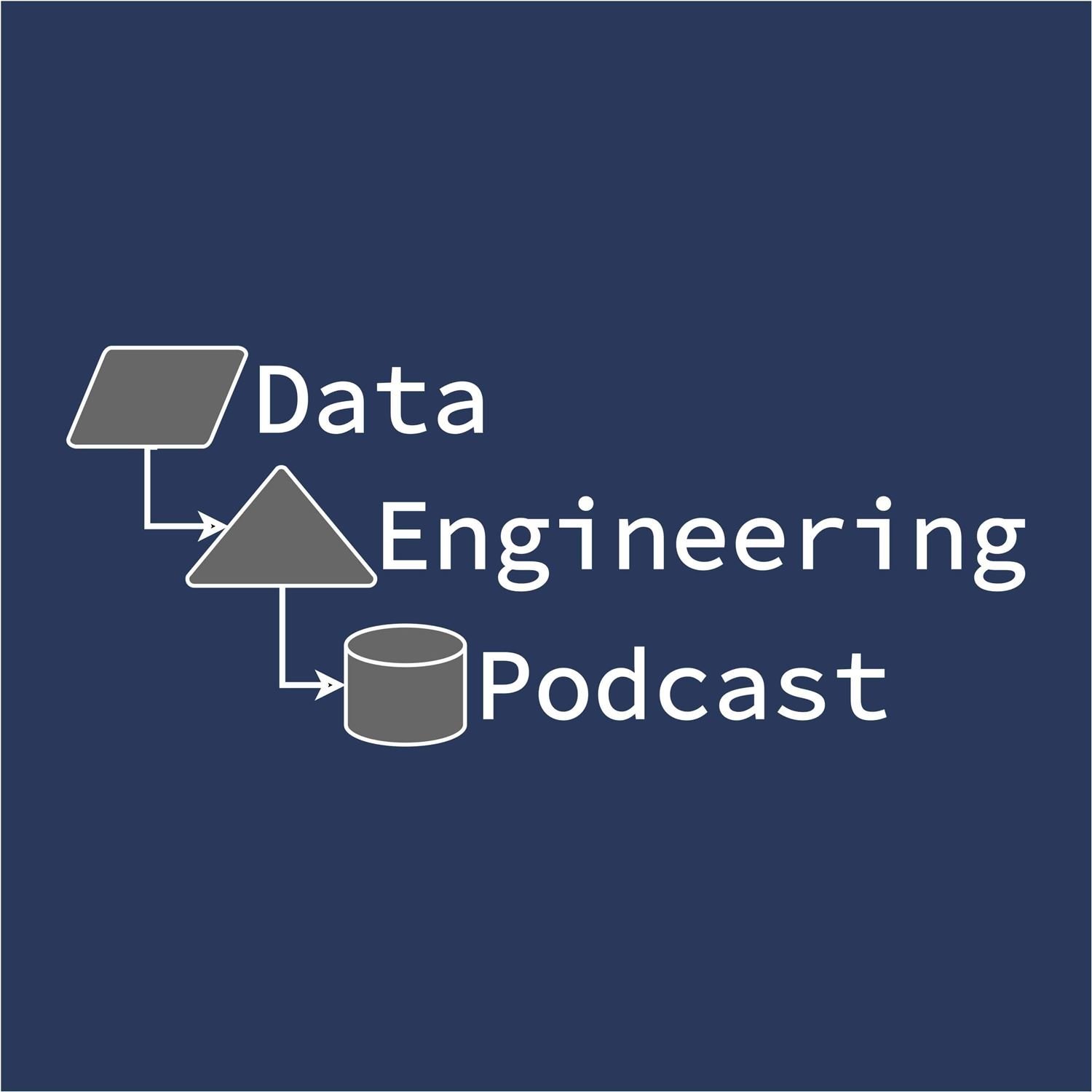
How Generative AI Is Impacting Data Engineering Teams
SummaryGenerative AI has rapidly gained adoption for numerous use cases. To support those applications, organizational data platforms need to add new features and data teams have increased responsibility. In this episode Lior Gavish, co-founder of Monte Carlo, discusses the various ways that data teams are evolving to support AI powered features and how they are incorporating AI into their work.AnnouncementsHello and welcome to the Data Engineering Podcast, the show about modern data managementData lakes are notoriously complex. For data engineers who battle to build and scale high quality data workflows on the data lake, Starburst is an end-to-end data lakehouse platform built on Trino, the query engine Apache Iceberg was designed for, with complete support for all table formats including Apache Iceberg, Hive, and Delta Lake. Trusted by teams of all sizes, including Comcast and Doordash. Want to see Starburst in action? Go to dataengineeringpodcast.com/starburst and get $500 in credits to try Starburst Galaxy today, the easiest and fastest way to get started using Trino.Your host is Tobias Macey and today I'm interviewing Lior Gavish about the impact of AI on data engineersInterviewIntroductionHow did you get involved in the area of data management?Can you start by clarifying what we are discussing when we say "AI"?Previous generations of machine learning (e.g. deep learning, reinforcement learning, etc.) required new features in the data platform. What new demands is the current generation of AI introducing?Generative AI also has the potential to be incorporated in the creation/execution of data pipelines. What are the risk/reward tradeoffs that you have seen in practice?What are the areas where LLMs have proven useful/effective in data engineering?Vector embeddings have rapidly become a ubiquitous data format as a result of the growth in retrieval augmented generation (RAG) for AI applications. What are the end-to-end operational requirements to support this use case effectively?As with all data, the reliability and quality of the vectors will impact the viability of the AI application. What are the different failure modes/quality metrics/error conditions that they are subject to?As much as vectors, vector databases, RAG, etc. seem exotic and new, it is all ultimately shades of the same work that we have been doing for years. What are the areas of overlap in the work required for running the current generation of AI, and what are the areas where it diverges?What new skills do data teams need to acquire to be effective in supporting AI applications?What are the most interesting, innovative, or unexpected ways that you have seen AI impact data engineering teams?What are the most interesting, unexpected, or challenging lessons that you have learned while working with the current generation of AI?When is AI the wrong choice?What are your predictions for the future impact of AI on data engineering teams?Contact InfoLinkedInParting QuestionFrom your perspective, what is the biggest gap in the tooling or technology for data management today?Closing AnnouncementsThank you for listening! Don't forget to check out our other shows. Podcast.__init__ covers the Python language, its community, and the innovative ways it is being used. The AI Engineering Podcast is your guide to the fast-moving world of building AI systems.Visit the site to subscribe to the show, sign up for the mailing list, and read the show notes.If you've learned something or tried out a project from the show then tell us about it! Email [email protected] with your LinksMonte CarloPodcast EpisodeNLP == Natural Language ProcessingLarge Language ModelsGenerative AIMLOpsML EngineerFeature StoreRetrieval Augmented Generation (RAG)LangchainThe intro and outro music is from The Hug by The Freak Fandango Orchestra / CC BY-SA
54:4521/07/2024
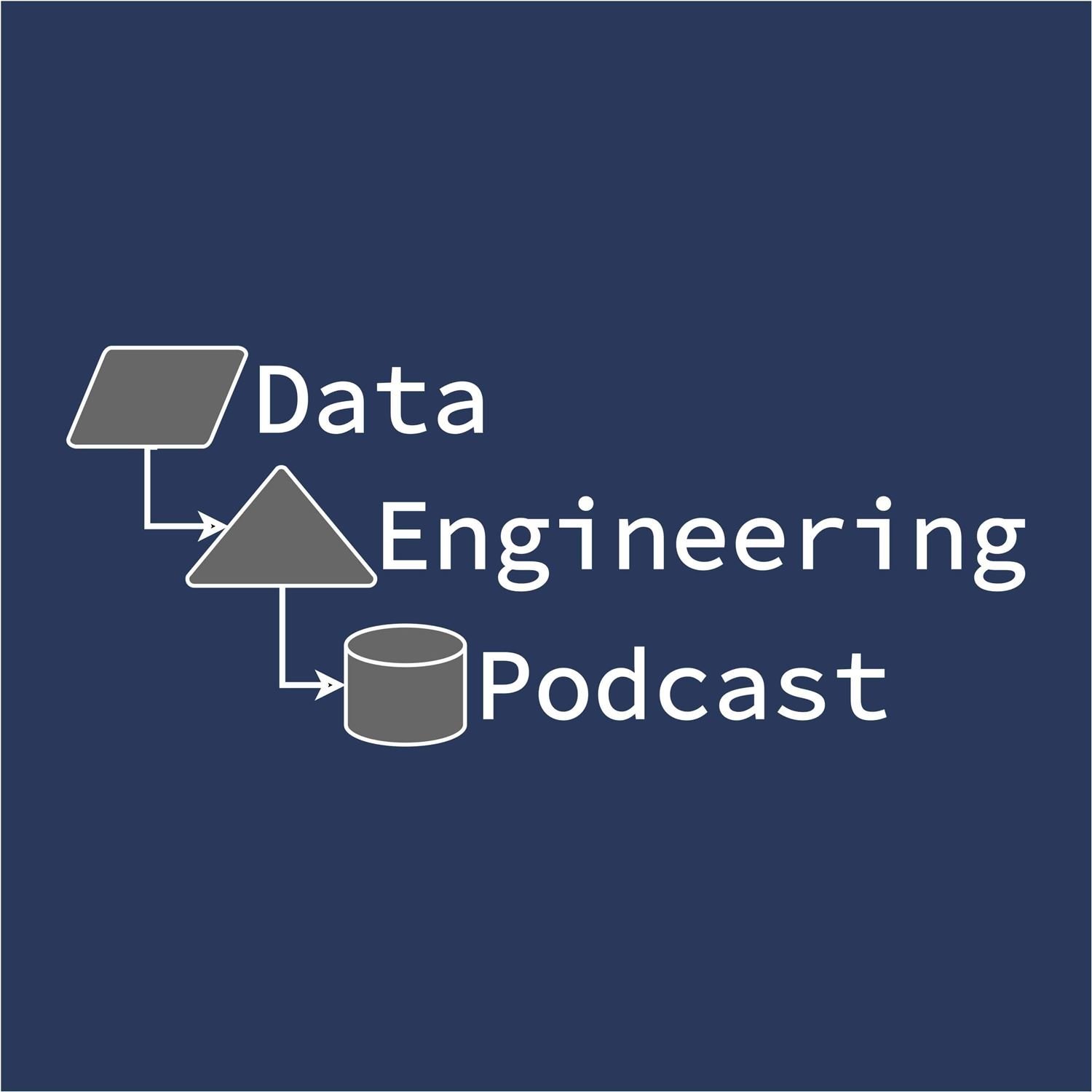
The Role of Product Managers in Data-Centric Organizations
SummaryIn this episode Praveen Gujar, Director of Product at LinkedIn, talks about the intricacies of product management for data and analytical platforms. Praveen shares his journey from Amazon to Twitter and now LinkedIn, highlighting his extensive experience in building data products and platforms, digital advertising, AI, and cloud services. He discusses the evolving role of product managers in data-centric environments, emphasizing the importance of clean, reliable, and compliant data. Praveen also delves into the challenges of building scalable data platforms, the need for organizational and cultural alignment, and the critical role of product managers in bridging the gap between engineering and business teams. He provides insights into the complexities of platformization, the significance of long-term planning, and the necessity of having a strong relationship with engineering teams. The episode concludes with Praveen offering advice for aspiring product managers and discussing the future of data management in the context of AI and regulatory compliance.AnnouncementsHello and welcome to the Data Engineering Podcast, the show about modern data managementData lakes are notoriously complex. For data engineers who battle to build and scale high quality data workflows on the data lake, Starburst is an end-to-end data lakehouse platform built on Trino, the query engine Apache Iceberg was designed for, with complete support for all table formats including Apache Iceberg, Hive, and Delta Lake. Trusted by teams of all sizes, including Comcast and Doordash. Want to see Starburst in action? Go to dataengineeringpodcast.com/starburst and get $500 in credits to try Starburst Galaxy today, the easiest and fastest way to get started using Trino.Your host is Tobias Macey and today I'm interviewing Praveen Gujar about product management for data and analytical platformsInterviewIntroductionHow did you get involved in the area of data management?Product management is typically thought of as being oriented toward customer facing functionality and features. What is involved in being a product manager for data systems?Many data-oriented products that are customer facing require substantial technical capacity to serve those use cases. How does that influence the process of determining what features to provide/create?investment in technical capacity/platformsidentifying groupings of features that can be served by a common platform investmentmanaging organizational pressures between engineering, product, business, finance, etc.What are the most interesting, innovative, or unexpected ways that you have seen "Data Products & Platforms @ Big-tech" used?What are the most interesting, unexpected, or challenging lessons that you have learned while working on "Building Data Products & Platforms for Big-tech"?When is "Data Products & Platforms @ Big-tech" the wrong choice?What do you have planned for the future of "Data Products & Platforms @ Big-tech"?Contact InfoLinkedInWebsiteParting QuestionFrom your perspective, what is the biggest gap in the tooling or technology for data management today?Closing AnnouncementsThank you for listening! Don't forget to check out our other shows. Podcast.__init__ covers the Python language, its community, and the innovative ways it is being used. The AI Engineering Podcast is your guide to the fast-moving world of building AI systems.Visit the site to subscribe to the show, sign up for the mailing list, and read the show notes.If you've learned something or tried out a project from the show then tell us about it! Email [email protected] with your story.LinksDataHubPodcast EpisodeRAG == Retrieval Augmented GenerationThe intro and outro music is from The Hug by The Freak Fandango Orchestra / CC BY-SA
52:5813/07/2024
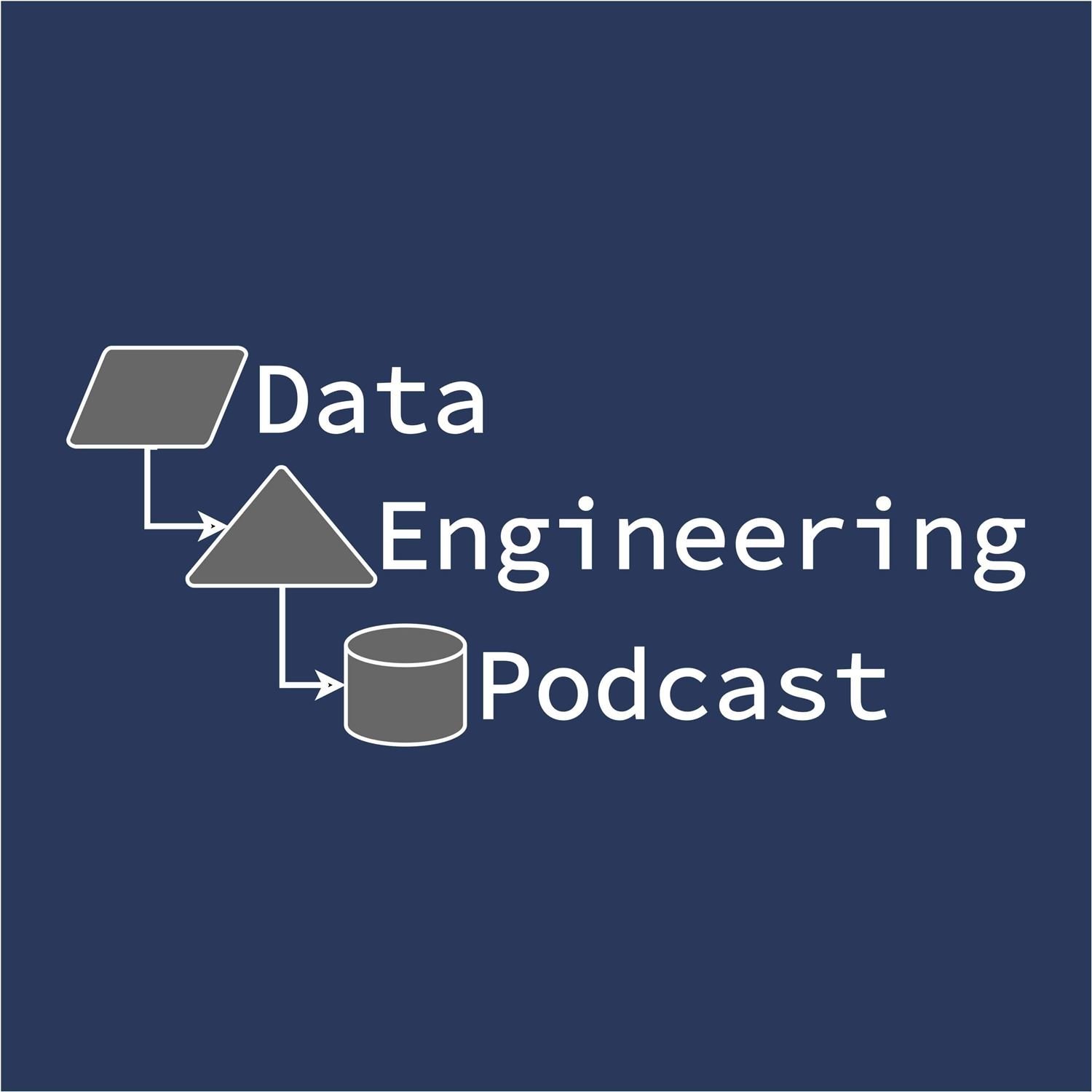
Neon: A Serverless And Developer Friendly Postgres
SummaryPostgres is one of the most widely respected and liked database engines ever. To make it even easier to use for developers to use, Nikita Shamgunov decided to makee it serverless, so that it can scale from zero to infinity. In this episode he explains the engineering involved to make that possible, as well as the numerous details that he and his team are packing into the Neon service to make it even more attractive for anyone who wants to build on top of Postgres.AnnouncementsHello and welcome to the Data Engineering Podcast, the show about modern data managementData lakes are notoriously complex. For data engineers who battle to build and scale high quality data workflows on the data lake, Starburst is an end-to-end data lakehouse platform built on Trino, the query engine Apache Iceberg was designed for, with complete support for all table formats including Apache Iceberg, Hive, and Delta Lake. Trusted by teams of all sizes, including Comcast and Doordash. Want to see Starburst in action? Go to dataengineeringpodcast.com/starburst and get $500 in credits to try Starburst Galaxy today, the easiest and fastest way to get started using Trino.Your host is Tobias Macey and today I'm interviewing Nikita Shamgunov about his work on making Postgres a serverless database at Neon.InterviewIntroductionHow did you get involved in the area of data management?Can you describe what Neon is and the story behind it?The ecosystem around Postgres is large and varied. What are the pain points that you are trying to address with Neon? What does it mean for a database to be serverless?What kinds of products and services are unlocked by making Postgres a serverless database?How does your vision for Neon compare/contrast with what you know of PlanetScale?Postgres is known for having a large ecosystem of plugins that add a lot of interesting and useful features, but the storage layer has not been as easily extensible historically. How have architectural changes in recent Postgres releases enabled your work on Neon?What are the core pieces of engineering that you have had to complete to make Neon possible?How have the design and goals of the project evolved since you first started working on it?The separation of storage and compute is one of the most fundamental promises of the cloud. What new capabilities does that enable in Postgres?How does the branching functionality change the ways that development teams are able to deliver and debug features?Because the storage is now a networked system, what new performance/latency challenges does that introduce? How have you addressed them in Neon?Anyone who has ever operated a Postgres instance has had to tackle the upgrade process. How does Neon address that process for end users?The rampant growth of AI has touched almost every aspect of computing, and Postgres is no exception. How does the introduction of pgvector and semantic/similarity search functionality impact the adoption and usage patterns of Postgres/Neon?What new challenges does that introduce for you as an operator and business owner?What are the lessons that you learned from MemSQL/SingleStore that have been most helpful in your work at Neon?What are the most interesting, innovative, or unexpected ways that you have seen Neon used?What are the most interesting, unexpected, or challenging lessons that you have learned while working on Neon?When is Neon the wrong choice? Postgres?What do you have planned for the future of Neon?Contact Info@nikitabase on TwitterLinkedInParting QuestionFrom your perspective, what is the biggest gap in the tooling or technology for data management today?Closing AnnouncementsThank you for listening! Don't forget to check out our other shows. Podcast.__init__ covers the Python language, its community, and the innovative ways it is being used. The Machine Learning Podcast helps you go from idea to production with machine learning.Visit the site to subscribe to the show, sign up for the mailing list, and read the show notes.If you've learned something or tried out a project from the show then tell us about it! Email [email protected] with your story.LinksNeonPostgreSQLNeon GithubPHPMySQLSQL ServerSingleStorePodcast EpisodeAWS AuroraKhosla VenturesYugabyteDBPodcast EpisodeCockroachDBPodcast EpisodePlanetScalePodcast EpisodeClickhousePodcast EpisodeDuckDBPodcast EpisodeWAL == Write-Ahead LogPgBouncerPureStoragePaxos)HNSW IndexIVF Flat IndexRAG == Retrieval Augmented GenerationAlloyDBNeon Serverless DriverDevinmagic.devThe intro and outro music is from The Hug by The Freak Fandango Orchestra / CC BY-SA
57:4308/07/2024
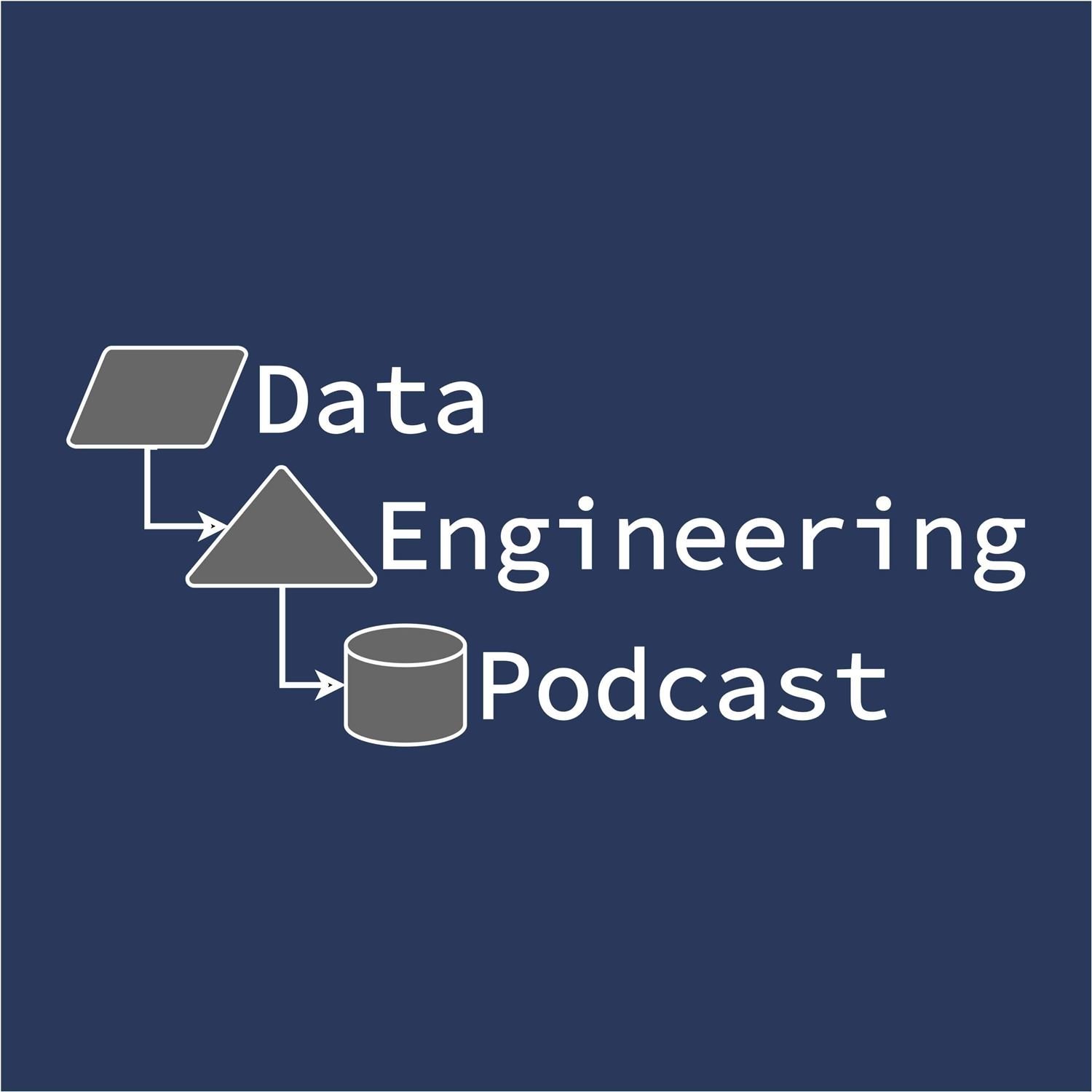
Improve Data Quality Through Engineering Rigor And Business Engagement With Synq
SummaryThis episode features an insightful conversation with Petr Janda, the CEO and founder of Synq. Petr shares his journey from being an engineer to founding Synq, emphasizing the importance of treating data systems with the same rigor as engineering systems. He discusses the challenges and solutions in data reliability, including the need for transparency and ownership in data systems. Synq's platform helps data teams manage incidents, understand data dependencies, and ensure data quality by providing insights and automation capabilities. Petr emphasizes the need for a holistic approach to data reliability, integrating data systems into broader business processes. He highlights the role of data teams in modern organizations and how Synq is empowering them to achieve this.AnnouncementsHello and welcome to the Data Engineering Podcast, the show about modern data managementData lakes are notoriously complex. For data engineers who battle to build and scale high quality data workflows on the data lake, Starburst is an end-to-end data lakehouse platform built on Trino, the query engine Apache Iceberg was designed for, with complete support for all table formats including Apache Iceberg, Hive, and Delta Lake. Trusted by teams of all sizes, including Comcast and Doordash. Want to see Starburst in action? Go to dataengineeringpodcast.com/starburst and get $500 in credits to try Starburst Galaxy today, the easiest and fastest way to get started using Trino.Your host is Tobias Macey and today I'm interviewing Petr Janda about Synq, a data reliability platform focused on leveling up data teams by supporting a culture of engineering rigorInterviewIntroductionHow did you get involved in the area of data management?Can you describe what Synq is and the story behind it? Data observability/reliability is a category that grew rapidly over the past ~5 years and has several vendors focused on different elements of the problem. What are the capabilities that you saw as lacking in the ecosystem which you are looking to address?Operational/infrastructure engineers have spent the past decade honing their approach to incident management and uptime commitments. How do those concepts map to the responsibilities and workflows of data teams? Tooling only plays a small part in SLAs and incident management. How does Synq help to support the cultural transformation that is necessary?What does an on-call rotation for a data engineer/data platform engineer look like as compared with an application-focused team?How does the focus on data assets/data products shift your approach to observability as compared to a table/pipeline centric approach?With the focus on sharing ownership beyond the boundaries on the data team there is a strong correlation with data governance principles. How do you see organizations incorporating Synq into their approach to data governance/compliance?Can you describe how Synq is designed/implemented? How have the scope and goals of the product changed since you first started working on it?For a team who is onboarding onto Synq, what are the steps required to get it integrated into their technology stack and workflows?What are the types of incidents/errors that you are able to identify and alert on? What does a typical incident/error resolution process look like with Synq?What are the most interesting, innovative, or unexpected ways that you have seen Synq used?What are the most interesting, unexpected, or challenging lessons that you have learned while working on Synq?When is Synq the wrong choice?What do you have planned for the future of Synq?Contact InfoLinkedInSubstackParting QuestionFrom your perspective, what is the biggest gap in the tooling or technology for data management today?Closing AnnouncementsThank you for listening! Don't forget to check out our other shows. Podcast.__init__ covers the Python language, its community, and the innovative ways it is being used. The Machine Learning Podcast helps you go from idea to production with machine learning.Visit the site to subscribe to the show, sign up for the mailing list, and read the show notes.If you've learned something or tried out a project from the show then tell us about it! Email [email protected] with your story.LinksSynqIncident ManagementSLA == Service Level AgreementData GovernancePodcast EpisodePagerDutyOpsGenieClickhousePodcast EpisodedbtPodcast EpisodeSQLMeshPodcast EpisodeThe intro and outro music is from The Hug by The Freak Fandango Orchestra / CC BY-SA
59:4830/06/2024
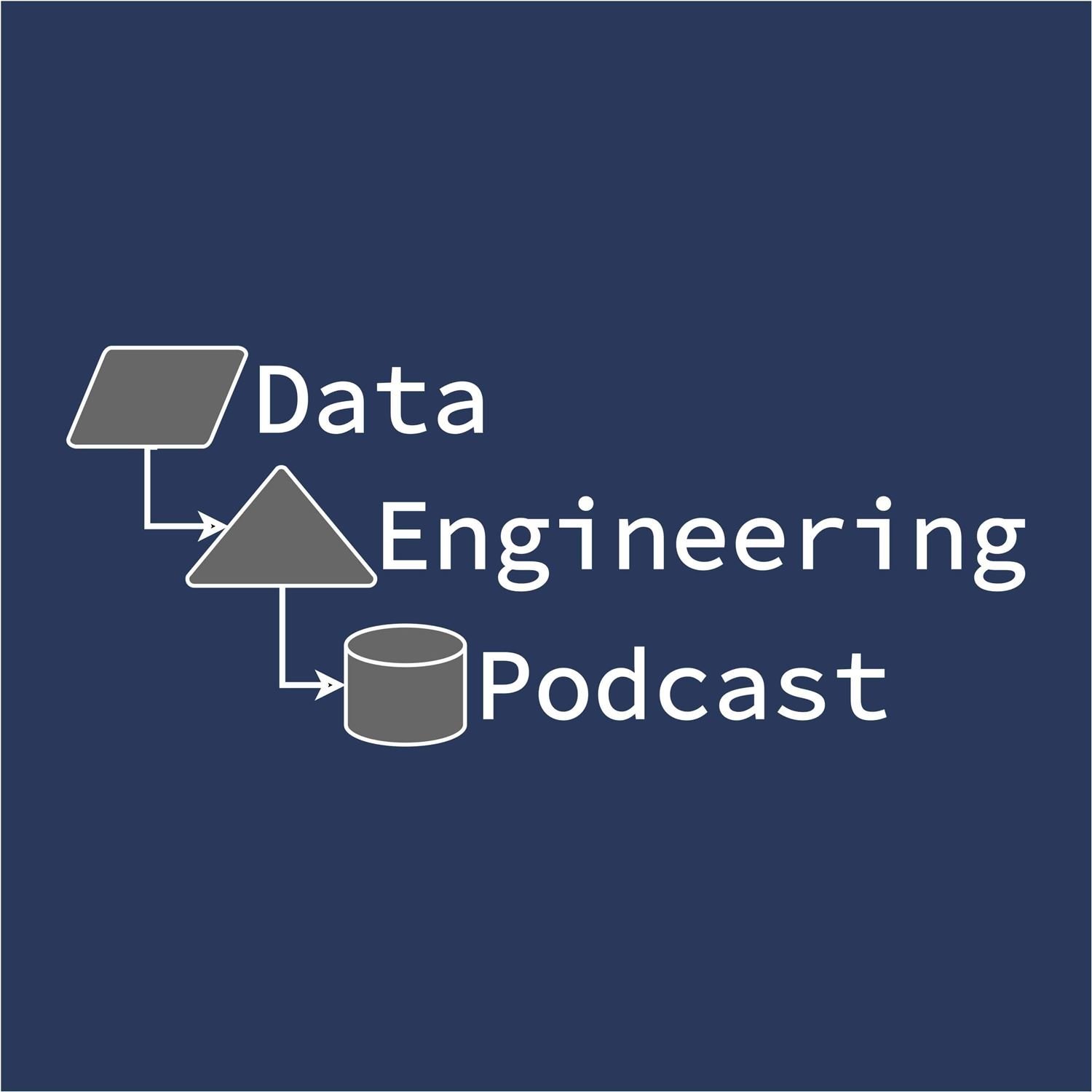
Stitching Together Enterprise Analytics With Microsoft Fabric
Summary
Data lakehouse architectures have been gaining significant adoption. To accelerate adoption in the enterprise Microsoft has created the Fabric platform, based on their OneLake architecture. In this episode Dipti Borkar shares her experiences working on the product team at Fabric and explains the various use cases for the Fabric service.
Announcements
Hello and welcome to the Data Engineering Podcast, the show about modern data management
Data lakes are notoriously complex. For data engineers who battle to build and scale high quality data workflows on the data lake, Starburst is an end-to-end data lakehouse platform built on Trino, the query engine Apache Iceberg was designed for, with complete support for all table formats including Apache Iceberg, Hive, and Delta Lake. Trusted by teams of all sizes, including Comcast and Doordash. Want to see Starburst in action? Go to dataengineeringpodcast.com/starburst and get $500 in credits to try Starburst Galaxy today, the easiest and fastest way to get started using Trino.
Your host is Tobias Macey and today I'm interviewing Dipti Borkar about her work on Microsoft Fabric and performing analytics on data withou
Interview
Introduction
How did you get involved in the area of data management?
Can you describe what Microsoft Fabric is and the story behind it?
Data lakes in various forms have been gaining significant popularity as a unified interface to an organization's analytics. What are the motivating factors that you see for that trend?
Microsoft has been investing heavily in open source in recent years, and the Fabric platform relies on several open components. What are the benefits of layering on top of existing technologies rather than building a fully custom solution?
What are the elements of Fabric that were engineered specifically for the service?
What are the most interesting/complicated integration challenges?
How has your prior experience with Ahana and Presto informed your current work at Microsoft?
AI plays a substantial role in the product. What are the benefits of embedding Copilot into the data engine?
What are the challenges in terms of safety and reliability?
What are the most interesting, innovative, or unexpected ways that you have seen the Fabric platform used?
What are the most interesting, unexpected, or challenging lessons that you have learned while working on data lakes generally, and Fabric specifically?
When is Fabric the wrong choice?
What do you have planned for the future of data lake analytics?
Contact Info
LinkedIn
Parting Question
From your perspective, what is the biggest gap in the tooling or technology for data management today?
Closing Announcements
Thank you for listening! Don't forget to check out our other shows. Podcast.__init__ covers the Python language, its community, and the innovative ways it is being used. The Machine Learning Podcast helps you go from idea to production with machine learning.
Visit the site to subscribe to the show, sign up for the mailing list, and read the show notes.
If you've learned something or tried out a project from the show then tell us about it! Email [email protected] with your story.
Links
Microsoft Fabric
Ahana episode
DB2 Distributed
Spark
Presto
Azure Data
MAD Landscape
Podcast Episode
ML Podcast Episode
Tableau
dbt
Medallion Architecture
Microsoft Onelake
ORC
Parquet
Avro
Delta Lake
Iceberg
Podcast Episode
Hudi
Podcast Episode
Hadoop
PowerBI
Podcast Episode
Velox
Gluten
Apache XTable
GraphQL
Formula 1
McLaren
The intro and outro music is from The Hug by The Freak Fandango Orchestra / CC BY-SASponsored By:Starburst: 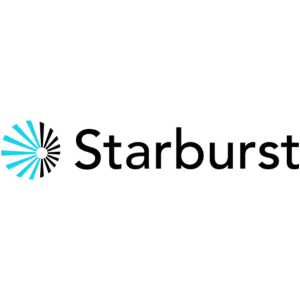
This episode is brought to you by Starburst - an end-to-end data lakehouse platform for data engineers who are battling to build and scale high quality data pipelines on the data lake. Powered by Trino, the query engine Apache Iceberg was designed for, Starburst is an open platform with support for all table formats including Apache Iceberg, Hive, and Delta Lake.
Trusted by the teams at Comcast and Doordash, Starburst delivers the adaptability and flexibility a lakehouse ecosystem promises, while providing a single point of access for your data and all your data governance allowing you to discover, transform, govern, and secure all in one place. Want to see Starburst in action? Try Starburst Galaxy today, the easiest and fastest way to get started using Trino, and get $500 of credits free. Go to [dataengineeringpodcast.com/starburst](https://www.dataengineeringpodcast.com/starburst)Support Data Engineering Podcast
53:2323/06/2024
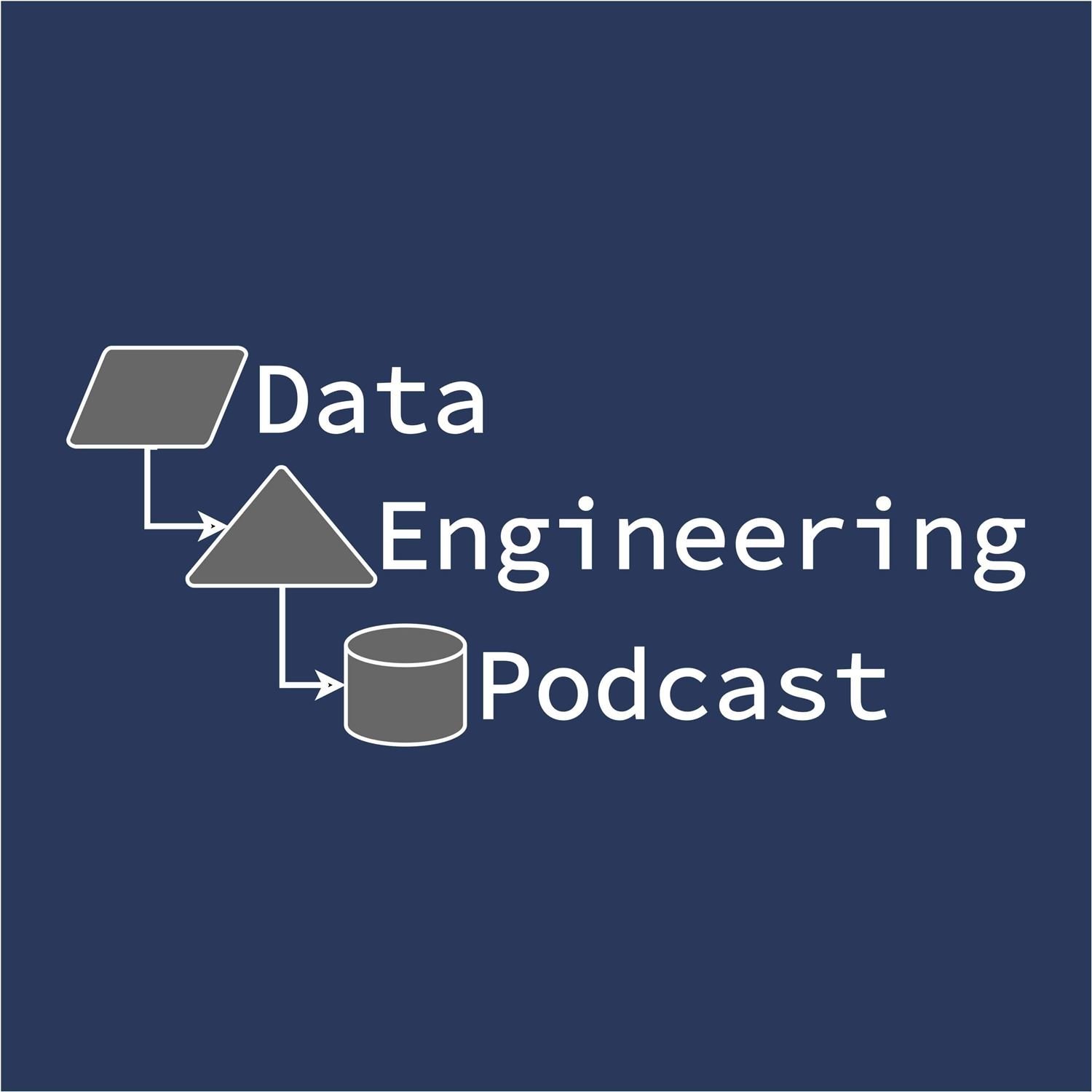
Being Data Driven At Stripe With Trino And Iceberg
Summary
Stripe is a company that relies on data to power their products and business. To support that functionality they have invested in Trino and Iceberg for their analytical workloads. In this episode Kevin Liu shares some of the interesting features that they have built by combining those technologies, as well as the challenges that they face in supporting the myriad workloads that are thrown at this layer of their data platform.
Announcements
Hello and welcome to the Data Engineering Podcast, the show about modern data management
Data lakes are notoriously complex. For data engineers who battle to build and scale high quality data workflows on the data lake, Starburst is an end-to-end data lakehouse platform built on Trino, the query engine Apache Iceberg was designed for, with complete support for all table formats including Apache Iceberg, Hive, and Delta Lake. Trusted by teams of all sizes, including Comcast and Doordash. Want to see Starburst in action? Go to dataengineeringpodcast.com/starburst and get $500 in credits to try Starburst Galaxy today, the easiest and fastest way to get started using Trino.
Your host is Tobias Macey and today I'm interviewing Kevin Liu about his use of Trino and Iceberg for Stripe's data lakehouse
Interview
Introduction
How did you get involved in the area of data management?
Can you describe what role Trino and Iceberg play in Stripe's data architecture?
What are the ways in which your job responsibilities intersect with Stripe's lakehouse infrastructure?
What were the requirements and selection criteria that led to the selection of that combination of technologies?
What are the other systems that feed into and rely on the Trino/Iceberg service?
what kinds of questions are you answering with table metadata
what use case/team does that support
comparative utility of iceberg REST catalog
What are the shortcomings of Trino and Iceberg?
What are the most interesting, innovative, or unexpected ways that you have seen Iceberg/Trino used?
What are the most interesting, unexpected, or challenging lessons that you have learned while working on Stripe's data infrastructure?
When is a lakehouse on Trino/Iceberg the wrong choice?
What do you have planned for the future of Trino and Iceberg at Stripe?
Contact Info
Substack
LinkedIn
Parting Question
From your perspective, what is the biggest gap in the tooling or technology for data management today?
Closing Announcements
Thank you for listening! Don't forget to check out our other shows. Podcast.__init__ covers the Python language, its community, and the innovative ways it is being used. The Machine Learning Podcast helps you go from idea to production with machine learning.
Visit the site to subscribe to the show, sign up for the mailing list, and read the show notes.
If you've learned something or tried out a project from the show then tell us about it! Email [email protected] with your story.
Links
Trino
Iceberg
Stripe
Spark
Redshift
Hive Metastore
Python Iceberg
Python Iceberg REST Catalog
Trino Metadata Table
Flink
Podcast Episode
Tabular
Podcast Episode
Delta Table
Podcast Episode
Databricks Unity Catalog
Starburst
AWS Athena
Kevin Trinofest Presentation
Alluxio
Podcast Episode
Parquet
Hudi
Trino Project Tardigrade
Trino On Ice
The intro and outro music is from The Hug by The Freak Fandango Orchestra / CC BY-SASponsored By:Starburst: 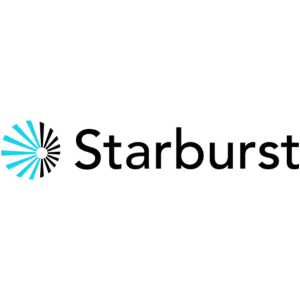
This episode is brought to you by Starburst - an end-to-end data lakehouse platform for data engineers who are battling to build and scale high quality data pipelines on the data lake. Powered by Trino, the query engine Apache Iceberg was designed for, Starburst is an open platform with support for all table formats including Apache Iceberg, Hive, and Delta Lake.
Trusted by the teams at Comcast and Doordash, Starburst delivers the adaptability and flexibility a lakehouse ecosystem promises, while providing a single point of access for your data and all your data governance allowing you to discover, transform, govern, and secure all in one place. Want to see Starburst in action? Try Starburst Galaxy today, the easiest and fastest way to get started using Trino, and get $500 of credits free. Go to [dataengineeringpodcast.com/starburst](https://www.dataengineeringpodcast.com/starburst)Support Data Engineering Podcast
53:2016/06/2024
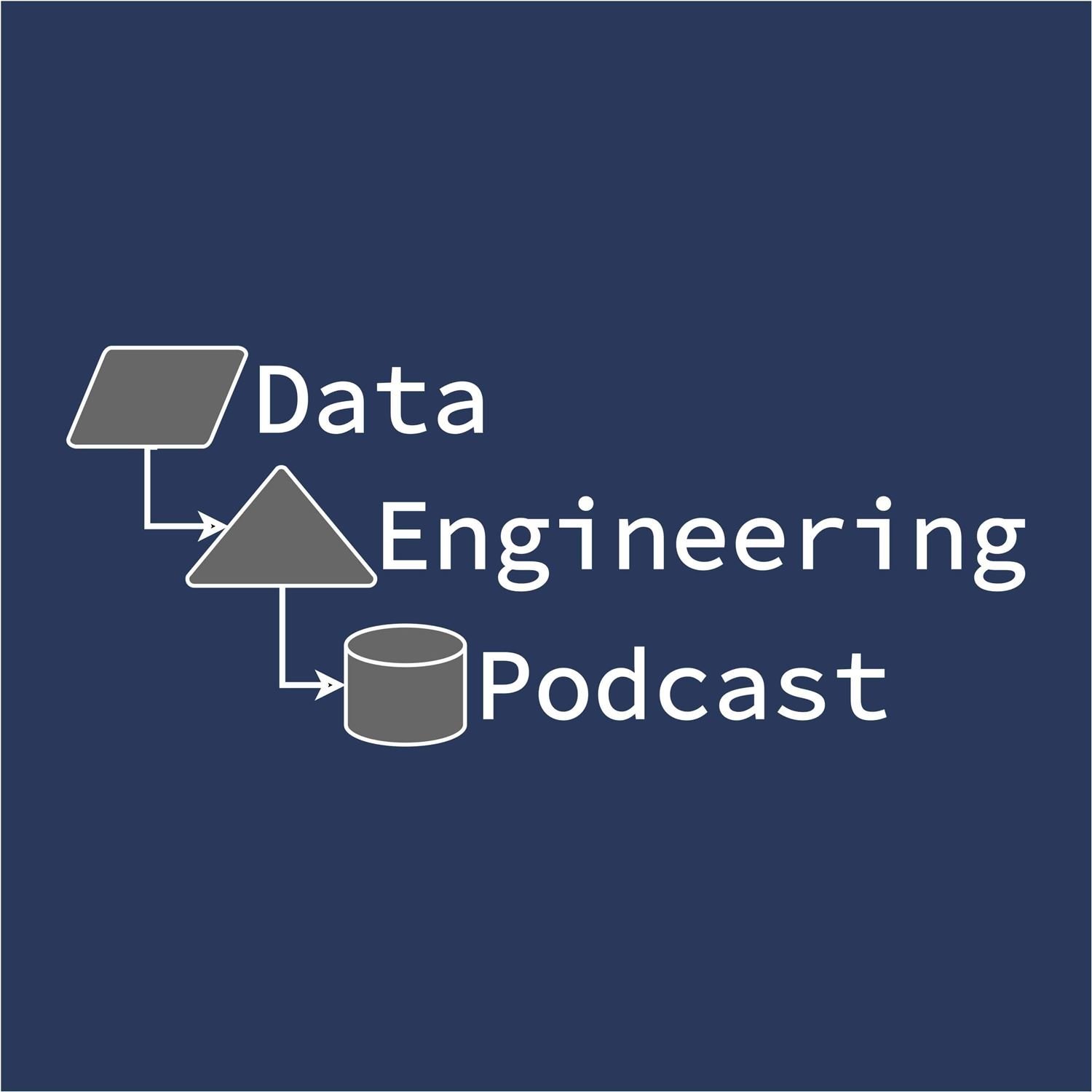
X-Ray Vision For Your Flink Stream Processing With Datorios
Summary
Streaming data processing enables new categories of data products and analytics. Unfortunately, reasoning about stream processing engines is complex and lacks sufficient tooling. To address this shortcoming Datorios created an observability platform for Flink that brings visibility to the internals of this popular stream processing system. In this episode Ronen Korman and Stav Elkayam discuss how the increased understanding provided by purpose built observability improves the usefulness of Flink.
Announcements
Hello and welcome to the Data Engineering Podcast, the show about modern data management
This episode is supported by Code Comments, an original podcast from Red Hat. As someone who listens to the Data Engineering Podcast, you know that the road from tool selection to production readiness is anything but smooth or straight. In Code Comments, host Jamie Parker, Red Hatter and experienced engineer, shares the journey of technologists from across the industry and their hard-won lessons in implementing new technologies. I listened to the recent episode "Transforming Your Database" and appreciated the valuable advice on how to approach the selection and integration of new databases in applications and the impact on team dynamics. There are 3 seasons of great episodes and new ones landing everywhere you listen to podcasts. Search for "Code Commentst" in your podcast player or go to dataengineeringpodcast.com/codecomments today to subscribe. My thanks to the team at Code Comments for their support.
Data lakes are notoriously complex. For data engineers who battle to build and scale high quality data workflows on the data lake, Starburst is an end-to-end data lakehouse platform built on Trino, the query engine Apache Iceberg was designed for, with complete support for all table formats including Apache Iceberg, Hive, and Delta Lake. Trusted by teams of all sizes, including Comcast and Doordash. Want to see Starburst in action? Go to dataengineeringpodcast.com/starburst and get $500 in credits to try Starburst Galaxy today, the easiest and fastest way to get started using Trino.
Your host is Tobias Macey and today I'm interviewing Ronen Korman and Stav Elkayam about pulling back the curtain on your real-time data streams by bringing intuitive observability to Flink streams
Interview
Introduction
How did you get involved in the area of data management?
Can you describe what Datorios is and the story behind it?
Data observability has been gaining adoption for a number of years now, with a large focus on data warehouses. What are some of the unique challenges posed by Flink?
How much of the complexity is due to the nature of streaming data vs. the architectural realities of Flink?
How has the lack of visibility into the flow of data in Flink impacted the ways that teams think about where/when/how to apply it?
How have the requirements of generative AI shifted the demand for streaming data systems?
What role does Flink play in the architecture of generative AI systems?
Can you describe how Datorios is implemented?
How has the design and goals of Datorios changed since you first started working on it?
How much of the Datorios architecture and functionality is specific to Flink and how are you thinking about its potential application to other streaming platforms?
Can you describe how Datorios is used in a day-to-day workflow for someone building streaming applications on Flink?
What are the most interesting, innovative, or unexpected ways that you have seen Datorios used?
What are the most interesting, unexpected, or challenging lessons that you have learned while working on Datorios?
When is Datorios the wrong choice?
What do you have planned for the future of Datorios?
Contact Info
Ronen
LinkedIn
Stav
LinkedIn
Parting Question
From your perspective, what is the biggest gap in the tooling or technology for data management today?
Closing Announcements
Thank you for listening! Don't forget to check out our other shows. Podcast.__init__ covers the Python language, its community, and the innovative ways it is being used. The Machine Learning Podcast helps you go from idea to production with machine learning.
Visit the site to subscribe to the show, sign up for the mailing list, and read the show notes.
If you've learned something or tried out a project from the show then tell us about it! Email [email protected] with your story.
Links
Datorios
Apache Flink
Podcast Episode
ChatGPT-4o
The intro and outro music is from The Hug by The Freak Fandango Orchestra / CC BY-SASponsored By:Starburst: 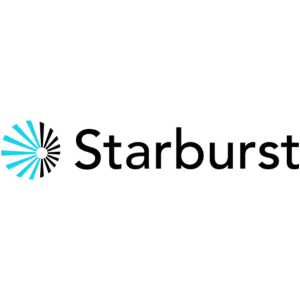
This episode is brought to you by Starburst - an end-to-end data lakehouse platform for data engineers who are battling to build and scale high quality data pipelines on the data lake. Powered by Trino, the query engine Apache Iceberg was designed for, Starburst is an open platform with support for all table formats including Apache Iceberg, Hive, and Delta Lake.
Trusted by the teams at Comcast and Doordash, Starburst delivers the adaptability and flexibility a lakehouse ecosystem promises, while providing a single point of access for your data and all your data governance allowing you to discover, transform, govern, and secure all in one place. Want to see Starburst in action? Try Starburst Galaxy today, the easiest and fastest way to get started using Trino, and get $500 of credits free. Go to [dataengineeringpodcast.com/starburst](https://www.dataengineeringpodcast.com/starburst)Red Hat Code Comments Podcast: 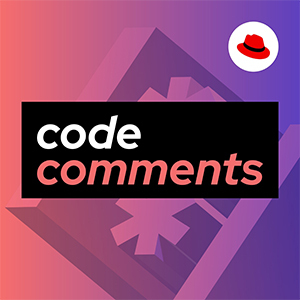
Putting new technology to use is an exciting prospect. But going from purchase to production isn’t always smooth—even when it’s something everyone is looking forward to. Code Comments covers the bumps, the hiccups, and the setbacks teams face when adjusting to new technology—and the triumphs they pull off once they really get going. Follow Code Comments [anywhere you listen to podcasts](https://link.chtbl.com/codecomments?sid=podcast.dataengineering).Support Data Engineering Podcast
42:2209/06/2024
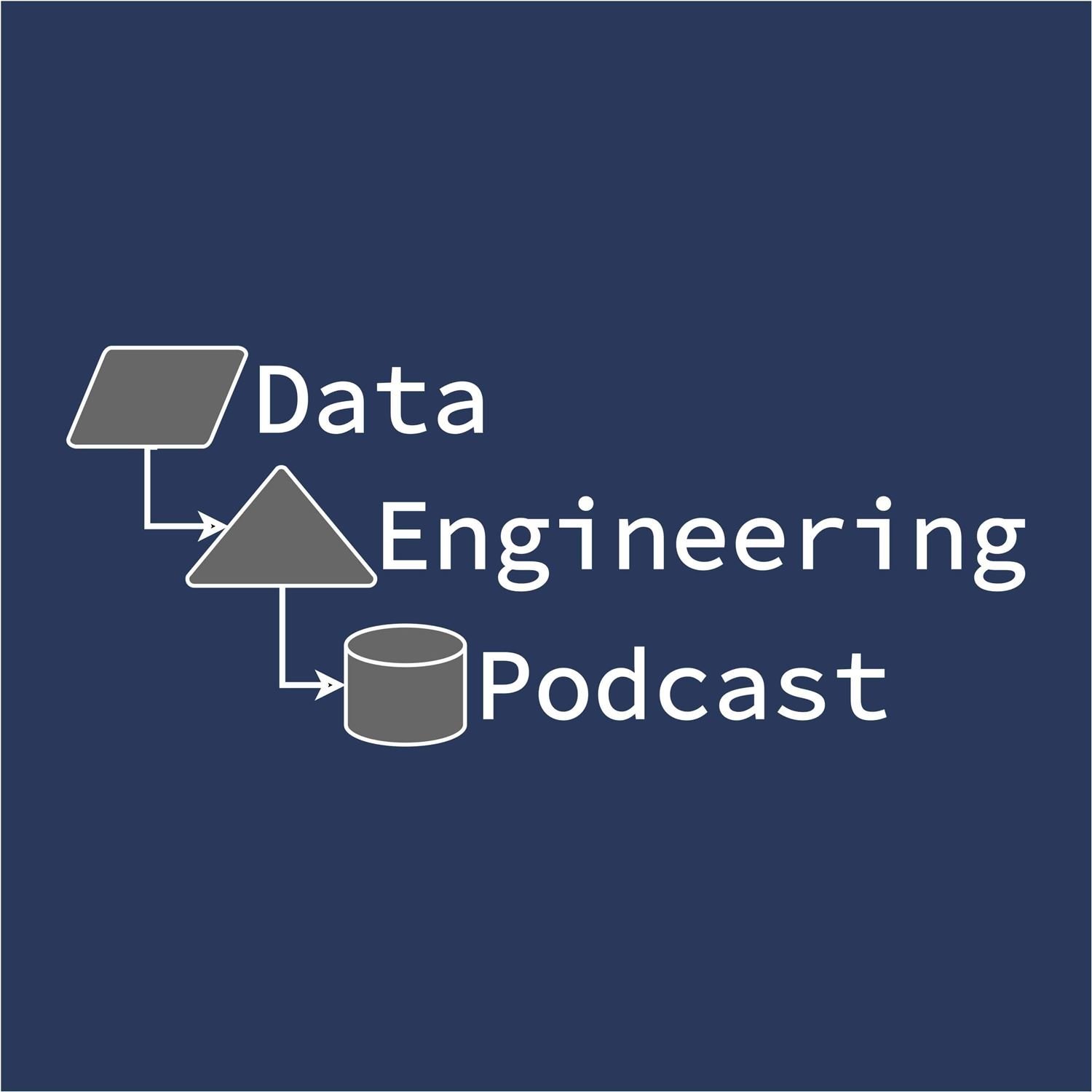
Practical First Steps In Data Governance For Long Term Success
Summary
Modern businesses aspire to be data driven, and technologists enjoy working through the challenge of building data systems to support that goal. Data governance is the binding force between these two parts of the organization. Nicola Askham found her way into data governance by accident, and stayed because of the benefit that she was able to provide by serving as a bridge between the technology and business. In this episode she shares the practical steps to implementing a data governance practice in your organization, and the pitfalls to avoid.
Announcements
Hello and welcome to the Data Engineering Podcast, the show about modern data management
Data lakes are notoriously complex. For data engineers who battle to build and scale high quality data workflows on the data lake, Starburst is an end-to-end data lakehouse platform built on Trino, the query engine Apache Iceberg was designed for, with complete support for all table formats including Apache Iceberg, Hive, and Delta Lake. Trusted by teams of all sizes, including Comcast and Doordash. Want to see Starburst in action? Go to dataengineeringpodcast.com/starburst and get $500 in credits to try Starburst Galaxy today, the easiest and fastest way to get started using Trino.
This episode is supported by Code Comments, an original podcast from Red Hat. As someone who listens to the Data Engineering Podcast, you know that the road from tool selection to production readiness is anything but smooth or straight. In Code Comments, host Jamie Parker, Red Hatter and experienced engineer, shares the journey of technologists from across the industry and their hard-won lessons in implementing new technologies. I listened to the recent episode "Transforming Your Database" and appreciated the valuable advice on how to approach the selection and integration of new databases in applications and the impact on team dynamics. There are 3 seasons of great episodes and new ones landing everywhere you listen to podcasts. Search for "Code Commentst" in your podcast player or go to dataengineeringpodcast.com/codecomments today to subscribe. My thanks to the team at Code Comments for their support.
Your host is Tobias Macey and today I'm interviewing Nicola Askham about the practical steps of building out a data governance practice in your organization
Interview
Introduction
How did you get involved in the area of data management?
Can you start by giving an overview of the scope and boundaries of data governance in an organization?
At what point does a lack of an explicit governance policy become a liability?
What are some of the misconceptions that you encounter about data governance?
What impact has the evolution of data technologies had on the implementation of governance practices? (e.g. number/scale of systems, types of data, AI)
Data governance can often become an exercise in boiling the ocean. What are the concrete first steps that will increase the success rate of a governance practice?
Once a data governance project is underway, what are some of the common roadblocks that might derail progress?
What are the net benefits to the data team and the organization when a data governance practice is established, active, and healthy?
What are the most interesting, innovative, or unexpected ways that you have seen data governance applied?
What are the most interesting, unexpected, or challenging lessons that you have learned while working on data governance/training/coaching?
What are some of the pitfalls in data governance?
What are some of the future trends in data governance that you are excited by?
Are there any trends that concern you?
Contact Info
Website
LinkedIn
Parting Question
From your perspective, what is the biggest gap in the tooling or technology for data management today?
Closing Announcements
Thank you for listening! Don't forget to check out our other shows. Podcast.__init__ covers the Python language, its community, and the innovative ways it is being used. The Machine Learning Podcast helps you go from idea to production with machine learning.
Visit the site to subscribe to the show, sign up for the mailing list, and read the show notes.
If you've learned something or tried out a project from the show then tell us about it! Email [email protected]) with your story.
Links
Website
Master Data Management
Cartesian Join
DAMA == Data Management Community
DMBOK == Data Management Body of Knowledge
DAMA DMBOK Wheel
CDMP (Certified Data Management Professional) Exam
Data Mesh
Data Governance First Steps Checklist
The Never Normal
The intro and outro music is from The Hug by The Freak Fandango Orchestra / CC BY-SASponsored By:Red Hat Code Comments Podcast: 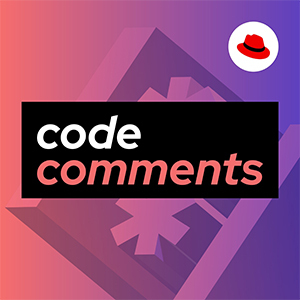
Putting new technology to use is an exciting prospect. But going from purchase to production isn’t always smooth—even when it’s something everyone is looking forward to. Code Comments covers the bumps, the hiccups, and the setbacks teams face when adjusting to new technology—and the triumphs they pull off once they really get going. Follow Code Comments [anywhere you listen to podcasts](https://link.chtbl.com/codecomments?sid=podcast.dataengineering).Starburst: 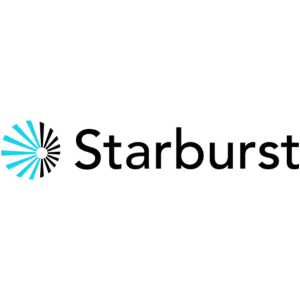
This episode is brought to you by Starburst - an end-to-end data lakehouse platform for data engineers who are battling to build and scale high quality data pipelines on the data lake. Powered by Trino, the query engine Apache Iceberg was designed for, Starburst is an open platform with support for all table formats including Apache Iceberg, Hive, and Delta Lake.
Trusted by the teams at Comcast and Doordash, Starburst delivers the adaptability and flexibility a lakehouse ecosystem promises, while providing a single point of access for your data and all your data governance allowing you to discover, transform, govern, and secure all in one place. Want to see Starburst in action? Try Starburst Galaxy today, the easiest and fastest way to get started using Trino, and get $500 of credits free. Go to [dataengineeringpodcast.com/starburst](https://www.dataengineeringpodcast.com/starburst)Support Data Engineering Podcast
01:00:4102/06/2024
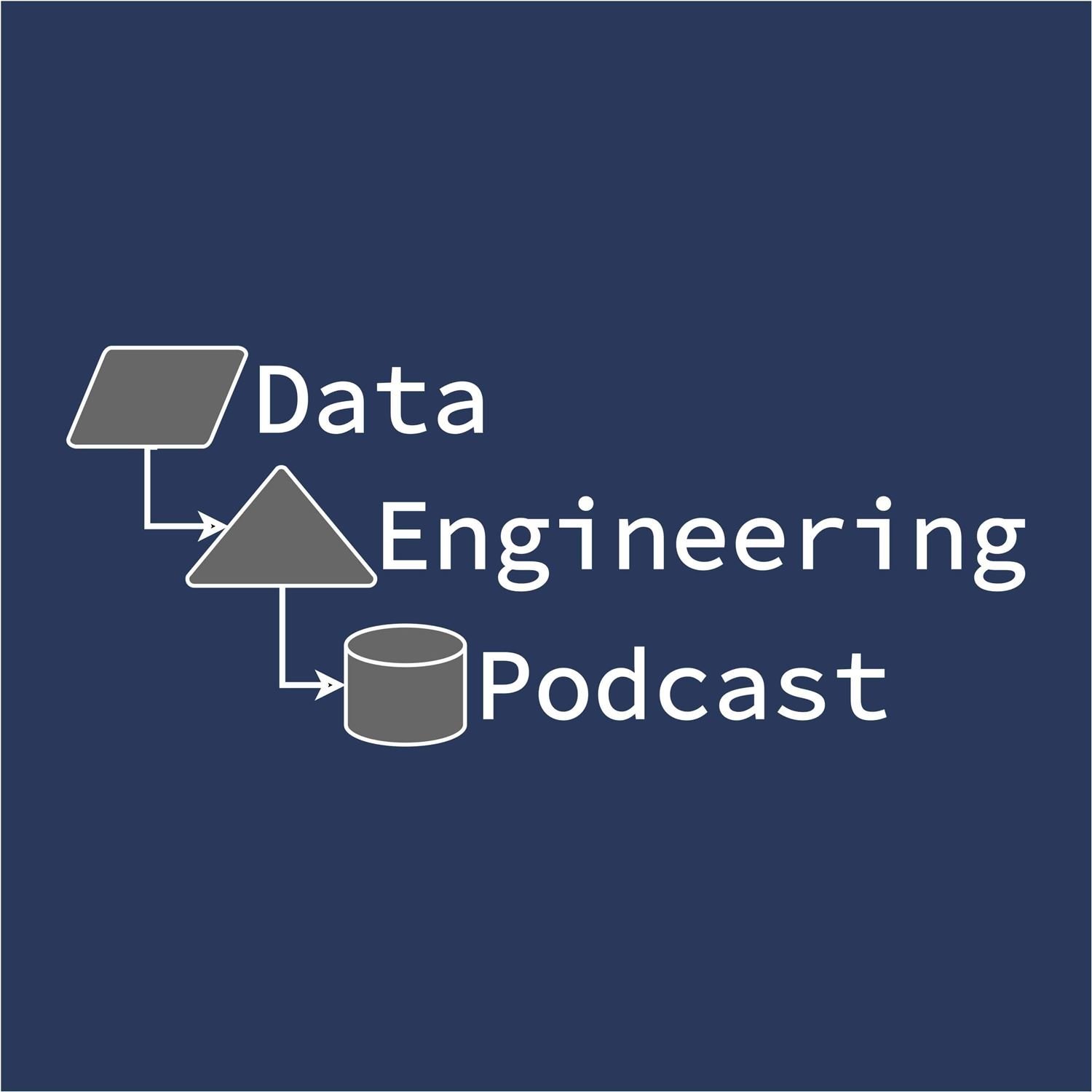
Data Migration Strategies For Large Scale Systems
Summary
Any software system that survives long enough will require some form of migration or evolution. When that system is responsible for the data layer the process becomes more challenging. Sriram Panyam has been involved in several projects that required migration of large volumes of data in high traffic environments. In this episode he shares some of the valuable lessons that he learned about how to make those projects successful.
Announcements
Hello and welcome to the Data Engineering Podcast, the show about modern data management
Data lakes are notoriously complex. For data engineers who battle to build and scale high quality data workflows on the data lake, Starburst is an end-to-end data lakehouse platform built on Trino, the query engine Apache Iceberg was designed for, with complete support for all table formats including Apache Iceberg, Hive, and Delta Lake. Trusted by teams of all sizes, including Comcast and Doordash. Want to see Starburst in action? Go to dataengineeringpodcast.com/starburst and get $500 in credits to try Starburst Galaxy today, the easiest and fastest way to get started using Trino.
This episode is supported by Code Comments, an original podcast from Red Hat. As someone who listens to the Data Engineering Podcast, you know that the road from tool selection to production readiness is anything but smooth or straight. In Code Comments, host Jamie Parker, Red Hatter and experienced engineer, shares the journey of technologists from across the industry and their hard-won lessons in implementing new technologies. I listened to the recent episode "Transforming Your Database" and appreciated the valuable advice on how to approach the selection and integration of new databases in applications and the impact on team dynamics. There are 3 seasons of great episodes and new ones landing everywhere you listen to podcasts. Search for "Code Commentst" in your podcast player or go to dataengineeringpodcast.com/codecomments today to subscribe. My thanks to the team at Code Comments for their support.
Your host is Tobias Macey and today I'm interviewing Sriram Panyam about his experiences conducting large scale data migrations and the useful strategies that he learned in the process
Interview
Introduction
How did you get involved in the area of data management?
Can you start by sharing some of your experiences with data migration projects?
As you have gone through successive migration projects, how has that influenced the ways that you think about architecting data systems?
How would you categorize the different types and motivations of migrations?
How does the motivation for a migration influence the ways that you plan for and execute that work?
Can you talk us through one or two specific projects that you have taken part in?
Part 1: The Triggers
Section 1: Technical Limitations triggering Data Migration
Scaling bottlenecks: Performance issues with databases, storage, or network infrastructure
Legacy compatibility: Difficulties integrating with modern tools and cloud platforms
System upgrades: The need to migrate data during major software changes (e.g., SQL Server version upgrade)
Section 2: Types of Migrations for Infrastructure Focus
Storage migration: Moving data between systems (HDD to SSD, SAN to NAS, etc.)
Data center migration: Physical relocation or consolidation of data centers
Virtualization migration: Moving from physical servers to virtual machines (or vice versa)
Section 3: Technical Decisions Driving Data Migrations
End-of-life support: Forced migration when older software or hardware is sunsetted
Security and compliance: Adopting new platforms with better security postures
Cost Optimization: Potential savings of cloud vs. on-premise data centers
Part 2: Challenges (and Anxieties)
Section 1: Technical Challenges
Data transformation challenges: Schema changes, complex data mappings
Network bandwidth and latency: Transferring large datasets efficiently
Performance testing and load balancing: Ensuring new systems can handle the workload
Live data consistency: Maintaining data integrity while updates occur in the source system
Minimizing Lag: Techniques to reduce delays in replicating changes to the new system
Change data capture: Identifying and tracking changes to the source system during migration
Section 2: Operational Challenges
Minimizing downtime: Strategies for service continuity during migration
Change management and rollback plans: Dealing with unexpected issues
Technical skills and resources: In-house expertise/data teams/external help
Section 3: Security & Compliance Challenges
Data encryption and protection: Methods for both in-transit and at-rest data
Meeting audit requirements: Documenting data lineage & the chain of custody
Managing access controls: Adjusting identity and role-based access to the new systems
Part 3: Patterns
Section 1: Infrastructure Migration Strategies
Lift and shift: Migrating as-is vs. modernization and re-architecting during the move
Phased vs. big bang approaches: Tradeoffs in risk vs. disruption
Tools and automation: Using specialized software to streamline the process
Dual writes: Managing updates to both old and new systems for a time
Change data capture (CDC) methods: Log-based vs. trigger-based approaches for tracking changes
Data validation & reconciliation: Ensuring consistency between source and target
Section 2: Maintaining Performance and Reliability
Disaster recovery planning: Failover mechanisms for the new environment
Monitoring and alerting: Proactively identifying and addressing issues
Capacity planning and forecasting growth to scale the new infrastructure
Section 3: Data Consistency and Replication
Replication tools - strategies and specialized tooling
Data synchronization techniques, eg Pros and cons of different methods (incremental vs. full)
Testing/Verification Strategies for validating data correctness in a live environment
Implication of large scale systems/environments
Comparison of interesting strategies:
DBLog, Debezium, Databus, Goldengate etc
What are the most interesting, innovative, or unexpected approaches to data migrations that you have seen or participated in?
What are the most interesting, unexpected, or challenging lessons that you have learned while working on data migrations?
When is a migration the wrong choice?
What are the characteristics or features of data technologies and the overall ecosystem that can reduce the burden of data migration in the future?
Contact Info
LinkedIn
Parting Question
From your perspective, what is the biggest gap in the tooling or technology for data management today?
Closing Announcements
Thank you for listening! Don't forget to check out our other shows. Podcast.__init__ covers the Python language, its community, and the innovative ways it is being used. The Machine Learning Podcast helps you go from idea to production with machine learning.
Visit the site to subscribe to the show, sign up for the mailing list, and read the show notes.
If you've learned something or tried out a project from the show then tell us about it! Email [email protected]) with your story.
Links
DagKnows
Google Cloud Dataflow
Seinfeld Risk Management
ACL == Access Control List
LinkedIn Databus - Change Data Capture
Espresso Storage
HDFS
Kafka
Postgres Replication Slots
Queueing Theory
Apache Beam
Debezium
Airbyte
[Fivetran](fivetran.com)
Designing Data Intensive Applications by Martin Kleppman (affiliate link)
Vector Databases
Pinecone
Weaviate
LAMP Stack
Netflix DBLog
The intro and outro music is from The Hug by The Freak Fandango Orchestra / CC BY-SASponsored By:Red Hat Code Comments Podcast: 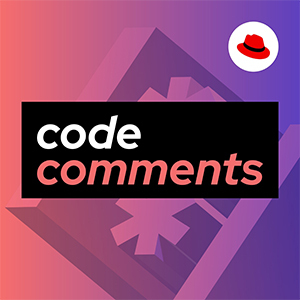
Putting new technology to use is an exciting prospect. But going from purchase to production isn’t always smooth—even when it’s something everyone is looking forward to. Code Comments covers the bumps, the hiccups, and the setbacks teams face when adjusting to new technology—and the triumphs they pull off once they really get going. Follow Code Comments [anywhere you listen to podcasts](https://link.chtbl.com/codecomments?sid=podcast.dataengineering).Starburst: 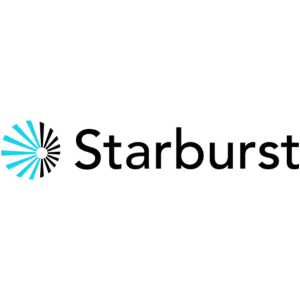
This episode is brought to you by Starburst - an end-to-end data lakehouse platform for data engineers who are battling to build and scale high quality data pipelines on the data lake. Powered by Trino, the query engine Apache Iceberg was designed for, Starburst is an open platform with support for all table formats including Apache Iceberg, Hive, and Delta Lake.
Trusted by the teams at Comcast and Doordash, Starburst delivers the adaptability and flexibility a lakehouse ecosystem promises, while providing a single point of access for your data and all your data governance allowing you to discover, transform, govern, and secure all in one place. Want to see Starburst in action? Try Starburst Galaxy today, the easiest and fastest way to get started using Trino, and get $500 of credits free. Go to [dataengineeringpodcast.com/starburst](https://www.dataengineeringpodcast.com/starburst)Support Data Engineering Podcast
01:00:0027/05/2024
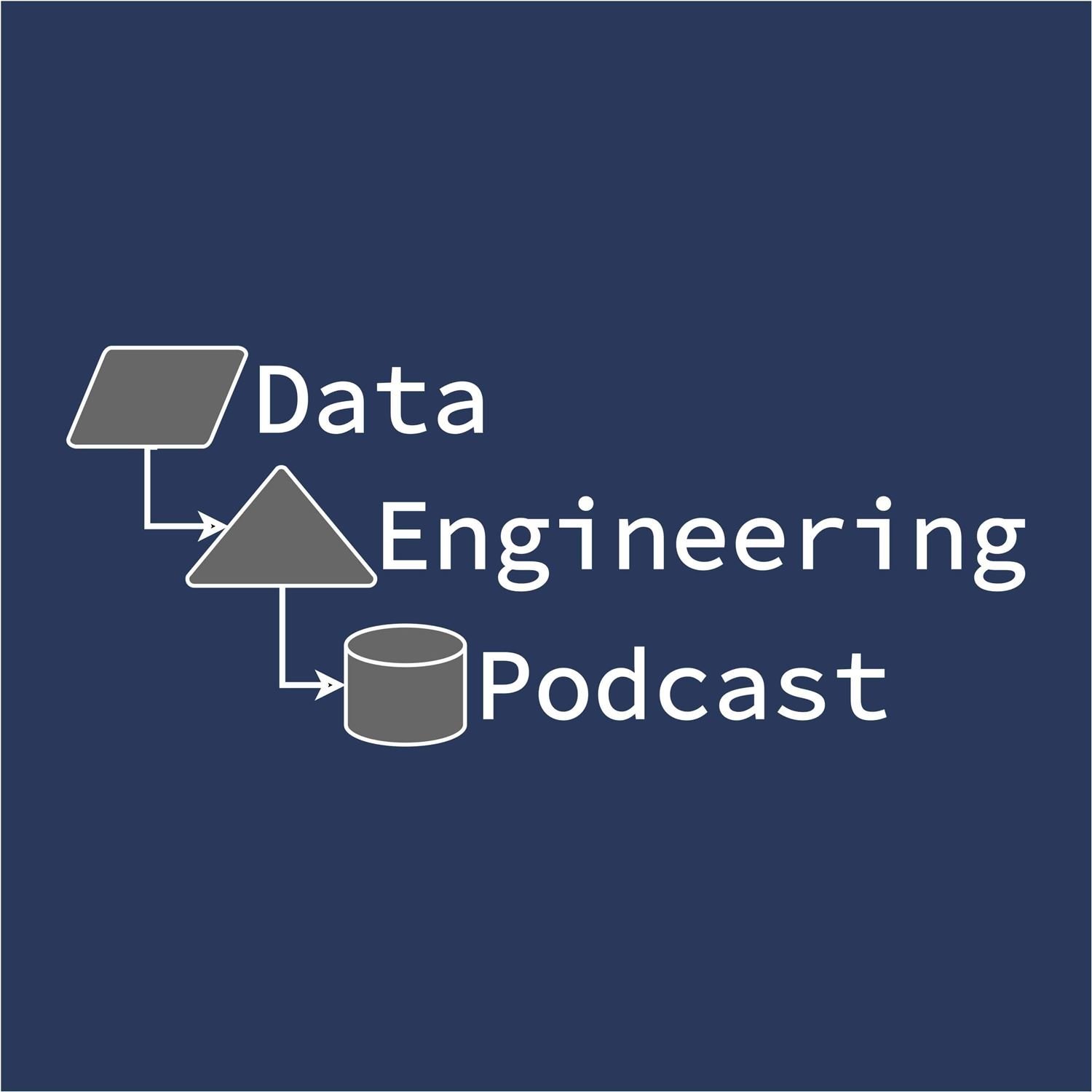
Zenlytic Is Building You A Better Coworker With AI Agents
Summary
The purpose of business intelligence systems is to allow anyone in the business to access and decode data to help them make informed decisions. Unfortunately this often turns into an exercise in frustration for everyone involved due to complex workflows and hard-to-understand dashboards. The team at Zenlytic have leaned on the promise of large language models to build an AI agent that lets you converse with your data. In this episode they share their journey through the fast-moving landscape of generative AI and unpack the difference between an AI chatbot and an AI agent.
Announcements
Hello and welcome to the Data Engineering Podcast, the show about modern data management
This episode is supported by Code Comments, an original podcast from Red Hat. As someone who listens to the Data Engineering Podcast, you know that the road from tool selection to production readiness is anything but smooth or straight. In Code Comments, host Jamie Parker, Red Hatter and experienced engineer, shares the journey of technologists from across the industry and their hard-won lessons in implementing new technologies. I listened to the recent episode "Transforming Your Database" and appreciated the valuable advice on how to approach the selection and integration of new databases in applications and the impact on team dynamics. There are 3 seasons of great episodes and new ones landing everywhere you listen to podcasts. Search for "Code Commentst" in your podcast player or go to dataengineeringpodcast.com/codecomments today to subscribe. My thanks to the team at Code Comments for their support.
Data lakes are notoriously complex. For data engineers who battle to build and scale high quality data workflows on the data lake, Starburst is an end-to-end data lakehouse platform built on Trino, the query engine Apache Iceberg was designed for, with complete support for all table formats including Apache Iceberg, Hive, and Delta Lake. Trusted by teams of all sizes, including Comcast and Doordash. Want to see Starburst in action? Go to dataengineeringpodcast.com/starburst and get $500 in credits to try Starburst Galaxy today, the easiest and fastest way to get started using Trino.
Your host is Tobias Macey and today I'm interviewing Ryan Janssen and Paul Blankley about their experiences building AI powered agents for interacting with your data
Interview
Introduction
How did you get involved in data? In AI?
Can you describe what Zenlytic is and the role that AI is playing in your platform?
What have been the key stages in your AI journey?
What are some of the dead ends that you ran into along the path to where you are today?
What are some of the persistent challenges that you are facing?
So tell us more about data agents. Firstly, what are data agents and why do you think they're important?
How are data agents different from chatbots?
Are data agents harder to build? How do you make them work in production?
What other technical architectures have you had to develop to support the use of AI in Zenlytic?
How have you approached the work of customer education as you introduce this functionality?
What are some of the most interesting or erroneous misconceptions that you have heard about what the AI can and can't do?
How have you balanced accuracy/trustworthiness with user experience and flexibility in the conversational AI, given the potential for these models to create erroneous responses?
What are the most interesting, innovative, or unexpected ways that you have seen your AI agent used?
What are the most interesting, unexpected, or challenging lessons that you have learned while working on building an AI agent for business intelligence?
When is an AI agent the wrong choice?
What do you have planned for the future of AI in the Zenlytic product?
Contact Info
Ryan
LinkedIn
Paul
LinkedIn
Parting Question
From your perspective, what is the biggest gap in the tooling or technology for data management today?
Closing Announcements
Thank you for listening! Don't forget to check out our other shows. Podcast.__init__ covers the Python language, its community, and the innovative ways it is being used. The Machine Learning Podcast helps you go from idea to production with machine learning.
Visit the site to subscribe to the show, sign up for the mailing list, and read the show notes.
If you've learned something or tried out a project from the show then tell us about it! Email [email protected]) with your story.
Links
Zenlytic
Podcast Episode
Attention is all you need
Transformers
BERT
The Bitter Lesson Richard Sutton
PID Loops
AutoGPT
Devin.ai
Google Gemini
Anthropic Claude
OpenAI Code Interpreter
Edward Tufte
Looker ActionHub
OAuth
GitHub Copilot
The intro and outro music is from The Hug by The Freak Fandango Orchestra / CC BY-SASponsored By:Starburst: 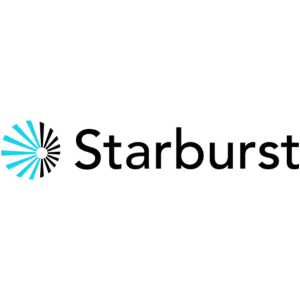
This episode is brought to you by Starburst - an end-to-end data lakehouse platform for data engineers who are battling to build and scale high quality data pipelines on the data lake. Powered by Trino, the query engine Apache Iceberg was designed for, Starburst is an open platform with support for all table formats including Apache Iceberg, Hive, and Delta Lake.
Trusted by the teams at Comcast and Doordash, Starburst delivers the adaptability and flexibility a lakehouse ecosystem promises, while providing a single point of access for your data and all your data governance allowing you to discover, transform, govern, and secure all in one place. Want to see Starburst in action? Try Starburst Galaxy today, the easiest and fastest way to get started using Trino, and get $500 of credits free. Go to [dataengineeringpodcast.com/starburst](https://www.dataengineeringpodcast.com/starburst)Red Hat Code Comments Podcast: 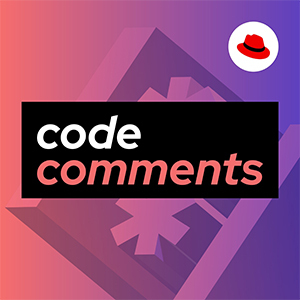
Putting new technology to use is an exciting prospect. But going from purchase to production isn’t always smooth—even when it’s something everyone is looking forward to. Code Comments covers the bumps, the hiccups, and the setbacks teams face when adjusting to new technology—and the triumphs they pull off once they really get going. Follow Code Comments [anywhere you listen to podcasts](https://link.chtbl.com/codecomments?sid=podcast.dataengineering).Support Data Engineering Podcast
54:1919/05/2024
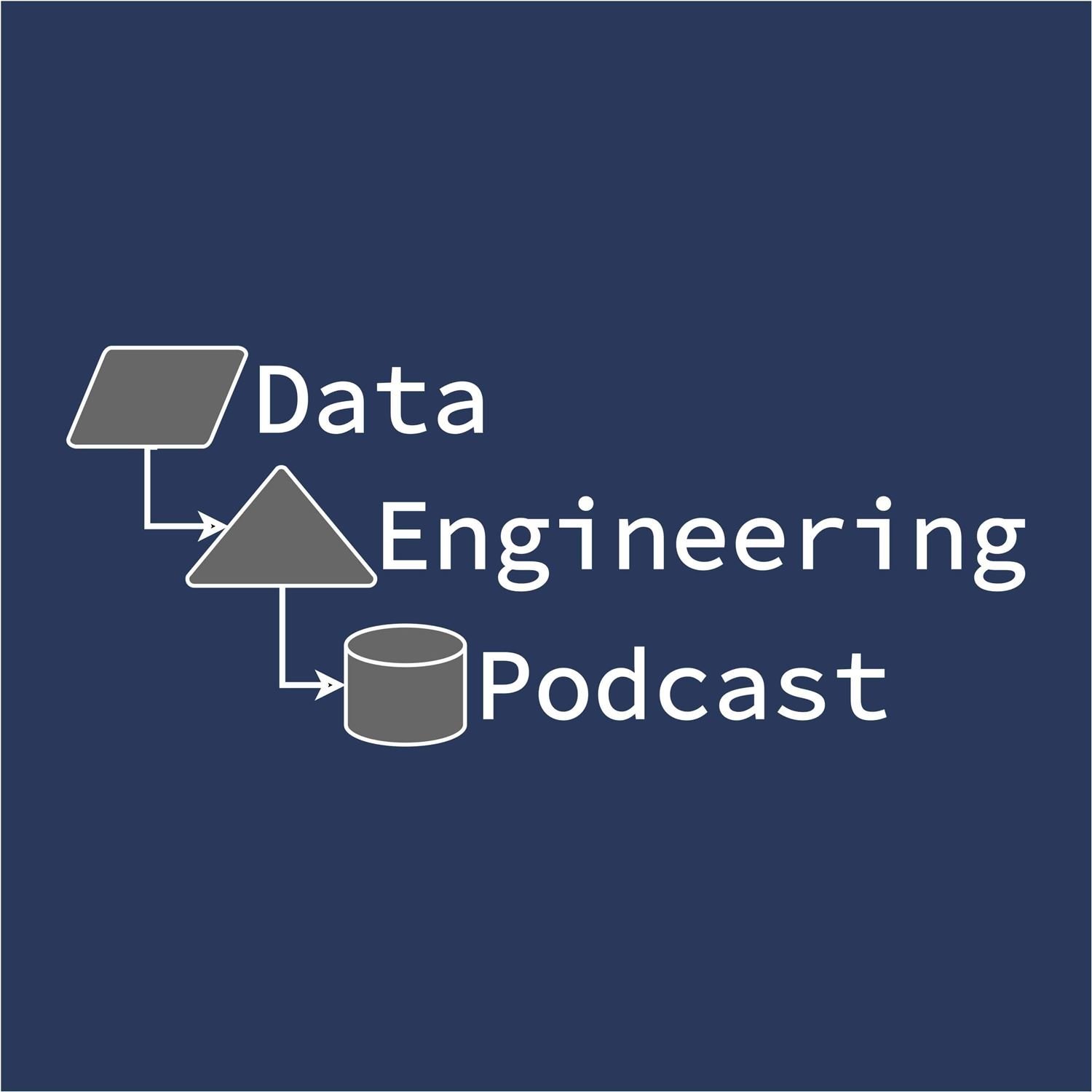
Release Management For Data Platform Services And Logic
Summary
Building a data platform is a substrantial engineering endeavor. Once it is running, the next challenge is figuring out how to address release management for all of the different component parts. The services and systems need to be kept up to date, but so does the code that controls their behavior. In this episode your host Tobias Macey reflects on his current challenges in this area and some of the factors that contribute to the complexity of the problem.
Announcements
Hello and welcome to the Data Engineering Podcast, the show about modern data management
This episode is supported by Code Comments, an original podcast from Red Hat. As someone who listens to the Data Engineering Podcast, you know that the road from tool selection to production readiness is anything but smooth or straight. In Code Comments, host Jamie Parker, Red Hatter and experienced engineer, shares the journey of technologists from across the industry and their hard-won lessons in implementing new technologies. I listened to the recent episode "Transforming Your Database" and appreciated the valuable advice on how to approach the selection and integration of new databases in applications and the impact on team dynamics. There are 3 seasons of great episodes and new ones landing everywhere you listen to podcasts. Search for "Code Commentst" in your podcast player or go to dataengineeringpodcast.com/codecomments today to subscribe. My thanks to the team at Code Comments for their support.
Data lakes are notoriously complex. For data engineers who battle to build and scale high quality data workflows on the data lake, Starburst is an end-to-end data lakehouse platform built on Trino, the query engine Apache Iceberg was designed for, with complete support for all table formats including Apache Iceberg, Hive, and Delta Lake. Trusted by teams of all sizes, including Comcast and Doordash. Want to see Starburst in action? Go to dataengineeringpodcast.com/starburst and get $500 in credits to try Starburst Galaxy today, the easiest and fastest way to get started using Trino.
Your host is Tobias Macey and today I want to talk about my experiences managing the QA and release management process of my data platform
Interview
Introduction
As a team, our overall goal is to ensure that the production environment for our data platform is highly stable and reliable. This is the foundational element of establishing and maintaining trust with the consumers of our data. In order to support this effort, we need to ensure that only changes that have been tested and verified are promoted to production.
Our current challenge is one that plagues all data teams. We want to have an environment that mirrors our production environment that is available for testing, but it’s not feasible to maintain a complete duplicate of all of the production data. Compounding that challenge is the fact that each of the components of our data platform interact with data in slightly different ways and need different processes for ensuring that changes are being promoted safely.
Contact Info
LinkedIn
Website
Closing Announcements
Thank you for listening! Don't forget to check out our other shows. Podcast.__init__ covers the Python language, its community, and the innovative ways it is being used. The Machine Learning Podcast helps you go from idea to production with machine learning.
Visit the site to subscribe to the show, sign up for the mailing list, and read the show notes.
If you've learned something or tried out a project from the show then tell us about it! Email [email protected] with your story.
Links
Data Platforms and Leaky Abstractions Episode
Building A Data Platform From Scratch
Airbyte
Podcast Episode
Trino
dbt
Starburst Galaxy
Superset
Dagster
LakeFS
Podcast Episode
Nessie
Podcast Episode
Iceberg
Snowflake
LocalStack
DSL == Domain Specific Language
The intro and outro music is from The Hug by The Freak Fandango Orchestra / CC BY-SASponsored By:Starburst: 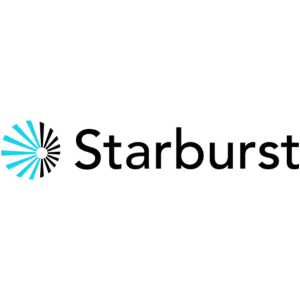
This episode is brought to you by Starburst - an end-to-end data lakehouse platform for data engineers who are battling to build and scale high quality data pipelines on the data lake. Powered by Trino, the query engine Apache Iceberg was designed for, Starburst is an open platform with support for all table formats including Apache Iceberg, Hive, and Delta Lake.
Trusted by the teams at Comcast and Doordash, Starburst delivers the adaptability and flexibility a lakehouse ecosystem promises, while providing a single point of access for your data and all your data governance allowing you to discover, transform, govern, and secure all in one place. Want to see Starburst in action? Try Starburst Galaxy today, the easiest and fastest way to get started using Trino, and get $500 of credits free. Go to [dataengineeringpodcast.com/starburst](https://www.dataengineeringpodcast.com/starburst)Red Hat Code Comments Podcast: 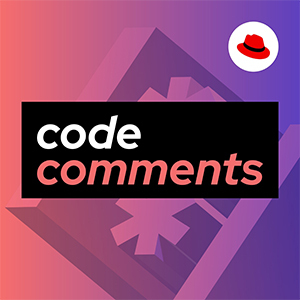
Putting new technology to use is an exciting prospect. But going from purchase to production isn’t always smooth—even when it’s something everyone is looking forward to. Code Comments covers the bumps, the hiccups, and the setbacks teams face when adjusting to new technology—and the triumphs they pull off once they really get going. Follow Code Comments [anywhere you listen to podcasts](https://link.chtbl.com/codecomments?sid=podcast.dataengineering).Support Data Engineering Podcast
20:0912/05/2024
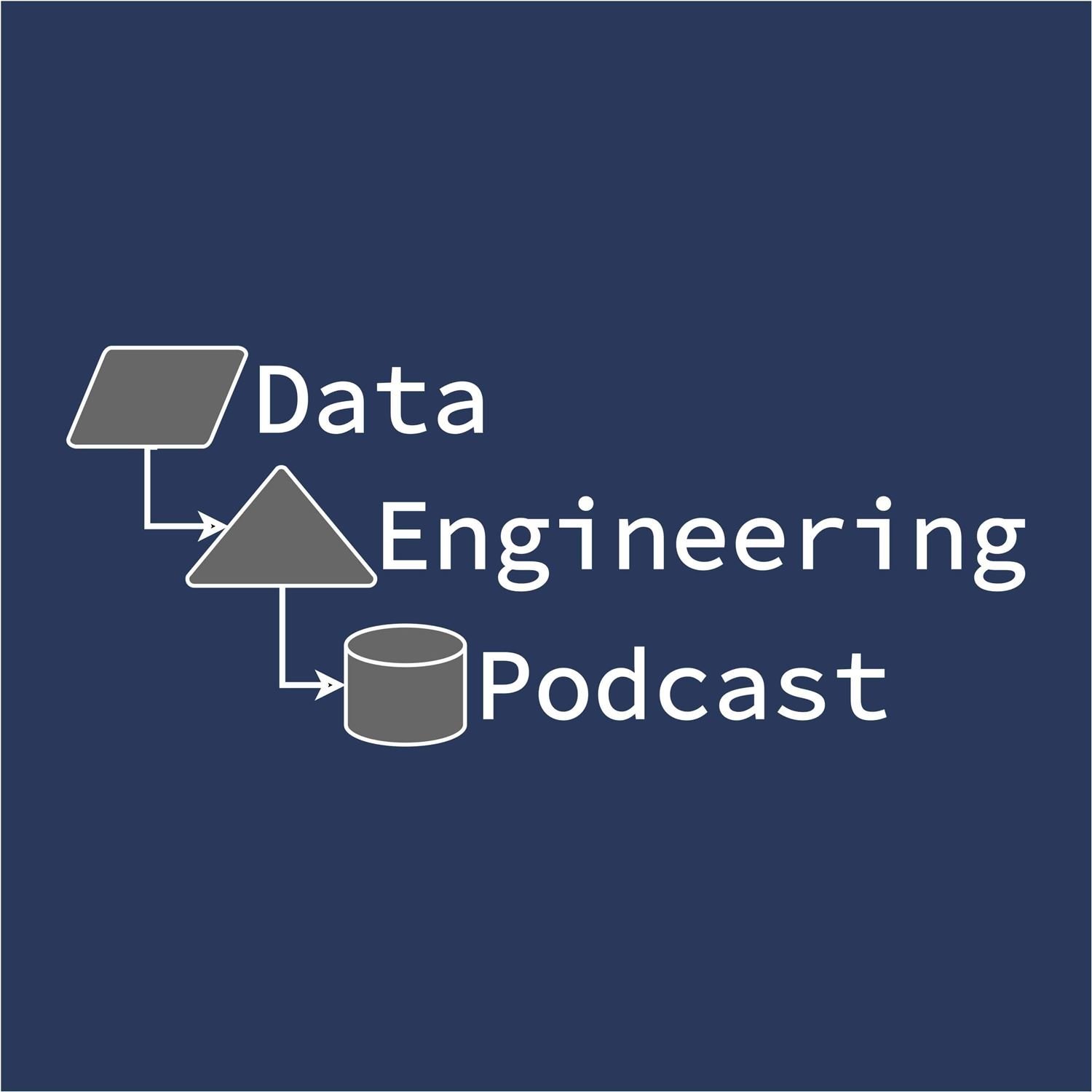
Barking Up The Wrong GPTree: Building Better AI With A Cognitive Approach
SummaryArtificial intelligence has dominated the headlines for several months due to the successes of large language models. This has prompted numerous debates about the possibility of, and timeline for, artificial general intelligence (AGI). Peter Voss has dedicated decades of his life to the pursuit of truly intelligent software through the approach of cognitive AI. In this episode he explains his approach to building AI in a more human-like fashion and the emphasis on learning rather than statistical prediction.AnnouncementsHello and welcome to the Data Engineering Podcast, the show about modern data managementDagster offers a new approach to building and running data platforms and data pipelines. It is an open-source, cloud-native orchestrator for the whole development lifecycle, with integrated lineage and observability, a declarative programming model, and best-in-class testability. Your team can get up and running in minutes thanks to Dagster Cloud, an enterprise-class hosted solution that offers serverless and hybrid deployments, enhanced security, and on-demand ephemeral test deployments. Go to dataengineeringpodcast.com/dagster today to get started. Your first 30 days are free!Data lakes are notoriously complex. For data engineers who battle to build and scale high quality data workflows on the data lake, Starburst powers petabyte-scale SQL analytics fast, at a fraction of the cost of traditional methods, so that you can meet all your data needs ranging from AI to data applications to complete analytics. Trusted by teams of all sizes, including Comcast and Doordash, Starburst is a data lake analytics platform that delivers the adaptability and flexibility a lakehouse ecosystem promises. And Starburst does all of this on an open architecture with first-class support for Apache Iceberg, Delta Lake and Hudi, so you always maintain ownership of your data. Want to see Starburst in action? Go to dataengineeringpodcast.com/starburst and get $500 in credits to try Starburst Galaxy today, the easiest and fastest way to get started using Trino.Your host is Tobias Macey and today I'm interviewing Peter Voss about what is involved in making your AI applications more "human"InterviewIntroductionHow did you get involved in machine learning?Can you start by unpacking the idea of "human-like" AI? How does that contrast with the conception of "AGI"?The applications and limitations of GPT/LLM models have been dominating the popular conversation around AI. How do you see that impacting the overrall ecosystem of ML/AI applications and investment?The fundamental/foundational challenge of every AI use case is sourcing appropriate data. What are the strategies that you have found useful to acquire, evaluate, and prepare data at an appropriate scale to build high quality models? What are the opportunities and limitations of causal modeling techniques for generalized AI models?As AI systems gain more sophistication there is a challenge with establishing and maintaining trust. What are the risks involved in deploying more human-level AI systems and monitoring their reliability?What are the practical/architectural methods necessary to build more cognitive AI systems? How would you characterize the ecosystem of tools/frameworks available for creating, evolving, and maintaining these applications?What are the most interesting, innovative, or unexpected ways that you have seen cognitive AI applied?What are the most interesting, unexpected, or challenging lessons that you have learned while working on desiging/developing cognitive AI systems?When is cognitive AI the wrong choice?What do you have planned for the future of cognitive AI applications at Aigo?Contact InfoLinkedInWebsiteParting QuestionFrom your perspective, what is the biggest barrier to adoption of machine learning today?Closing AnnouncementsThank you for listening! Don't forget to check out our other shows. Podcast.__init__ covers the Python language, its community, and the innovative ways it is being used. The Machine Learning Podcast helps you go from idea to production with machine learning.Visit the site to subscribe to the show, sign up for the mailing list, and read the show notes.If you've learned something or tried out a project from the show then tell us about it! Email [email protected]) with your story.LinksAigo.aiArtificial General IntelligenceCognitive AIKnowledge GraphCausal ModelingBayesian StatisticsThinking Fast & Slow by Daniel Kahneman (affiliate link)Agent-Based ModelingReinforcement LearningDARPA 3 Waves of AI presentationWhy Don't We Have AGI Yet? whitepaperConcepts Is All You Need WhitepaperHellen KellerStephen HawkingThe intro and outro music is from Hitman's Lovesong feat. Paola Graziano by The Freak Fandango Orchestra/CC BY-SA 3.0
54:1705/05/2024
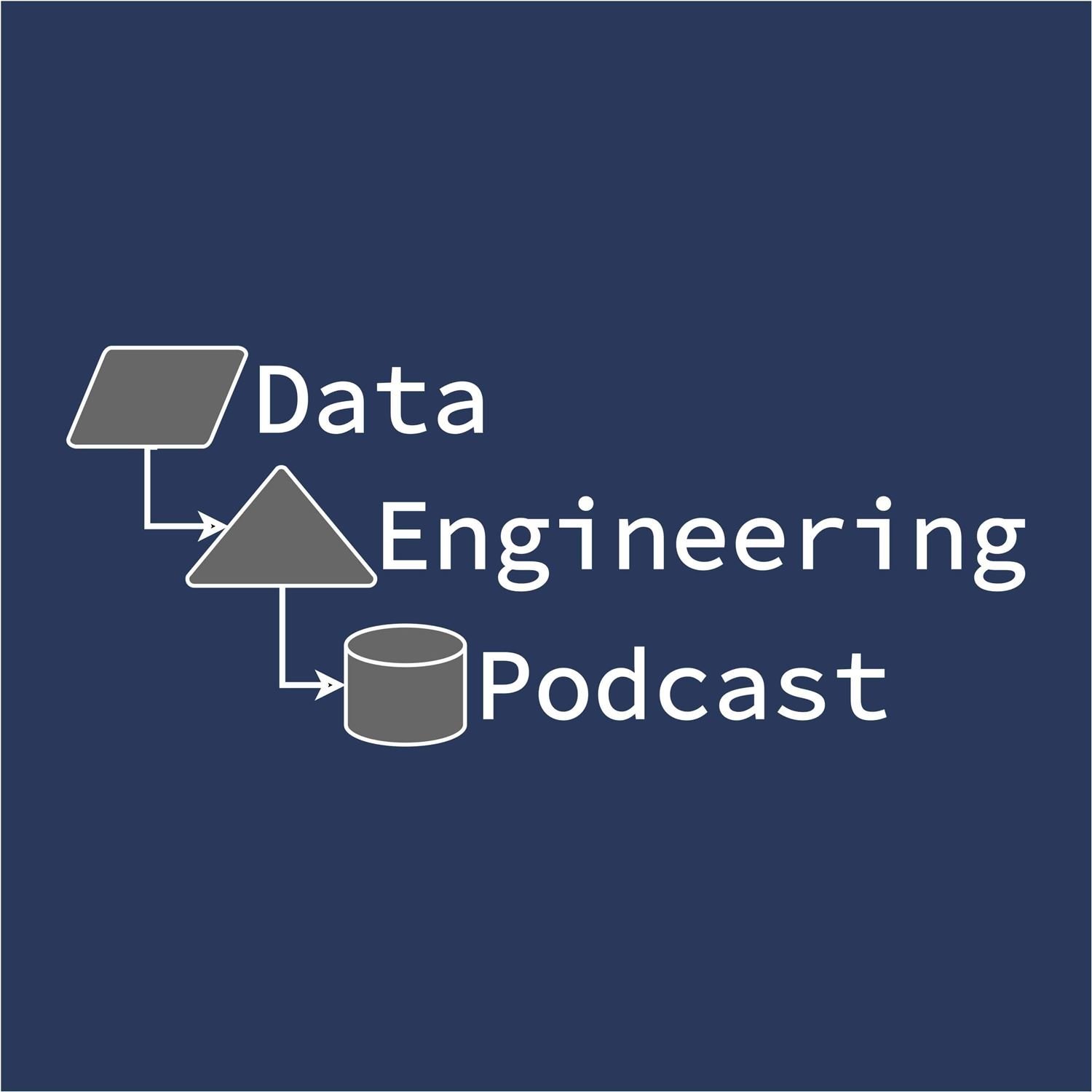
Build Your Second Brain One Piece At A Time
SummaryGenerative AI promises to accelerate the productivity of human collaborators. Currently the primary way of working with these tools is through a conversational prompt, which is often cumbersome and unwieldy. In order to simplify the integration of AI capabilities into developer workflows Tsavo Knott helped create Pieces, a powerful collection of tools that complements the tools that developers already use. In this episode he explains the data collection and preparation process, the collection of model types and sizes that work together to power the experience, and how to incorporate it into your workflow to act as a second brain.AnnouncementsHello and welcome to the Data Engineering Podcast, the show about modern data managementDagster offers a new approach to building and running data platforms and data pipelines. It is an open-source, cloud-native orchestrator for the whole development lifecycle, with integrated lineage and observability, a declarative programming model, and best-in-class testability. Your team can get up and running in minutes thanks to Dagster Cloud, an enterprise-class hosted solution that offers serverless and hybrid deployments, enhanced security, and on-demand ephemeral test deployments. Go to dataengineeringpodcast.com/dagster today to get started. Your first 30 days are free!Data lakes are notoriously complex. For data engineers who battle to build and scale high quality data workflows on the data lake, Starburst powers petabyte-scale SQL analytics fast, at a fraction of the cost of traditional methods, so that you can meet all your data needs ranging from AI to data applications to complete analytics. Trusted by teams of all sizes, including Comcast and Doordash, Starburst is a data lake analytics platform that delivers the adaptability and flexibility a lakehouse ecosystem promises. And Starburst does all of this on an open architecture with first-class support for Apache Iceberg, Delta Lake and Hudi, so you always maintain ownership of your data. Want to see Starburst in action? Go to dataengineeringpodcast.com/starburst and get $500 in credits to try Starburst Galaxy today, the easiest and fastest way to get started using Trino.Your host is Tobias Macey and today I'm interviewing Tsavo Knott about Pieces, a personal AI toolkit to improve the efficiency of developersInterviewIntroductionHow did you get involved in machine learning?Can you describe what Pieces is and the story behind it?The past few months have seen an endless series of personalized AI tools launched. What are the features and focus of Pieces that might encourage someone to use it over the alternatives?model selectionsarchitecture of Pieces applicationlocal vs. hybrid vs. online modelsmodel update/delivery processdata preparation/serving for models in context of Pieces appapplication of AI to developer workflowstypes of workflows that people are building with piecesWhat are the most interesting, innovative, or unexpected ways that you have seen Pieces used?What are the most interesting, unexpected, or challenging lessons that you have learned while working on Pieces?When is Pieces the wrong choice?What do you have planned for the future of Pieces?Contact InfoLinkedInParting QuestionFrom your perspective, what is the biggest barrier to adoption of machine learning today?Closing AnnouncementsThank you for listening! Don't forget to check out our other shows. Podcast.__init__ covers the Python language, its community, and the innovative ways it is being used. The Machine Learning Podcast helps you go from idea to production with machine learning.Visit the site to subscribe to the show, sign up for the mailing list, and read the show notes.If you've learned something or tried out a project from the show then tell us about it! Email [email protected]) with your story.LinksPiecesNPU == Neural Processing UnitTensor ChipLoRA == Low Rank AdaptationGenerative Adversarial NetworksMistralEmacsVimNeoVimDartFlutterTypescriptLuaRetrieval Augmented GenerationONNXLSTM == Long Short-Term MemoryLLama 2GitHub CopilotTabninePodcast EpisodeThe intro and outro music is from Hitman's Lovesong feat. Paola Graziano by The Freak Fandango Orchestra/CC BY-SA 3.0
50:1028/04/2024
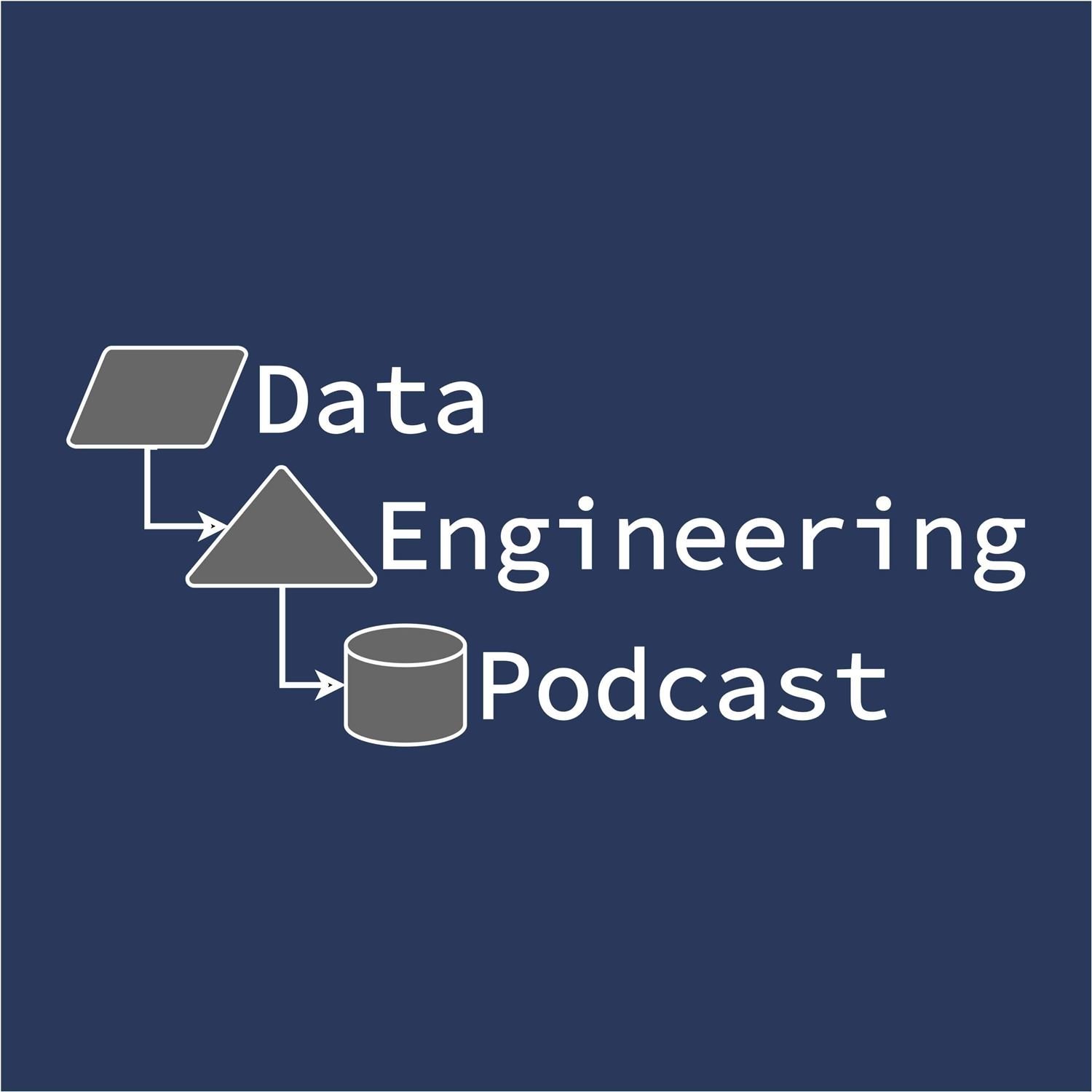
Making Email Better With AI At Shortwave
Summary
Generative AI has rapidly transformed everything in the technology sector. When Andrew Lee started work on Shortwave he was focused on making email more productive. When AI started gaining adoption he realized that he had even more potential for a transformative experience. In this episode he shares the technical challenges that he and his team have overcome in integrating AI into their product, as well as the benefits and features that it provides to their customers.
Announcements
Hello and welcome to the Data Engineering Podcast, the show about modern data management
Dagster offers a new approach to building and running data platforms and data pipelines. It is an open-source, cloud-native orchestrator for the whole development lifecycle, with integrated lineage and observability, a declarative programming model, and best-in-class testability. Your team can get up and running in minutes thanks to Dagster Cloud, an enterprise-class hosted solution that offers serverless and hybrid deployments, enhanced security, and on-demand ephemeral test deployments. Go to dataengineeringpodcast.com/dagster today to get started. Your first 30 days are free!
Data lakes are notoriously complex. For data engineers who battle to build and scale high quality data workflows on the data lake, Starburst powers petabyte-scale SQL analytics fast, at a fraction of the cost of traditional methods, so that you can meet all your data needs ranging from AI to data applications to complete analytics. Trusted by teams of all sizes, including Comcast and Doordash, Starburst is a data lake analytics platform that delivers the adaptability and flexibility a lakehouse ecosystem promises. And Starburst does all of this on an open architecture with first-class support for Apache Iceberg, Delta Lake and Hudi, so you always maintain ownership of your data. Want to see Starburst in action? Go to dataengineeringpodcast.com/starburst and get $500 in credits to try Starburst Galaxy today, the easiest and fastest way to get started using Trino.
Your host is Tobias Macey and today I'm interviewing Andrew Lee about his work on Shortwave, an AI powered email client
Interview
Introduction
How did you get involved in the area of data management?
Can you describe what Shortwave is and the story behind it?
What is the core problem that you are addressing with Shortwave?
Email has been a central part of communication and business productivity for decades now. What are the overall themes that continue to be problematic?
What are the strengths that email maintains as a protocol and ecosystem?
From a product perspective, what are the data challenges that are posed by email?
Can you describe how you have architected the Shortwave platform?
How have the design and goals of the product changed since you started it?
What are the ways that the advent and evolution of language models have influenced your product roadmap?
How do you manage the personalization of the AI functionality in your system for each user/team?
For users and teams who are using Shortwave, how does it change their workflow and communication patterns?
Can you describe how I would use Shortwave for managing the workflow of evaluating, planning, and promoting my podcast episodes?
What are the most interesting, innovative, or unexpected ways that you have seen Shortwave used?
What are the most interesting, unexpected, or challenging lessons that you have learned while working on Shortwave?
When is Shortwave the wrong choice?
What do you have planned for the future of Shortwave?
Contact Info
LinkedIn
Blog
Parting Question
From your perspective, what is the biggest gap in the tooling or technology for data management today?
Closing Announcements
Thank you for listening! Don't forget to check out our other shows. Podcast.__init__ covers the Python language, its community, and the innovative ways it is being used. The Machine Learning Podcast helps you go from idea to production with machine learning.
Visit the site to subscribe to the show, sign up for the mailing list, and read the show notes.
If you've learned something or tried out a project from the show then tell us about it! Email [email protected]) with your story.
Links
Shortwave
Firebase
Google Inbox
Hey
Ezra Klein Hey Article
Superhuman
Pinecone
Podcast Episode
Elastic
Hybrid Search
Semantic Search
Mistral
GPT 3.5
IMAP
The intro and outro music is from The Hug by The Freak Fandango Orchestra / CC BY-SASponsored By:Starburst: 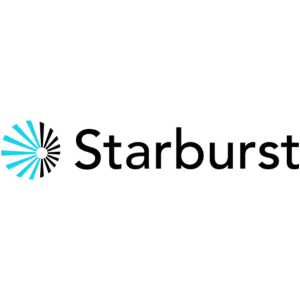
This episode is brought to you by Starburst - a data lake analytics platform for data engineers who are battling to build and scale high quality data pipelines on the data lake. Powered by Trino, Starburst runs petabyte-scale SQL analytics fast at a fraction of the cost of traditional methods, helping you meet all your data needs ranging from AI/ML workloads to data applications to complete analytics.
Trusted by the teams at Comcast and Doordash, Starburst delivers the adaptability and flexibility a lakehouse ecosystem promises, while providing a single point of access for your data and all your data governance allowing you to discover, transform, govern, and secure all in one place. Starburst does all of this on an open architecture with first-class support for Apache Iceberg, Delta Lake and Hudi, so you always maintain ownership of your data. Want to see Starburst in action? Try Starburst Galaxy today, the easiest and fastest way to get started using Trino, and get $500 of credits free. [dataengineeringpodcast.com/starburst](https://www.dataengineeringpodcast.com/starburst)Dagster: 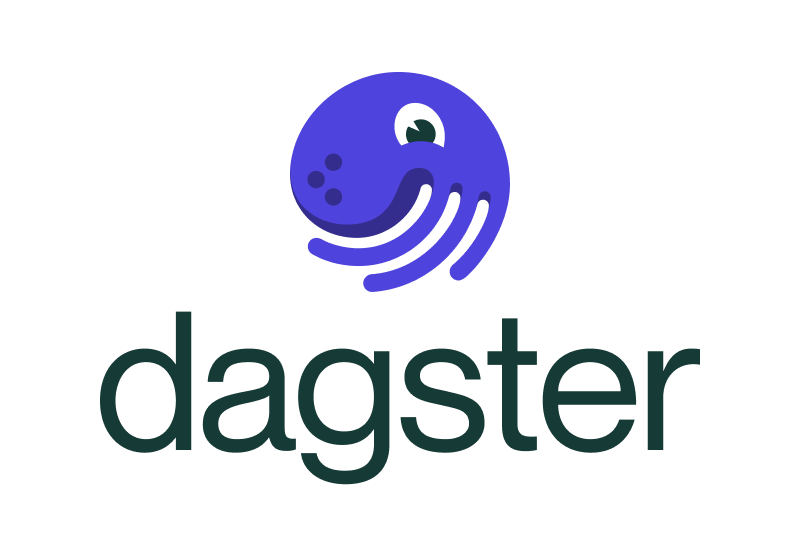
Data teams are tasked with helping organizations deliver on the premise of data, and with ML and AI maturing rapidly, expectations have never been this high. However data engineers are challenged by both technical complexity and organizational complexity, with heterogeneous technologies to adopt, multiple data disciplines converging, legacy systems to support, and costs to manage.
Dagster is an open-source orchestration solution that helps data teams reign in this complexity and build data platforms that provide unparalleled observability, and testability, all while fostering collaboration across the enterprise. With enterprise-grade hosting on Dagster Cloud, you gain even more capabilities, adding cost management, security, and CI support to further boost your teams' productivity. Go to [dagster.io](https://dagster.io/lp/dagster-cloud-trial?source=data-eng-podcast) today to get your first 30 days free!Support Data Engineering Podcast
53:4321/04/2024
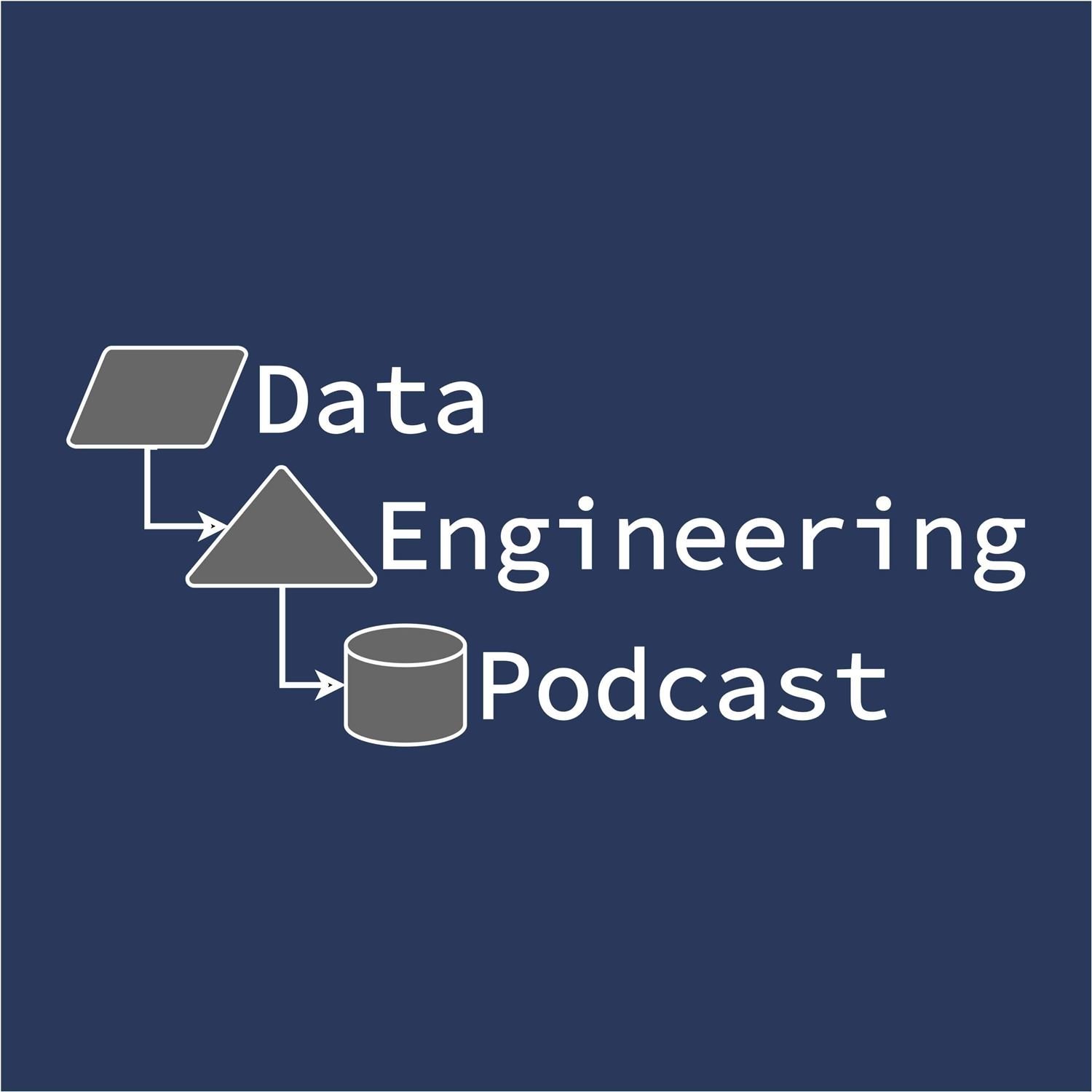
Designing A Non-Relational Database Engine
Summary
Databases come in a variety of formats for different use cases. The default association with the term "database" is relational engines, but non-relational engines are also used quite widely. In this episode Oren Eini, CEO and creator of RavenDB, explores the nuances of relational vs. non-relational engines, and the strategies for designing a non-relational database.
Announcements
Hello and welcome to the Data Engineering Podcast, the show about modern data management
This episode is brought to you by Datafold – a testing automation platform for data engineers that prevents data quality issues from entering every part of your data workflow, from migration to dbt deployment. Datafold has recently launched data replication testing, providing ongoing validation for source-to-target replication. Leverage Datafold's fast cross-database data diffing and Monitoring to test your replication pipelines automatically and continuously. Validate consistency between source and target at any scale, and receive alerts about any discrepancies. Learn more about Datafold by visiting dataengineeringpodcast.com/datafold.
Dagster offers a new approach to building and running data platforms and data pipelines. It is an open-source, cloud-native orchestrator for the whole development lifecycle, with integrated lineage and observability, a declarative programming model, and best-in-class testability. Your team can get up and running in minutes thanks to Dagster Cloud, an enterprise-class hosted solution that offers serverless and hybrid deployments, enhanced security, and on-demand ephemeral test deployments. Go to dataengineeringpodcast.com/dagster today to get started. Your first 30 days are free!
Data lakes are notoriously complex. For data engineers who battle to build and scale high quality data workflows on the data lake, Starburst powers petabyte-scale SQL analytics fast, at a fraction of the cost of traditional methods, so that you can meet all your data needs ranging from AI to data applications to complete analytics. Trusted by teams of all sizes, including Comcast and Doordash, Starburst is a data lake analytics platform that delivers the adaptability and flexibility a lakehouse ecosystem promises. And Starburst does all of this on an open architecture with first-class support for Apache Iceberg, Delta Lake and Hudi, so you always maintain ownership of your data. Want to see Starburst in action? Go to dataengineeringpodcast.com/starburst and get $500 in credits to try Starburst Galaxy today, the easiest and fastest way to get started using Trino.
Your host is Tobias Macey and today I'm interviewing Oren Eini about the work of designing and building a NoSQL database engine
Interview
Introduction
How did you get involved in the area of data management?
Can you describe what constitutes a NoSQL database?
How have the requirements and applications of NoSQL engines changed since they first became popular ~15 years ago?
What are the factors that convince teams to use a NoSQL vs. SQL database?
NoSQL is a generalized term that encompasses a number of different data models. How does the underlying representation (e.g. document, K/V, graph) change that calculus?
How have the evolution in data formats (e.g. N-dimensional vectors, point clouds, etc.) changed the landscape for NoSQL engines?
When designing and building a database, what are the initial set of questions that need to be answered?
How many "core capabilities" can you reasonably design around before they conflict with each other?
How have you approached the evolution of RavenDB as you add new capabilities and mature the project?
What are some of the early decisions that had to be unwound to enable new capabilities?
If you were to start from scratch today, what database would you build?
What are the most interesting, innovative, or unexpected ways that you have seen RavenDB/NoSQL databases used?
What are the most interesting, unexpected, or challenging lessons that you have learned while working on RavenDB?
When is a NoSQL database/RavenDB the wrong choice?
What do you have planned for the future of RavenDB?
Contact Info
Blog
LinkedIn
Parting Question
From your perspective, what is the biggest gap in the tooling or technology for data management today?
Closing Announcements
Thank you for listening! Don't forget to check out our other shows. Podcast.__init__ covers the Python language, its community, and the innovative ways it is being used. The Machine Learning Podcast helps you go from idea to production with machine learning.
Visit the site to subscribe to the show, sign up for the mailing list, and read the show notes.
If you've learned something or tried out a project from the show then tell us about it! Email [email protected]) with your story.
Links
RavenDB
RSS
Object Relational Mapper (ORM)
Relational Database
NoSQL
CouchDB
Navigational Database
MongoDB
Redis
Neo4J
Cassandra
Column-Family
SQLite
LevelDB
Firebird DB
fsync
Esent DB?
KNN == K-Nearest Neighbors
RocksDB
C# Language
ASP.NET
QUIC
Dynamo Paper
Database Internals book (affiliate link)
Designing Data Intensive Applications book (affiliate link)
The intro and outro music is from The Hug by The Freak Fandango Orchestra / CC BY-SASponsored By:Starburst: 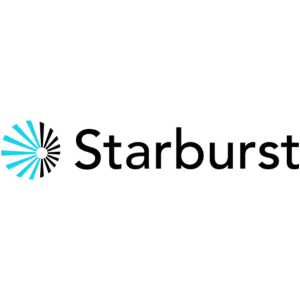
This episode is brought to you by Starburst - a data lake analytics platform for data engineers who are battling to build and scale high quality data pipelines on the data lake. Powered by Trino, Starburst runs petabyte-scale SQL analytics fast at a fraction of the cost of traditional methods, helping you meet all your data needs ranging from AI/ML workloads to data applications to complete analytics.
Trusted by the teams at Comcast and Doordash, Starburst delivers the adaptability and flexibility a lakehouse ecosystem promises, while providing a single point of access for your data and all your data governance allowing you to discover, transform, govern, and secure all in one place. Starburst does all of this on an open architecture with first-class support for Apache Iceberg, Delta Lake and Hudi, so you always maintain ownership of your data. Want to see Starburst in action? Try Starburst Galaxy today, the easiest and fastest way to get started using Trino, and get $500 of credits free. [dataengineeringpodcast.com/starburst](https://www.dataengineeringpodcast.com/starburst)Datafold: 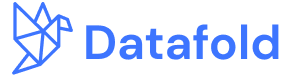
This episode is brought to you by Datafold – a testing automation platform for data engineers that prevents data quality issues from entering every part of your data workflow, from migration to dbt deployment. Datafold has recently launched data replication testing, providing ongoing validation for source-to-target replication. Leverage Datafold's fast cross-database data diffing and Monitoring to test your replication pipelines automatically and continuously. Validate consistency between source and target at any scale, and receive alerts about any discrepancies. Learn more about Datafold by visiting https://get.datafold.com/replication-de-podcast.Dagster: 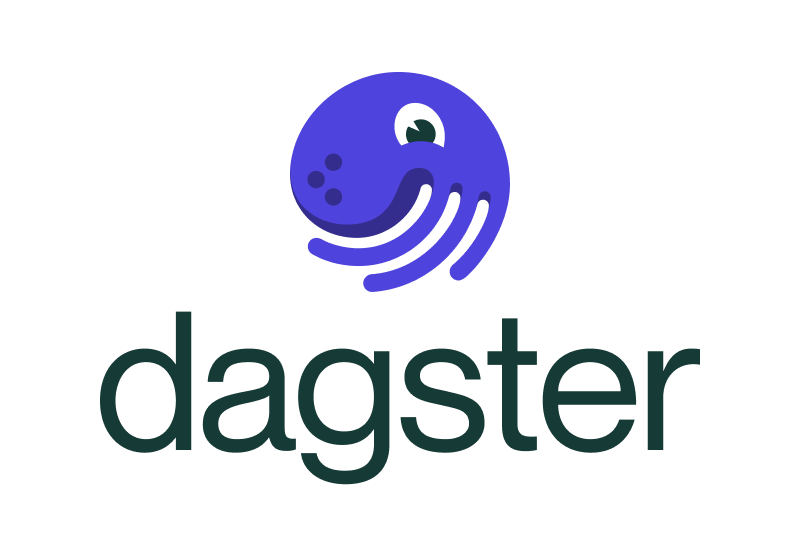
Data teams are tasked with helping organizations deliver on the premise of data, and with ML and AI maturing rapidly, expectations have never been this high. However data engineers are challenged by both technical complexity and organizational complexity, with heterogeneous technologies to adopt, multiple data disciplines converging, legacy systems to support, and costs to manage.
Dagster is an open-source orchestration solution that helps data teams reign in this complexity and build data platforms that provide unparalleled observability, and testability, all while fostering collaboration across the enterprise. With enterprise-grade hosting on Dagster Cloud, you gain even more capabilities, adding cost management, security, and CI support to further boost your teams' productivity. Go to [dagster.io](https://dagster.io/lp/dagster-cloud-trial?source=data-eng-podcast) today to get your first 30 days free!Support Data Engineering Podcast
01:16:0214/04/2024
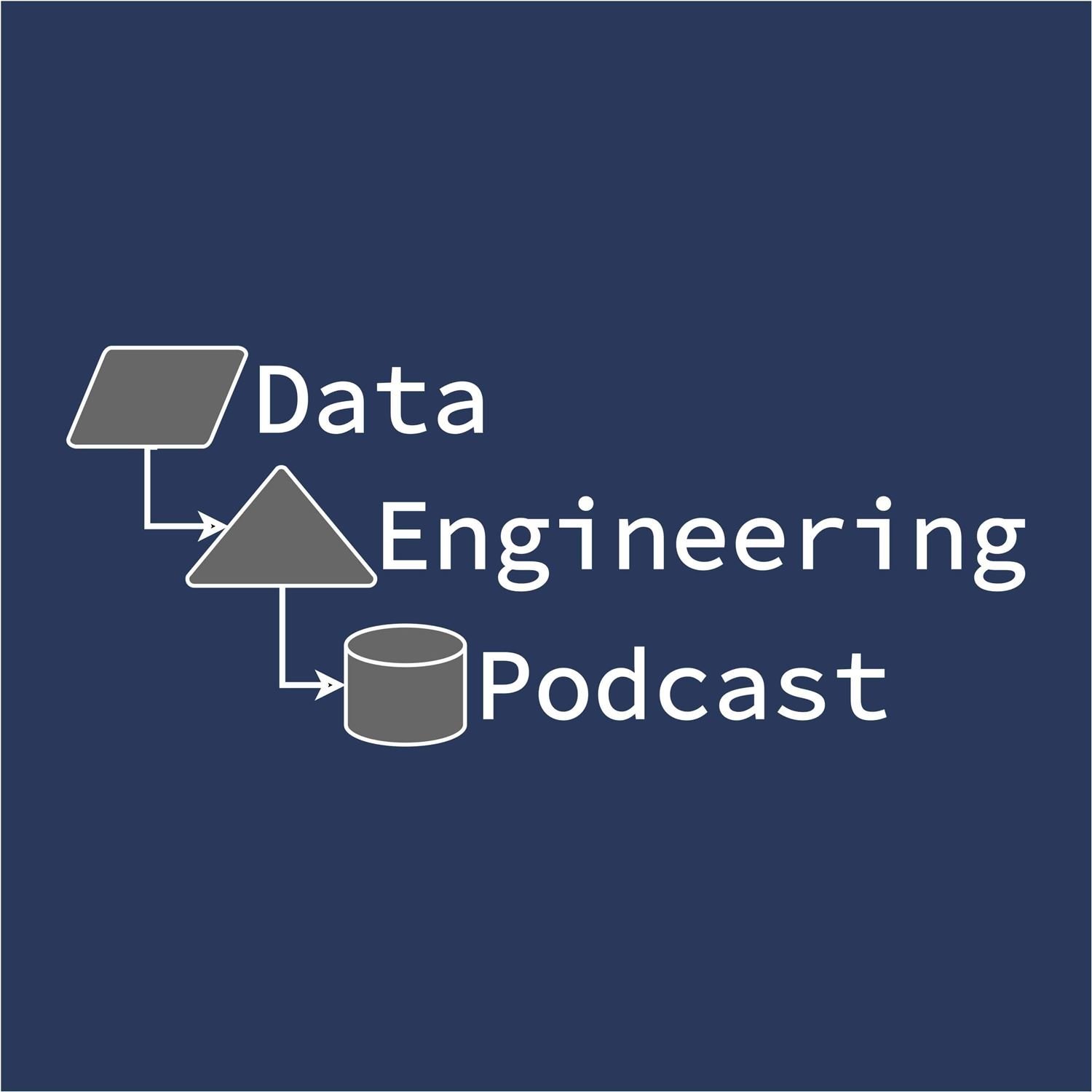
Establish A Single Source Of Truth For Your Data Consumers With A Semantic Layer
Summary
Maintaining a single source of truth for your data is the biggest challenge in data engineering. Different roles and tasks in the business need their own ways to access and analyze the data in the organization. In order to enable this use case, while maintaining a single point of access, the semantic layer has evolved as a technological solution to the problem. In this episode Artyom Keydunov, creator of Cube, discusses the evolution and applications of the semantic layer as a component of your data platform, and how Cube provides speed and cost optimization for your data consumers.
Announcements
Hello and welcome to the Data Engineering Podcast, the show about modern data management
This episode is brought to you by Datafold – a testing automation platform for data engineers that prevents data quality issues from entering every part of your data workflow, from migration to dbt deployment. Datafold has recently launched data replication testing, providing ongoing validation for source-to-target replication. Leverage Datafold's fast cross-database data diffing and Monitoring to test your replication pipelines automatically and continuously. Validate consistency between source and target at any scale, and receive alerts about any discrepancies. Learn more about Datafold by visiting dataengineeringpodcast.com/datafold.
Dagster offers a new approach to building and running data platforms and data pipelines. It is an open-source, cloud-native orchestrator for the whole development lifecycle, with integrated lineage and observability, a declarative programming model, and best-in-class testability. Your team can get up and running in minutes thanks to Dagster Cloud, an enterprise-class hosted solution that offers serverless and hybrid deployments, enhanced security, and on-demand ephemeral test deployments. Go to dataengineeringpodcast.com/dagster today to get started. Your first 30 days are free!
Data lakes are notoriously complex. For data engineers who battle to build and scale high quality data workflows on the data lake, Starburst powers petabyte-scale SQL analytics fast, at a fraction of the cost of traditional methods, so that you can meet all your data needs ranging from AI to data applications to complete analytics. Trusted by teams of all sizes, including Comcast and Doordash, Starburst is a data lake analytics platform that delivers the adaptability and flexibility a lakehouse ecosystem promises. And Starburst does all of this on an open architecture with first-class support for Apache Iceberg, Delta Lake and Hudi, so you always maintain ownership of your data. Want to see Starburst in action? Go to dataengineeringpodcast.com/starburst and get $500 in credits to try Starburst Galaxy today, the easiest and fastest way to get started using Trino.
Your host is Tobias Macey and today I'm interviewing Artyom Keydunov about the role of the semantic layer in your data platform
Interview
Introduction
How did you get involved in the area of data management?
Can you start by outlining the technical elements of what it means to have a "semantic layer"?
In the past couple of years there was a rapid hype cycle around the "metrics layer" and "headless BI", which has largely faded. Can you give your assessment of the current state of the industry around the adoption/implementation of these concepts?
What are the benefits of having a discrete service that offers the business metrics/semantic mappings as opposed to implementing those concepts as part of a more general system? (e.g. dbt, BI, warehouse marts, etc.)
At what point does it become necessary/beneficial for a team to adopt such a service?
What are the challenges involved in retrofitting a semantic layer into a production data system?
evolution of requirements/usage patterns
technical complexities/performance and cost optimization
What are the most interesting, innovative, or unexpected ways that you have seen Cube used?
What are the most interesting, unexpected, or challenging lessons that you have learned while working on Cube?
When is Cube/a semantic layer the wrong choice?
What do you have planned for the future of Cube?
Contact Info
LinkedIn
keydunov on GitHub
Parting Question
From your perspective, what is the biggest gap in the tooling or technology for data management today?
Closing Announcements
Thank you for listening! Don't forget to check out our other shows. Podcast.__init__ covers the Python language, its community, and the innovative ways it is being used. The Machine Learning Podcast helps you go from idea to production with machine learning.
Visit the site to subscribe to the show, sign up for the mailing list, and read the show notes.
If you've learned something or tried out a project from the show then tell us about it! Email [email protected]) with your story.
Links
Cube
Semantic Layer
Business Objects
Tableau
Looker
Podcast Episode
Mode
Thoughtspot
LightDash
Podcast Episode
Embedded Analytics
Dimensional Modeling
Clickhouse
Podcast Episode
Druid
BigQuery
Starburst
Pinot
Snowflake
Podcast Episode
Arrow Datafusion
Metabase
Podcast Episode
Superset
Alation
Collibra
Podcast Episode
Atlan
Podcast Episode
The intro and outro music is from The Hug by The Freak Fandango Orchestra / CC BY-SASponsored By:Starburst: 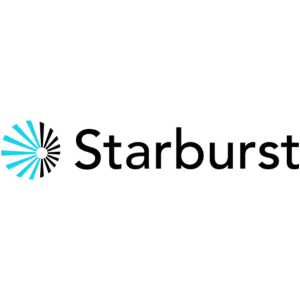
This episode is brought to you by Starburst - a data lake analytics platform for data engineers who are battling to build and scale high quality data pipelines on the data lake. Powered by Trino, Starburst runs petabyte-scale SQL analytics fast at a fraction of the cost of traditional methods, helping you meet all your data needs ranging from AI/ML workloads to data applications to complete analytics.
Trusted by the teams at Comcast and Doordash, Starburst delivers the adaptability and flexibility a lakehouse ecosystem promises, while providing a single point of access for your data and all your data governance allowing you to discover, transform, govern, and secure all in one place. Starburst does all of this on an open architecture with first-class support for Apache Iceberg, Delta Lake and Hudi, so you always maintain ownership of your data. Want to see Starburst in action? Try Starburst Galaxy today, the easiest and fastest way to get started using Trino, and get $500 of credits free. [dataengineeringpodcast.com/starburst](https://www.dataengineeringpodcast.com/starburst)Datafold: 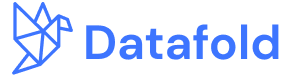
This episode is brought to you by Datafold – a testing automation platform for data engineers that prevents data quality issues from entering every part of your data workflow, from migration to dbt deployment. Datafold has recently launched data replication testing, providing ongoing validation for source-to-target replication. Leverage Datafold's fast cross-database data diffing and Monitoring to test your replication pipelines automatically and continuously. Validate consistency between source and target at any scale, and receive alerts about any discrepancies. Learn more about Datafold by visiting https://get.datafold.com/replication-de-podcast.Dagster: 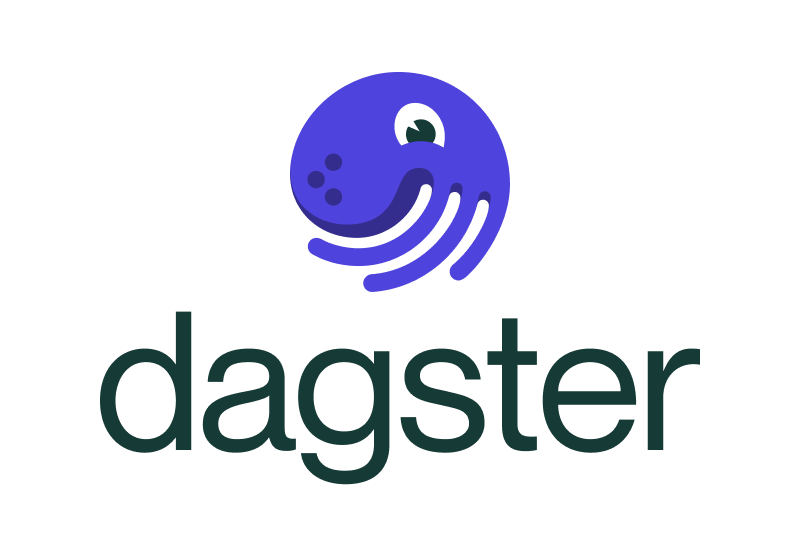
Data teams are tasked with helping organizations deliver on the premise of data, and with ML and AI maturing rapidly, expectations have never been this high. However data engineers are challenged by both technical complexity and organizational complexity, with heterogeneous technologies to adopt, multiple data disciplines converging, legacy systems to support, and costs to manage.
Dagster is an open-source orchestration solution that helps data teams reign in this complexity and build data platforms that provide unparalleled observability, and testability, all while fostering collaboration across the enterprise. With enterprise-grade hosting on Dagster Cloud, you gain even more capabilities, adding cost management, security, and CI support to further boost your teams' productivity. Go to [dagster.io](https://dagster.io/lp/dagster-cloud-trial?source=data-eng-podcast) today to get your first 30 days free!Support Data Engineering Podcast
56:2307/04/2024
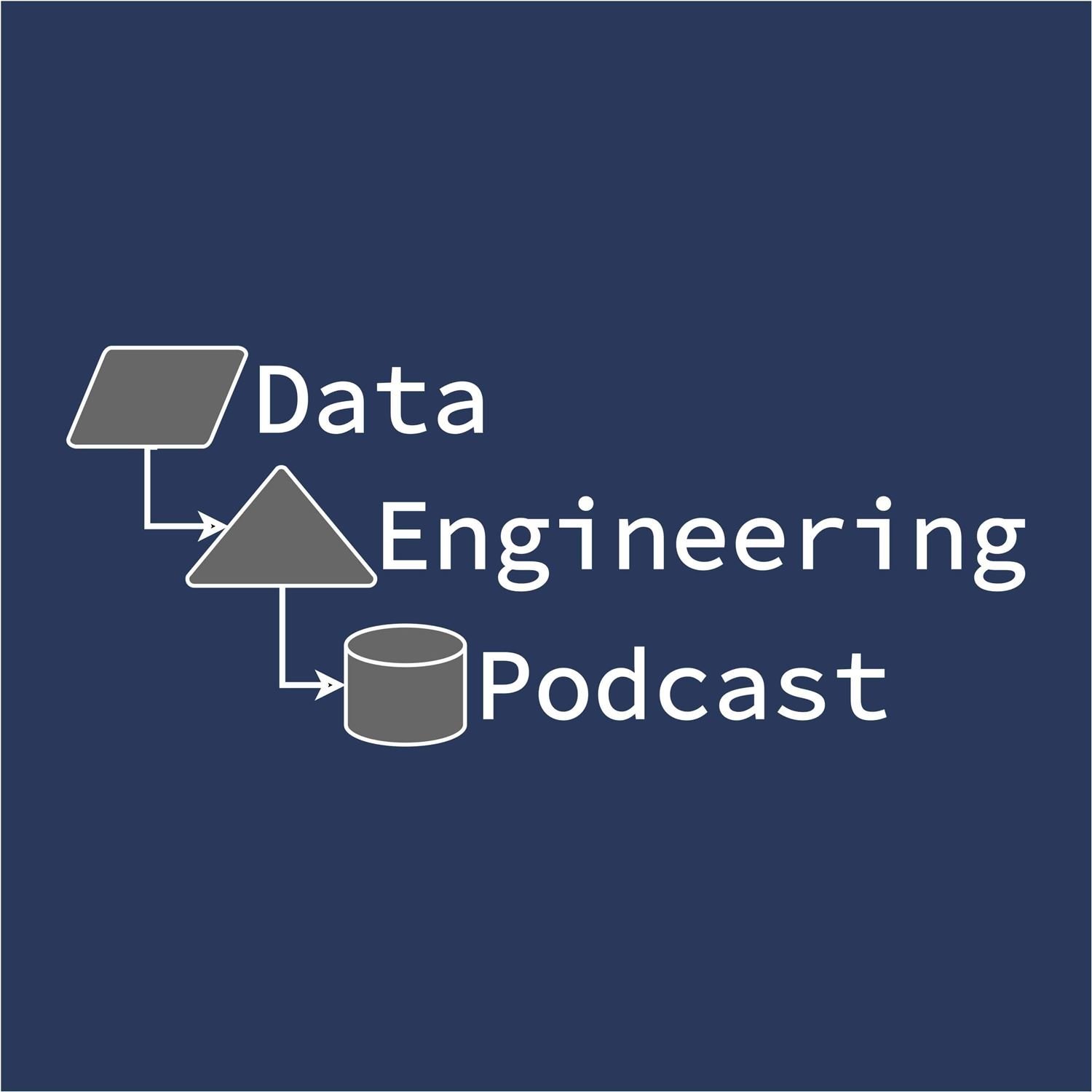
Adding Anomaly Detection And Observability To Your dbt Projects Is Elementary
Summary
Working with data is a complicated process, with numerous chances for something to go wrong. Identifying and accounting for those errors is a critical piece of building trust in the organization that your data is accurate and up to date. While there are numerous products available to provide that visibility, they all have different technologies and workflows that they focus on. To bring observability to dbt projects the team at Elementary embedded themselves into the workflow. In this episode Maayan Salom explores the approach that she has taken to bring observability, enhanced testing capabilities, and anomaly detection into every step of the dbt developer experience.
Announcements
Hello and welcome to the Data Engineering Podcast, the show about modern data management
Data lakes are notoriously complex. For data engineers who battle to build and scale high quality data workflows on the data lake, Starburst powers petabyte-scale SQL analytics fast, at a fraction of the cost of traditional methods, so that you can meet all your data needs ranging from AI to data applications to complete analytics. Trusted by teams of all sizes, including Comcast and Doordash, Starburst is a data lake analytics platform that delivers the adaptability and flexibility a lakehouse ecosystem promises. And Starburst does all of this on an open architecture with first-class support for Apache Iceberg, Delta Lake and Hudi, so you always maintain ownership of your data. Want to see Starburst in action? Go to dataengineeringpodcast.com/starburst and get $500 in credits to try Starburst Galaxy today, the easiest and fastest way to get started using Trino.
Dagster offers a new approach to building and running data platforms and data pipelines. It is an open-source, cloud-native orchestrator for the whole development lifecycle, with integrated lineage and observability, a declarative programming model, and best-in-class testability. Your team can get up and running in minutes thanks to Dagster Cloud, an enterprise-class hosted solution that offers serverless and hybrid deployments, enhanced security, and on-demand ephemeral test deployments. Go to dataengineeringpodcast.com/dagster today to get started. Your first 30 days are free!
This episode is brought to you by Datafold – a testing automation platform for data engineers that prevents data quality issues from entering every part of your data workflow, from migration to dbt deployment. Datafold has recently launched data replication testing, providing ongoing validation for source-to-target replication. Leverage Datafold's fast cross-database data diffing and Monitoring to test your replication pipelines automatically and continuously. Validate consistency between source and target at any scale, and receive alerts about any discrepancies. Learn more about Datafold by visiting dataengineeringpodcast.com/datafold.
Your host is Tobias Macey and today I'm interviewing Maayan Salom about how to incorporate observability into a dbt-oriented workflow and how Elementary can help
Interview
Introduction
How did you get involved in the area of data management?
Can you start by outlining what elements of observability are most relevant for dbt projects?
What are some of the common ad-hoc/DIY methods that teams develop to acquire those insights?
What are the challenges/shortcomings associated with those approaches?
Over the past ~3 years there were numerous data observability systems/products created. What are some of the ways that the specifics of dbt workflows are not covered by those generalized tools?
What are the insights that can be more easily generated by embedding into the dbt toolchain and development cycle?
Can you describe what Elementary is and how it is designed to enhance the development and maintenance work in dbt projects?
How is Elementary designed/implemented?
How have the scope and goals of the project changed since you started working on it?
What are the engineering challenges/frustrations that you have dealt with in the creation and evolution of Elementary?
Can you talk us through the setup and workflow for teams adopting Elementary in their dbt projects?
How does the incorporation of Elementary change the development habits of the teams who are using it?
What are the most interesting, innovative, or unexpected ways that you have seen Elementary used?
What are the most interesting, unexpected, or challenging lessons that you have learned while working on Elementary?
When is Elementary the wrong choice?
What do you have planned for the future of Elementary?
Contact Info
LinkedIn
Parting Question
From your perspective, what is the biggest gap in the tooling or technology for data management today?
Closing Announcements
Thank you for listening! Don't forget to check out our other shows. Podcast.__init__ covers the Python language, its community, and the innovative ways it is being used. The Machine Learning Podcast helps you go from idea to production with machine learning.
Visit the site to subscribe to the show, sign up for the mailing list, and read the show notes.
If you've learned something or tried out a project from the show then tell us about it! Email [email protected]) with your story.
Links
Elementary
Data Observability
dbt
Datadog
pre-commit
dbt packages
SQLMesh
Malloy
SDF
The intro and outro music is from The Hug by The Freak Fandango Orchestra / CC BY-SASponsored By:Starburst: 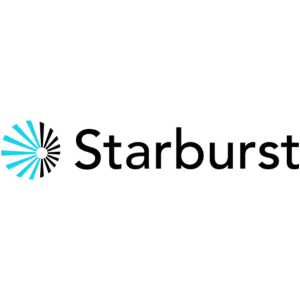
This episode is brought to you by Starburst - a data lake analytics platform for data engineers who are battling to build and scale high quality data pipelines on the data lake. Powered by Trino, Starburst runs petabyte-scale SQL analytics fast at a fraction of the cost of traditional methods, helping you meet all your data needs ranging from AI/ML workloads to data applications to complete analytics.
Trusted by the teams at Comcast and Doordash, Starburst delivers the adaptability and flexibility a lakehouse ecosystem promises, while providing a single point of access for your data and all your data governance allowing you to discover, transform, govern, and secure all in one place. Starburst does all of this on an open architecture with first-class support for Apache Iceberg, Delta Lake and Hudi, so you always maintain ownership of your data. Want to see Starburst in action? Try Starburst Galaxy today, the easiest and fastest way to get started using Trino, and get $500 of credits free. [dataengineeringpodcast.com/starburst](https://www.dataengineeringpodcast.com/starburst)Datafold: 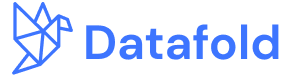
This episode is brought to you by Datafold – a testing automation platform for data engineers that prevents data quality issues from entering every part of your data workflow, from migration to dbt deployment. Datafold has recently launched data replication testing, providing ongoing validation for source-to-target replication. Leverage Datafold's fast cross-database data diffing and Monitoring to test your replication pipelines automatically and continuously. Validate consistency between source and target at any scale, and receive alerts about any discrepancies. Learn more about Datafold by visiting https://get.datafold.com/replication-de-podcast.Dagster: 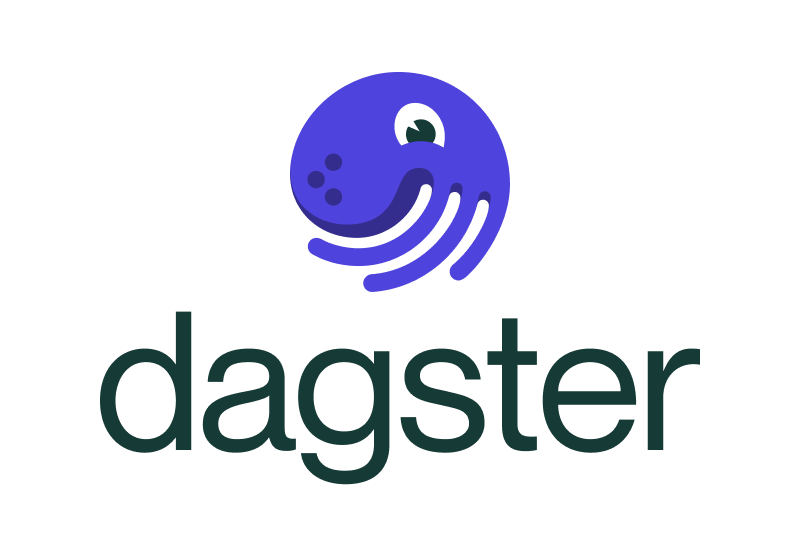
Data teams are tasked with helping organizations deliver on the premise of data, and with ML and AI maturing rapidly, expectations have never been this high. However data engineers are challenged by both technical complexity and organizational complexity, with heterogeneous technologies to adopt, multiple data disciplines converging, legacy systems to support, and costs to manage.
Dagster is an open-source orchestration solution that helps data teams reign in this complexity and build data platforms that provide unparalleled observability, and testability, all while fostering collaboration across the enterprise. With enterprise-grade hosting on Dagster Cloud, you gain even more capabilities, adding cost management, security, and CI support to further boost your teams' productivity. Go to [dagster.io](https://dagster.io/lp/dagster-cloud-trial?source=data-eng-podcast) today to get your first 30 days free!Support Data Engineering Podcast
50:4431/03/2024
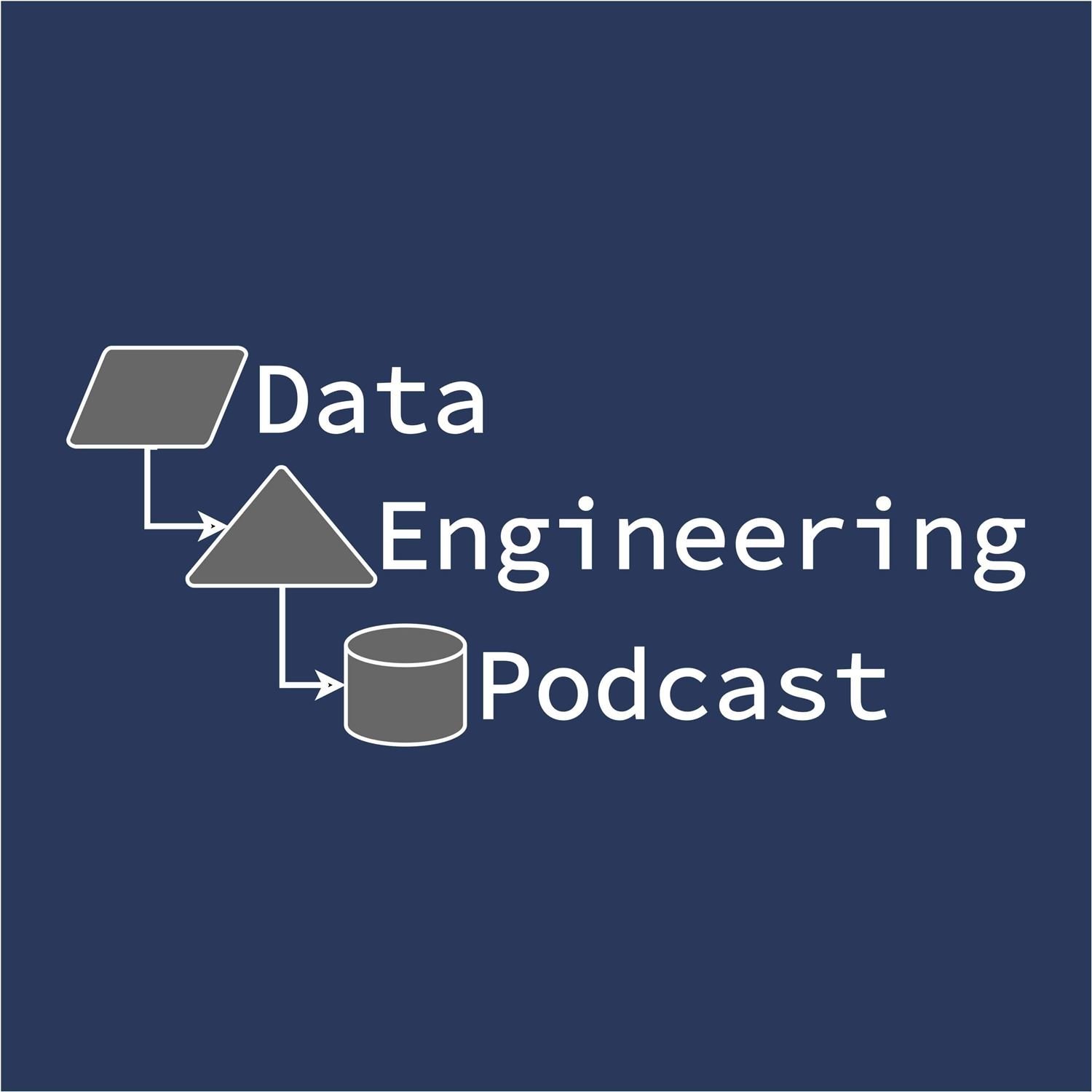
Ship Smarter Not Harder With Declarative And Collaborative Data Orchestration On Dagster+
Summary
A core differentiator of Dagster in the ecosystem of data orchestration is their focus on software defined assets as a means of building declarative workflows. With their launch of Dagster+ as the redesigned commercial companion to the open source project they are investing in that capability with a suite of new features. In this episode Pete Hunt, CEO of Dagster labs, outlines these new capabilities, how they reduce the burden on data teams, and the increased collaboration that they enable across teams and business units.
Announcements
Hello and welcome to the Data Engineering Podcast, the show about modern data management
Dagster offers a new approach to building and running data platforms and data pipelines. It is an open-source, cloud-native orchestrator for the whole development lifecycle, with integrated lineage and observability, a declarative programming model, and best-in-class testability. Your team can get up and running in minutes thanks to Dagster Cloud, an enterprise-class hosted solution that offers serverless and hybrid deployments, enhanced security, and on-demand ephemeral test deployments. Go to dataengineeringpodcast.com/dagster today to get started. Your first 30 days are free!
Data lakes are notoriously complex. For data engineers who battle to build and scale high quality data workflows on the data lake, Starburst powers petabyte-scale SQL analytics fast, at a fraction of the cost of traditional methods, so that you can meet all your data needs ranging from AI to data applications to complete analytics. Trusted by teams of all sizes, including Comcast and Doordash, Starburst is a data lake analytics platform that delivers the adaptability and flexibility a lakehouse ecosystem promises. And Starburst does all of this on an open architecture with first-class support for Apache Iceberg, Delta Lake and Hudi, so you always maintain ownership of your data. Want to see Starburst in action? Go to dataengineeringpodcast.com/starburst and get $500 in credits to try Starburst Galaxy today, the easiest and fastest way to get started using Trino.
Your host is Tobias Macey and today I'm interviewing Pete Hunt about how the launch of Dagster+ will level up your data platform and orchestrate across language platforms
Interview
Introduction
How did you get involved in the area of data management?
Can you describe what the focus of Dagster+ is and the story behind it?
What problems are you trying to solve with Dagster+?
What are the notable enhancements beyond the Dagster Core project that this updated platform provides?
How is it different from the current Dagster Cloud product?
In the launch announcement you tease new capabilities that would be great to explore in turns:
Make data a team sport, enabling data teams across the organization
Deliver reliable, high quality data the organization can trust
Observe and manage data platform costs
Master the heterogeneous collection of technologies—both traditional and Modern Data Stack
What are the business/product goals that you are focused on improving with the launch of Dagster+
What are the most interesting, innovative, or unexpected ways that you have seen Dagster used?
What are the most interesting, unexpected, or challenging lessons that you have learned while working on the design and launch of Dagster+?
When is Dagster+ the wrong choice?
What do you have planned for the future of Dagster/Dagster Cloud/Dagster+?
Contact Info
Twitter
LinkedIn
Parting Question
From your perspective, what is the biggest gap in the tooling or technology for data management today?
Closing Announcements
Thank you for listening! Don't forget to check out our other shows. Podcast.__init__ covers the Python language, its community, and the innovative ways it is being used. The Machine Learning Podcast helps you go from idea to production with machine learning.
Visit the site to subscribe to the show, sign up for the mailing list, and read the show notes.
If you've learned something or tried out a project from the show then tell us about it! Email [email protected]) with your story.
Links
Dagster
Podcast Episode
Dagster+ Launch Event
Hadoop
MapReduce
Pydantic
Software Defined Assets
Dagster Insights
Dagster Pipes
Conway's Law
Data Mesh
Dagster Code Locations
Dagster Asset Checks
Dave & Buster's
SQLMesh
Podcast Episode
SDF
Malloy
The intro and outro music is from The Hug by The Freak Fandango Orchestra / CC BY-SASponsored By:Starburst: 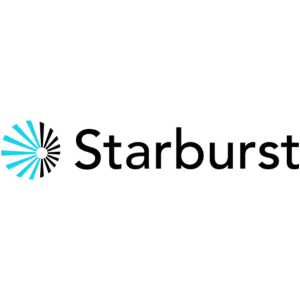
This episode is brought to you by Starburst - a data lake analytics platform for data engineers who are battling to build and scale high quality data pipelines on the data lake. Powered by Trino, Starburst runs petabyte-scale SQL analytics fast at a fraction of the cost of traditional methods, helping you meet all your data needs ranging from AI/ML workloads to data applications to complete analytics.
Trusted by the teams at Comcast and Doordash, Starburst delivers the adaptability and flexibility a lakehouse ecosystem promises, while providing a single point of access for your data and all your data governance allowing you to discover, transform, govern, and secure all in one place. Starburst does all of this on an open architecture with first-class support for Apache Iceberg, Delta Lake and Hudi, so you always maintain ownership of your data. Want to see Starburst in action? Try Starburst Galaxy today, the easiest and fastest way to get started using Trino, and get $500 of credits free. [dataengineeringpodcast.com/starburst](https://www.dataengineeringpodcast.com/starburst)Dagster: 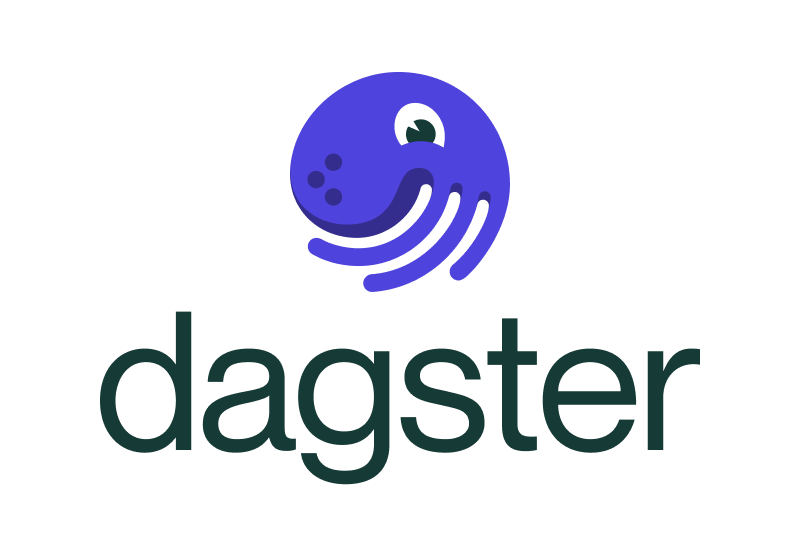
Data teams are tasked with helping organizations deliver on the premise of data, and with ML and AI maturing rapidly, expectations have never been this high. However data engineers are challenged by both technical complexity and organizational complexity, with heterogeneous technologies to adopt, multiple data disciplines converging, legacy systems to support, and costs to manage.
Dagster is an open-source orchestration solution that helps data teams reign in this complexity and build data platforms that provide unparalleled observability, and testability, all while fostering collaboration across the enterprise. With enterprise-grade hosting on Dagster Cloud, you gain even more capabilities, adding cost management, security, and CI support to further boost your teams' productivity. Go to [dagster.io](https://dagster.io/lp/dagster-cloud-trial?source=data-eng-podcast) today to get your first 30 days free!Support Data Engineering Podcast
55:4024/03/2024
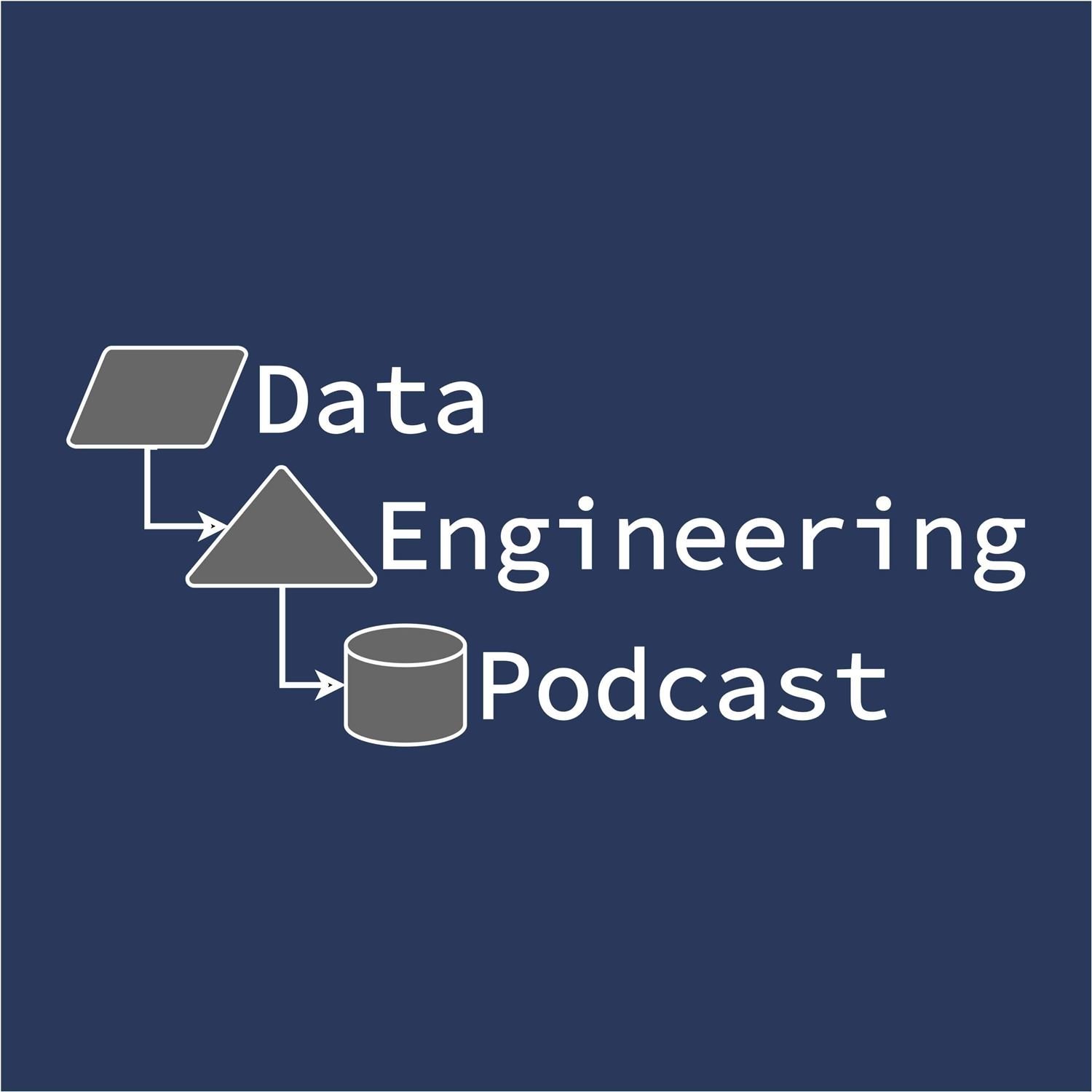
Reconciling The Data In Your Databases With Datafold
Summary
A significant portion of data workflows involve storing and processing information in database engines. Validating that the information is stored and processed correctly can be complex and time-consuming, especially when the source and destination speak different dialects of SQL. In this episode Gleb Mezhanskiy, founder and CEO of Datafold, discusses the different error conditions and solutions that you need to know about to ensure the accuracy of your data.
Announcements
Hello and welcome to the Data Engineering Podcast, the show about modern data management
Dagster offers a new approach to building and running data platforms and data pipelines. It is an open-source, cloud-native orchestrator for the whole development lifecycle, with integrated lineage and observability, a declarative programming model, and best-in-class testability. Your team can get up and running in minutes thanks to Dagster Cloud, an enterprise-class hosted solution that offers serverless and hybrid deployments, enhanced security, and on-demand ephemeral test deployments. Go to dataengineeringpodcast.com/dagster today to get started. Your first 30 days are free!
Data lakes are notoriously complex. For data engineers who battle to build and scale high quality data workflows on the data lake, Starburst powers petabyte-scale SQL analytics fast, at a fraction of the cost of traditional methods, so that you can meet all your data needs ranging from AI to data applications to complete analytics. Trusted by teams of all sizes, including Comcast and Doordash, Starburst is a data lake analytics platform that delivers the adaptability and flexibility a lakehouse ecosystem promises. And Starburst does all of this on an open architecture with first-class support for Apache Iceberg, Delta Lake and Hudi, so you always maintain ownership of your data. Want to see Starburst in action? Go to dataengineeringpodcast.com/starburst and get $500 in credits to try Starburst Galaxy today, the easiest and fastest way to get started using Trino.
Join us at the top event for the global data community, Data Council Austin. From March 26-28th 2024, we'll play host to hundreds of attendees, 100 top speakers and dozens of startups that are advancing data science, engineering and AI. Data Council attendees are amazing founders, data scientists, lead engineers, CTOs, heads of data, investors and community organizers who are all working together to build the future of data and sharing their insights and learnings through deeply technical talks. As a listener to the Data Engineering Podcast you can get a special discount off regular priced and late bird tickets by using the promo code dataengpod20. Don't miss out on our only event this year! Visit dataengineeringpodcast.com/data-council and use code dataengpod20 to register today!
Your host is Tobias Macey and today I'm welcoming back Gleb Mezhanskiy to talk about how to reconcile data in database environments
Interview
Introduction
How did you get involved in the area of data management?
Can you start by outlining some of the situations where reconciling data between databases is needed?
What are examples of the error conditions that you are likely to run into when duplicating information between database engines?
When these errors do occur, what are some of the problems that they can cause?
When teams are replicating data between database engines, what are some of the common patterns for managing those flows?
How does that change between continual and one-time replication?
What are some of the steps involved in verifying the integrity of data replication between database engines?
If the source or destination isn't a traditional database engine (e.g. data lakehouse) how does that change the work involved in verifying the success of the replication?
What are the challenges of validating and reconciling data?
Sheer scale and cost of pulling data out, have to do in-place
Performance. Pushing databases to the limit, especially hard for OLTP and legacy
Cross-database compatibilty
Data types
What are the most interesting, innovative, or unexpected ways that you have seen Datafold/data-diff used in the context of cross-database validation?
What are the most interesting, unexpected, or challenging lessons that you have learned while working on Datafold?
When is Datafold/data-diff the wrong choice?
What do you have planned for the future of Datafold?
Contact Info
LinkedIn
Parting Question
From your perspective, what is the biggest gap in the tooling or technology for data management today?
Closing Announcements
Thank you for listening! Don't forget to check out our other shows. Podcast.__init__ covers the Python language, its community, and the innovative ways it is being used. The Machine Learning Podcast helps you go from idea to production with machine learning.
Visit the site to subscribe to the show, sign up for the mailing list, and read the show notes.
If you've learned something or tried out a project from the show then tell us about it! Email [email protected]) with your story.
Links
Datafold
Podcast Episode
data-diff
Podcast Episode
Hive
Presto
Spark
SAP HANA
Change Data Capture
Nessie
Podcast Episode
LakeFS
Podcast Episode
Iceberg Tables
Podcast Episode
SQLGlot
Trino
GitHub Copilot
The intro and outro music is from The Hug by The Freak Fandango Orchestra / CC BY-SASponsored By:Starburst: 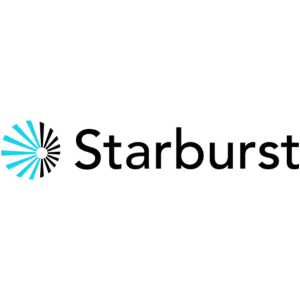
This episode is brought to you by Starburst - a data lake analytics platform for data engineers who are battling to build and scale high quality data pipelines on the data lake. Powered by Trino, Starburst runs petabyte-scale SQL analytics fast at a fraction of the cost of traditional methods, helping you meet all your data needs ranging from AI/ML workloads to data applications to complete analytics.
Trusted by the teams at Comcast and Doordash, Starburst delivers the adaptability and flexibility a lakehouse ecosystem promises, while providing a single point of access for your data and all your data governance allowing you to discover, transform, govern, and secure all in one place. Starburst does all of this on an open architecture with first-class support for Apache Iceberg, Delta Lake and Hudi, so you always maintain ownership of your data. Want to see Starburst in action? Try Starburst Galaxy today, the easiest and fastest way to get started using Trino, and get $500 of credits free. [dataengineeringpodcast.com/starburst](https://www.dataengineeringpodcast.com/starburst)Dagster: 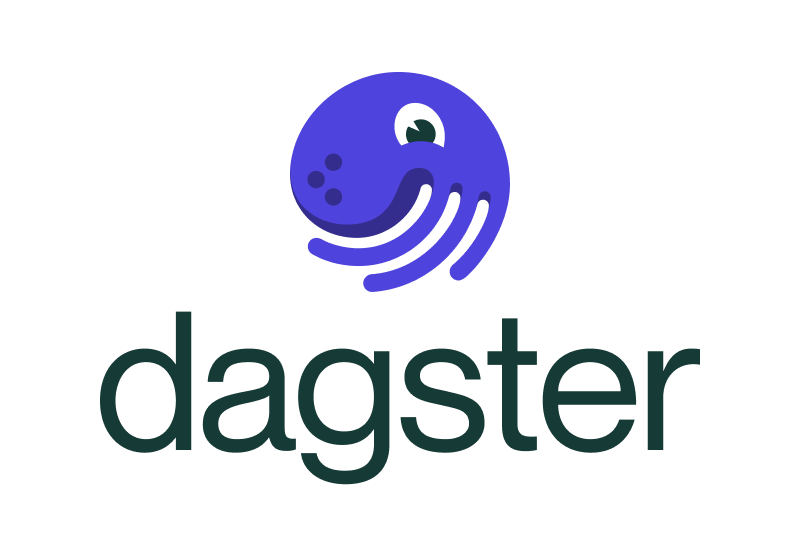
Data teams are tasked with helping organizations deliver on the premise of data, and with ML and AI maturing rapidly, expectations have never been this high. However data engineers are challenged by both technical complexity and organizational complexity, with heterogeneous technologies to adopt, multiple data disciplines converging, legacy systems to support, and costs to manage.
Dagster is an open-source orchestration solution that helps data teams reign in this complexity and build data platforms that provide unparalleled observability, and testability, all while fostering collaboration across the enterprise. With enterprise-grade hosting on Dagster Cloud, you gain even more capabilities, adding cost management, security, and CI support to further boost your teams' productivity. Go to [dagster.io](https://dagster.io/lp/dagster-cloud-trial?source=data-eng-podcast) today to get your first 30 days free!Data Council: 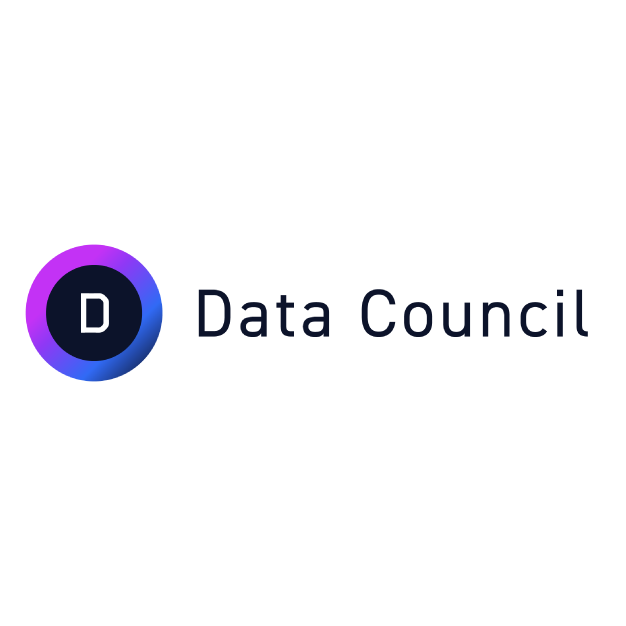
Join us at the top event for the global data community, Data Council Austin. From March 26-28th 2024, we'll play host to hundreds of attendees, 100 top speakers and dozens of startups that are advancing data science, engineering and AI. Data Council attendees are amazing founders, data scientists, lead engineers, CTOs, heads of data, investors and community organizers who are all working together to build the future of data and sharing their insights and learnings through deeply technical talks. As a listener to the Data Engineering Podcast you can get a special discount off regular priced and late bird tickets by using the promo code dataengpod20. Don't miss out on our only event this year! Visit [dataengineeringpodcast.com/data-council](https://www.dataengineeringpodcast.com/data-council) and use code **dataengpod20** to register today! Promo Code: dataengpod20Support Data Engineering Podcast
58:1417/03/2024
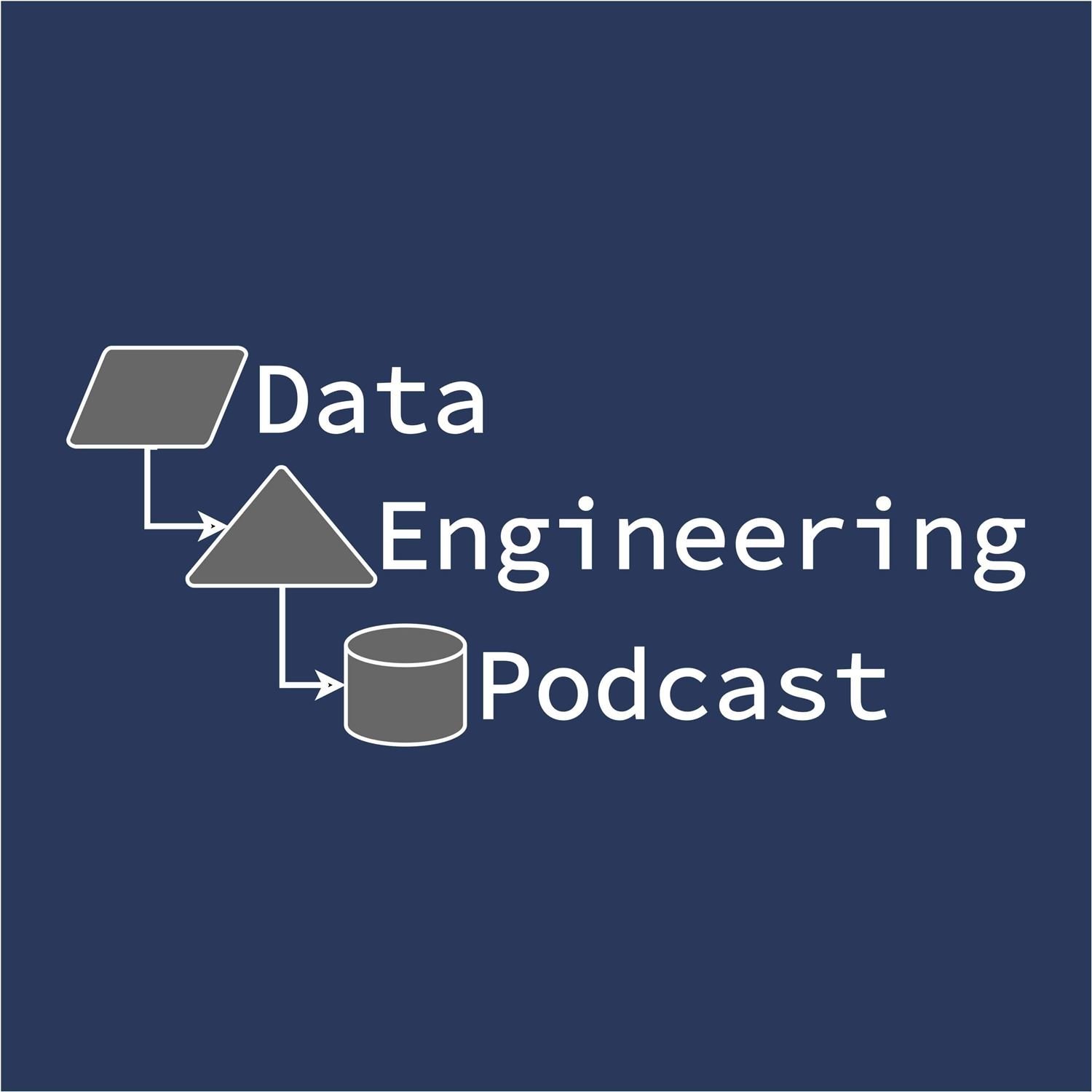
Version Your Data Lakehouse Like Your Software With Nessie
Summary
Data lakehouse architectures are gaining popularity due to the flexibility and cost effectiveness that they offer. The link that bridges the gap between data lake and warehouse capabilities is the catalog. The primary purpose of the catalog is to inform the query engine of what data exists and where, but the Nessie project aims to go beyond that simple utility. In this episode Alex Merced explains how the branching and merging functionality in Nessie allows you to use the same versioning semantics for your data lakehouse that you are used to from Git.
Announcements
Hello and welcome to the Data Engineering Podcast, the show about modern data management
Dagster offers a new approach to building and running data platforms and data pipelines. It is an open-source, cloud-native orchestrator for the whole development lifecycle, with integrated lineage and observability, a declarative programming model, and best-in-class testability. Your team can get up and running in minutes thanks to Dagster Cloud, an enterprise-class hosted solution that offers serverless and hybrid deployments, enhanced security, and on-demand ephemeral test deployments. Go to dataengineeringpodcast.com/dagster today to get started. Your first 30 days are free!
Data lakes are notoriously complex. For data engineers who battle to build and scale high quality data workflows on the data lake, Starburst powers petabyte-scale SQL analytics fast, at a fraction of the cost of traditional methods, so that you can meet all your data needs ranging from AI to data applications to complete analytics. Trusted by teams of all sizes, including Comcast and Doordash, Starburst is a data lake analytics platform that delivers the adaptability and flexibility a lakehouse ecosystem promises. And Starburst does all of this on an open architecture with first-class support for Apache Iceberg, Delta Lake and Hudi, so you always maintain ownership of your data. Want to see Starburst in action? Go to dataengineeringpodcast.com/starburst and get $500 in credits to try Starburst Galaxy today, the easiest and fastest way to get started using Trino.
Join us at the top event for the global data community, Data Council Austin. From March 26-28th 2024, we'll play host to hundreds of attendees, 100 top speakers and dozens of startups that are advancing data science, engineering and AI. Data Council attendees are amazing founders, data scientists, lead engineers, CTOs, heads of data, investors and community organizers who are all working together to build the future of data and sharing their insights and learnings through deeply technical talks. As a listener to the Data Engineering Podcast you can get a special discount off regular priced and late bird tickets by using the promo code dataengpod20. Don't miss out on our only event this year! Visit dataengineeringpodcast.com/data-council and use code dataengpod20 to register today!
Your host is Tobias Macey and today I'm interviewing Alex Merced, developer advocate at Dremio and co-author of the upcoming book from O'reilly, "Apache Iceberg, The definitive Guide", about Nessie, a git-like versioned catalog for data lakes using Apache Iceberg
Interview
Introduction
How did you get involved in the area of data management?
Can you describe what Nessie is and the story behind it?
What are the core problems/complexities that Nessie is designed to solve?
The closest analogue to Nessie that I've seen in the ecosystem is LakeFS. What are the features that would lead someone to choose one or the other for a given use case?
Why would someone choose Nessie over native table-level branching in the Apache Iceberg spec?
How do the versioning capabilities compare to/augment the data versioning in Iceberg?
What are some of the sources of, and challenges in resolving, merge conflicts between table branches?
Can you describe the architecture of Nessie?
How have the design and goals of the project changed since it was first created?
What is involved in integrating Nessie into a given data stack?
For cases where a given query/compute engine doesn't natively support Nessie, what are the options for using it effectively?
How does the inclusion of Nessie in a data lake influence the overall workflow of developing/deploying/evolving processing flows?
What are the most interesting, innovative, or unexpected ways that you have seen Nessie used?
What are the most interesting, unexpected, or challenging lessons that you have learned while working with Nessie?
When is Nessie the wrong choice?
What have you heard is planned for the future of Nessie?
Contact Info
LinkedIn
Twitter
Alex's Article on Dremio's Blog
Alex's Substack
Parting Question
From your perspective, what is the biggest gap in the tooling or technology for data management today?
Closing Announcements
Thank you for listening! Don't forget to check out our other shows. Podcast.__init__ covers the Python language, its community, and the innovative ways it is being used. The Machine Learning Podcast helps you go from idea to production with machine learning.
Visit the site to subscribe to the show, sign up for the mailing list, and read the show notes.
If you've learned something or tried out a project from the show then tell us about it! Email [email protected]) with your story.
Links
Project Nessie
Article: What is Nessie, Catalog Versioning and Git-for-Data?
Article: What is Lakehouse Management?: Git-for-Data, Automated Apache Iceberg Table Maintenance and more
Free Early Release Copy of "Apache Iceberg: The Definitive Guide"
Iceberg
Podcast Episode
Arrow
Podcast Episode
Data Lakehouse
LakeFS
Podcast Episode
AWS Glue
Tabular
Podcast Episode
Trino
Presto
Dremio
Podcast Episode
RocksDB
Delta Lake
Podcast Episode
Hive Metastore
PyIceberg
Optimistic Concurrency Control
The intro and outro music is from The Hug by The Freak Fandango Orchestra / CC BY-SASponsored By:Starburst: 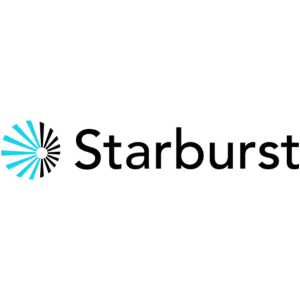
This episode is brought to you by Starburst - a data lake analytics platform for data engineers who are battling to build and scale high quality data pipelines on the data lake. Powered by Trino, Starburst runs petabyte-scale SQL analytics fast at a fraction of the cost of traditional methods, helping you meet all your data needs ranging from AI/ML workloads to data applications to complete analytics.
Trusted by the teams at Comcast and Doordash, Starburst delivers the adaptability and flexibility a lakehouse ecosystem promises, while providing a single point of access for your data and all your data governance allowing you to discover, transform, govern, and secure all in one place. Starburst does all of this on an open architecture with first-class support for Apache Iceberg, Delta Lake and Hudi, so you always maintain ownership of your data. Want to see Starburst in action? Try Starburst Galaxy today, the easiest and fastest way to get started using Trino, and get $500 of credits free. [dataengineeringpodcast.com/starburst](https://www.dataengineeringpodcast.com/starburst)Data Council: 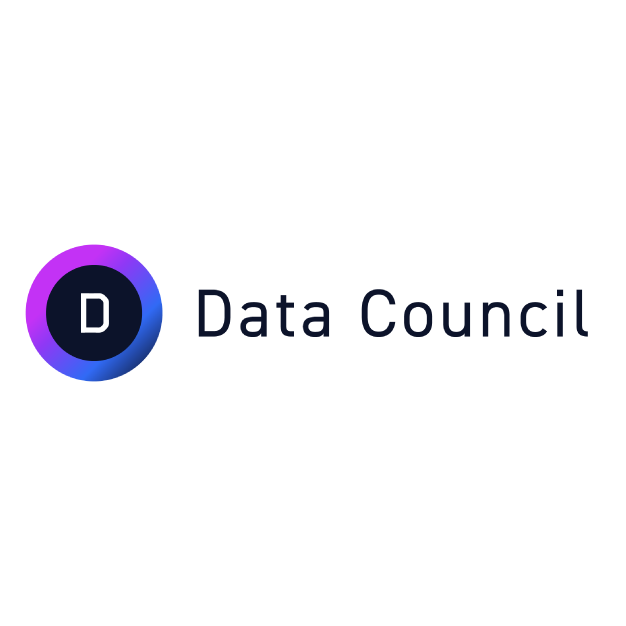
Join us at the top event for the global data community, Data Council Austin. From March 26-28th 2024, we'll play host to hundreds of attendees, 100 top speakers and dozens of startups that are advancing data science, engineering and AI. Data Council attendees are amazing founders, data scientists, lead engineers, CTOs, heads of data, investors and community organizers who are all working together to build the future of data and sharing their insights and learnings through deeply technical talks. As a listener to the Data Engineering Podcast you can get a special discount off regular priced and late bird tickets by using the promo code dataengpod20. Don't miss out on our only event this year! Visit [dataengineeringpodcast.com/data-council](https://www.dataengineeringpodcast.com/data-council) and use code **dataengpod20** to register today! Promo Code: dataengpod20Dagster: 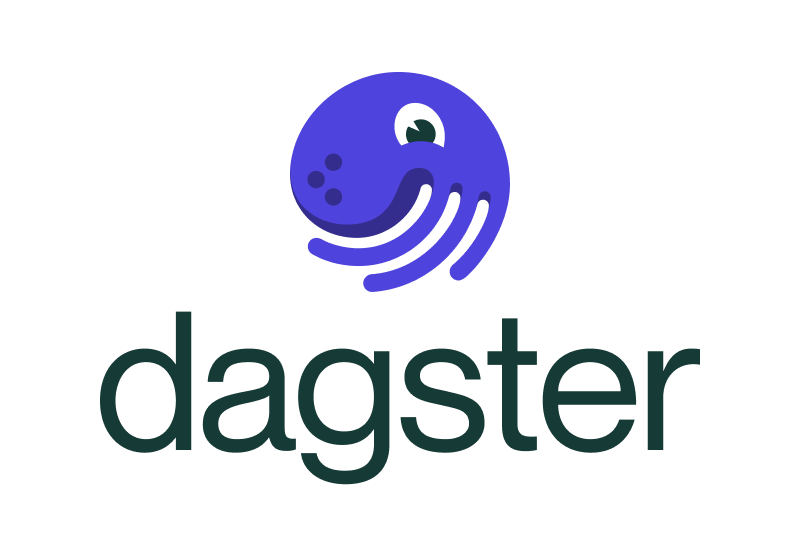
Data teams are tasked with helping organizations deliver on the premise of data, and with ML and AI maturing rapidly, expectations have never been this high. However data engineers are challenged by both technical complexity and organizational complexity, with heterogeneous technologies to adopt, multiple data disciplines converging, legacy systems to support, and costs to manage.
Dagster is an open-source orchestration solution that helps data teams reign in this complexity and build data platforms that provide unparalleled observability, and testability, all while fostering collaboration across the enterprise. With enterprise-grade hosting on Dagster Cloud, you gain even more capabilities, adding cost management, security, and CI support to further boost your teams' productivity. Go to [dagster.io](https://dagster.io/lp/dagster-cloud-trial?source=data-eng-podcast) today to get your first 30 days free!Support Data Engineering Podcast
40:5510/03/2024
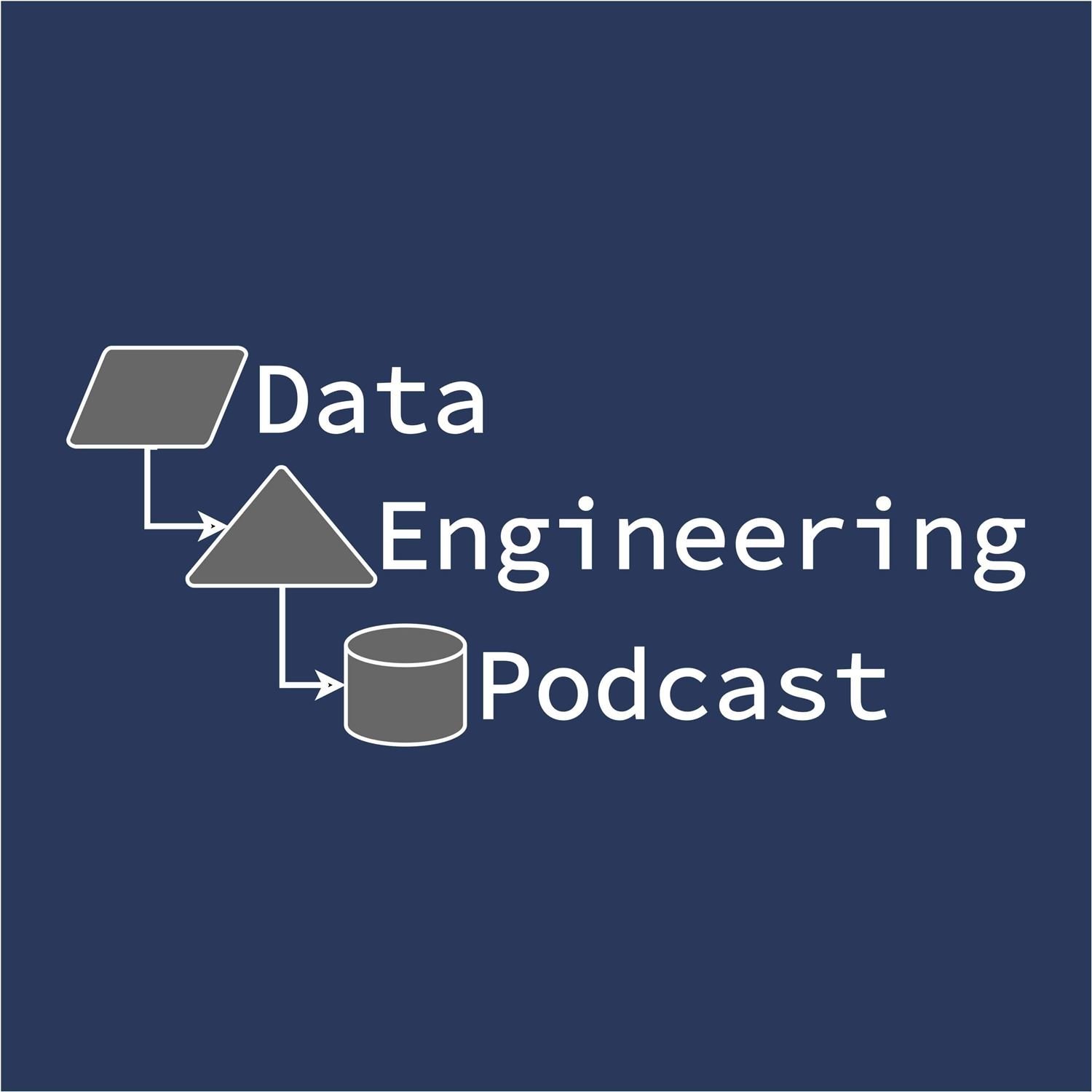
When And How To Conduct An AI Program
Summary
Artificial intelligence technologies promise to revolutionize business and produce new sources of value. In order to make those promises a reality there is a substantial amount of strategy and investment required. Colleen Tartow has worked across all stages of the data lifecycle, and in this episode she shares her hard-earned wisdom about how to conduct an AI program for your organization.
Announcements
Hello and welcome to the Data Engineering Podcast, the show about modern data management
Dagster offers a new approach to building and running data platforms and data pipelines. It is an open-source, cloud-native orchestrator for the whole development lifecycle, with integrated lineage and observability, a declarative programming model, and best-in-class testability. Your team can get up and running in minutes thanks to Dagster Cloud, an enterprise-class hosted solution that offers serverless and hybrid deployments, enhanced security, and on-demand ephemeral test deployments. Go to dataengineeringpodcast.com/dagster today to get started. Your first 30 days are free!
Data lakes are notoriously complex. For data engineers who battle to build and scale high quality data workflows on the data lake, Starburst powers petabyte-scale SQL analytics fast, at a fraction of the cost of traditional methods, so that you can meet all your data needs ranging from AI to data applications to complete analytics. Trusted by teams of all sizes, including Comcast and Doordash, Starburst is a data lake analytics platform that delivers the adaptability and flexibility a lakehouse ecosystem promises. And Starburst does all of this on an open architecture with first-class support for Apache Iceberg, Delta Lake and Hudi, so you always maintain ownership of your data. Want to see Starburst in action? Go to dataengineeringpodcast.com/starburst and get $500 in credits to try Starburst Galaxy today, the easiest and fastest way to get started using Trino.
Join us at the top event for the global data community, Data Council Austin. From March 26-28th 2024, we'll play host to hundreds of attendees, 100 top speakers and dozens of startups that are advancing data science, engineering and AI. Data Council attendees are amazing founders, data scientists, lead engineers, CTOs, heads of data, investors and community organizers who are all working together to build the future of data and sharing their insights and learnings through deeply technical talks. As a listener to the Data Engineering Podcast you can get a special discount off regular priced and late bird tickets by using the promo code dataengpod20. Don't miss out on our only event this year! Visit dataengineeringpodcast.com/data-council and use code dataengpod20 to register today!
Your host is Tobias Macey and today I'm interviewing Colleen Tartow about the questions to answer before and during the development of an AI program
Interview
Introduction
How did you get involved in the area of data management?
When you say "AI Program", what are the organizational, technical, and strategic elements that it encompasses?
How does the idea of an "AI Program" differ from an "AI Product"?
What are some of the signals to watch for that indicate an objective for which AI is not a reasonable solution?
Who needs to be involved in the process of defining and developing that program?
What are the skills and systems that need to be in place to effectively execute on an AI program?
"AI" has grown to be an even more overloaded term than it already was. What are some of the useful clarifying/scoping questions to address when deciding the path to deployment for different definitions of "AI"?
Organizations can easily fall into the trap of green-lighting an AI project before they have done the work of ensuring they have the necessary data and the ability to process it. What are the steps to take to build confidence in the availability of the data?
Even if you are sure that you can get the data, what are the implementation pitfalls that teams should be wary of while building out the data flows for powering the AI system?
What are the key considerations for powering AI applications that are substantially different from analytical applications?
The ecosystem for ML/AI is a rapidly moving target. What are the foundational/fundamental principles that you need to design around to allow for future flexibility?
What are the most interesting, innovative, or unexpected ways that you have seen AI programs implemented?
What are the most interesting, unexpected, or challenging lessons that you have learned while working on powering AI systems?
When is AI the wrong choice?
What do you have planned for the future of your work at VAST Data?
Contact Info
LinkedIn
Parting Question
From your perspective, what is the biggest gap in the tooling or technology for data management today?
Closing Announcements
Thank you for listening! Don't forget to check out our other shows. Podcast.__init__ covers the Python language, its community, and the innovative ways it is being used. The Machine Learning Podcast helps you go from idea to production with machine learning.
Visit the site to subscribe to the show, sign up for the mailing list, and read the show notes.
If you've learned something or tried out a project from the show then tell us about it! Email [email protected]) with your story.
Links
VAST Data
Colleen's Previous Appearance
Linear Regression
CoreWeave
Lambda Labs
MAD Landscape
Podcast Episode
ML Episode
The intro and outro music is from The Hug by The Freak Fandango Orchestra / CC BY-SASponsored By:Dagster: 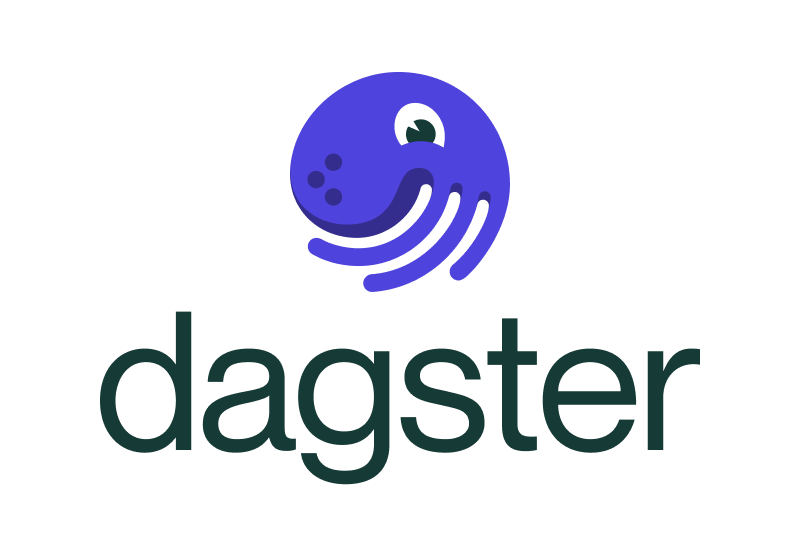
Data teams are tasked with helping organizations deliver on the premise of data, and with ML and AI maturing rapidly, expectations have never been this high. However data engineers are challenged by both technical complexity and organizational complexity, with heterogeneous technologies to adopt, multiple data disciplines converging, legacy systems to support, and costs to manage.
Dagster is an open-source orchestration solution that helps data teams reign in this complexity and build data platforms that provide unparalleled observability, and testability, all while fostering collaboration across the enterprise. With enterprise-grade hosting on Dagster Cloud, you gain even more capabilities, adding cost management, security, and CI support to further boost your teams' productivity. Go to [dagster.io](https://dagster.io/lp/dagster-cloud-trial?source=data-eng-podcast) today to get your first 30 days free!Data Council: 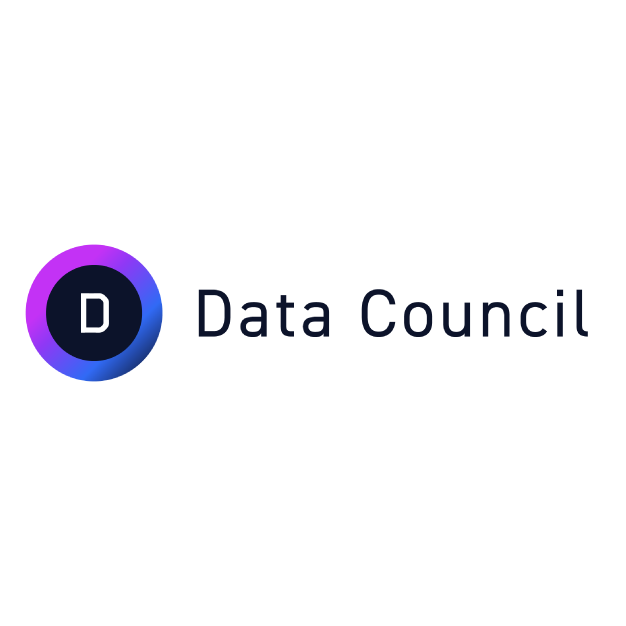
Join us at the top event for the global data community, Data Council Austin. From March 26-28th 2024, we'll play host to hundreds of attendees, 100 top speakers and dozens of startups that are advancing data science, engineering and AI. Data Council attendees are amazing founders, data scientists, lead engineers, CTOs, heads of data, investors and community organizers who are all working together to build the future of data and sharing their insights and learnings through deeply technical talks. As a listener to the Data Engineering Podcast you can get a special discount off regular priced and late bird tickets by using the promo code dataengpod20. Don't miss out on our only event this year! Visit [dataengineeringpodcast.com/data-council](https://www.dataengineeringpodcast.com/data-council) and use code **dataengpod20** to register today! Promo Code: dataengpod20Starburst: 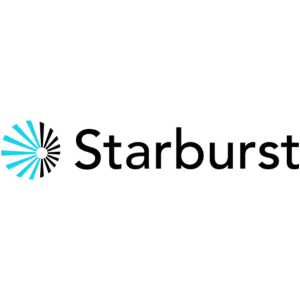
This episode is brought to you by Starburst - a data lake analytics platform for data engineers who are battling to build and scale high quality data pipelines on the data lake. Powered by Trino, Starburst runs petabyte-scale SQL analytics fast at a fraction of the cost of traditional methods, helping you meet all your data needs ranging from AI/ML workloads to data applications to complete analytics.
Trusted by the teams at Comcast and Doordash, Starburst delivers the adaptability and flexibility a lakehouse ecosystem promises, while providing a single point of access for your data and all your data governance allowing you to discover, transform, govern, and secure all in one place. Starburst does all of this on an open architecture with first-class support for Apache Iceberg, Delta Lake and Hudi, so you always maintain ownership of your data. Want to see Starburst in action? Try Starburst Galaxy today, the easiest and fastest way to get started using Trino, and get $500 of credits free. [dataengineeringpodcast.com/starburst](https://www.dataengineeringpodcast.com/starburst)Support Data Engineering Podcast
46:2503/03/2024
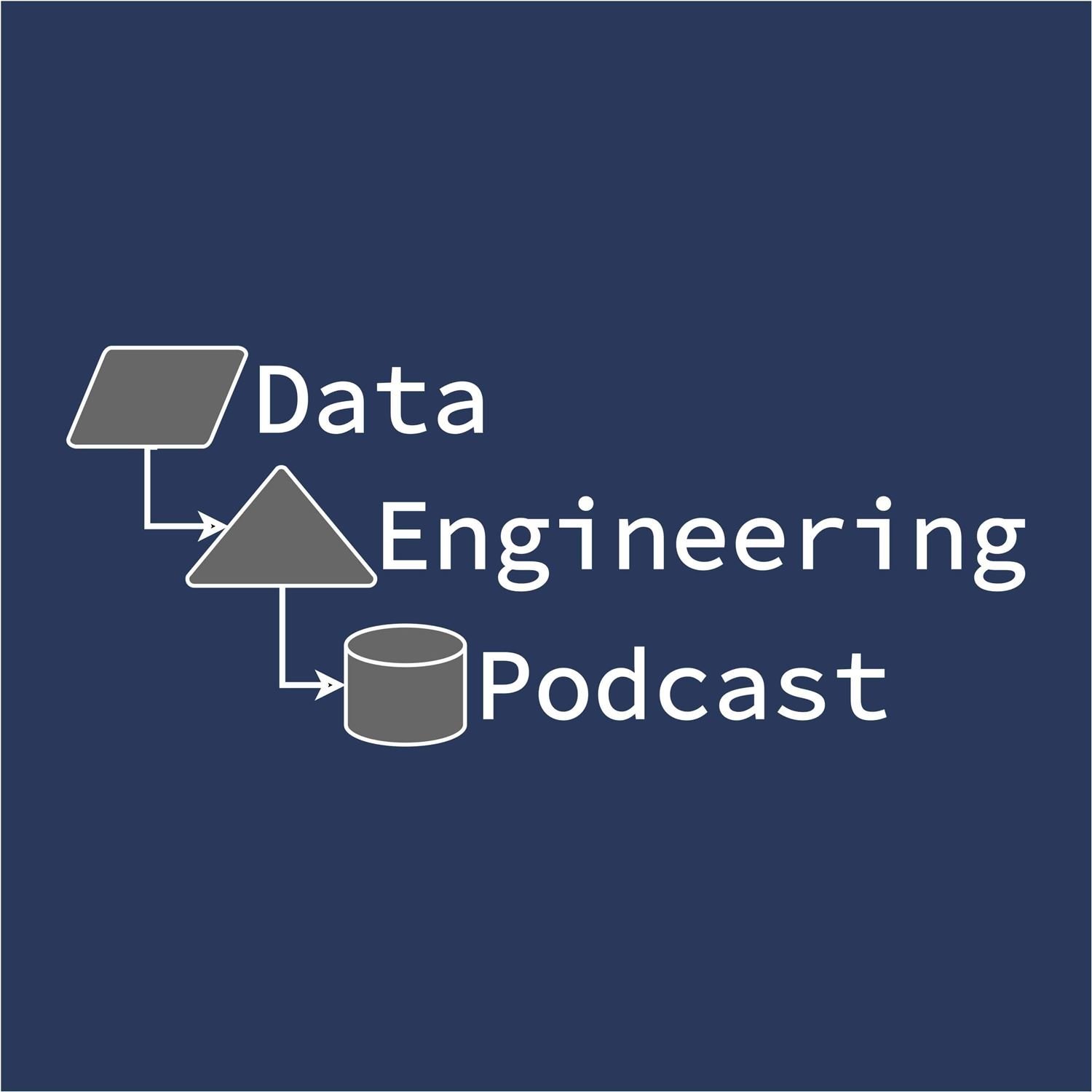
Find Out About The Technology Behind The Latest PFAD In Analytical Database Development
Summary
Building a database engine requires a substantial amount of engineering effort and time investment. Over the decades of research and development into building these software systems there are a number of common components that are shared across implementations. When Paul Dix decided to re-write the InfluxDB engine he found the Apache Arrow ecosystem ready and waiting with useful building blocks to accelerate the process. In this episode he explains how he used the combination of Apache Arrow, Flight, Datafusion, and Parquet to lay the foundation of the newest version of his time-series database.
Announcements
Hello and welcome to the Data Engineering Podcast, the show about modern data management
Dagster offers a new approach to building and running data platforms and data pipelines. It is an open-source, cloud-native orchestrator for the whole development lifecycle, with integrated lineage and observability, a declarative programming model, and best-in-class testability. Your team can get up and running in minutes thanks to Dagster Cloud, an enterprise-class hosted solution that offers serverless and hybrid deployments, enhanced security, and on-demand ephemeral test deployments. Go to dataengineeringpodcast.com/dagster today to get started. Your first 30 days are free!
Data lakes are notoriously complex. For data engineers who battle to build and scale high quality data workflows on the data lake, Starburst powers petabyte-scale SQL analytics fast, at a fraction of the cost of traditional methods, so that you can meet all your data needs ranging from AI to data applications to complete analytics. Trusted by teams of all sizes, including Comcast and Doordash, Starburst is a data lake analytics platform that delivers the adaptability and flexibility a lakehouse ecosystem promises. And Starburst does all of this on an open architecture with first-class support for Apache Iceberg, Delta Lake and Hudi, so you always maintain ownership of your data. Want to see Starburst in action? Go to dataengineeringpodcast.com/starburst and get $500 in credits to try Starburst Galaxy today, the easiest and fastest way to get started using Trino.
Join us at the top event for the global data community, Data Council Austin. From March 26-28th 2024, we'll play host to hundreds of attendees, 100 top speakers and dozens of startups that are advancing data science, engineering and AI. Data Council attendees are amazing founders, data scientists, lead engineers, CTOs, heads of data, investors and community organizers who are all working together to build the future of data and sharing their insights and learnings through deeply technical talks. As a listener to the Data Engineering Podcast you can get a special discount off regular priced and late bird tickets by using the promo code dataengpod20. Don't miss out on our only event this year! Visit dataengineeringpodcast.com/data-council and use code dataengpod20 to register today!
Your host is Tobias Macey and today I'm interviewing Paul Dix about his investment in the Apache Arrow ecosystem and how it led him to create the latest PFAD in database design
Interview
Introduction
How did you get involved in the area of data management?
Can you start by describing the FDAP stack and how the components combine to provide a foundational architecture for database engines?
This was the core of your recent re-write of the InfluxDB engine. What were the design goals and constraints that led you to this architecture?
Each of the architectural components are well engineered for their particular scope. What is the engineering work that is involved in building a cohesive platform from those components?
One of the major benefits of using open source components is the network effect of ecosystem integrations. That can also be a risk when the community vision for the project doesn't align with your own goals. How have you worked to mitigate that risk in your specific platform?
Can you describe the operational/architectural aspects of building a full data engine on top of the FDAP stack?
What are the elements of the overall product/user experience that you had to build to create a cohesive platform?
What are some of the other tools/technologies that can benefit from some or all of the pieces of the FDAP stack?
What are the pieces of the Arrow ecosystem that are still immature or need further investment from the community?
What are the most interesting, innovative, or unexpected ways that you have seen parts or all of the FDAP stack used?
What are the most interesting, unexpected, or challenging lessons that you have learned while working on/with the FDAP stack?
When is the FDAP stack the wrong choice?
What do you have planned for the future of the InfluxDB IOx engine and the FDAP stack?
Contact Info
LinkedIn
pauldix on GitHub
Parting Question
From your perspective, what is the biggest gap in the tooling or technology for data management today?
Closing Announcements
Thank you for listening! Don't forget to check out our other shows. Podcast.__init__ covers the Python language, its community, and the innovative ways it is being used. The Machine Learning Podcast helps you go from idea to production with machine learning.
Visit the site to subscribe to the show, sign up for the mailing list, and read the show notes.
If you've learned something or tried out a project from the show then tell us about it! Email [email protected]) with your story.
Links
FDAP Stack Blog Post
Apache Arrow
DataFusion
Arrow Flight
Apache Parquet
InfluxDB
Influx Data
Podcast Episode
Rust Language
DuckDB
ClickHouse
Voltron Data
Podcast Episode
Velox
Iceberg
Podcast Episode
Trino
ODBC == Open DataBase Connectivity
GeoParquet
ORC == Optimized Row Columnar
Avro
Protocol Buffers
gRPC
The intro and outro music is from The Hug by The Freak Fandango Orchestra / CC BY-SASponsored By:Starburst: 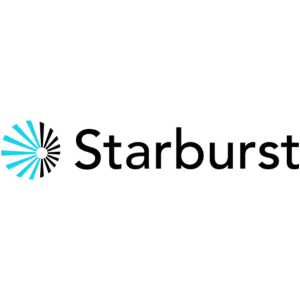
This episode is brought to you by Starburst - a data lake analytics platform for data engineers who are battling to build and scale high quality data pipelines on the data lake. Powered by Trino, Starburst runs petabyte-scale SQL analytics fast at a fraction of the cost of traditional methods, helping you meet all your data needs ranging from AI/ML workloads to data applications to complete analytics.
Trusted by the teams at Comcast and Doordash, Starburst delivers the adaptability and flexibility a lakehouse ecosystem promises, while providing a single point of access for your data and all your data governance allowing you to discover, transform, govern, and secure all in one place. Starburst does all of this on an open architecture with first-class support for Apache Iceberg, Delta Lake and Hudi, so you always maintain ownership of your data. Want to see Starburst in action? Try Starburst Galaxy today, the easiest and fastest way to get started using Trino, and get $500 of credits free. [dataengineeringpodcast.com/starburst](https://www.dataengineeringpodcast.com/starburst)Data Council: 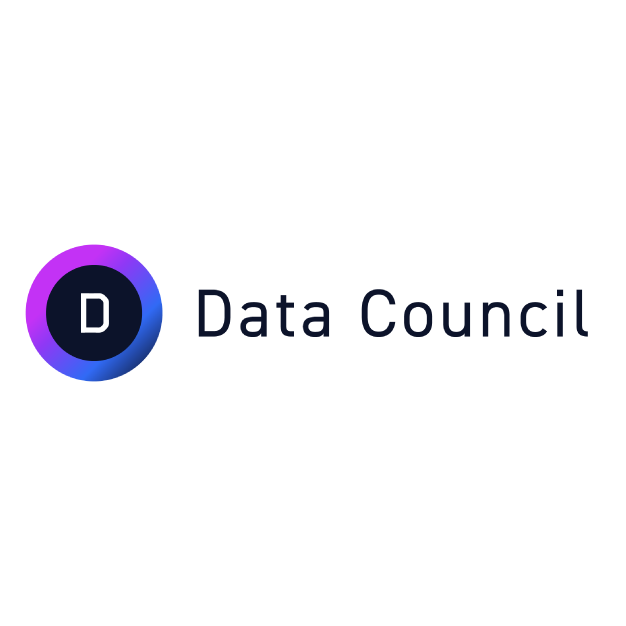
Join us at the top event for the global data community, Data Council Austin. From March 26-28th 2024, we'll play host to hundreds of attendees, 100 top speakers and dozens of startups that are advancing data science, engineering and AI. Data Council attendees are amazing founders, data scientists, lead engineers, CTOs, heads of data, investors and community organizers who are all working together to build the future of data and sharing their insights and learnings through deeply technical talks. As a listener to the Data Engineering Podcast you can get a special discount off regular priced and late bird tickets by using the promo code dataengpod20. Don't miss out on our only event this year! Visit [dataengineeringpodcast.com/data-council](https://www.dataengineeringpodcast.com/data-council) and use code **dataengpod20** to register today! Promo Code: dataengpod20Dagster: 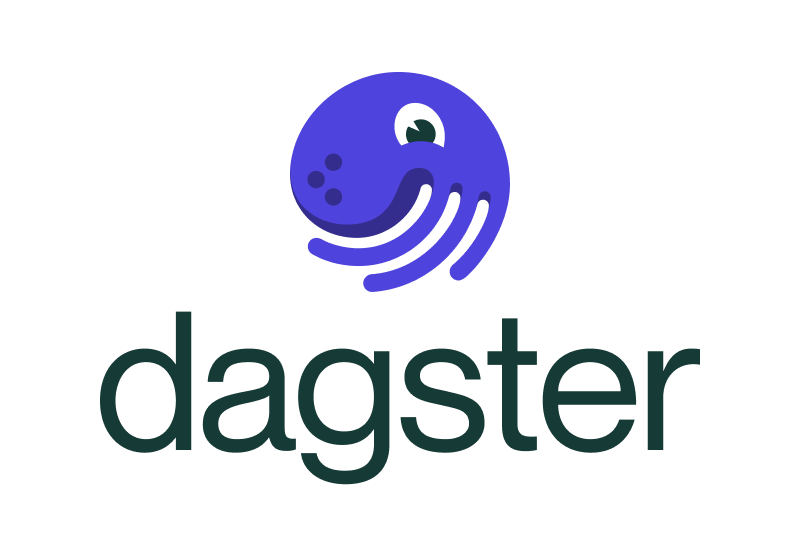
Data teams are tasked with helping organizations deliver on the premise of data, and with ML and AI maturing rapidly, expectations have never been this high. However data engineers are challenged by both technical complexity and organizational complexity, with heterogeneous technologies to adopt, multiple data disciplines converging, legacy systems to support, and costs to manage.
Dagster is an open-source orchestration solution that helps data teams reign in this complexity and build data platforms that provide unparalleled observability, and testability, all while fostering collaboration across the enterprise. With enterprise-grade hosting on Dagster Cloud, you gain even more capabilities, adding cost management, security, and CI support to further boost your teams' productivity. Go to [dagster.io](https://dagster.io/lp/dagster-cloud-trial?source=data-eng-podcast) today to get your first 30 days free!Support Data Engineering Podcast
56:0125/02/2024
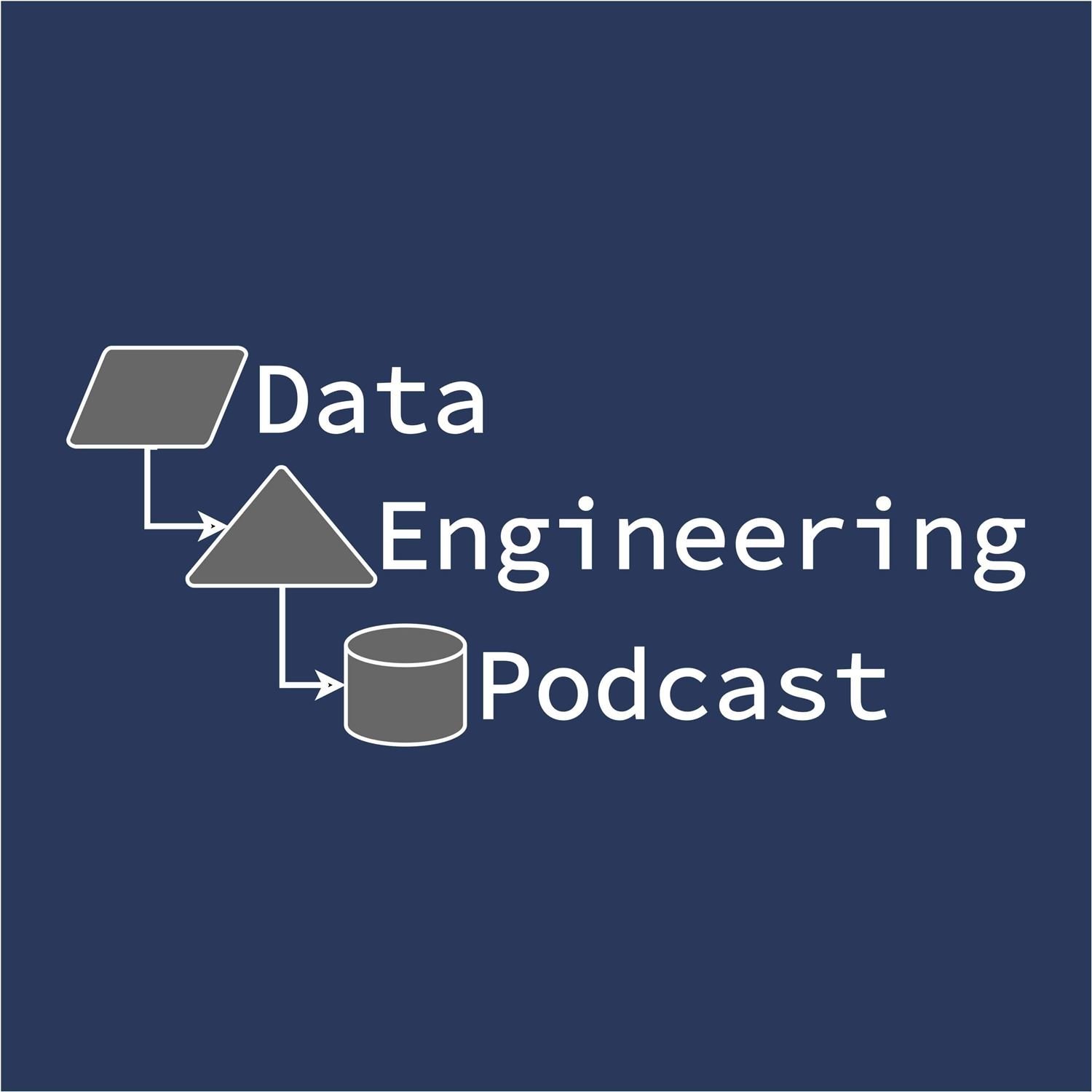
Using Trino And Iceberg As The Foundation Of Your Data Lakehouse
Summary
A data lakehouse is intended to combine the benefits of data lakes (cost effective, scalable storage and compute) and data warehouses (user friendly SQL interface). Multiple open source projects and vendors have been working together to make this vision a reality. In this episode Dain Sundstrom, CTO of Starburst, explains how the combination of the Trino query engine and the Iceberg table format offer the ease of use and execution speed of data warehouses with the infinite storage and scalability of data lakes.
Announcements
Hello and welcome to the Data Engineering Podcast, the show about modern data management
Dagster offers a new approach to building and running data platforms and data pipelines. It is an open-source, cloud-native orchestrator for the whole development lifecycle, with integrated lineage and observability, a declarative programming model, and best-in-class testability. Your team can get up and running in minutes thanks to Dagster Cloud, an enterprise-class hosted solution that offers serverless and hybrid deployments, enhanced security, and on-demand ephemeral test deployments. Go to dataengineeringpodcast.com/dagster today to get started. Your first 30 days are free!
Data lakes are notoriously complex. For data engineers who battle to build and scale high quality data workflows on the data lake, Starburst powers petabyte-scale SQL analytics fast, at a fraction of the cost of traditional methods, so that you can meet all your data needs ranging from AI to data applications to complete analytics. Trusted by teams of all sizes, including Comcast and Doordash, Starburst is a data lake analytics platform that delivers the adaptability and flexibility a lakehouse ecosystem promises. And Starburst does all of this on an open architecture with first-class support for Apache Iceberg, Delta Lake and Hudi, so you always maintain ownership of your data. Want to see Starburst in action? Go to dataengineeringpodcast.com/starburst and get $500 in credits to try Starburst Galaxy today, the easiest and fastest way to get started using Trino.
Join in with the event for the global data community, Data Council Austin. From March 26th-28th 2024, they'll play host to hundreds of attendees, 100 top speakers, and dozens of startups that are advancing data science, engineering and AI. Data Council attendees are amazing founders, data scientists, lead engineers, CTOs, heads of data, investors and community organizers who are all working togethr to build the future of data. As a listener to the Data Engineering Podcast you can get a special discount of 20% off your ticket by using the promo code dataengpod20. Don't miss out on their only event this year! Visit: dataengineeringpodcast.com/data-council today.
Your host is Tobias Macey and today I'm interviewing Dain Sundstrom about building a data lakehouse with Trino and Iceberg
Interview
Introduction
How did you get involved in the area of data management?
To start, can you share your definition of what constitutes a "Data Lakehouse"?
What are the technical/architectural/UX challenges that have hindered the progression of lakehouses?
What are the notable advancements in recent months/years that make them a more viable platform choice?
There are multiple tools and vendors that have adopted the "data lakehouse" terminology. What are the benefits offered by the combination of Trino and Iceberg?
What are the key points of comparison for that combination in relation to other possible selections?
What are the pain points that are still prevalent in lakehouse architectures as compared to warehouse or vertically integrated systems?
What progress is being made (within or across the ecosystem) to address those sharp edges?
For someone who is interested in building a data lakehouse with Trino and Iceberg, how does that influence their selection of other platform elements?
What are the differences in terms of pipeline design/access and usage patterns when using a Trino/Iceberg lakehouse as compared to other popular warehouse/lakehouse structures?
What are the most interesting, innovative, or unexpected ways that you have seen Trino lakehouses used?
What are the most interesting, unexpected, or challenging lessons that you have learned while working on the data lakehouse ecosystem?
When is a lakehouse the wrong choice?
What do you have planned for the future of Trino/Starburst?
Contact Info
LinkedIn
dain on GitHub
Parting Question
From your perspective, what is the biggest gap in the tooling or technology for data management today?
Closing Announcements
Thank you for listening! Don't forget to check out our other shows. Podcast.__init__ covers the Python language, its community, and the innovative ways it is being used. The Machine Learning Podcast helps you go from idea to production with machine learning.
Visit the site to subscribe to the show, sign up for the mailing list, and read the show notes.
If you've learned something or tried out a project from the show then tell us about it! Email [email protected]) with your story.
Links
Trino
Starburst
Presto
JBoss
Java EE
HDFS
S3
GCS == Google Cloud Storage
Hive
Hive ACID
Apache Ranger
OPA == Open Policy Agent
Oso
AWS Lakeformation
Tabular
Iceberg
Podcast Episode
Delta Lake
Podcast Episode
Debezium
Podcast Episode
Materialized View
Clickhouse
Druid
Hudi
Podcast Episode
The intro and outro music is from The Hug by The Freak Fandango Orchestra / CC BY-SASponsored By:Data Council: 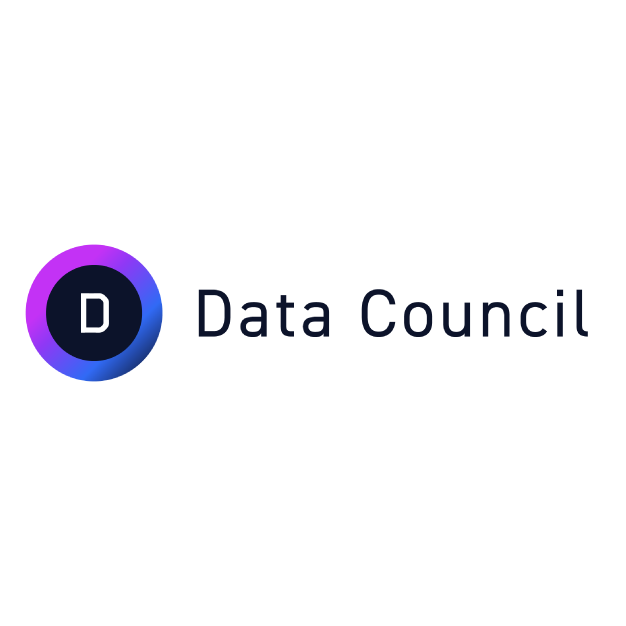
Join us at the top event for the global data community, Data Council Austin. From March 26-28th 2024, we'll play host to hundreds of attendees, 100 top speakers and dozens of startups that are advancing data science, engineering and AI. Data Council attendees are amazing founders, data scientists, lead engineers, CTOs, heads of data, investors and community organizers who are all working together to build the future of data and sharing their insights and learnings through deeply technical talks. As a listener to the Data Engineering Podcast you can get a special discount off regular priced and late bird tickets by using the promo code dataengpod20. Don't miss out on our only event this year! Visit [dataengineeringpodcast.com/data-council](https://www.dataengineeringpodcast.com/data-council) and use code **dataengpod20** to register today! Promo Code: dataengpod20Starburst: 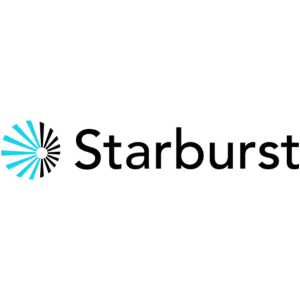
This episode is brought to you by Starburst - a data lake analytics platform for data engineers who are battling to build and scale high quality data pipelines on the data lake. Powered by Trino, Starburst runs petabyte-scale SQL analytics fast at a fraction of the cost of traditional methods, helping you meet all your data needs ranging from AI/ML workloads to data applications to complete analytics.
Trusted by the teams at Comcast and Doordash, Starburst delivers the adaptability and flexibility a lakehouse ecosystem promises, while providing a single point of access for your data and all your data governance allowing you to discover, transform, govern, and secure all in one place. Starburst does all of this on an open architecture with first-class support for Apache Iceberg, Delta Lake and Hudi, so you always maintain ownership of your data. Want to see Starburst in action? Try Starburst Galaxy today, the easiest and fastest way to get started using Trino, and get $500 of credits free. [dataengineeringpodcast.com/starburst](https://www.dataengineeringpodcast.com/starburst)Dagster: 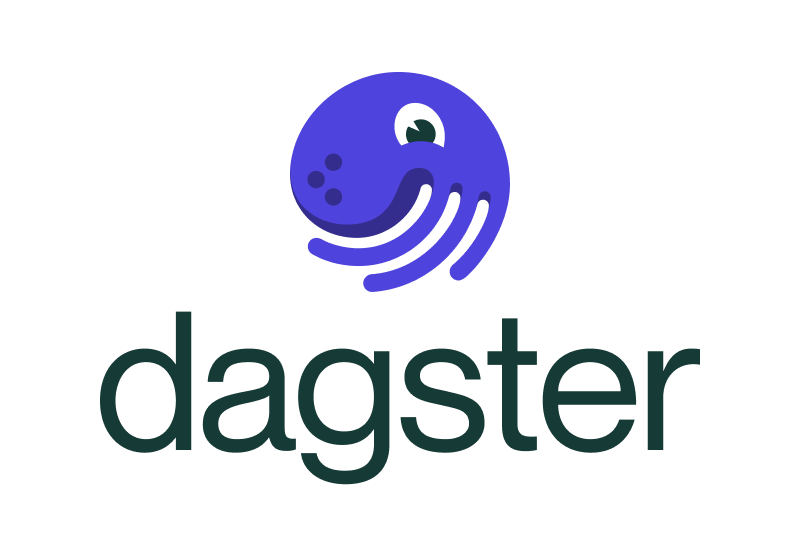
Data teams are tasked with helping organizations deliver on the premise of data, and with ML and AI maturing rapidly, expectations have never been this high. However data engineers are challenged by both technical complexity and organizational complexity, with heterogeneous technologies to adopt, multiple data disciplines converging, legacy systems to support, and costs to manage.
Dagster is an open-source orchestration solution that helps data teams reign in this complexity and build data platforms that provide unparalleled observability, and testability, all while fostering collaboration across the enterprise. With enterprise-grade hosting on Dagster Cloud, you gain even more capabilities, adding cost management, security, and CI support to further boost your teams' productivity. Go to [dagster.io](https://dagster.io/lp/dagster-cloud-trial?source=data-eng-podcast) today to get your first 30 days free!Support Data Engineering Podcast
58:4618/02/2024
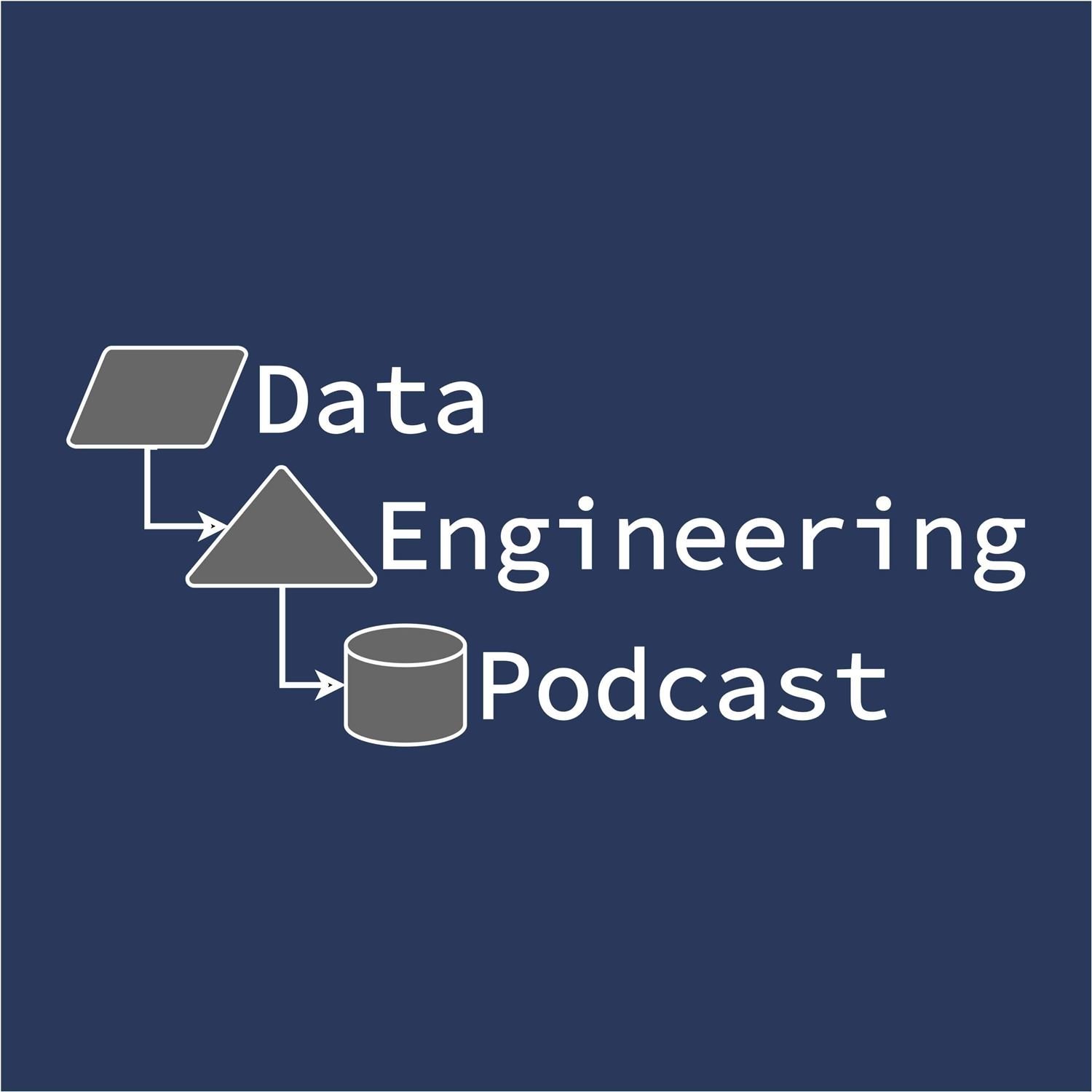
Data Sharing Across Business And Platform Boundaries
Summary
Sharing data is a simple concept, but complicated to implement well. There are numerous business rules and regulatory concerns that need to be applied. There are also numerous technical considerations to be made, particularly if the producer and consumer of the data aren't using the same platforms. In this episode Andrew Jefferson explains the complexities of building a robust system for data sharing, the techno-social considerations, and how the Bobsled platform that he is building aims to simplify the process.
Announcements
Hello and welcome to the Data Engineering Podcast, the show about modern data management
Data lakes are notoriously complex. For data engineers who battle to build and scale high quality data workflows on the data lake, Starburst powers petabyte-scale SQL analytics fast, at a fraction of the cost of traditional methods, so that you can meet all your data needs ranging from AI to data applications to complete analytics. Trusted by teams of all sizes, including Comcast and Doordash, Starburst is a data lake analytics platform that delivers the adaptability and flexibility a lakehouse ecosystem promises. And Starburst does all of this on an open architecture with first-class support for Apache Iceberg, Delta Lake and Hudi, so you always maintain ownership of your data. Want to see Starburst in action? Go to dataengineeringpodcast.com/starburst and get $500 in credits to try Starburst Galaxy today, the easiest and fastest way to get started using Trino.
Dagster offers a new approach to building and running data platforms and data pipelines. It is an open-source, cloud-native orchestrator for the whole development lifecycle, with integrated lineage and observability, a declarative programming model, and best-in-class testability. Your team can get up and running in minutes thanks to Dagster Cloud, an enterprise-class hosted solution that offers serverless and hybrid deployments, enhanced security, and on-demand ephemeral test deployments. Go to dataengineeringpodcast.com/dagster today to get started. Your first 30 days are free!
Your host is Tobias Macey and today I'm interviewing Andy Jefferson about how to solve the problem of data sharing
Interview
Introduction
How did you get involved in the area of data management?
Can you start by giving some context and scope of what we mean by "data sharing" for the purposes of this conversation?
What is the current state of the ecosystem for data sharing protocols/practices/platforms?
What are some of the main challenges/shortcomings that teams/organizations experience with these options?
What are the technical capabilities that need to be present for an effective data sharing solution?
How does that change as a function of the type of data? (e.g. tabular, image, etc.)
What are the requirements around governance and auditability of data access that need to be addressed when sharing data?
What are the typical boundaries along which data access requires special consideration for how the sharing is managed?
Many data platform vendors have their own interfaces for data sharing. What are the shortcomings of those options, and what are the opportunities for abstracting the sharing capability from the underlying platform?
What are the most interesting, innovative, or unexpected ways that you have seen data sharing/Bobsled used?
What are the most interesting, unexpected, or challenging lessons that you have learned while working on data sharing?
When is Bobsled the wrong choice?
What do you have planned for the future of data sharing?
Contact Info
LinkedIn
Parting Question
From your perspective, what is the biggest gap in the tooling or technology for data management today?
Closing Announcements
Thank you for listening! Don't forget to check out our other shows. Podcast.__init__ covers the Python language, its community, and the innovative ways it is being used. The Machine Learning Podcast helps you go from idea to production with machine learning.
Visit the site to subscribe to the show, sign up for the mailing list, and read the show notes.
If you've learned something or tried out a project from the show then tell us about it! Email [email protected]) with your story.
Links
Bobsled
OLAP == OnLine Analytical Processing
Cassandra
Podcast Episode
Neo4J
FTP == File Transfer Protocol
S3 Access Points
Snowflake Sharing
BigQuery Sharing
Databricks Delta Sharing
DuckDB
Podcast Episode
The intro and outro music is from The Hug by The Freak Fandango Orchestra / CC BY-SASponsored By:Starburst: 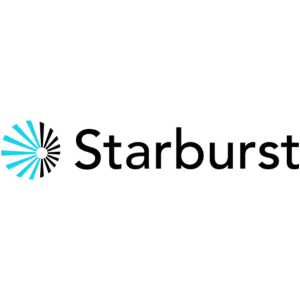
This episode is brought to you by Starburst - a data lake analytics platform for data engineers who are battling to build and scale high quality data pipelines on the data lake. Powered by Trino, Starburst runs petabyte-scale SQL analytics fast at a fraction of the cost of traditional methods, helping you meet all your data needs ranging from AI/ML workloads to data applications to complete analytics.
Trusted by the teams at Comcast and Doordash, Starburst delivers the adaptability and flexibility a lakehouse ecosystem promises, while providing a single point of access for your data and all your data governance allowing you to discover, transform, govern, and secure all in one place. Starburst does all of this on an open architecture with first-class support for Apache Iceberg, Delta Lake and Hudi, so you always maintain ownership of your data. Want to see Starburst in action? Try Starburst Galaxy today, the easiest and fastest way to get started using Trino, and get $500 of credits free. [dataengineeringpodcast.com/starburst](https://www.dataengineeringpodcast.com/starburst)Dagster: 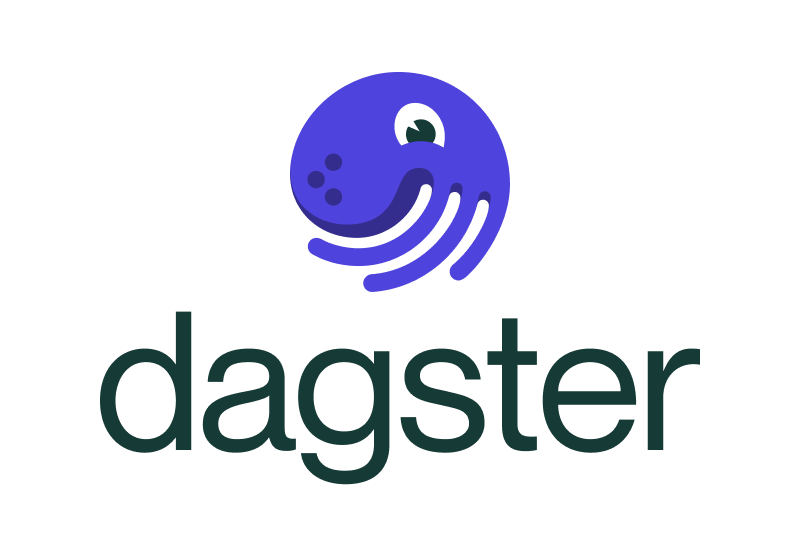
Data teams are tasked with helping organizations deliver on the premise of data, and with ML and AI maturing rapidly, expectations have never been this high. However data engineers are challenged by both technical complexity and organizational complexity, with heterogeneous technologies to adopt, multiple data disciplines converging, legacy systems to support, and costs to manage.
Dagster is an open-source orchestration solution that helps data teams reign in this complexity and build data platforms that provide unparalleled observability, and testability, all while fostering collaboration across the enterprise. With enterprise-grade hosting on Dagster Cloud, you gain even more capabilities, adding cost management, security, and CI support to further boost your teams' productivity. Go to [dagster.io](https://dagster.io/lp/dagster-cloud-trial?source=data-eng-podcast) today to get your first 30 days free!Support Data Engineering Podcast
59:5611/02/2024
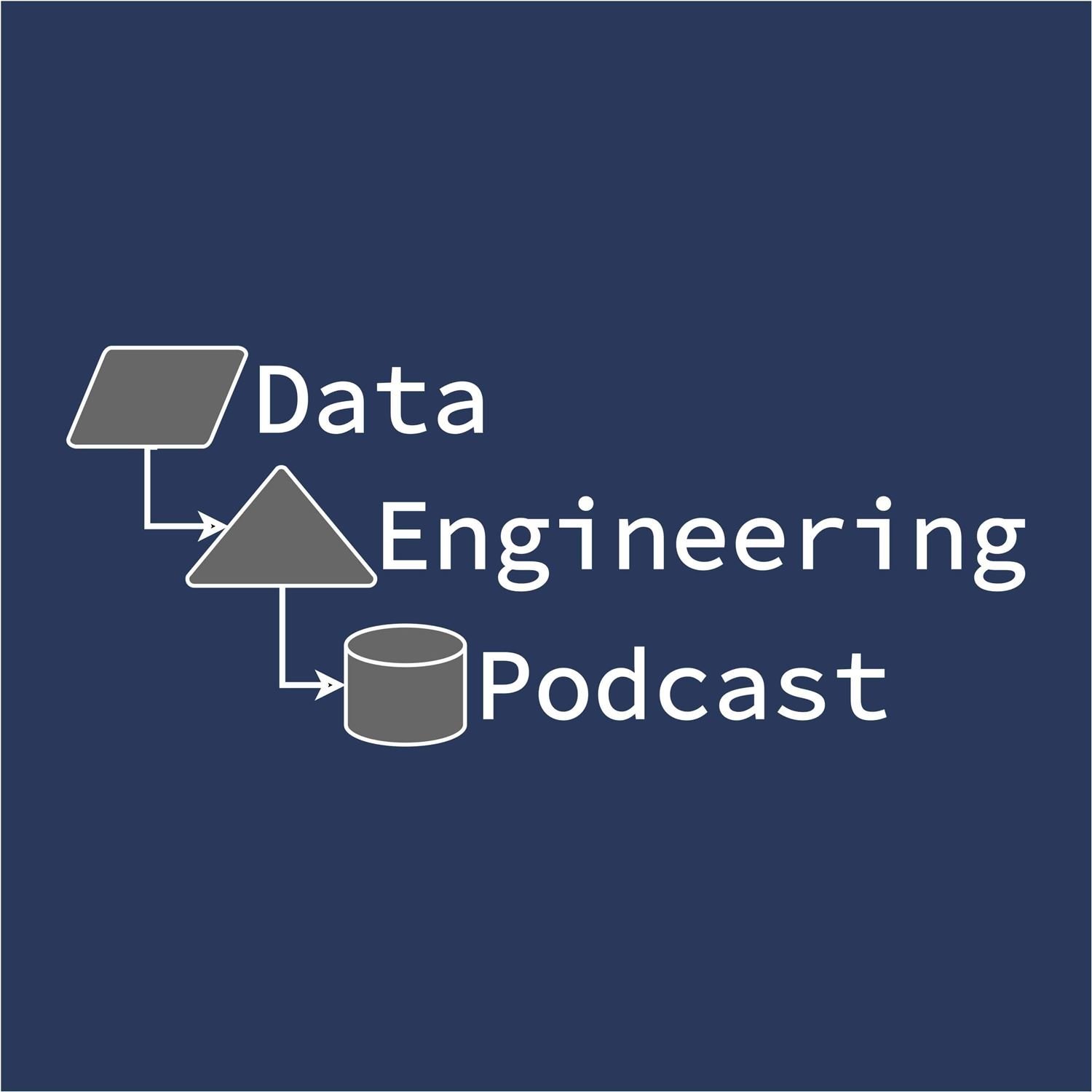
Tackling Real Time Streaming Data With SQL Using RisingWave
Summary
Stream processing systems have long been built with a code-first design, adding SQL as a layer on top of the existing framework. RisingWave is a database engine that was created specifically for stream processing, with S3 as the storage layer. In this episode Yingjun Wu explains how it is architected to power analytical workflows on continuous data flows, and the challenges of making it responsive and scalable.
Announcements
Hello and welcome to the Data Engineering Podcast, the show about modern data management
Data lakes are notoriously complex. For data engineers who battle to build and scale high quality data workflows on the data lake, Starburst powers petabyte-scale SQL analytics fast, at a fraction of the cost of traditional methods, so that you can meet all your data needs ranging from AI to data applications to complete analytics. Trusted by teams of all sizes, including Comcast and Doordash, Starburst is a data lake analytics platform that delivers the adaptability and flexibility a lakehouse ecosystem promises. And Starburst does all of this on an open architecture with first-class support for Apache Iceberg, Delta Lake and Hudi, so you always maintain ownership of your data. Want to see Starburst in action? Go to dataengineeringpodcast.com/starburst and get $500 in credits to try Starburst Galaxy today, the easiest and fastest way to get started using Trino.
Dagster offers a new approach to building and running data platforms and data pipelines. It is an open-source, cloud-native orchestrator for the whole development lifecycle, with integrated lineage and observability, a declarative programming model, and best-in-class testability. Your team can get up and running in minutes thanks to Dagster Cloud, an enterprise-class hosted solution that offers serverless and hybrid deployments, enhanced security, and on-demand ephemeral test deployments. Go to dataengineeringpodcast.com/dagster today to get started. Your first 30 days are free!
Your host is Tobias Macey and today I'm interviewing Yingjun Wu about the RisingWave database and the intricacies of building a stream processing engine on S3
Interview
Introduction
How did you get involved in the area of data management?
Can you describe what RisingWave is and the story behind it?
There are numerous stream processing engines, near-real-time database engines, streaming SQL systems, etc. What is the specific niche that RisingWave addresses?
What are some of the platforms/architectures that teams are replacing with RisingWave?
What are some of the unique capabilities/use cases that RisingWave provides over other offerings in the current ecosystem?
Can you describe how RisingWave is architected and implemented?
How have the design and goals/scope changed since you first started working on it?
What are the core design philosophies that you rely on to prioritize the ongoing development of the project?
What are the most complex engineering challenges that you have had to address in the creation of RisingWave?
Can you describe a typical workflow for teams that are building on top of RisingWave?
What are the user/developer experience elements that you have prioritized most highly?
What are the situations where RisingWave can/should be a system of record vs. a point-in-time view of data in transit, with a data warehouse/lakehouse as the longitudinal storage and query engine?
What are the most interesting, innovative, or unexpected ways that you have seen RisingWave used?
What are the most interesting, unexpected, or challenging lessons that you have learned while working on RisingWave?
When is RisingWave the wrong choice?
What do you have planned for the future of RisingWave?
Contact Info
yingjunwu on GitHub
Personal Website
LinkedIn
Parting Question
From your perspective, what is the biggest gap in the tooling or technology for data management today?
Closing Announcements
Thank you for listening! Don't forget to check out our other shows. Podcast.__init__ covers the Python language, its community, and the innovative ways it is being used. The Machine Learning Podcast helps you go from idea to production with machine learning.
Visit the site to subscribe to the show, sign up for the mailing list, and read the show notes.
If you've learned something or tried out a project from the show then tell us about it! Email [email protected]) with your story.
Links
RisingWave
AWS Redshift
Flink
Podcast Episode
Clickhouse
Podcast Episode
Druid
Materialize
Spark
Trino
Snowflake
Kafka
Iceberg
Podcast Episode
Hudi
Podcast Episode
Postgres
Debezium
Podcast Episode
The intro and outro music is from The Hug by The Freak Fandango Orchestra / CC BY-SASponsored By:Dagster: 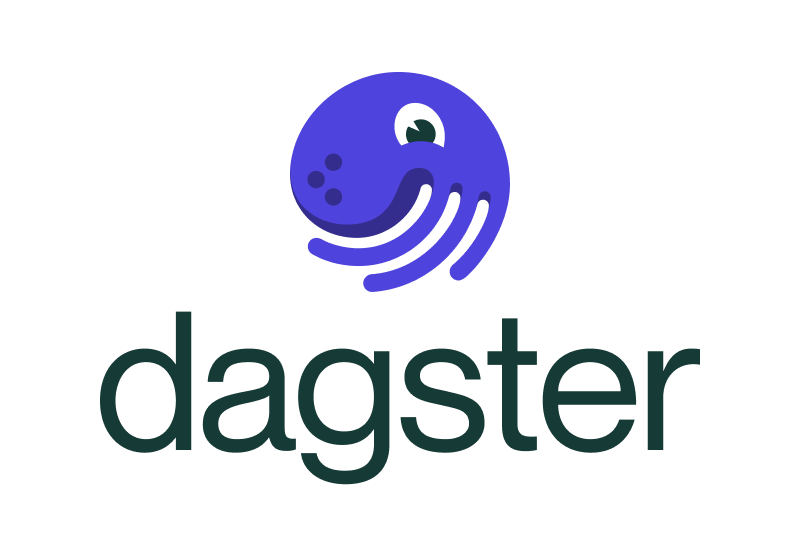
Data teams are tasked with helping organizations deliver on the premise of data, and with ML and AI maturing rapidly, expectations have never been this high. However data engineers are challenged by both technical complexity and organizational complexity, with heterogeneous technologies to adopt, multiple data disciplines converging, legacy systems to support, and costs to manage.
Dagster is an open-source orchestration solution that helps data teams reign in this complexity and build data platforms that provide unparalleled observability, and testability, all while fostering collaboration across the enterprise. With enterprise-grade hosting on Dagster Cloud, you gain even more capabilities, adding cost management, security, and CI support to further boost your teams' productivity. Go to [dagster.io](https://dagster.io/lp/dagster-cloud-trial?source=data-eng-podcast) today to get your first 30 days free!Starburst: 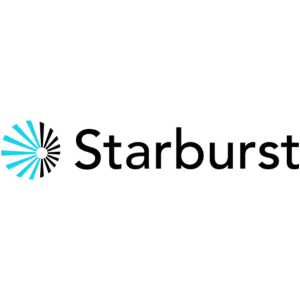
This episode is brought to you by Starburst - a data lake analytics platform for data engineers who are battling to build and scale high quality data pipelines on the data lake. Powered by Trino, Starburst runs petabyte-scale SQL analytics fast at a fraction of the cost of traditional methods, helping you meet all your data needs ranging from AI/ML workloads to data applications to complete analytics.
Trusted by the teams at Comcast and Doordash, Starburst delivers the adaptability and flexibility a lakehouse ecosystem promises, while providing a single point of access for your data and all your data governance allowing you to discover, transform, govern, and secure all in one place. Starburst does all of this on an open architecture with first-class support for Apache Iceberg, Delta Lake and Hudi, so you always maintain ownership of your data. Want to see Starburst in action? Try Starburst Galaxy today, the easiest and fastest way to get started using Trino, and get $500 of credits free. [dataengineeringpodcast.com/starburst](https://www.dataengineeringpodcast.com/starburst)Support Data Engineering Podcast
56:5504/02/2024
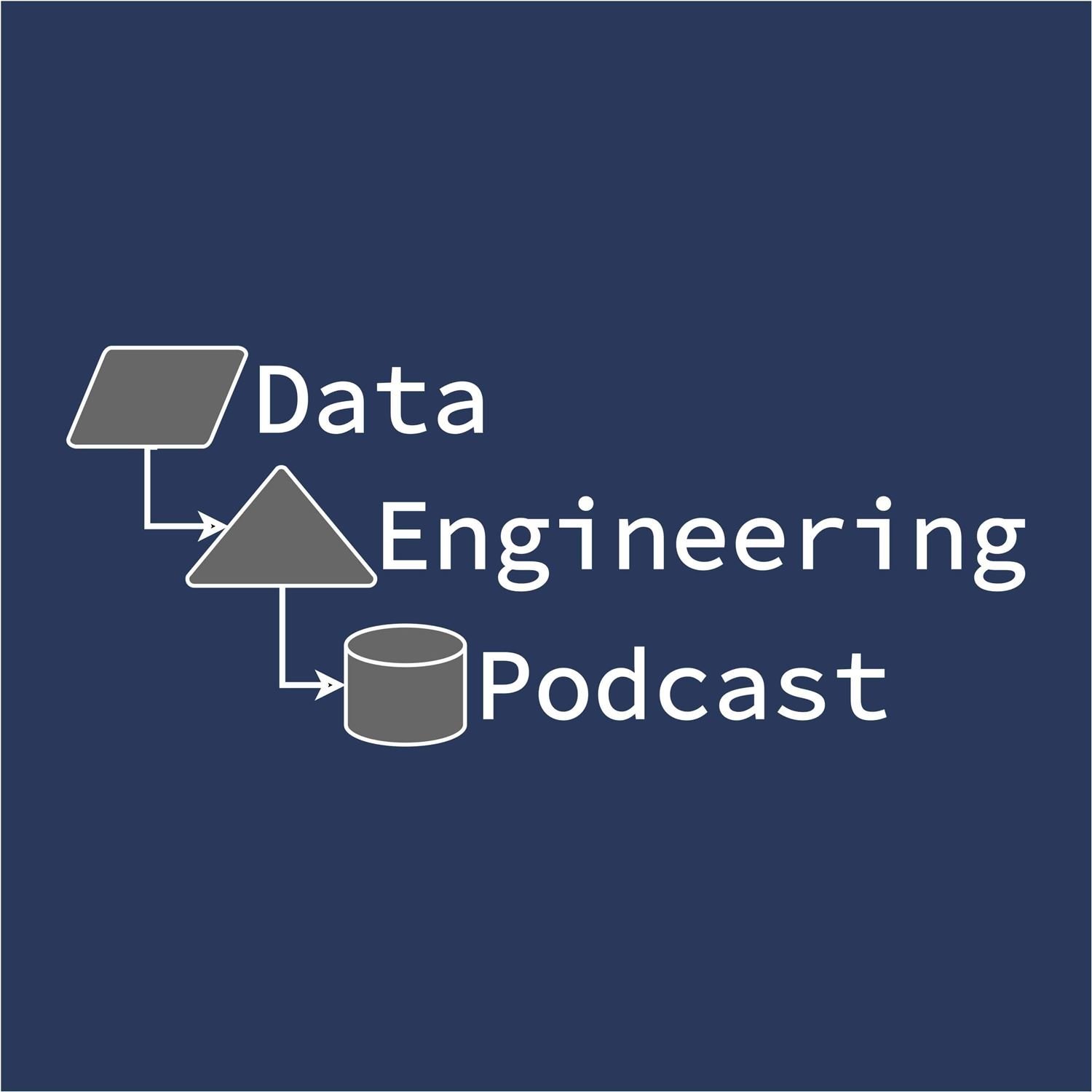
Build A Data Lake For Your Security Logs With Scanner
Summary
Monitoring and auditing IT systems for security events requires the ability to quickly analyze massive volumes of unstructured log data. The majority of products that are available either require too much effort to structure the logs, or aren't fast enough for interactive use cases. Cliff Crosland co-founded Scanner to provide fast querying of high scale log data for security auditing. In this episode he shares the story of how it got started, how it works, and how you can get started with it.
Announcements
Hello and welcome to the Data Engineering Podcast, the show about modern data management
Data lakes are notoriously complex. For data engineers who battle to build and scale high quality data workflows on the data lake, Starburst powers petabyte-scale SQL analytics fast, at a fraction of the cost of traditional methods, so that you can meet all your data needs ranging from AI to data applications to complete analytics. Trusted by teams of all sizes, including Comcast and Doordash, Starburst is a data lake analytics platform that delivers the adaptability and flexibility a lakehouse ecosystem promises. And Starburst does all of this on an open architecture with first-class support for Apache Iceberg, Delta Lake and Hudi, so you always maintain ownership of your data. Want to see Starburst in action? Go to dataengineeringpodcast.com/starburst and get $500 in credits to try Starburst Galaxy today, the easiest and fastest way to get started using Trino.
Your host is Tobias Macey and today I'm interviewing Cliff Crosland about Scanner, a security data lake platform for analyzing security logs and identifying issues quickly and cost-effectively
Interview
Introduction
How did you get involved in the area of data management?
Can you describe what Scanner is and the story behind it?
What were the shortcomings of other tools that are available in the ecosystem?
What is Scanner explicitly not trying to solve for in the security space? (e.g. SIEM)
A query engine is useless without data to analyze. What are the data acquisition paths/sources that you are designed to work with?- e.g. cloudtrail logs, app logs, etc.
What are some of the other sources of signal for security monitoring that would be valuable to incorporate or integrate with through Scanner?
Log data is notoriously messy, with no strictly defined format. How do you handle introspection and querying across loosely structured records that might span multiple sources and inconsistent labelling strategies?
Can you describe the architecture of the Scanner platform?
What were the motivating constraints that led you to your current implementation?
How have the design and goals of the product changed since you first started working on it?
Given the security oriented customer base that you are targeting, how do you address trust/network boundaries for compliance with regulatory/organizational policies?
What are the personas of the end-users for Scanner?
How has that influenced the way that you think about the query formats, APIs, user experience etc. for the prroduct?
For teams who are working with Scanner can you describe how it fits into their workflow?
What are the most interesting, innovative, or unexpected ways that you have seen Scanner used?
What are the most interesting, unexpected, or challenging lessons that you have learned while working on Scanner?
When is Scanner the wrong choice?
What do you have planned for the future of Scanner?
Contact Info
LinkedIn
Parting Question
From your perspective, what is the biggest gap in the tooling or technology for data management today?
Closing Announcements
Thank you for listening! Don't forget to check out our other shows. Podcast.__init__ covers the Python language, its community, and the innovative ways it is being used. The Machine Learning Podcast helps you go from idea to production with machine learning.
Visit the site to subscribe to the show, sign up for the mailing list, and read the show notes.
If you've learned something or tried out a project from the show then tell us about it! Email [email protected]) with your story.
Links
Scanner
cURL
Rust
Splunk
S3
AWS Athena
Loki
Snowflake
Podcast Episode
Presto
[Trino](thttps://trino.io/)
AWS CloudTrail
GitHub Audit Logs
Okta
Cribl
Vector.dev
Tines
Torq
Jira
Linear
ECS Fargate
SQS
Monoid
Group Theory
Avro
Parquet
OCSF
VPC Flow Logs
The intro and outro music is from The Hug by The Freak Fandango Orchestra / CC BY-SASponsored By:Starburst: 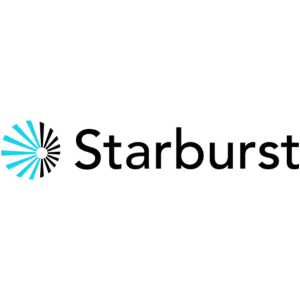
This episode is brought to you by Starburst - a data lake analytics platform for data engineers who are battling to build and scale high quality data pipelines on the data lake. Powered by Trino, Starburst runs petabyte-scale SQL analytics fast at a fraction of the cost of traditional methods, helping you meet all your data needs ranging from AI/ML workloads to data applications to complete analytics.
Trusted by the teams at Comcast and Doordash, Starburst delivers the adaptability and flexibility a lakehouse ecosystem promises, while providing a single point of access for your data and all your data governance allowing you to discover, transform, govern, and secure all in one place. Starburst does all of this on an open architecture with first-class support for Apache Iceberg, Delta Lake and Hudi, so you always maintain ownership of your data. Want to see Starburst in action? Try Starburst Galaxy today, the easiest and fastest way to get started using Trino, and get $500 of credits free. [dataengineeringpodcast.com/starburst](https://www.dataengineeringpodcast.com/starburst)Support Data Engineering Podcast
01:02:3829/01/2024
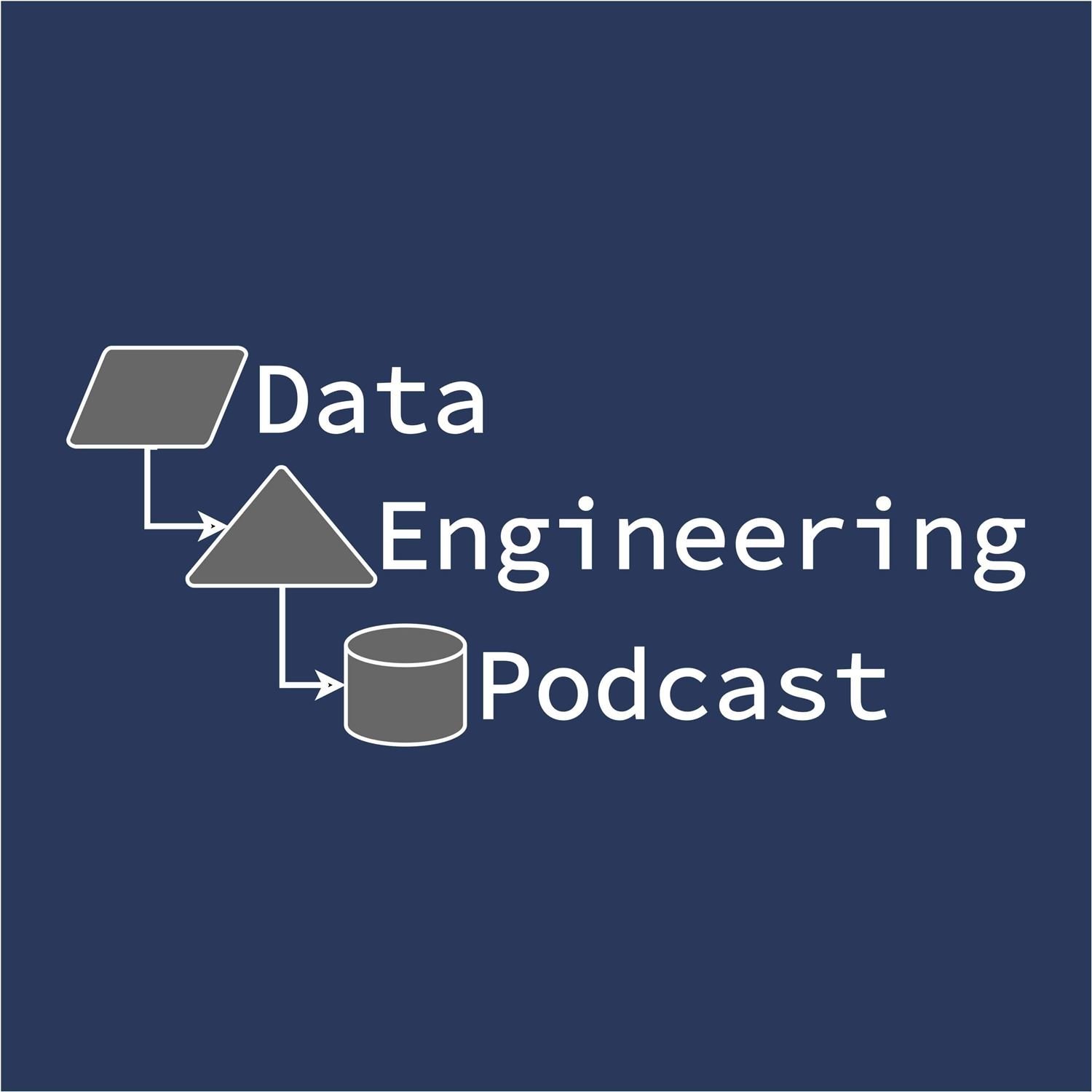
Modern Customer Data Platform Principles
Summary
Databases and analytics architectures have gone through several generational shifts. A substantial amount of the data that is being managed in these systems is related to customers and their interactions with an organization. In this episode Tasso Argyros, CEO of ActionIQ, gives a summary of the major epochs in database technologies and how he is applying the capabilities of cloud data warehouses to the challenge of building more comprehensive experiences for end-users through a modern customer data platform (CDP).
Announcements
Hello and welcome to the Data Engineering Podcast, the show about modern data management
Data lakes are notoriously complex. For data engineers who battle to build and scale high quality data workflows on the data lake, Starburst powers petabyte-scale SQL analytics fast, at a fraction of the cost of traditional methods, so that you can meet all your data needs ranging from AI to data applications to complete analytics. Trusted by teams of all sizes, including Comcast and Doordash, Starburst is a data lake analytics platform that delivers the adaptability and flexibility a lakehouse ecosystem promises. And Starburst does all of this on an open architecture with first-class support for Apache Iceberg, Delta Lake and Hudi, so you always maintain ownership of your data. Want to see Starburst in action? Go to dataengineeringpodcast.com/starburst and get $500 in credits to try Starburst Galaxy today, the easiest and fastest way to get started using Trino.
Data projects are notoriously complex. With multiple stakeholders to manage across varying backgrounds and toolchains even simple reports can become unwieldy to maintain. Miro is your single pane of glass where everyone can discover, track, and collaborate on your organization's data. I especially like the ability to combine your technical diagrams with data documentation and dependency mapping, allowing your data engineers and data consumers to communicate seamlessly about your projects. Find simplicity in your most complex projects with Miro. Your first three Miro boards are free when you sign up today at dataengineeringpodcast.com/miro. That’s three free boards at dataengineeringpodcast.com/miro.
Your host is Tobias Macey and today I'm interviewing Tasso Argyros about the role of a customer data platform in the context of the modern data stack
Interview
Introduction
How did you get involved in the area of data management?
Can you describe what the role of the CDP is in the context of a businesses data ecosystem?
What are the core technical challenges associated with building and maintaining a CDP?
What are the organizational/business factors that contribute to the complexity of these systems?
The early days of CDPs came with the promise of "Customer 360". Can you unpack that concept and how it has changed over the past ~5 years?
Recent years have seen the adoption of reverse ETL, cloud data warehouses, and sophisticated product analytics suites. How has that changed the architectural approach to CDPs?
How have the architectural shifts changed the ways that organizations interact with their customer data?
How have the responsibilities shifted across different roles?
What are the governance policy and enforcement challenges that are added with the expansion of access and responsibility?
What are the most interesting, innovative, or unexpected ways that you have seen CDPs built/used?
What are the most interesting, unexpected, or challenging lessons that you have learned while working on CDPs?
When is a CDP the wrong choice?
What do you have planned for the future of ActionIQ?
Contact Info
LinkedIn
@Tasso on Twitter
Parting Question
From your perspective, what is the biggest gap in the tooling or technology for data management today?
Closing Announcements
Thank you for listening! Don't forget to check out our other shows. Podcast.__init__ covers the Python language, its community, and the innovative ways it is being used. The Machine Learning Podcast helps you go from idea to production with machine learning.
Visit the site to subscribe to the show, sign up for the mailing list, and read the show notes.
If you've learned something or tried out a project from the show then tell us about it! Email [email protected]) with your story.
To help other people find the show please leave a review on Apple Podcasts and tell your friends and co-workers
Links
Action IQ
Aster Data
Teradata
Filemaker
Hadoop
NoSQL
Hive
Informix
Parquet
Snowflake
Podcast Episode
Spark
Redshift
Unity Catalog
Customer Data Platform
CDP Market Guide
Kaizen
The intro and outro music is from The Hug by The Freak Fandango Orchestra / CC BY-SASponsored By:Starburst: 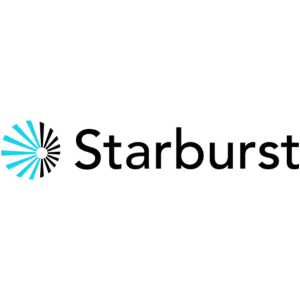
This episode is brought to you by Starburst - a data lake analytics platform for data engineers who are battling to build and scale high quality data pipelines on the data lake. Powered by Trino, Starburst runs petabyte-scale SQL analytics fast at a fraction of the cost of traditional methods, helping you meet all your data needs ranging from AI/ML workloads to data applications to complete analytics.
Trusted by the teams at Comcast and Doordash, Starburst delivers the adaptability and flexibility a lakehouse ecosystem promises, while providing a single point of access for your data and all your data governance allowing you to discover, transform, govern, and secure all in one place. Starburst does all of this on an open architecture with first-class support for Apache Iceberg, Delta Lake and Hudi, so you always maintain ownership of your data. Want to see Starburst in action? Try Starburst Galaxy today, the easiest and fastest way to get started using Trino, and get $500 of credits free. [dataengineeringpodcast.com/starburst](https://www.dataengineeringpodcast.com/starburst)Miro: 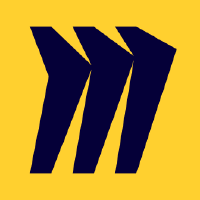
Data projects are notoriously complex. With multiple stakeholders to manage across varying backgrounds and toolchains even simple reports can become unwieldy to maintain. Miro is your single pane of glass where everyone can discover, track, and collaborate on your organization's data. I especially like the ability to combine your technical diagrams with data documentation and dependency mapping, allowing your data engineers and data consumers to communicate seamlessly about your projects. Find simplicity in your most complex projects with Miro. Your first three Miro boards are free when you sign up today at [dataengineeringpodcast.com/miro](https://www.dataengineeringpodcast.com/miro).Support Data Engineering Podcast
01:01:3322/01/2024
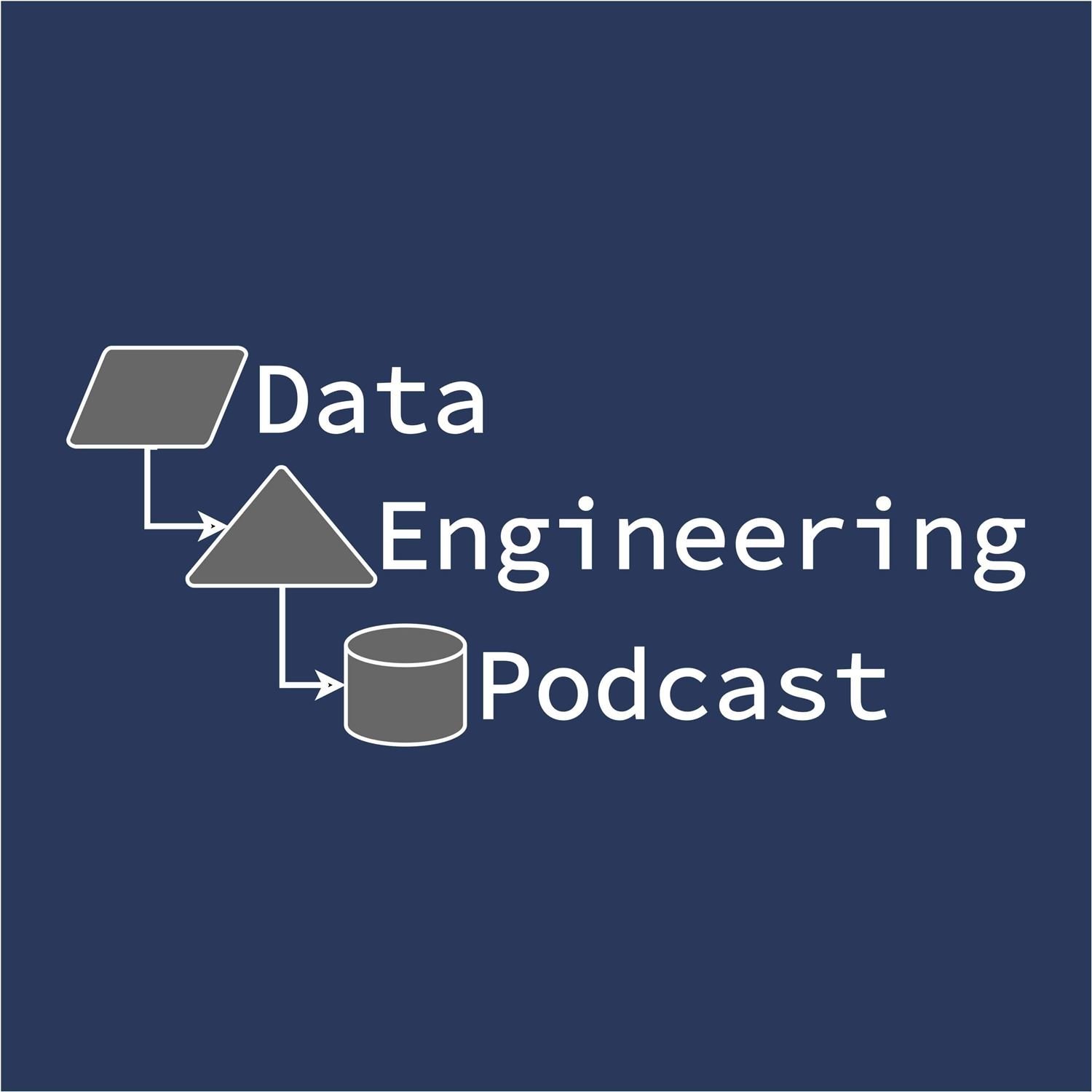
Pushing The Limits Of Scalability And User Experience For Data Processing WIth Jignesh Patel
Summary
Data processing technologies have dramatically improved in their sophistication and raw throughput. Unfortunately, the volumes of data that are being generated continue to double, requiring further advancements in the platform capabilities to keep up. As the sophistication increases, so does the complexity, leading to challenges for user experience. Jignesh Patel has been researching these areas for several years in his work as a professor at Carnegie Mellon University. In this episode he illuminates the landscape of problems that we are faced with and how his research is aimed at helping to solve these problems.
Announcements
Hello and welcome to the Data Engineering Podcast, the show about modern data management
Data lakes are notoriously complex. For data engineers who battle to build and scale high quality data workflows on the data lake, Starburst powers petabyte-scale SQL analytics fast, at a fraction of the cost of traditional methods, so that you can meet all your data needs ranging from AI to data applications to complete analytics. Trusted by teams of all sizes, including Comcast and Doordash, Starburst is a data lake analytics platform that delivers the adaptability and flexibility a lakehouse ecosystem promises. And Starburst does all of this on an open architecture with first-class support for Apache Iceberg, Delta Lake and Hudi, so you always maintain ownership of your data. Want to see Starburst in action? Go to dataengineeringpodcast.com/starburst and get $500 in credits to try Starburst Galaxy today, the easiest and fastest way to get started using Trino.
Your host is Tobias Macey and today I'm interviewing Jignesh Patel about the research that he is conducting on technical scalability and user experience improvements around data management
Interview
Introduction
How did you get involved in the area of data management?
Can you start by summarizing your current areas of research and the motivations behind them?
What are the open questions today in technical scalability of data engines?
What are the experimental methods that you are using to gain understanding in the opportunities and practical limits of those systems?
As you strive to push the limits of technical capacity in data systems, how does that impact the usability of the resulting systems?
When performing research and building prototypes of the projects, what is your process for incorporating user experience into the implementation of the product?
What are the main sources of tension between technical scalability and user experience/ease of comprehension?
What are some of the positive synergies that you have been able to realize between your teaching, research, and corporate activities?
In what ways do they produce conflict, whether personally or technically?
What are the most interesting, innovative, or unexpected ways that you have seen your research used?
What are the most interesting, unexpected, or challenging lessons that you have learned while working on research of the scalability limits of data systems?
What is your heuristic for when a given research project needs to be terminated or productionized?
What do you have planned for the future of your academic research?
Contact Info
Website
LinkedIn
Parting Question
From your perspective, what is the biggest gap in the tooling or technology for data management today?
Closing Announcements
Thank you for listening! Don't forget to check out our other shows. Podcast.__init__ covers the Python language, its community, and the innovative ways it is being used. The Machine Learning Podcast helps you go from idea to production with machine learning.
Visit the site to subscribe to the show, sign up for the mailing list, and read the show notes.
If you've learned something or tried out a project from the show then tell us about it! Email [email protected]) with your story.
To help other people find the show please leave a review on Apple Podcasts and tell your friends and co-workers
Links
Carnegie Mellon Universe
Parallel Databases
Genomics
Proteomics
Moore's Law
Dennard Scaling
Generative AI
Quantum Computing
Voltron Data
Podcast Episode
Von Neumann Architecture
Two's Complement
Ottertune
Podcast Episode
dbt
Informatica
Mozart Data
Podcast Episode
DataChat
Von Neumann Bottleneck
The intro and outro music is from The Hug by The Freak Fandango Orchestra / CC BY-SASponsored By:Starburst: 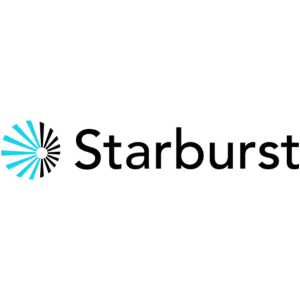
This episode is brought to you by Starburst - a data lake analytics platform for data engineers who are battling to build and scale high quality data pipelines on the data lake. Powered by Trino, Starburst runs petabyte-scale SQL analytics fast at a fraction of the cost of traditional methods, helping you meet all your data needs ranging from AI/ML workloads to data applications to complete analytics.
Trusted by the teams at Comcast and Doordash, Starburst delivers the adaptability and flexibility a lakehouse ecosystem promises, while providing a single point of access for your data and all your data governance allowing you to discover, transform, govern, and secure all in one place. Starburst does all of this on an open architecture with first-class support for Apache Iceberg, Delta Lake and Hudi, so you always maintain ownership of your data. Want to see Starburst in action? Try Starburst Galaxy today, the easiest and fastest way to get started using Trino, and get $500 of credits free. [dataengineeringpodcast.com/starburst](https://www.dataengineeringpodcast.com/starburst)Support Data Engineering Podcast
50:2607/01/2024
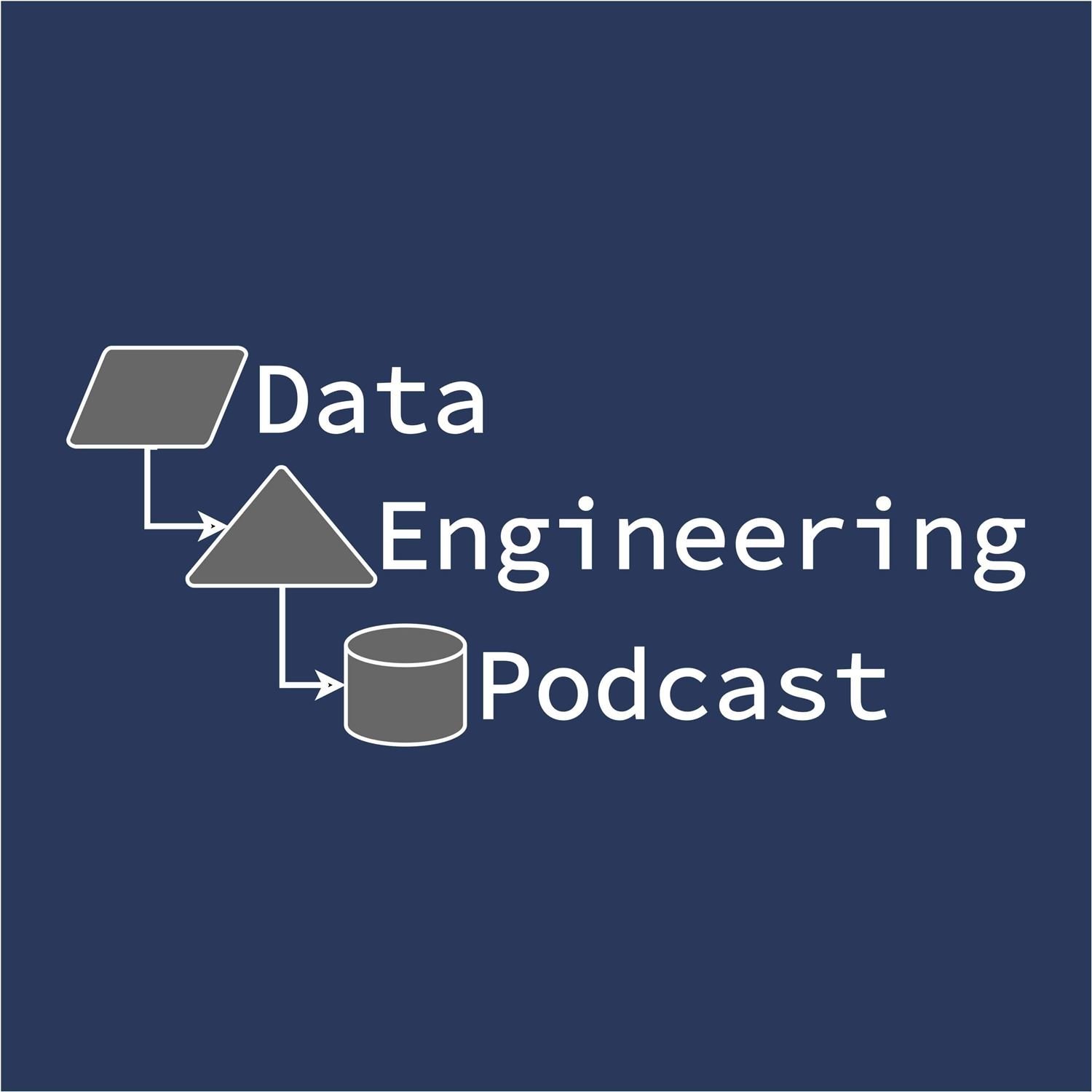
Designing Data Platforms For Fintech Companies
Summary
Working with financial data requires a high degree of rigor due to the numerous regulations and the risks involved in security breaches. In this episode Andrey Korchack, CTO of fintech startup Monite, discusses the complexities of designing and implementing a data platform in that sector.
Announcements
Hello and welcome to the Data Engineering Podcast, the show about modern data management
Data lakes are notoriously complex. For data engineers who battle to build and scale high quality data workflows on the data lake, Starburst powers petabyte-scale SQL analytics fast, at a fraction of the cost of traditional methods, so that you can meet all your data needs ranging from AI to data applications to complete analytics. Trusted by teams of all sizes, including Comcast and Doordash, Starburst is a data lake analytics platform that delivers the adaptability and flexibility a lakehouse ecosystem promises. And Starburst does all of this on an open architecture with first-class support for Apache Iceberg, Delta Lake and Hudi, so you always maintain ownership of your data. Want to see Starburst in action? Go to dataengineeringpodcast.com/starburst and get $500 in credits to try Starburst Galaxy today, the easiest and fastest way to get started using Trino.
Introducing RudderStack Profiles. RudderStack Profiles takes the SaaS guesswork and SQL grunt work out of building complete customer profiles so you can quickly ship actionable, enriched data to every downstream team. You specify the customer traits, then Profiles runs the joins and computations for you to create complete customer profiles. Get all of the details and try the new product today at dataengineeringpodcast.com/rudderstack
You shouldn't have to throw away the database to build with fast-changing data. You should be able to keep the familiarity of SQL and the proven architecture of cloud warehouses, but swap the decades-old batch computation model for an efficient incremental engine to get complex queries that are always up-to-date. With Materialize, you can! It’s the only true SQL streaming database built from the ground up to meet the needs of modern data products. Whether it’s real-time dashboarding and analytics, personalization and segmentation or automation and alerting, Materialize gives you the ability to work with fresh, correct, and scalable results — all in a familiar SQL interface. Go to dataengineeringpodcast.com/materialize today to get 2 weeks free!
Your host is Tobias Macey and today I'm interviewing Andrey Korchak about how to manage data in a fintech environment
Interview
Introduction
How did you get involved in the area of data management?
Can you start by summarizing the data challenges that are particular to the fintech ecosystem?
What are the primary sources and types of data that fintech organizations are working with?
What are the business-level capabilities that are dependent on this data?
How do the regulatory and business requirements influence the technology landscape in fintech organizations?
What does a typical build vs. buy decision process look like?
Fraud prediction in e.g. banks is one of the most well-established applications of machine learning in industry. What are some of the other ways that ML plays a part in fintech?
How does that influence the architectural design/capabilities for data platforms in those organizations?
Data governance is a notoriously challenging problem. What are some of the strategies that fintech companies are able to apply to this problem given their regulatory burdens?
What are the most interesting, innovative, or unexpected approaches to data management that you have seen in the fintech sector?
What are the most interesting, unexpected, or challenging lessons that you have learned while working on data in fintech?
What do you have planned for the future of your data capabilities at Monite?
Contact Info
LinkedIn
Parting Question
From your perspective, what is the biggest gap in the tooling or technology for data management today?
Closing Announcements
Thank you for listening! Don't forget to check out our other shows. Podcast.__init__ covers the Python language, its community, and the innovative ways it is being used. The Machine Learning Podcast helps you go from idea to production with machine learning.
Visit the site to subscribe to the show, sign up for the mailing list, and read the show notes.
If you've learned something or tried out a project from the show then tell us about it! Email [email protected]) with your story.
To help other people find the show please leave a review on Apple Podcasts and tell your friends and co-workers
Links
Monite
ISO 270001
Tesseract
GitOps
SWIFT Protocol
The intro and outro music is from The Hug by The Freak Fandango Orchestra / CC BY-SASponsored By:Starburst: 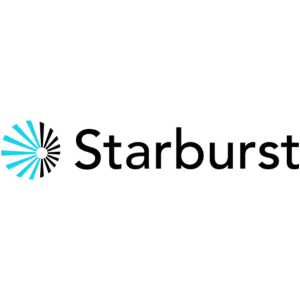
This episode is brought to you by Starburst - a data lake analytics platform for data engineers who are battling to build and scale high quality data pipelines on the data lake. Powered by Trino, Starburst runs petabyte-scale SQL analytics fast at a fraction of the cost of traditional methods, helping you meet all your data needs ranging from AI/ML workloads to data applications to complete analytics.
Trusted by the teams at Comcast and Doordash, Starburst delivers the adaptability and flexibility a lakehouse ecosystem promises, while providing a single point of access for your data and all your data governance allowing you to discover, transform, govern, and secure all in one place. Starburst does all of this on an open architecture with first-class support for Apache Iceberg, Delta Lake and Hudi, so you always maintain ownership of your data. Want to see Starburst in action? Try Starburst Galaxy today, the easiest and fastest way to get started using Trino, and get $500 of credits free. [dataengineeringpodcast.com/starburst](https://www.dataengineeringpodcast.com/starburst)Rudderstack: 
Introducing RudderStack Profiles. RudderStack Profiles takes the SaaS guesswork and SQL grunt work out of building complete customer profiles so you can quickly ship actionable, enriched data to every downstream team. You specify the customer traits, then Profiles runs the joins and computations for you to create complete customer profiles. Get all of the details and try the new product today at [dataengineeringpodcast.com/rudderstack](https://www.dataengineeringpodcast.com/rudderstack)Materialize: 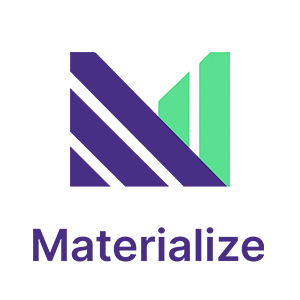
You shouldn't have to throw away the database to build with fast-changing data. Keep the familiar SQL, keep the proven architecture of cloud warehouses, but swap the decades-old batch computation model for an efficient incremental engine to get complex queries that are always up-to-date.
That is Materialize, the only true SQL streaming database built from the ground up to meet the needs of modern data products: Fresh, Correct, Scalable — all in a familiar SQL UI. Built on Timely Dataflow and Differential Dataflow, open source frameworks created by cofounder Frank McSherry at Microsoft Research, Materialize is trusted by data and engineering teams at Ramp, Pluralsight, Onward and more to build real-time data products without the cost, complexity, and development time of stream processing.
Go to [materialize.com](https://materialize.com/register/?utm_source=depodcast&utm_medium=paid&utm_campaign=early-access) today and get 2 weeks free!Support Data Engineering Podcast
47:5701/01/2024
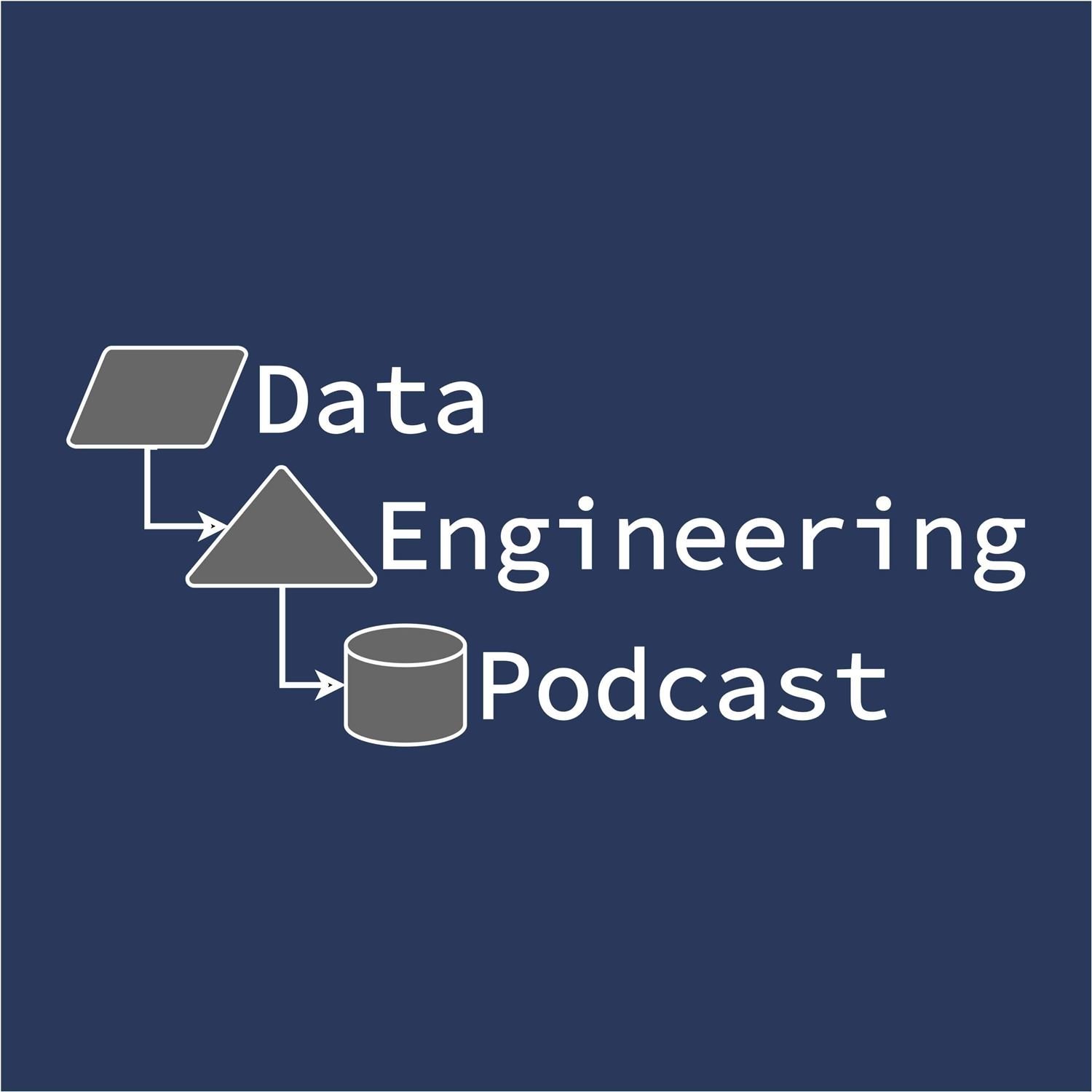
Troubleshooting Kafka In Production
Summary
Kafka has become a ubiquitous technology, offering a simple method for coordinating events and data across different systems. Operating it at scale, however, is notoriously challenging. Elad Eldor has experienced these challenges first-hand, leading to his work writing the book "Kafka: : Troubleshooting in Production". In this episode he highlights the sources of complexity that contribute to Kafka's operational difficulties, and some of the main ways to identify and mitigate potential sources of trouble.
Announcements
Hello and welcome to the Data Engineering Podcast, the show about modern data management
Introducing RudderStack Profiles. RudderStack Profiles takes the SaaS guesswork and SQL grunt work out of building complete customer profiles so you can quickly ship actionable, enriched data to every downstream team. You specify the customer traits, then Profiles runs the joins and computations for you to create complete customer profiles. Get all of the details and try the new product today at dataengineeringpodcast.com/rudderstack
You shouldn't have to throw away the database to build with fast-changing data. You should be able to keep the familiarity of SQL and the proven architecture of cloud warehouses, but swap the decades-old batch computation model for an efficient incremental engine to get complex queries that are always up-to-date. With Materialize, you can! It’s the only true SQL streaming database built from the ground up to meet the needs of modern data products. Whether it’s real-time dashboarding and analytics, personalization and segmentation or automation and alerting, Materialize gives you the ability to work with fresh, correct, and scalable results — all in a familiar SQL interface. Go to dataengineeringpodcast.com/materialize today to get 2 weeks free!
Data lakes are notoriously complex. For data engineers who battle to build and scale high quality data workflows on the data lake, Starburst powers petabyte-scale SQL analytics fast, at a fraction of the cost of traditional methods, so that you can meet all your data needs ranging from AI to data applications to complete analytics. Trusted by teams of all sizes, including Comcast and Doordash, Starburst is a data lake analytics platform that delivers the adaptability and flexibility a lakehouse ecosystem promises. And Starburst does all of this on an open architecture with first-class support for Apache Iceberg, Delta Lake and Hudi, so you always maintain ownership of your data. Want to see Starburst in action? Go to dataengineeringpodcast.com/starburst and get $500 in credits to try Starburst Galaxy today, the easiest and fastest way to get started using Trino.
Your host is Tobias Macey and today I'm interviewing Elad Eldor about operating Kafka in production and how to keep your clusters stable and performant
Interview
Introduction
How did you get involved in the area of data management?
Can you describe your experiences with Kafka?
What are the operational challenges that you have had to overcome while working with Kafka?
What motivated to write a book about how to manage Kafka in production?
There are many options now for persistent data queues. What are the factors to consider when determining whether Kafka is the right choice?
In the case where Kafka is the appropriate tool, there are many ways to run it now. What are the considerations that teams need to work through when determining whether/where/how to operate a cluster?
When provisioning a Kafka cluster, what are the requirements that need to be considered when determining the sizing?
What are the axes along which size/scale need to be determined?
The core promise of Kafka is that it is a durable store for continuous data. What are the mechanisms that are available for preventing data loss?
Under what circumstances can data be lost?
What are the different failure conditions that cluster operators need to be aware of?
What are the monitoring strategies that are most helpful for identifying (proactively or reactively) those errors?
In the event of these different cluster errors, what are the strategies for mitigating and recovering from those failures?
When a cluster's usage expands beyond the original designed capacity, what are the options/procedures for expanding that capacity?
When a cluster is underutilized, how can it be scaled down to reduce cost?
What are the most interesting, innovative, or unexpected ways that you have seen Kafka used?
What are the most interesting, unexpected, or challenging lessons that you have learned while working with Kafka?
When is Kafka the wrong choice?
What are the changes that you would like to see in Kafka to make it easier to operate?
Contact Info
LinkedIn
Parting Question
From your perspective, what is the biggest gap in the tooling or technology for data management today?
Closing Announcements
Thank you for listening! Don't forget to check out our other shows. Podcast.__init__ covers the Python language, its community, and the innovative ways it is being used. The Machine Learning Podcast helps you go from idea to production with machine learning.
Visit the site to subscribe to the show, sign up for the mailing list, and read the show notes.
If you've learned something or tried out a project from the show then tell us about it! Email [email protected]) with your story.
To help other people find the show please leave a review on Apple Podcasts and tell your friends and co-workers
Links
Kafka: Troubleshooting in Production book (affiliate link)
IronSource
Druid
Trino
Kafka
Spark
SRE == Site Reliability Engineer
Presto
System Performance by Brendan Gregg (affiliate link)
HortonWorks
RAID == Redundant Array of Inexpensive Disks
JBOD == Just a Bunch Of Disks
AWS MSK
Confluent
Aiven
JStat
Kafka Tiered Storage
Brendan Gregg iostat utilization explanation
The intro and outro music is from The Hug by The Freak Fandango Orchestra / CC BY-SASponsored By:Starburst: 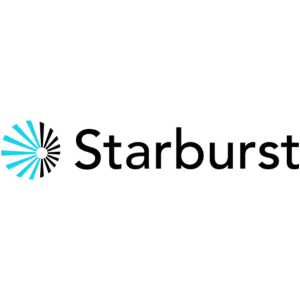
This episode is brought to you by Starburst - a data lake analytics platform for data engineers who are battling to build and scale high quality data pipelines on the data lake. Powered by Trino, Starburst runs petabyte-scale SQL analytics fast at a fraction of the cost of traditional methods, helping you meet all your data needs ranging from AI/ML workloads to data applications to complete analytics.
Trusted by the teams at Comcast and Doordash, Starburst delivers the adaptability and flexibility a lakehouse ecosystem promises, while providing a single point of access for your data and all your data governance allowing you to discover, transform, govern, and secure all in one place. Starburst does all of this on an open architecture with first-class support for Apache Iceberg, Delta Lake and Hudi, so you always maintain ownership of your data. Want to see Starburst in action? Try Starburst Galaxy today, the easiest and fastest way to get started using Trino, and get $500 of credits free. [dataengineeringpodcast.com/starburst](https://www.dataengineeringpodcast.com/starburst)Rudderstack: 
Introducing RudderStack Profiles. RudderStack Profiles takes the SaaS guesswork and SQL grunt work out of building complete customer profiles so you can quickly ship actionable, enriched data to every downstream team. You specify the customer traits, then Profiles runs the joins and computations for you to create complete customer profiles. Get all of the details and try the new product today at [dataengineeringpodcast.com/rudderstack](https://www.dataengineeringpodcast.com/rudderstack)Materialize: 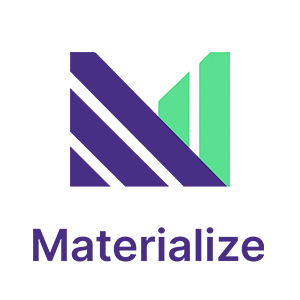
You shouldn't have to throw away the database to build with fast-changing data. Keep the familiar SQL, keep the proven architecture of cloud warehouses, but swap the decades-old batch computation model for an efficient incremental engine to get complex queries that are always up-to-date.
That is Materialize, the only true SQL streaming database built from the ground up to meet the needs of modern data products: Fresh, Correct, Scalable — all in a familiar SQL UI. Built on Timely Dataflow and Differential Dataflow, open source frameworks created by cofounder Frank McSherry at Microsoft Research, Materialize is trusted by data and engineering teams at Ramp, Pluralsight, Onward and more to build real-time data products without the cost, complexity, and development time of stream processing.
Go to [materialize.com](https://materialize.com/register/?utm_source=depodcast&utm_medium=paid&utm_campaign=early-access) today and get 2 weeks free!Support Data Engineering Podcast
01:14:4424/12/2023
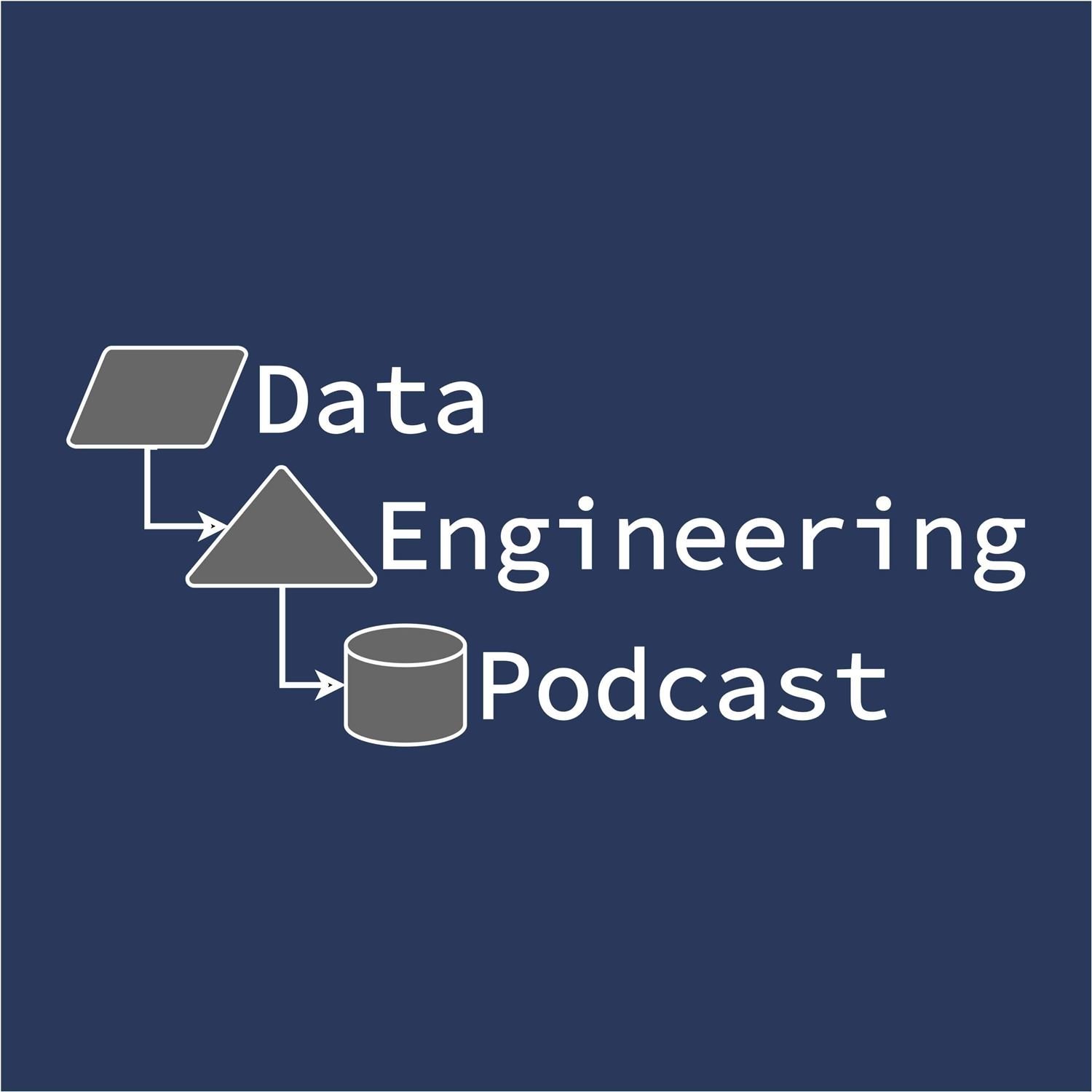
Adding An Easy Mode For The Modern Data Stack With 5X
Summary
The "modern data stack" promised a scalable, composable data platform that gave everyone the flexibility to use the best tools for every job. The reality was that it left data teams in the position of spending all of their engineering effort on integrating systems that weren't designed with compatible user experiences. The team at 5X understand the pain involved and the barriers to productivity and set out to solve it by pre-integrating the best tools from each layer of the stack. In this episode founder Tarush Aggarwal explains how the realities of the modern data stack are impacting data teams and the work that they are doing to accelerate time to value.
Announcements
Hello and welcome to the Data Engineering Podcast, the show about modern data management
Introducing RudderStack Profiles. RudderStack Profiles takes the SaaS guesswork and SQL grunt work out of building complete customer profiles so you can quickly ship actionable, enriched data to every downstream team. You specify the customer traits, then Profiles runs the joins and computations for you to create complete customer profiles. Get all of the details and try the new product today at dataengineeringpodcast.com/rudderstack
You shouldn't have to throw away the database to build with fast-changing data. You should be able to keep the familiarity of SQL and the proven architecture of cloud warehouses, but swap the decades-old batch computation model for an efficient incremental engine to get complex queries that are always up-to-date. With Materialize, you can! It’s the only true SQL streaming database built from the ground up to meet the needs of modern data products. Whether it’s real-time dashboarding and analytics, personalization and segmentation or automation and alerting, Materialize gives you the ability to work with fresh, correct, and scalable results — all in a familiar SQL interface. Go to dataengineeringpodcast.com/materialize today to get 2 weeks free!
Data lakes are notoriously complex. For data engineers who battle to build and scale high quality data workflows on the data lake, Starburst powers petabyte-scale SQL analytics fast, at a fraction of the cost of traditional methods, so that you can meet all your data needs ranging from AI to data applications to complete analytics. Trusted by teams of all sizes, including Comcast and Doordash, Starburst is a data lake analytics platform that delivers the adaptability and flexibility a lakehouse ecosystem promises. And Starburst does all of this on an open architecture with first-class support for Apache Iceberg, Delta Lake and Hudi, so you always maintain ownership of your data. Want to see Starburst in action? Go to dataengineeringpodcast.com/starburst and get $500 in credits to try Starburst Galaxy today, the easiest and fastest way to get started using Trino.
Your host is Tobias Macey and today I'm welcoming back Tarush Aggarwal to talk about what he and his team at 5x data are building to improve the user experience of the modern data stack.
Interview
Introduction
How did you get involved in the area of data management?
Can you describe what 5x is and the story behind it?
We last spoke in March of 2022. What are the notable changes in the 5x business and product?
What are the notable shifts in the data ecosystem that have influenced your adoption and product direction?
What trends are you most focused on tracking as you plan the continued evolution of your offerings?
What are the points of friction that teams run into when trying to build their data platform?
Can you describe design of the system that you have built?
What are the strategies that you rely on to support adaptability and speed of onboarding for new integrations?
What are some of the types of edge cases that you have to deal with while integrating and operating the platform implementations that you design for your customers?
What is your process for selection of vendors to support?
How would you characterize your relationships with the vendors that you rely on?
For customers who have pre-existing investment in a portion of the data stack, what is your process for engaging with them to understand how best to support their goals?
What are the most interesting, innovative, or unexpected ways that you have seen 5XData used?
What are the most interesting, unexpected, or challenging lessons that you have learned while working on 5XData?
When is 5X the wrong choice?
What do you have planned for the future of 5X?
Contact Info
LinkedIn
@tarush on Twitter
Parting Question
From your perspective, what is the biggest gap in the tooling or technology for data management today?
Closing Announcements
Thank you for listening! Don't forget to check out our other shows. Podcast.__init__ covers the Python language, its community, and the innovative ways it is being used. The Machine Learning Podcast helps you go from idea to production with machine learning.
Visit the site to subscribe to the show, sign up for the mailing list, and read the show notes.
If you've learned something or tried out a project from the show then tell us about it! Email [email protected]) with your story.
To help other people find the show please leave a review on Apple Podcasts and tell your friends and co-workers
Links
5X
Informatica
Snowflake
Podcast Episode
Looker
Podcast Episode
DuckDB
Podcast Episode
Redshift
Reverse ETL
Fivetran
Podcast Episode
Rudderstack
Podcast Episode
Peak.ai
The intro and outro music is from The Hug by The Freak Fandango Orchestra / CC BY-SASponsored By:Starburst: 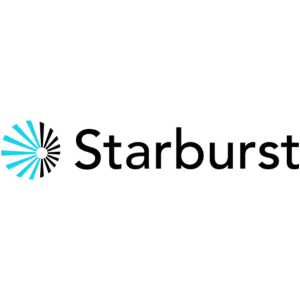
This episode is brought to you by Starburst - a data lake analytics platform for data engineers who are battling to build and scale high quality data pipelines on the data lake. Powered by Trino, Starburst runs petabyte-scale SQL analytics fast at a fraction of the cost of traditional methods, helping you meet all your data needs ranging from AI/ML workloads to data applications to complete analytics.
Trusted by the teams at Comcast and Doordash, Starburst delivers the adaptability and flexibility a lakehouse ecosystem promises, while providing a single point of access for your data and all your data governance allowing you to discover, transform, govern, and secure all in one place. Starburst does all of this on an open architecture with first-class support for Apache Iceberg, Delta Lake and Hudi, so you always maintain ownership of your data. Want to see Starburst in action? Try Starburst Galaxy today, the easiest and fastest way to get started using Trino, and get $500 of credits free. [dataengineeringpodcast.com/starburst](https://www.dataengineeringpodcast.com/starburst)Rudderstack: 
Introducing RudderStack Profiles. RudderStack Profiles takes the SaaS guesswork and SQL grunt work out of building complete customer profiles so you can quickly ship actionable, enriched data to every downstream team. You specify the customer traits, then Profiles runs the joins and computations for you to create complete customer profiles. Get all of the details and try the new product today at [dataengineeringpodcast.com/rudderstack](https://www.dataengineeringpodcast.com/rudderstack)Materialize: 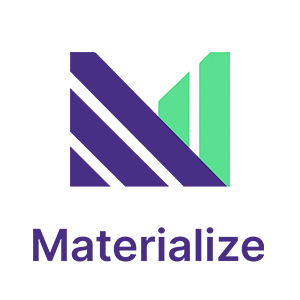
You shouldn't have to throw away the database to build with fast-changing data. Keep the familiar SQL, keep the proven architecture of cloud warehouses, but swap the decades-old batch computation model for an efficient incremental engine to get complex queries that are always up-to-date.
That is Materialize, the only true SQL streaming database built from the ground up to meet the needs of modern data products: Fresh, Correct, Scalable — all in a familiar SQL UI. Built on Timely Dataflow and Differential Dataflow, open source frameworks created by cofounder Frank McSherry at Microsoft Research, Materialize is trusted by data and engineering teams at Ramp, Pluralsight, Onward and more to build real-time data products without the cost, complexity, and development time of stream processing.
Go to [materialize.com](https://materialize.com/register/?utm_source=depodcast&utm_medium=paid&utm_campaign=early-access) today and get 2 weeks free!Support Data Engineering Podcast
56:1218/12/2023
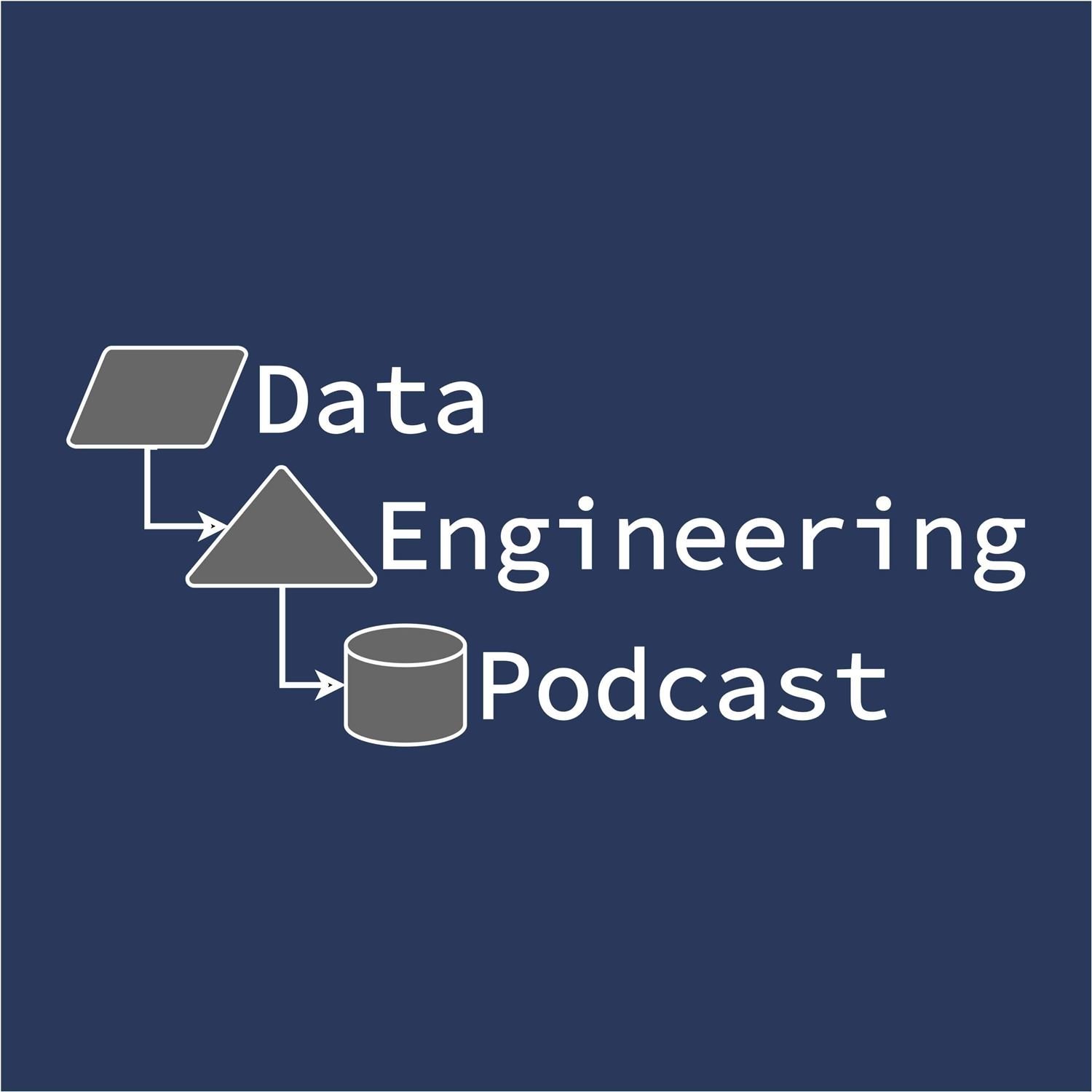
Run Your Own Anomaly Detection For Your Critical Business Metrics With Anomstack
Summary
If your business metrics looked weird tomorrow, would you know about it first? Anomaly detection is focused on identifying those outliers for you, so that you are the first to know when a business critical dashboard isn't right. Unfortunately, it can often be complex or expensive to incorporate anomaly detection into your data platform. Andrew Maguire got tired of solving that problem for each of the different roles he has ended up in, so he created the open source Anomstack project. In this episode he shares what it is, how it works, and how you can start using it today to get notified when the critical metrics in your business aren't quite right.
Announcements
Hello and welcome to the Data Engineering Podcast, the show about modern data management
You shouldn't have to throw away the database to build with fast-changing data. You should be able to keep the familiarity of SQL and the proven architecture of cloud warehouses, but swap the decades-old batch computation model for an efficient incremental engine to get complex queries that are always up-to-date. With Materialize, you can! It’s the only true SQL streaming database built from the ground up to meet the needs of modern data products. Whether it’s real-time dashboarding and analytics, personalization and segmentation or automation and alerting, Materialize gives you the ability to work with fresh, correct, and scalable results — all in a familiar SQL interface. Go to dataengineeringpodcast.com/materialize today to get 2 weeks free!
Introducing RudderStack Profiles. RudderStack Profiles takes the SaaS guesswork and SQL grunt work out of building complete customer profiles so you can quickly ship actionable, enriched data to every downstream team. You specify the customer traits, then Profiles runs the joins and computations for you to create complete customer profiles. Get all of the details and try the new product today at dataengineeringpodcast.com/rudderstack
Data projects are notoriously complex. With multiple stakeholders to manage across varying backgrounds and toolchains even simple reports can become unwieldy to maintain. Miro is your single pane of glass where everyone can discover, track, and collaborate on your organization's data. I especially like the ability to combine your technical diagrams with data documentation and dependency mapping, allowing your data engineers and data consumers to communicate seamlessly about your projects. Find simplicity in your most complex projects with Miro. Your first three Miro boards are free when you sign up today at dataengineeringpodcast.com/miro. That’s three free boards at dataengineeringpodcast.com/miro.
Data lakes are notoriously complex. For data engineers who battle to build and scale high quality data workflows on the data lake, Starburst powers petabyte-scale SQL analytics fast, at a fraction of the cost of traditional methods, so that you can meet all your data needs ranging from AI to data applications to complete analytics. Trusted by teams of all sizes, including Comcast and Doordash, Starburst is a data lake analytics platform that delivers the adaptability and flexibility a lakehouse ecosystem promises. And Starburst does all of this on an open architecture with first-class support for Apache Iceberg, Delta Lake and Hudi, so you always maintain ownership of your data. Want to see Starburst in action? Go to dataengineeringpodcast.com/starburst and get $500 in credits to try Starburst Galaxy today, the easiest and fastest way to get started using Trino.
Your host is Tobias Macey and today I'm interviewing Andrew Maguire about his work on the Anomstack project and how you can use it to run your own anomaly detection for your metrics
Interview
Introduction
How did you get involved in the area of data management?
Can you describe what Anomstack is and the story behind it?
What are your goals for this project?
What other tools/products might teams be evaluating while they consider Anomstack?
In the context of Anomstack, what constitutes a "metric"?
What are some examples of useful metrics that a data team might want to monitor?
You put in a lot of work to make Anomstack as easy as possible to get started with. How did this focus on ease of adoption influence the way that you approached the overall design of the project?
What are the core capabilities and constraints that you selected to provide the focus and architecture of the project?
Can you describe how Anomstack is implemented?
How have the design and goals of the project changed since you first started working on it?
What are the steps to getting Anomstack running and integrated as part of the operational fabric of a data platform?
What are the sharp edges that are still present in the system?
What are the interfaces that are available for teams to customize or enhance the capabilities of Anomstack?
What are the most interesting, innovative, or unexpected ways that you have seen Anomstack used?
What are the most interesting, unexpected, or challenging lessons that you have learned while working on Anomstack?
When is Anomstack the wrong choice?
What do you have planned for the future of Anomstack?
Contact Info
LinkedIn
Twitter
GitHub
Parting Question
From your perspective, what is the biggest gap in the tooling or technology for data management today?
Closing Announcements
Thank you for listening! Don't forget to check out our other shows. Podcast.__init__ covers the Python language, its community, and the innovative ways it is being used. The Machine Learning Podcast helps you go from idea to production with machine learning.
Visit the site to subscribe to the show, sign up for the mailing list, and read the show notes.
If you've learned something or tried out a project from the show then tell us about it! Email [email protected]) with your story.
To help other people find the show please leave a review on Apple Podcasts and tell your friends and co-workers
Links
Anomstack Github repo
Airflow Anomaly Detection Provider Github repo
Netdata
Metric Tree
Semantic Layer
Prometheus
Anodot
Chaos Genius
Metaplane
Anomalo
PyOD
Airflow
DuckDB
Anomstack Gallery
Dagster
InfluxDB
TimeGPT
Prophet
GreyKite
OpenLineage
The intro and outro music is from The Hug by The Freak Fandango Orchestra / CC BY-SASponsored By:Starburst: 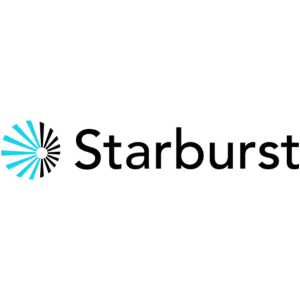
This episode is brought to you by Starburst - a data lake analytics platform for data engineers who are battling to build and scale high quality data pipelines on the data lake. Powered by Trino, Starburst runs petabyte-scale SQL analytics fast at a fraction of the cost of traditional methods, helping you meet all your data needs ranging from AI/ML workloads to data applications to complete analytics.
Trusted by the teams at Comcast and Doordash, Starburst delivers the adaptability and flexibility a lakehouse ecosystem promises, while providing a single point of access for your data and all your data governance allowing you to discover, transform, govern, and secure all in one place. Starburst does all of this on an open architecture with first-class support for Apache Iceberg, Delta Lake and Hudi, so you always maintain ownership of your data. Want to see Starburst in action? Try Starburst Galaxy today, the easiest and fastest way to get started using Trino, and get $500 of credits free. [dataengineeringpodcast.com/starburst](https://www.dataengineeringpodcast.com/starburst)Rudderstack: 
Introducing RudderStack Profiles. RudderStack Profiles takes the SaaS guesswork and SQL grunt work out of building complete customer profiles so you can quickly ship actionable, enriched data to every downstream team. You specify the customer traits, then Profiles runs the joins and computations for you to create complete customer profiles. Get all of the details and try the new product today at [dataengineeringpodcast.com/rudderstack](https://www.dataengineeringpodcast.com/rudderstack)Miro: 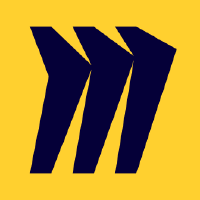
Data projects are notoriously complex. With multiple stakeholders to manage across varying backgrounds and toolchains even simple reports can become unwieldy to maintain. Miro is your single pane of glass where everyone can discover, track, and collaborate on your organization's data. I especially like the ability to combine your technical diagrams with data documentation and dependency mapping, allowing your data engineers and data consumers to communicate seamlessly about your projects. Find simplicity in your most complex projects with Miro. Your first three Miro boards are free when you sign up today at [dataengineeringpodcast.com/miro](https://www.dataengineeringpodcast.com/miro).Materialize: 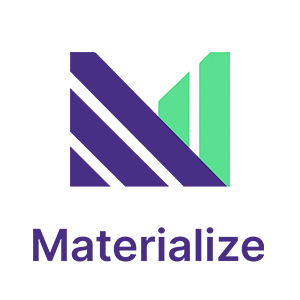
You shouldn't have to throw away the database to build with fast-changing data. Keep the familiar SQL, keep the proven architecture of cloud warehouses, but swap the decades-old batch computation model for an efficient incremental engine to get complex queries that are always up-to-date.
That is Materialize, the only true SQL streaming database built from the ground up to meet the needs of modern data products: Fresh, Correct, Scalable — all in a familiar SQL UI. Built on Timely Dataflow and Differential Dataflow, open source frameworks created by cofounder Frank McSherry at Microsoft Research, Materialize is trusted by data and engineering teams at Ramp, Pluralsight, Onward and more to build real-time data products without the cost, complexity, and development time of stream processing.
Go to [materialize.com](https://materialize.com/register/?utm_source=depodcast&utm_medium=paid&utm_campaign=early-access) today and get 2 weeks free!Support Data Engineering Podcast
51:1811/12/2023
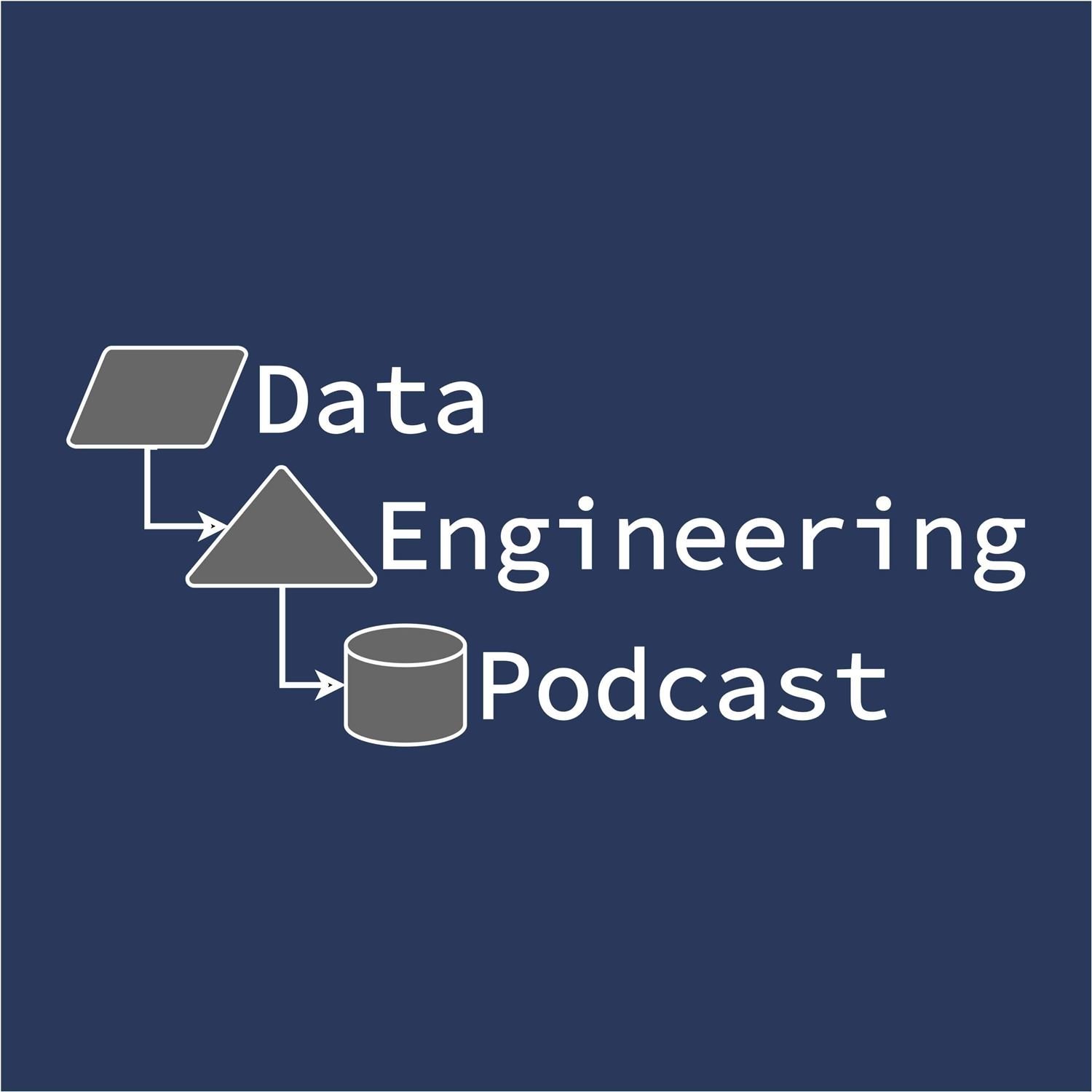
Designing Data Transfer Systems That Scale
Summary
The first step of data pipelines is to move the data to a place where you can process and prepare it for its eventual purpose. Data transfer systems are a critical component of data enablement, and building them to support large volumes of information is a complex endeavor. Andrei Tserakhau has dedicated his careeer to this problem, and in this episode he shares the lessons that he has learned and the work he is doing on his most recent data transfer system at DoubleCloud.
Announcements
Hello and welcome to the Data Engineering Podcast, the show about modern data management
Introducing RudderStack Profiles. RudderStack Profiles takes the SaaS guesswork and SQL grunt work out of building complete customer profiles so you can quickly ship actionable, enriched data to every downstream team. You specify the customer traits, then Profiles runs the joins and computations for you to create complete customer profiles. Get all of the details and try the new product today at dataengineeringpodcast.com/rudderstack
You shouldn't have to throw away the database to build with fast-changing data. You should be able to keep the familiarity of SQL and the proven architecture of cloud warehouses, but swap the decades-old batch computation model for an efficient incremental engine to get complex queries that are always up-to-date. With Materialize, you can! It’s the only true SQL streaming database built from the ground up to meet the needs of modern data products. Whether it’s real-time dashboarding and analytics, personalization and segmentation or automation and alerting, Materialize gives you the ability to work with fresh, correct, and scalable results — all in a familiar SQL interface. Go to dataengineeringpodcast.com/materialize today to get 2 weeks free!
This episode is brought to you by Datafold – a testing automation platform for data engineers that finds data quality issues for every part of your data workflow, from migration to deployment. Datafold has recently launched a 3-in-1 product experience to support accelerated data migrations. With Datafold, you can seamlessly plan, translate, and validate data across systems, massively accelerating your migration project. Datafold leverages cross-database diffing to compare tables across environments in seconds, column-level lineage for smarter migration planning, and a SQL translator to make moving your SQL scripts easier. Learn more about Datafold by visiting dataengineeringpodcast.com/datafold today!
Data lakes are notoriously complex. For data engineers who battle to build and scale high quality data workflows on the data lake, Starburst powers petabyte-scale SQL analytics fast, at a fraction of the cost of traditional methods, so that you can meet all your data needs ranging from AI to data applications to complete analytics. Trusted by teams of all sizes, including Comcast and Doordash, Starburst is a data lake analytics platform that delivers the adaptability and flexibility a lakehouse ecosystem promises. And Starburst does all of this on an open architecture with first-class support for Apache Iceberg, Delta Lake and Hudi, so you always maintain ownership of your data. Want to see Starburst in action? Go to dataengineeringpodcast.com/starburst and get $500 in credits to try Starburst Galaxy today, the easiest and fastest way to get started using Trino.
Your host is Tobias Macey and today I'm interviewing Andrei Tserakhau about operationalizing high bandwidth and low-latency change-data capture
Interview
Introduction
How did you get involved in the area of data management?
Your most recent project involves operationalizing a generalized data transfer service. What was the original problem that you were trying to solve?
What were the shortcomings of other options in the ecosystem that led you to building a new system?
What was the design of your initial solution to the problem?
What are the sharp edges that you had to deal with to operate and use that initial implementation?
What were the limitations of the system as you started to scale it?
Can you describe the current architecture of your data transfer platform?
What are the capabilities and constraints that you are optimizing for?
As you move beyond the initial use case that started you down this path, what are the complexities involved in generalizing to add new functionality or integrate with additional platforms?
What are the most interesting, innovative, or unexpected ways that you have seen your data transfer service used?
What are the most interesting, unexpected, or challenging lessons that you have learned while working on the data transfer system?
When is DoubleCloud Data Transfer the wrong choice?
What do you have planned for the future of DoubleCloud Data Transfer?
Contact Info
LinkedIn
Parting Question
From your perspective, what is the biggest gap in the tooling or technology for data management today?
Closing Announcements
Thank you for listening! Don't forget to check out our other shows. Podcast.__init__ covers the Python language, its community, and the innovative ways it is being used. The Machine Learning Podcast helps you go from idea to production with machine learning.
Visit the site to subscribe to the show, sign up for the mailing list, and read the show notes.
If you've learned something or tried out a project from the show then tell us about it! Email [email protected]) with your story.
To help other people find the show please leave a review on Apple Podcasts and tell your friends and co-workers
Links
DoubleCloud
Kafka
MapReduce
Change Data Capture
Clickhouse
Podcast Episode
Iceberg
Podcast Episode
Delta Lake
Podcast Episode
dbt
OpenMetadata
Podcast Episode
The intro and outro music is from The Hug by The Freak Fandango Orchestra / CC BY-SA
Speaker - Andrei Tserakhau, DoubleCloud Tech Lead. He has over 10 years of IT engineering experience and for the last 4 years was working on distributed systems with a focus on data delivery systems.Sponsored By:Starburst: 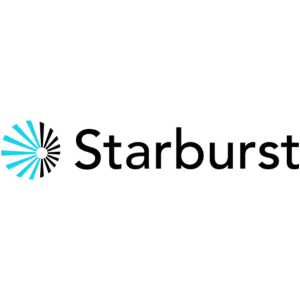
This episode is brought to you by Starburst - a data lake analytics platform for data engineers who are battling to build and scale high quality data pipelines on the data lake. Powered by Trino, Starburst runs petabyte-scale SQL analytics fast at a fraction of the cost of traditional methods, helping you meet all your data needs ranging from AI/ML workloads to data applications to complete analytics.
Trusted by the teams at Comcast and Doordash, Starburst delivers the adaptability and flexibility a lakehouse ecosystem promises, while providing a single point of access for your data and all your data governance allowing you to discover, transform, govern, and secure all in one place. Starburst does all of this on an open architecture with first-class support for Apache Iceberg, Delta Lake and Hudi, so you always maintain ownership of your data. Want to see Starburst in action? Try Starburst Galaxy today, the easiest and fastest way to get started using Trino, and get $500 of credits free. [dataengineeringpodcast.com/starburst](https://www.dataengineeringpodcast.com/starburst)Rudderstack: 
Introducing RudderStack Profiles. RudderStack Profiles takes the SaaS guesswork and SQL grunt work out of building complete customer profiles so you can quickly ship actionable, enriched data to every downstream team. You specify the customer traits, then Profiles runs the joins and computations for you to create complete customer profiles. Get all of the details and try the new product today at [dataengineeringpodcast.com/rudderstack](https://www.dataengineeringpodcast.com/rudderstack)Materialize: 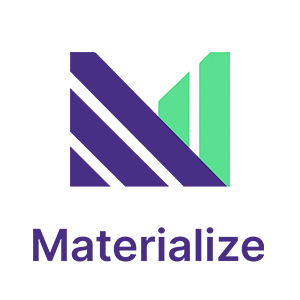
You shouldn't have to throw away the database to build with fast-changing data. Keep the familiar SQL, keep the proven architecture of cloud warehouses, but swap the decades-old batch computation model for an efficient incremental engine to get complex queries that are always up-to-date.
That is Materialize, the only true SQL streaming database built from the ground up to meet the needs of modern data products: Fresh, Correct, Scalable — all in a familiar SQL UI. Built on Timely Dataflow and Differential Dataflow, open source frameworks created by cofounder Frank McSherry at Microsoft Research, Materialize is trusted by data and engineering teams at Ramp, Pluralsight, Onward and more to build real-time data products without the cost, complexity, and development time of stream processing.
Go to [materialize.com](https://materialize.com/register/?utm_source=depodcast&utm_medium=paid&utm_campaign=early-access) today and get 2 weeks free!Datafold: 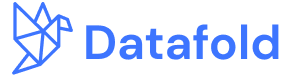
This episode is brought to you by Datafold – a testing automation platform for data engineers that finds data quality issues for every part of your data workflow, from migration to deployment. Datafold has recently launched a 3-in-1 product experience to support accelerated data migrations. With Datafold, you can seamlessly plan, translate, and validate data across systems, massively accelerating your migration project. Datafold leverages cross-database diffing to compare tables across environments in seconds, column-level lineage for smarter migration planning, and a SQL translator to make moving your SQL scripts easier. Learn more about Datafold by visiting [dataengineeringpodcast.com/datafold](https://www.dataengineeringpodcast.com/datafold) today!Support Data Engineering Podcast
01:03:5704/12/2023
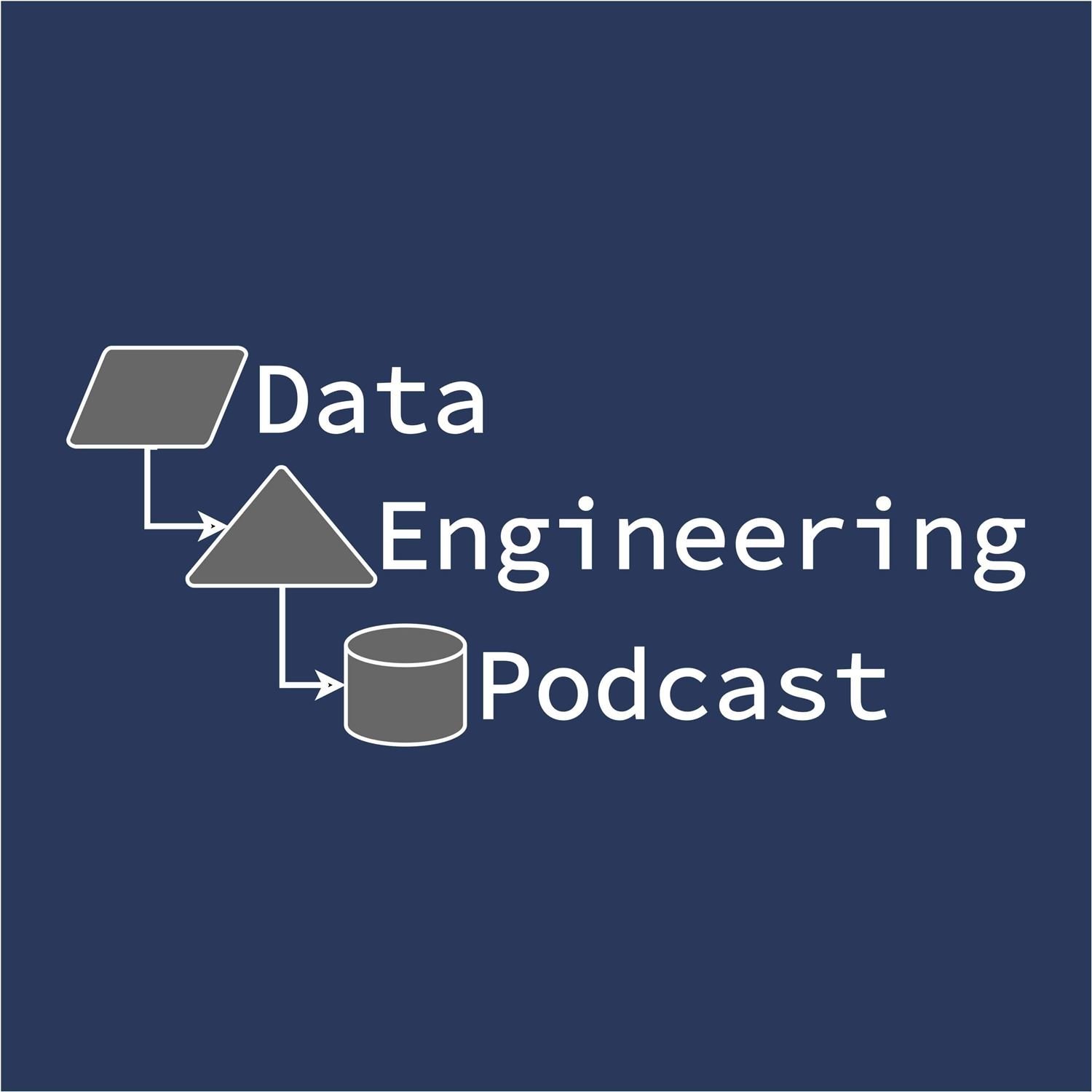
Addressing The Challenges Of Component Integration In Data Platform Architectures
Summary
Building a data platform that is enjoyable and accessible for all of its end users is a substantial challenge. One of the core complexities that needs to be addressed is the fractal set of integrations that need to be managed across the individual components. In this episode Tobias Macey shares his thoughts on the challenges that he is facing as he prepares to build the next set of architectural layers for his data platform to enable a larger audience to start accessing the data being managed by his team.
Announcements
Hello and welcome to the Data Engineering Podcast, the show about modern data management
Introducing RudderStack Profiles. RudderStack Profiles takes the SaaS guesswork and SQL grunt work out of building complete customer profiles so you can quickly ship actionable, enriched data to every downstream team. You specify the customer traits, then Profiles runs the joins and computations for you to create complete customer profiles. Get all of the details and try the new product today at dataengineeringpodcast.com/rudderstack
You shouldn't have to throw away the database to build with fast-changing data. You should be able to keep the familiarity of SQL and the proven architecture of cloud warehouses, but swap the decades-old batch computation model for an efficient incremental engine to get complex queries that are always up-to-date. With Materialize, you can! It’s the only true SQL streaming database built from the ground up to meet the needs of modern data products. Whether it’s real-time dashboarding and analytics, personalization and segmentation or automation and alerting, Materialize gives you the ability to work with fresh, correct, and scalable results — all in a familiar SQL interface. Go to dataengineeringpodcast.com/materialize today to get 2 weeks free!
Developing event-driven pipelines is going to be a lot easier - Meet Functions! Memphis functions enable developers and data engineers to build an organizational toolbox of functions to process, transform, and enrich ingested events “on the fly” in a serverless manner using AWS Lambda syntax, without boilerplate, orchestration, error handling, and infrastructure in almost any language, including Go, Python, JS, .NET, Java, SQL, and more. Go to dataengineeringpodcast.com/memphis today to get started!
Data lakes are notoriously complex. For data engineers who battle to build and scale high quality data workflows on the data lake, Starburst powers petabyte-scale SQL analytics fast, at a fraction of the cost of traditional methods, so that you can meet all your data needs ranging from AI to data applications to complete analytics. Trusted by teams of all sizes, including Comcast and Doordash, Starburst is a data lake analytics platform that delivers the adaptability and flexibility a lakehouse ecosystem promises. And Starburst does all of this on an open architecture with first-class support for Apache Iceberg, Delta Lake and Hudi, so you always maintain ownership of your data. Want to see Starburst in action? Go to dataengineeringpodcast.com/starburst and get $500 in credits to try Starburst Galaxy today, the easiest and fastest way to get started using Trino.
Your host is Tobias Macey and today I'll be sharing an update on my own journey of building a data platform, with a particular focus on the challenges of tool integration and maintaining a single source of truth
Interview
Introduction
How did you get involved in the area of data management?
data sharing
weight of history
existing integrations with dbt
switching cost for e.g. SQLMesh
de facto standard of Airflow
Single source of truth
permissions management across application layers
Database engine
Storage layer in a lakehouse
Presentation/access layer (BI)
Data flows
dbt -> table level lineage
orchestration engine -> pipeline flows
task based vs. asset based
Metadata platform as the logical place for horizontal view
Contact Info
LinkedIn
Website
Parting Question
From your perspective, what is the biggest gap in the tooling or technology for data management today?
Closing Announcements
Thank you for listening! Don't forget to check out our other shows. Podcast.__init__ covers the Python language, its community, and the innovative ways it is being used. The Machine Learning Podcast helps you go from idea to production with machine learning.
Visit the site to subscribe to the show, sign up for the mailing list, and read the show notes.
If you've learned something or tried out a project from the show then tell us about it! Email [email protected]) with your story.
To help other people find the show please leave a review on Apple Podcasts and tell your friends and co-workers
Links
Monologue Episode On Data Platform Design
Monologue Episode On Leaky Abstractions
Airbyte
Podcast Episode
Trino
Dagster
dbt
Snowflake
BigQuery
OpenMetadata
OpenLineage
Data Platform Shadow IT Episode
Preset
LightDash
Podcast Episode
SQLMesh
Podcast Episode
Airflow
Spark
Flink
Tabular
Iceberg
Open Policy Agent
The intro and outro music is from The Hug by The Freak Fandango Orchestra / CC BY-SASponsored By:Memphis: 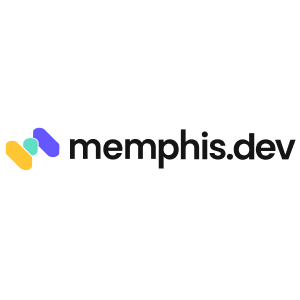
Developing event-driven pipelines is going to be a lot easier - Meet Functions!
Memphis functions enable developers and data engineers to build an organizational toolbox of functions to process, transform, and enrich ingested events “on the fly” in a serverless manner using AWS Lambda syntax, without boilerplate, orchestration, error handling, and infrastructure in almost any language, including Go, Python, JS, .NET, Java, SQL, and more. Go to [dataengineeringpodcast.com/memphis](https://www.dataengineeringpodcast.com/memphis) today to get started!Rudderstack: 
Introducing RudderStack Profiles. RudderStack Profiles takes the SaaS guesswork and SQL grunt work out of building complete customer profiles so you can quickly ship actionable, enriched data to every downstream team. You specify the customer traits, then Profiles runs the joins and computations for you to create complete customer profiles. Get all of the details and try the new product today at [dataengineeringpodcast.com/rudderstack](https://www.dataengineeringpodcast.com/rudderstack)Starburst: 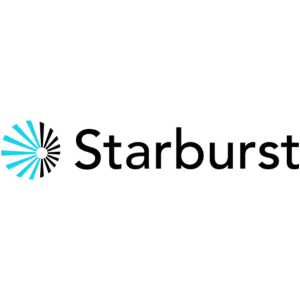
This episode is brought to you by Starburst - a data lake analytics platform for data engineers who are battling to build and scale high quality data pipelines on the data lake. Powered by Trino, Starburst runs petabyte-scale SQL analytics fast at a fraction of the cost of traditional methods, helping you meet all your data needs ranging from AI/ML workloads to data applications to complete analytics.
Trusted by the teams at Comcast and Doordash, Starburst delivers the adaptability and flexibility a lakehouse ecosystem promises, while providing a single point of access for your data and all your data governance allowing you to discover, transform, govern, and secure all in one place. Starburst does all of this on an open architecture with first-class support for Apache Iceberg, Delta Lake and Hudi, so you always maintain ownership of your data. Want to see Starburst in action? Try Starburst Galaxy today, the easiest and fastest way to get started using Trino, and get $500 of credits free. [dataengineeringpodcast.com/starburst](https://www.dataengineeringpodcast.com/starburst)Materialize: 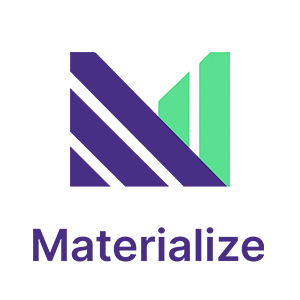
You shouldn't have to throw away the database to build with fast-changing data. Keep the familiar SQL, keep the proven architecture of cloud warehouses, but swap the decades-old batch computation model for an efficient incremental engine to get complex queries that are always up-to-date.
That is Materialize, the only true SQL streaming database built from the ground up to meet the needs of modern data products: Fresh, Correct, Scalable — all in a familiar SQL UI. Built on Timely Dataflow and Differential Dataflow, open source frameworks created by cofounder Frank McSherry at Microsoft Research, Materialize is trusted by data and engineering teams at Ramp, Pluralsight, Onward and more to build real-time data products without the cost, complexity, and development time of stream processing.
Go to [materialize.com](https://materialize.com/register/?utm_source=depodcast&utm_medium=paid&utm_campaign=early-access) today and get 2 weeks free!Support Data Engineering Podcast
29:4327/11/2023
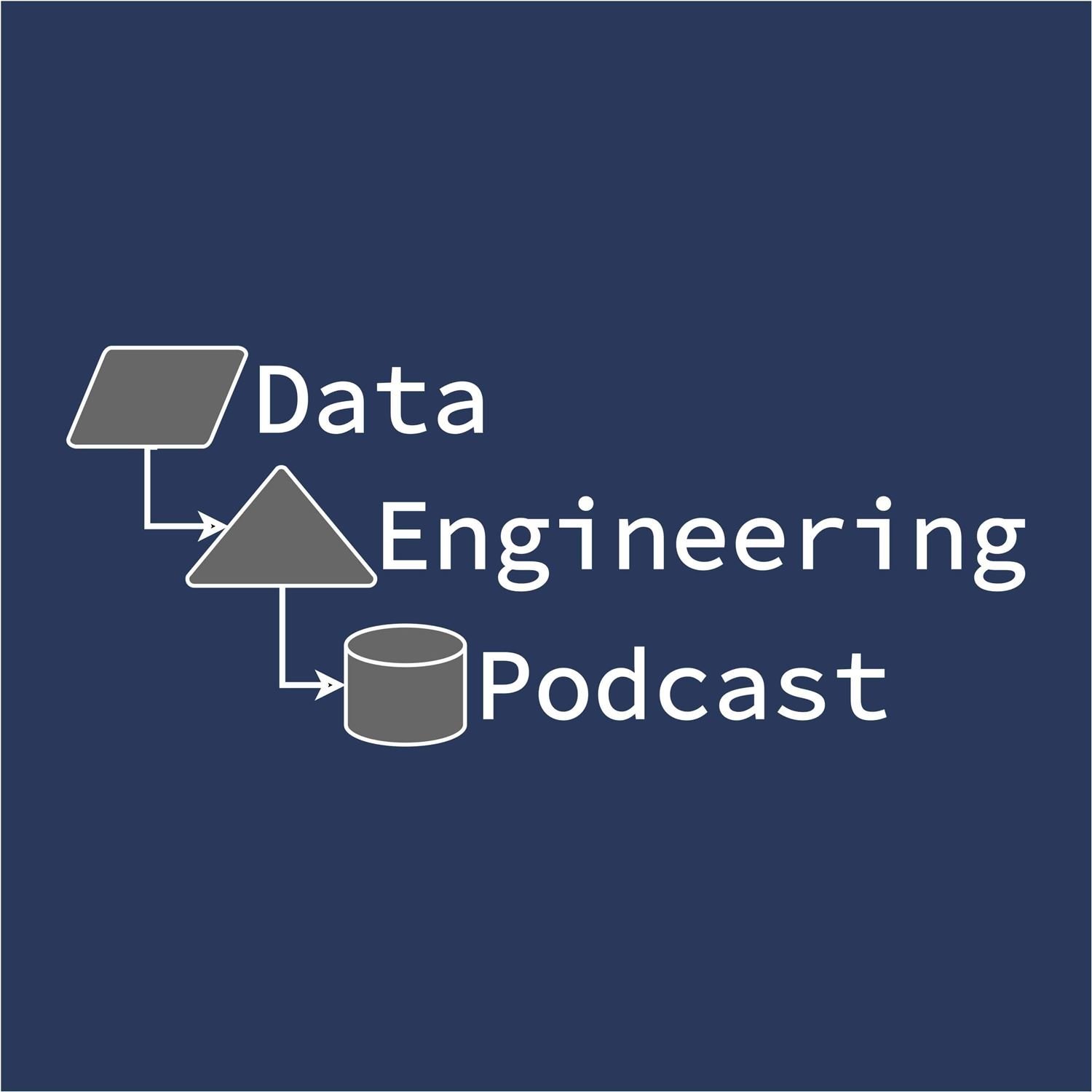
Unlocking Your dbt Projects With Practical Advice For Practitioners
Summary
The dbt project has become overwhelmingly popular across analytics and data engineering teams. While it is easy to adopt, there are many potential pitfalls. Dustin Dorsey and Cameron Cyr co-authored a practical guide to building your dbt project. In this episode they share their hard-won wisdom about how to build and scale your dbt projects.
Announcements
Hello and welcome to the Data Engineering Podcast, the show about modern data management
Data projects are notoriously complex. With multiple stakeholders to manage across varying backgrounds and toolchains even simple reports can become unwieldy to maintain. Miro is your single pane of glass where everyone can discover, track, and collaborate on your organization's data. I especially like the ability to combine your technical diagrams with data documentation and dependency mapping, allowing your data engineers and data consumers to communicate seamlessly about your projects. Find simplicity in your most complex projects with Miro. Your first three Miro boards are free when you sign up today at dataengineeringpodcast.com/miro. That’s three free boards at dataengineeringpodcast.com/miro.
Introducing RudderStack Profiles. RudderStack Profiles takes the SaaS guesswork and SQL grunt work out of building complete customer profiles so you can quickly ship actionable, enriched data to every downstream team. You specify the customer traits, then Profiles runs the joins and computations for you to create complete customer profiles. Get all of the details and try the new product today at dataengineeringpodcast.com/rudderstack
You shouldn't have to throw away the database to build with fast-changing data. You should be able to keep the familiarity of SQL and the proven architecture of cloud warehouses, but swap the decades-old batch computation model for an efficient incremental engine to get complex queries that are always up-to-date. With Materialize, you can! It’s the only true SQL streaming database built from the ground up to meet the needs of modern data products. Whether it’s real-time dashboarding and analytics, personalization and segmentation or automation and alerting, Materialize gives you the ability to work with fresh, correct, and scalable results — all in a familiar SQL interface. Go to dataengineeringpodcast.com/materialize today to get 2 weeks free!
Data lakes are notoriously complex. For data engineers who battle to build and scale high quality data workflows on the data lake, Starburst powers petabyte-scale SQL analytics fast, at a fraction of the cost of traditional methods, so that you can meet all your data needs ranging from AI to data applications to complete analytics. Trusted by teams of all sizes, including Comcast and Doordash, Starburst is a data lake analytics platform that delivers the adaptability and flexibility a lakehouse ecosystem promises. And Starburst does all of this on an open architecture with first-class support for Apache Iceberg, Delta Lake and Hudi, so you always maintain ownership of your data. Want to see Starburst in action? Go to dataengineeringpodcast.com/starburst and get $500 in credits to try Starburst Galaxy today, the easiest and fastest way to get started using Trino.
Your host is Tobias Macey and today I'm interviewing Dustin Dorsey and Cameron Cyr about how to design your dbt projects
Interview
Introduction
How did you get involved in the area of data management?
What was your path to adoption of dbt?
What did you use prior to its existence?
When/why/how did you start using it?
What are some of the common challenges that teams experience when getting started with dbt?
How does prior experience in analytics and/or software engineering impact those outcomes?
You recently wrote a book to give a crash course in best practices for dbt. What motivated you to invest that time and effort?
What new lessons did you learn about dbt in the process of writing the book?
The introduction of dbt is largely responsible for catalyzing the growth of "analytics engineering". As practitioners in the space, what do you see as the net result of that trend?
What are the lessons that we all need to invest in independent of the tool?
For someone starting a new dbt project today, can you talk through the decisions that will be most critical for ensuring future success?
As dbt projects scale, what are the elements of technical debt that are most likely to slow down engineers?
What are the capabilities in the dbt framework that can be used to mitigate the effects of that debt?
What tools or processes outside of dbt can help alleviate the incidental complexity of a large dbt project?
What are the most interesting, innovative, or unexpected ways that you have seen dbt used?
What are the most interesting, unexpected, or challenging lessons that you have learned while working with dbt? (as engineers and/or as autors)
What is on your personal wish-list for the future of dbt (or its competition?)?
Contact Info
Dustin
LinkedIn
Cameron
LinkedIn
Parting Question
From your perspective, what is the biggest gap in the tooling or technology for data management today?
Closing Announcements
Thank you for listening! Don't forget to check out our other shows. Podcast.__init__ covers the Python language, its community, and the innovative ways it is being used. The Machine Learning Podcast helps you go from idea to production with machine learning.
Visit the site to subscribe to the show, sign up for the mailing list, and read the show notes.
If you've learned something or tried out a project from the show then tell us about it! Email [email protected]) with your story.
To help other people find the show please leave a review on Apple Podcasts and tell your friends and co-workers
Links
Biobot Analytic
Breezeway
dbt
Podcast Episode
Synapse Analytics
Snowflake
Podcast Episode
Fivetran
Podcast Episode
Analytics Power Hour
DDL == Data Definition Language
DML == Data Manipulation Language
dbt codegen
Unlocking dbt book (affiliate link)
dbt Mesh
dbt Semantic Layer
GitHub Actions
Metaplane
Podcast Episode
DataTune Conference
The intro and outro music is from The Hug by The Freak Fandango Orchestra / CC BY-SASponsored By:Miro: 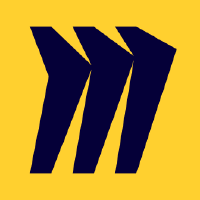
Data projects are notoriously complex. With multiple stakeholders to manage across varying backgrounds and toolchains even simple reports can become unwieldy to maintain. Miro is your single pane of glass where everyone can discover, track, and collaborate on your organization's data. I especially like the ability to combine your technical diagrams with data documentation and dependency mapping, allowing your data engineers and data consumers to communicate seamlessly about your projects. Find simplicity in your most complex projects with Miro. Your first three Miro boards are free when you sign up today at [dataengineeringpodcast.com/miro](https://www.dataengineeringpodcast.com/miro).Starburst: 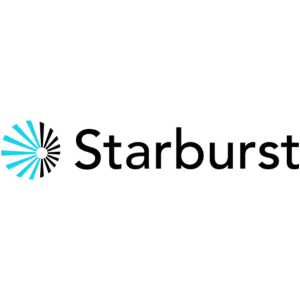
This episode is brought to you by Starburst - a data lake analytics platform for data engineers who are battling to build and scale high quality data pipelines on the data lake. Powered by Trino, Starburst runs petabyte-scale SQL analytics fast at a fraction of the cost of traditional methods, helping you meet all your data needs ranging from AI/ML workloads to data applications to complete analytics.
Trusted by the teams at Comcast and Doordash, Starburst delivers the adaptability and flexibility a lakehouse ecosystem promises, while providing a single point of access for your data and all your data governance allowing you to discover, transform, govern, and secure all in one place. Starburst does all of this on an open architecture with first-class support for Apache Iceberg, Delta Lake and Hudi, so you always maintain ownership of your data. Want to see Starburst in action? Try Starburst Galaxy today, the easiest and fastest way to get started using Trino, and get $500 of credits free. [dataengineeringpodcast.com/starburst](https://www.dataengineeringpodcast.com/starburst)Rudderstack: 
Introducing RudderStack Profiles. RudderStack Profiles takes the SaaS guesswork and SQL grunt work out of building complete customer profiles so you can quickly ship actionable, enriched data to every downstream team. You specify the customer traits, then Profiles runs the joins and computations for you to create complete customer profiles. Get all of the details and try the new product today at [dataengineeringpodcast.com/rudderstack](https://www.dataengineeringpodcast.com/rudderstack)Materialize: 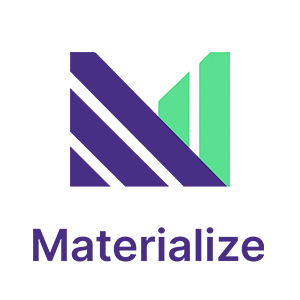
You shouldn't have to throw away the database to build with fast-changing data. Keep the familiar SQL, keep the proven architecture of cloud warehouses, but swap the decades-old batch computation model for an efficient incremental engine to get complex queries that are always up-to-date.
That is Materialize, the only true SQL streaming database built from the ground up to meet the needs of modern data products: Fresh, Correct, Scalable — all in a familiar SQL UI. Built on Timely Dataflow and Differential Dataflow, open source frameworks created by cofounder Frank McSherry at Microsoft Research, Materialize is trusted by data and engineering teams at Ramp, Pluralsight, Onward and more to build real-time data products without the cost, complexity, and development time of stream processing.
Go to [materialize.com](https://materialize.com/register/?utm_source=depodcast&utm_medium=paid&utm_campaign=early-access) today and get 2 weeks free!Support Data Engineering Podcast
01:16:0420/11/2023
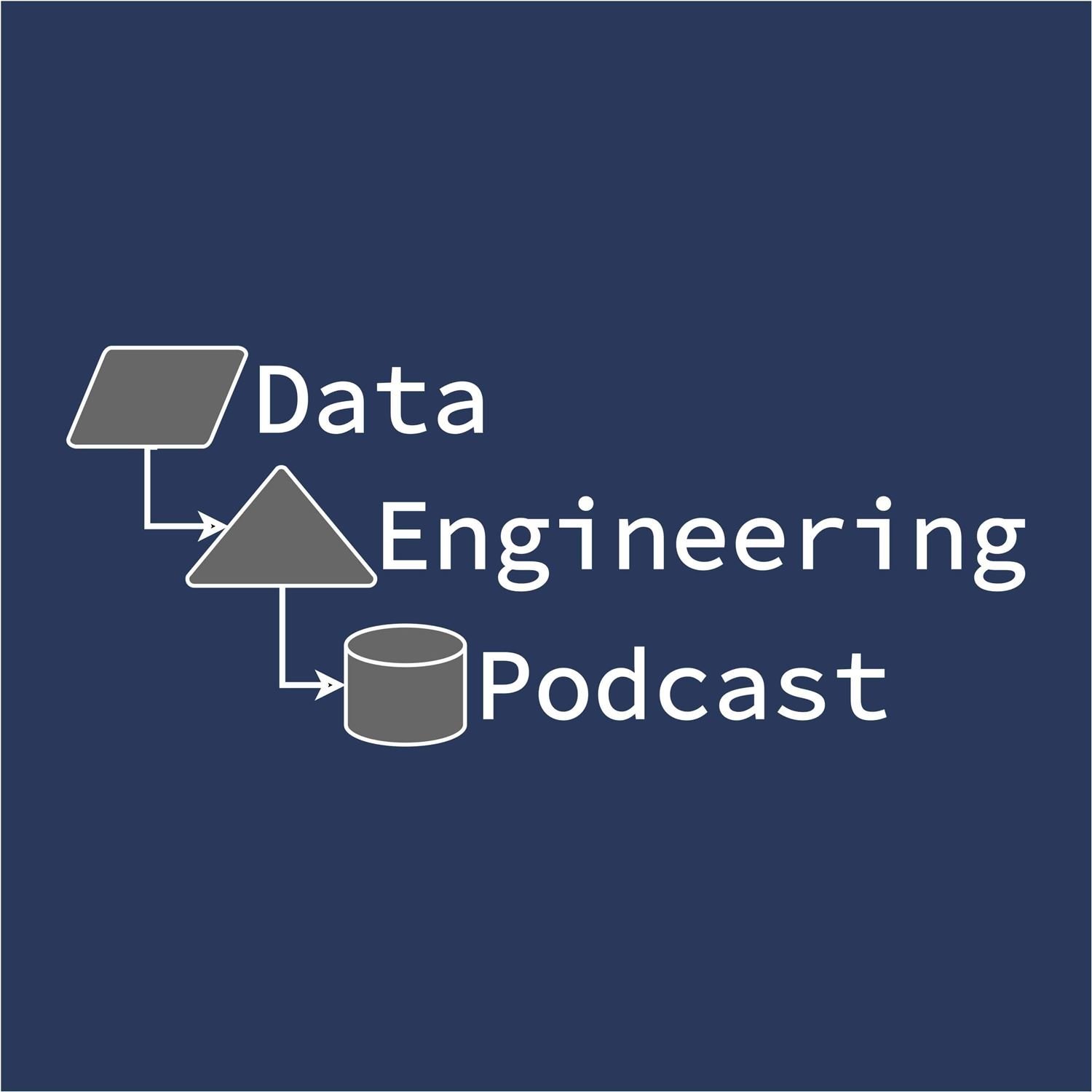
Enhancing The Abilities Of Software Engineers With Generative AI At Tabnine
Summary
Software development involves an interesting balance of creativity and repetition of patterns. Generative AI has accelerated the ability of developer tools to provide useful suggestions that speed up the work of engineers. Tabnine is one of the main platforms offering an AI powered assistant for software engineers. In this episode Eran Yahav shares the journey that he has taken in building this product and the ways that it enhances the ability of humans to get their work done, and when the humans have to adapt to the tool.
Announcements
Hello and welcome to the Data Engineering Podcast, the show about modern data management
Introducing RudderStack Profiles. RudderStack Profiles takes the SaaS guesswork and SQL grunt work out of building complete customer profiles so you can quickly ship actionable, enriched data to every downstream team. You specify the customer traits, then Profiles runs the joins and computations for you to create complete customer profiles. Get all of the details and try the new product today at dataengineeringpodcast.com/rudderstack
This episode is brought to you by Datafold – a testing automation platform for data engineers that finds data quality issues before the code and data are deployed to production. Datafold leverages data-diffing to compare production and development environments and column-level lineage to show you the exact impact of every code change on data, metrics, and BI tools, keeping your team productive and stakeholders happy. Datafold integrates with dbt, the modern data stack, and seamlessly plugs in your data CI for team-wide and automated testing. If you are migrating to a modern data stack, Datafold can also help you automate data and code validation to speed up the migration. Learn more about Datafold by visiting dataengineeringpodcast.com/datafold
Data lakes are notoriously complex. For data engineers who battle to build and scale high quality data workflows on the data lake, Starburst powers petabyte-scale SQL analytics fast, at a fraction of the cost of traditional methods, so that you can meet all your data needs ranging from AI to data applications to complete analytics. Trusted by teams of all sizes, including Comcast and Doordash, Starburst is a data lake analytics platform that delivers the adaptability and flexibility a lakehouse ecosystem promises. And Starburst does all of this on an open architecture with first-class support for Apache Iceberg, Delta Lake and Hudi, so you always maintain ownership of your data. Want to see Starburst in action? Go to dataengineeringpodcast.com/starburst and get $500 in credits to try Starburst Galaxy today, the easiest and fastest way to get started using Trino.
You shouldn't have to throw away the database to build with fast-changing data. You should be able to keep the familiarity of SQL and the proven architecture of cloud warehouses, but swap the decades-old batch computation model for an efficient incremental engine to get complex queries that are always up-to-date. With Materialize, you can! It’s the only true SQL streaming database built from the ground up to meet the needs of modern data products. Whether it’s real-time dashboarding and analytics, personalization and segmentation or automation and alerting, Materialize gives you the ability to work with fresh, correct, and scalable results — all in a familiar SQL interface. Go to dataengineeringpodcast.com/materialize today to get 2 weeks free!
Your host is Tobias Macey and today I'm interviewing Eran Yahav about building an AI powered developer assistant at Tabnine
Interview
Introduction
How did you get involved in machine learning?
Can you describe what Tabnine is and the story behind it?
What are the individual and organizational motivations for using AI to generate code?
What are the real-world limitations of generative AI for creating software? (e.g. size/complexity of the outputs, naming conventions, etc.)
What are the elements of skepticism/oversight that developers need to exercise while using a system like Tabnine?
What are some of the primary ways that developers interact with Tabnine during their development workflow?
Are there any particular styles of software for which an AI is more appropriate/capable? (e.g. webapps vs. data pipelines vs. exploratory analysis, etc.)
For natural languages there is a strong bias toward English in the current generation of LLMs. How does that translate into computer languages? (e.g. Python, Java, C++, etc.)
Can you describe the structure and implementation of Tabnine?
Do you rely primarily on a single core model, or do you have multiple models with subspecialization?
How have the design and goals of the product changed since you first started working on it?
What are the biggest challenges in building a custom LLM for code?
What are the opportunities for specialization of the model architecture given the highly structured nature of the problem domain?
For users of Tabnine, how do you assess/monitor the accuracy of recommendations?
What are the feedback and reinforcement mechanisms for the model(s)?
What are the most interesting, innovative, or unexpected ways that you have seen Tabnine's LLM powered coding assistant used?
What are the most interesting, unexpected, or challenging lessons that you have learned while working on AI assisted development at Tabnine?
When is an AI developer assistant the wrong choice?
What do you have planned for the future of Tabnine?
Contact Info
LinkedIn
Website
Parting Question
From your perspective, what is the biggest barrier to adoption of machine learning today?
Closing Announcements
Thank you for listening! Don't forget to check out our other shows. Podcast.__init__ covers the Python language, its community, and the innovative ways it is being used. The Machine Learning Podcast helps you go from idea to production with machine learning.
Visit the site to subscribe to the show, sign up for the mailing list, and read the show notes.
If you've learned something or tried out a project from the show then tell us about it! Email [email protected]) with your story.
To help other people find the show please leave a review on Apple Podcasts and tell your friends and co-workers
Links
TabNine
Technion University
Program Synthesis
Context Stuffing
Elixir
Dependency Injection
COBOL
Verilog
MidJourney
The intro and outro music is from Hitman's Lovesong feat. Paola Graziano by The Freak Fandango Orchestra/CC BY-SA 3.0Sponsored By:Starburst: 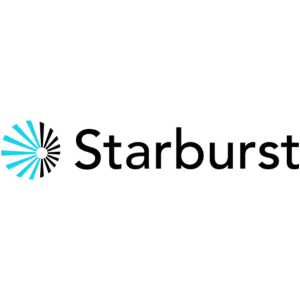
This episode is brought to you by Starburst - a data lake analytics platform for data engineers who are battling to build and scale high quality data pipelines on the data lake. Powered by Trino, Starburst runs petabyte-scale SQL analytics fast at a fraction of the cost of traditional methods, helping you meet all your data needs ranging from AI/ML workloads to data applications to complete analytics.
Trusted by the teams at Comcast and Doordash, Starburst delivers the adaptability and flexibility a lakehouse ecosystem promises, while providing a single point of access for your data and all your data governance allowing you to discover, transform, govern, and secure all in one place. Starburst does all of this on an open architecture with first-class support for Apache Iceberg, Delta Lake and Hudi, so you always maintain ownership of your data. Want to see Starburst in action? Try Starburst Galaxy today, the easiest and fastest way to get started using Trino, and get $500 of credits free. [dataengineeringpodcast.com/starburst](https://www.dataengineeringpodcast.com/starburst)Rudderstack: 
Introducing RudderStack Profiles. RudderStack Profiles takes the SaaS guesswork and SQL grunt work out of building complete customer profiles so you can quickly ship actionable, enriched data to every downstream team. You specify the customer traits, then Profiles runs the joins and computations for you to create complete customer profiles. Get all of the details and try the new product today at [dataengineeringpodcast.com/rudderstack](https://www.dataengineeringpodcast.com/rudderstack)Materialize: 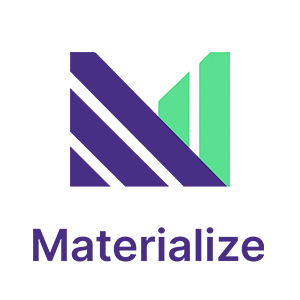
You shouldn't have to throw away the database to build with fast-changing data. Keep the familiar SQL, keep the proven architecture of cloud warehouses, but swap the decades-old batch computation model for an efficient incremental engine to get complex queries that are always up-to-date.
That is Materialize, the only true SQL streaming database built from the ground up to meet the needs of modern data products: Fresh, Correct, Scalable — all in a familiar SQL UI. Built on Timely Dataflow and Differential Dataflow, open source frameworks created by cofounder Frank McSherry at Microsoft Research, Materialize is trusted by data and engineering teams at Ramp, Pluralsight, Onward and more to build real-time data products without the cost, complexity, and development time of stream processing.
Go to [materialize.com](https://materialize.com/register/?utm_source=depodcast&utm_medium=paid&utm_campaign=early-access) today and get 2 weeks free!Datafold: 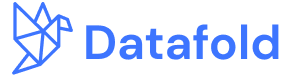
This episode is brought to you by Datafold – a testing automation platform for data engineers that finds data quality issues before the code and data are deployed to production. Datafold leverages data-diffing to compare production and development environments and column-level lineage to show you the exact impact of every code change on data, metrics, and BI tools, keeping your team productive and stakeholders happy. Datafold integrates with dbt, the modern data stack, and seamlessly plugs in your data CI for team-wide and automated testing. If you are migrating to a modern data stack, Datafold can also help you automate data and code validation to speed up the migration. Learn more about Datafold by visiting [dataengineeringpodcast.com/datafold](https://www.dataengineeringpodcast.com/datafold) today!Support Data Engineering Podcast
01:07:5213/11/2023
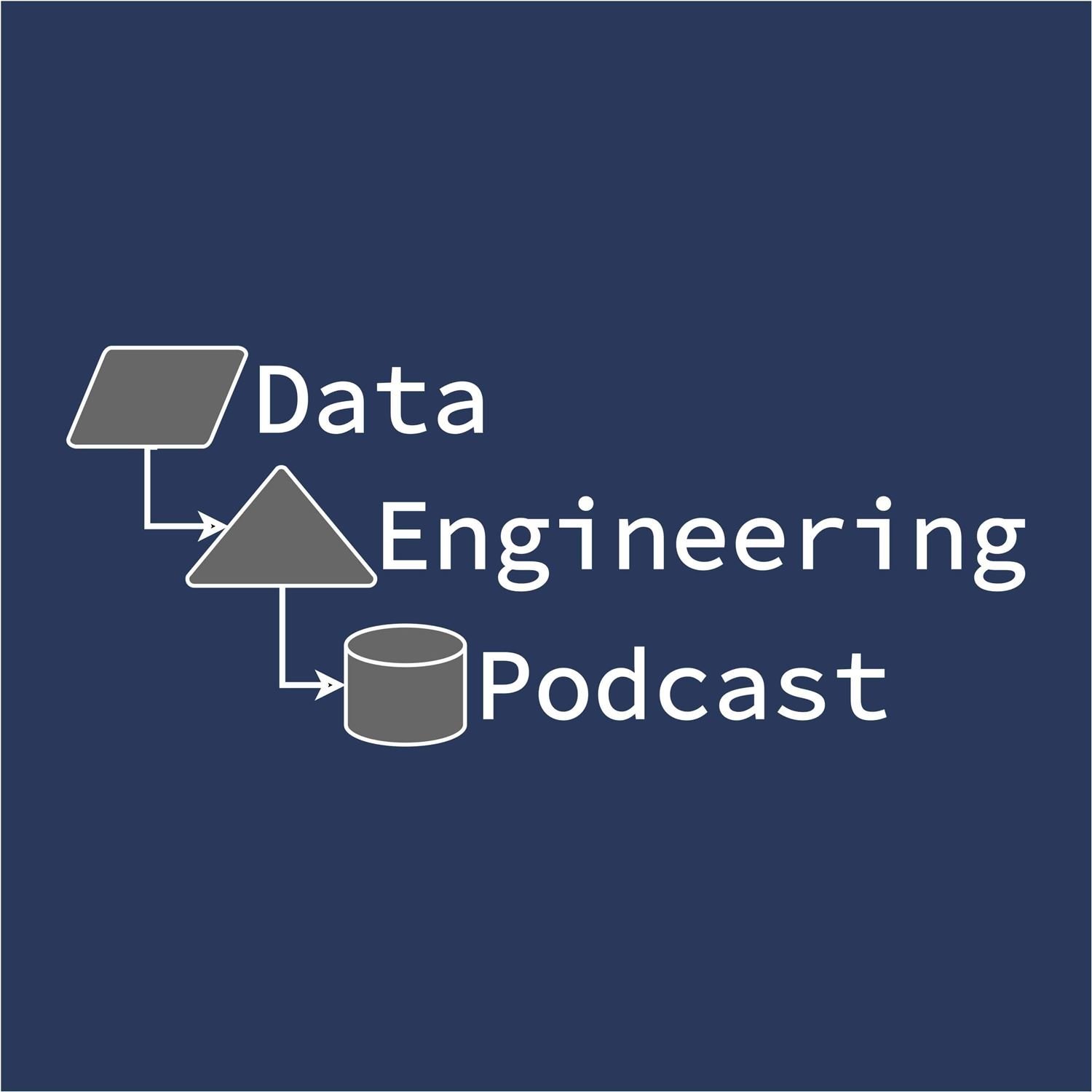
Shining Some Light In The Black Box Of PostgreSQL Performance
Summary
Databases are the core of most applications, but they are often treated as inscrutable black boxes. When an application is slow, there is a good probability that the database needs some attention. In this episode Lukas Fittl shares some hard-won wisdom about the causes and solution of many performance bottlenecks and the work that he is doing to shine some light on PostgreSQL to make it easier to understand how to keep it running smoothly.
Announcements
Hello and welcome to the Data Engineering Podcast, the show about modern data management
Introducing RudderStack Profiles. RudderStack Profiles takes the SaaS guesswork and SQL grunt work out of building complete customer profiles so you can quickly ship actionable, enriched data to every downstream team. You specify the customer traits, then Profiles runs the joins and computations for you to create complete customer profiles. Get all of the details and try the new product today at dataengineeringpodcast.com/rudderstack
You shouldn't have to throw away the database to build with fast-changing data. You should be able to keep the familiarity of SQL and the proven architecture of cloud warehouses, but swap the decades-old batch computation model for an efficient incremental engine to get complex queries that are always up-to-date. With Materialize, you can! It’s the only true SQL streaming database built from the ground up to meet the needs of modern data products. Whether it’s real-time dashboarding and analytics, personalization and segmentation or automation and alerting, Materialize gives you the ability to work with fresh, correct, and scalable results — all in a familiar SQL interface. Go to dataengineeringpodcast.com/materialize today to get 2 weeks free!
Data lakes are notoriously complex. For data engineers who battle to build and scale high quality data workflows on the data lake, Starburst powers petabyte-scale SQL analytics fast, at a fraction of the cost of traditional methods, so that you can meet all your data needs ranging from AI to data applications to complete analytics. Trusted by teams of all sizes, including Comcast and Doordash, Starburst is a data lake analytics platform that delivers the adaptability and flexibility a lakehouse ecosystem promises. And Starburst does all of this on an open architecture with first-class support for Apache Iceberg, Delta Lake and Hudi, so you always maintain ownership of your data. Want to see Starburst in action? Go to dataengineeringpodcast.com/starburst and get $500 in credits to try Starburst Galaxy today, the easiest and fastest way to get started using Trino.
This episode is brought to you by Datafold – a testing automation platform for data engineers that finds data quality issues before the code and data are deployed to production. Datafold leverages data-diffing to compare production and development environments and column-level lineage to show you the exact impact of every code change on data, metrics, and BI tools, keeping your team productive and stakeholders happy. Datafold integrates with dbt, the modern data stack, and seamlessly plugs in your data CI for team-wide and automated testing. If you are migrating to a modern data stack, Datafold can also help you automate data and code validation to speed up the migration. Learn more about Datafold by visiting dataengineeringpodcast.com/datafold
Your host is Tobias Macey and today I'm interviewing Lukas Fittl about optimizing your database performance and tips for tuning Postgres
Interview
Introduction
How did you get involved in the area of data management?
What are the different ways that database performance problems impact the business?
What are the most common contributors to performance issues?
What are the useful signals that indicate performance challenges in the database?
For a given symptom, what are the steps that you recommend for determining the proximate cause?
What are the potential negative impacts to be aware of when tuning the configuration of your database?
How does the database engine influence the methods used to identify and resolve performance challenges?
Most of the database engines that are in common use today have been around for decades. How have the lessons learned from running these systems over the years influenced the ways to think about designing new engines or evolving the ones we have today?
What are the most interesting, innovative, or unexpected ways that you have seen to address database performance?
What are the most interesting, unexpected, or challenging lessons that you have learned while working on databases?
What are your goals for the future of database engines?
Contact Info
LinkedIn
@LukasFittl on Twitter
Parting Question
From your perspective, what is the biggest gap in the tooling or technology for data management today?
Closing Announcements
Thank you for listening! Don't forget to check out our other shows. Podcast.__init__ covers the Python language, its community, and the innovative ways it is being used. The Machine Learning Podcast helps you go from idea to production with machine learning.
Visit the site to subscribe to the show, sign up for the mailing list, and read the show notes.
If you've learned something or tried out a project from the show then tell us about it! Email [email protected]) with your story.
To help other people find the show please leave a review on Apple Podcasts and tell your friends and co-workers
Links
PGAnalyze
Citus Data
Podcast Episode
ORM == Object Relational Mapper
N+1 Query
Autovacuum
Write-ahead Log
pg_stat_io
random_page_cost
pgvector
Vector Database
Ottertune
Podcast Episode
Citus Extension
Hydra
Clickhouse
Podcast Episode
MyISAM
MyRocks
InnoDB
Great Expectations
Podcast Episode
OpenTelemetry
The intro and outro music is from The Hug by The Freak Fandango Orchestra / CC BY-SASponsored By:Starburst: 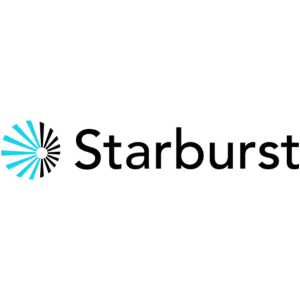
This episode is brought to you by Starburst - a data lake analytics platform for data engineers who are battling to build and scale high quality data pipelines on the data lake. Powered by Trino, Starburst runs petabyte-scale SQL analytics fast at a fraction of the cost of traditional methods, helping you meet all your data needs ranging from AI/ML workloads to data applications to complete analytics.
Trusted by the teams at Comcast and Doordash, Starburst delivers the adaptability and flexibility a lakehouse ecosystem promises, while providing a single point of access for your data and all your data governance allowing you to discover, transform, govern, and secure all in one place. Starburst does all of this on an open architecture with first-class support for Apache Iceberg, Delta Lake and Hudi, so you always maintain ownership of your data. Want to see Starburst in action? Try Starburst Galaxy today, the easiest and fastest way to get started using Trino, and get $500 of credits free. [dataengineeringpodcast.com/starburst](https://www.dataengineeringpodcast.com/starburst)Rudderstack: 
Introducing RudderStack Profiles. RudderStack Profiles takes the SaaS guesswork and SQL grunt work out of building complete customer profiles so you can quickly ship actionable, enriched data to every downstream team. You specify the customer traits, then Profiles runs the joins and computations for you to create complete customer profiles. Get all of the details and try the new product today at [dataengineeringpodcast.com/rudderstack](https://www.dataengineeringpodcast.com/rudderstack)Materialize: 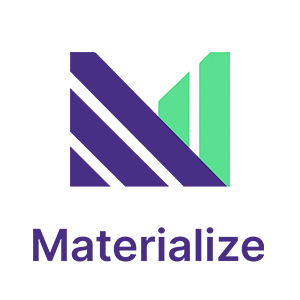
You shouldn't have to throw away the database to build with fast-changing data. Keep the familiar SQL, keep the proven architecture of cloud warehouses, but swap the decades-old batch computation model for an efficient incremental engine to get complex queries that are always up-to-date.
That is Materialize, the only true SQL streaming database built from the ground up to meet the needs of modern data products: Fresh, Correct, Scalable — all in a familiar SQL UI. Built on Timely Dataflow and Differential Dataflow, open source frameworks created by cofounder Frank McSherry at Microsoft Research, Materialize is trusted by data and engineering teams at Ramp, Pluralsight, Onward and more to build real-time data products without the cost, complexity, and development time of stream processing.
Go to [materialize.com](https://materialize.com/register/?utm_source=depodcast&utm_medium=paid&utm_campaign=early-access) today and get 2 weeks free!Datafold: 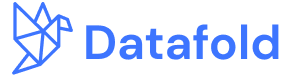
This episode is brought to you by Datafold – a testing automation platform for data engineers that finds data quality issues before the code and data are deployed to production. Datafold leverages data-diffing to compare production and development environments and column-level lineage to show you the exact impact of every code change on data, metrics, and BI tools, keeping your team productive and stakeholders happy. Datafold integrates with dbt, the modern data stack, and seamlessly plugs in your data CI for team-wide and automated testing. If you are migrating to a modern data stack, Datafold can also help you automate data and code validation to speed up the migration. Learn more about Datafold by visiting [dataengineeringpodcast.com/datafold](https://www.dataengineeringpodcast.com/datafold) today!Support Data Engineering Podcast
54:5206/11/2023
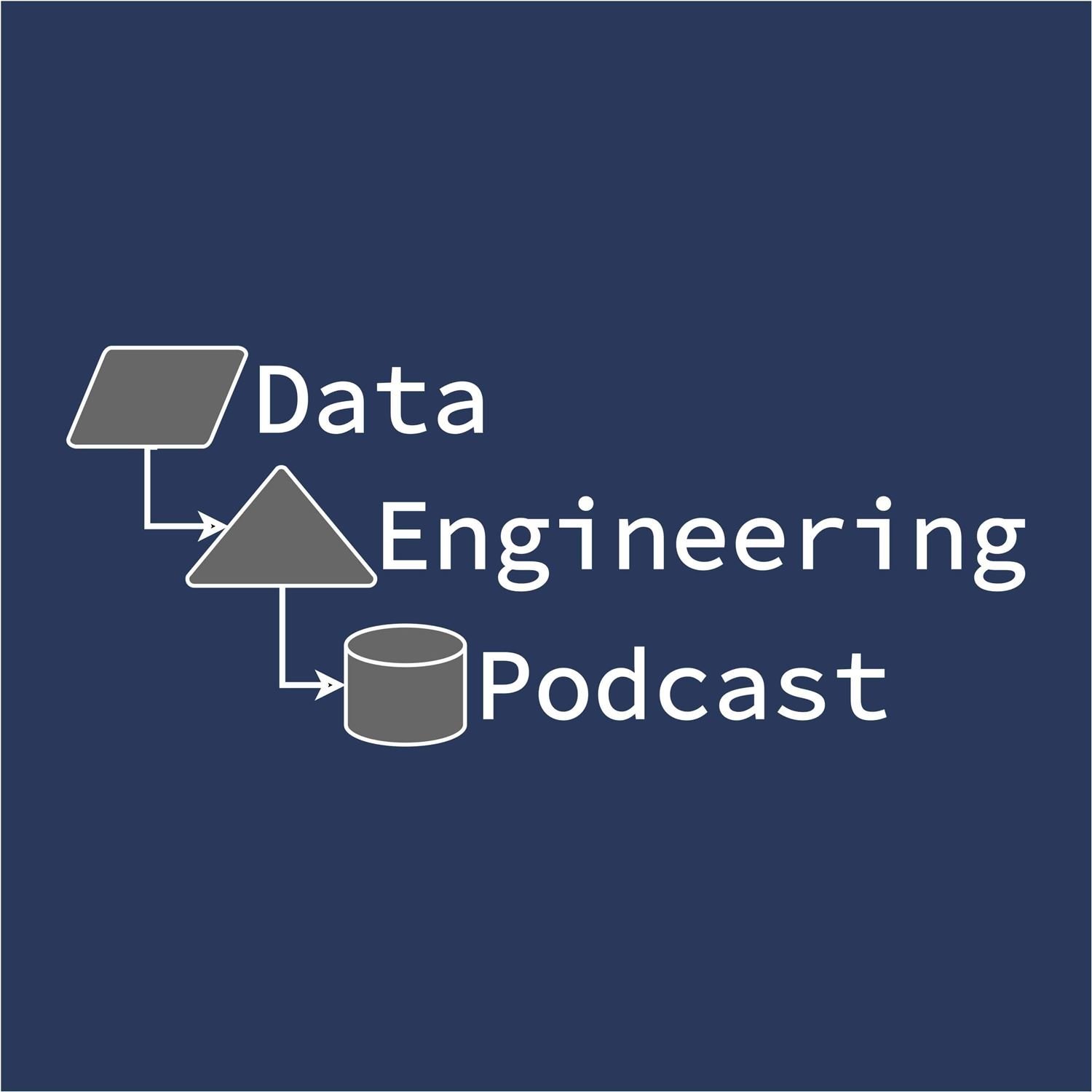
Surveying The Market Of Database Products
Summary
Databases are the core of most applications, whether transactional or analytical. In recent years the selection of database products has exploded, making the critical decision of which engine(s) to use even more difficult. In this episode Tanya Bragin shares her experiences as a product manager for two major vendors and the lessons that she has learned about how teams should approach the process of tool selection.
Announcements
Hello and welcome to the Data Engineering Podcast, the show about modern data management
Introducing RudderStack Profiles. RudderStack Profiles takes the SaaS guesswork and SQL grunt work out of building complete customer profiles so you can quickly ship actionable, enriched data to every downstream team. You specify the customer traits, then Profiles runs the joins and computations for you to create complete customer profiles. Get all of the details and try the new product today at dataengineeringpodcast.com/rudderstack
You shouldn't have to throw away the database to build with fast-changing data. You should be able to keep the familiarity of SQL and the proven architecture of cloud warehouses, but swap the decades-old batch computation model for an efficient incremental engine to get complex queries that are always up-to-date. With Materialize, you can! It’s the only true SQL streaming database built from the ground up to meet the needs of modern data products. Whether it’s real-time dashboarding and analytics, personalization and segmentation or automation and alerting, Materialize gives you the ability to work with fresh, correct, and scalable results — all in a familiar SQL interface. Go to dataengineeringpodcast.com/materialize today to get 2 weeks free!
This episode is brought to you by Datafold – a testing automation platform for data engineers that finds data quality issues before the code and data are deployed to production. Datafold leverages data-diffing to compare production and development environments and column-level lineage to show you the exact impact of every code change on data, metrics, and BI tools, keeping your team productive and stakeholders happy. Datafold integrates with dbt, the modern data stack, and seamlessly plugs in your data CI for team-wide and automated testing. If you are migrating to a modern data stack, Datafold can also help you automate data and code validation to speed up the migration. Learn more about Datafold by visiting dataengineeringpodcast.com/datafold
Data projects are notoriously complex. With multiple stakeholders to manage across varying backgrounds and toolchains even simple reports can become unwieldy to maintain. Miro is your single pane of glass where everyone can discover, track, and collaborate on your organization's data. I especially like the ability to combine your technical diagrams with data documentation and dependency mapping, allowing your data engineers and data consumers to communicate seamlessly about your projects. Find simplicity in your most complex projects with Miro. Your first three Miro boards are free when you sign up today at dataengineeringpodcast.com/miro. That’s three free boards at dataengineeringpodcast.com/miro.
Your host is Tobias Macey and today I'm interviewing Tanya Bragin about her views on the database products market
Interview
Introduction
How did you get involved in the area of data management?
What are the aspects of the database market that keep you interested as a VP of product?
How have your experiences at Elastic informed your current work at Clickhouse?
What are the main product categories for databases today?
What are the industry trends that have the most impact on the development and growth of different product categories?
Which categories do you see growing the fastest?
When a team is selecting a database technology for a given task, what are the types of questions that they should be asking?
Transactional engines like Postgres, SQL Server, Oracle, etc. were long used as analytical databases as well. What is driving the broad adoption of columnar stores as a separate environment from transactional systems?
What are the inefficiencies/complexities that this introduces?
How can the database engine used for analytical systems work more closely with the transactional systems?
When building analytical systems there are numerous moving parts with intricate dependencies. What is the role of the database in simplifying observability of these applications?
What are the most interesting, innovative, or unexpected ways that you have seen Clickhouse used?
What are the most interesting, unexpected, or challenging lessons that you have learned while working on database products?
What are your prodictions for the future of the database market?
Contact Info
LinkedIn
Parting Question
From your perspective, what is the biggest gap in the tooling or technology for data management today?
Closing Announcements
Thank you for listening! Don't forget to check out our other shows. Podcast.__init__ covers the Python language, its community, and the innovative ways it is being used. The Machine Learning Podcast helps you go from idea to production with machine learning.
Visit the site to subscribe to the show, sign up for the mailing list, and read the show notes.
If you've learned something or tried out a project from the show then tell us about it! Email [email protected]) with your story.
To help other people find the show please leave a review on Apple Podcasts and tell your friends and co-workers
Links
Clickhouse
Podcast Episode
Elastic
OLAP
OLTP
Graph Database
Vector Database
Trino
Presto
Foreign data wrapper
dbt
Podcast Episode
OpenTelemetry
Iceberg
Podcast Episode
Parquet
The intro and outro music is from The Hug by The Freak Fandango Orchestra / CC BY-SASponsored By:Miro: 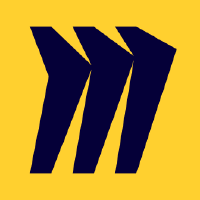
Data projects are notoriously complex. With multiple stakeholders to manage across varying backgrounds and toolchains even simple reports can become unwieldy to maintain. Miro is your single pane of glass where everyone can discover, track, and collaborate on your organization's data. I especially like the ability to combine your technical diagrams with data documentation and dependency mapping, allowing your data engineers and data consumers to communicate seamlessly about your projects. Find simplicity in your most complex projects with Miro. Your first three Miro boards are free when you sign up today at [dataengineeringpodcast.com/miro](https://www.dataengineeringpodcast.com/miro).Rudderstack: 
Introducing RudderStack Profiles. RudderStack Profiles takes the SaaS guesswork and SQL grunt work out of building complete customer profiles so you can quickly ship actionable, enriched data to every downstream team. You specify the customer traits, then Profiles runs the joins and computations for you to create complete customer profiles. Get all of the details and try the new product today at [dataengineeringpodcast.com/rudderstack](https://www.dataengineeringpodcast.com/rudderstack)Materialize: 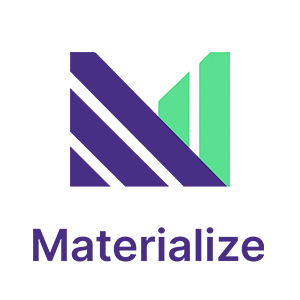
You shouldn't have to throw away the database to build with fast-changing data. Keep the familiar SQL, keep the proven architecture of cloud warehouses, but swap the decades-old batch computation model for an efficient incremental engine to get complex queries that are always up-to-date.
That is Materialize, the only true SQL streaming database built from the ground up to meet the needs of modern data products: Fresh, Correct, Scalable — all in a familiar SQL UI. Built on Timely Dataflow and Differential Dataflow, open source frameworks created by cofounder Frank McSherry at Microsoft Research, Materialize is trusted by data and engineering teams at Ramp, Pluralsight, Onward and more to build real-time data products without the cost, complexity, and development time of stream processing.
Go to [materialize.com](https://materialize.com/register/?utm_source=depodcast&utm_medium=paid&utm_campaign=early-access) today and get 2 weeks free!Datafold: 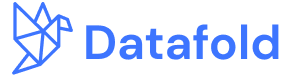
This episode is brought to you by Datafold – a testing automation platform for data engineers that finds data quality issues before the code and data are deployed to production. Datafold leverages data-diffing to compare production and development environments and column-level lineage to show you the exact impact of every code change on data, metrics, and BI tools, keeping your team productive and stakeholders happy. Datafold integrates with dbt, the modern data stack, and seamlessly plugs in your data CI for team-wide and automated testing. If you are migrating to a modern data stack, Datafold can also help you automate data and code validation to speed up the migration. Learn more about Datafold by visiting [dataengineeringpodcast.com/datafold](https://www.dataengineeringpodcast.com/datafold) today!Support Data Engineering Podcast
47:1230/10/2023
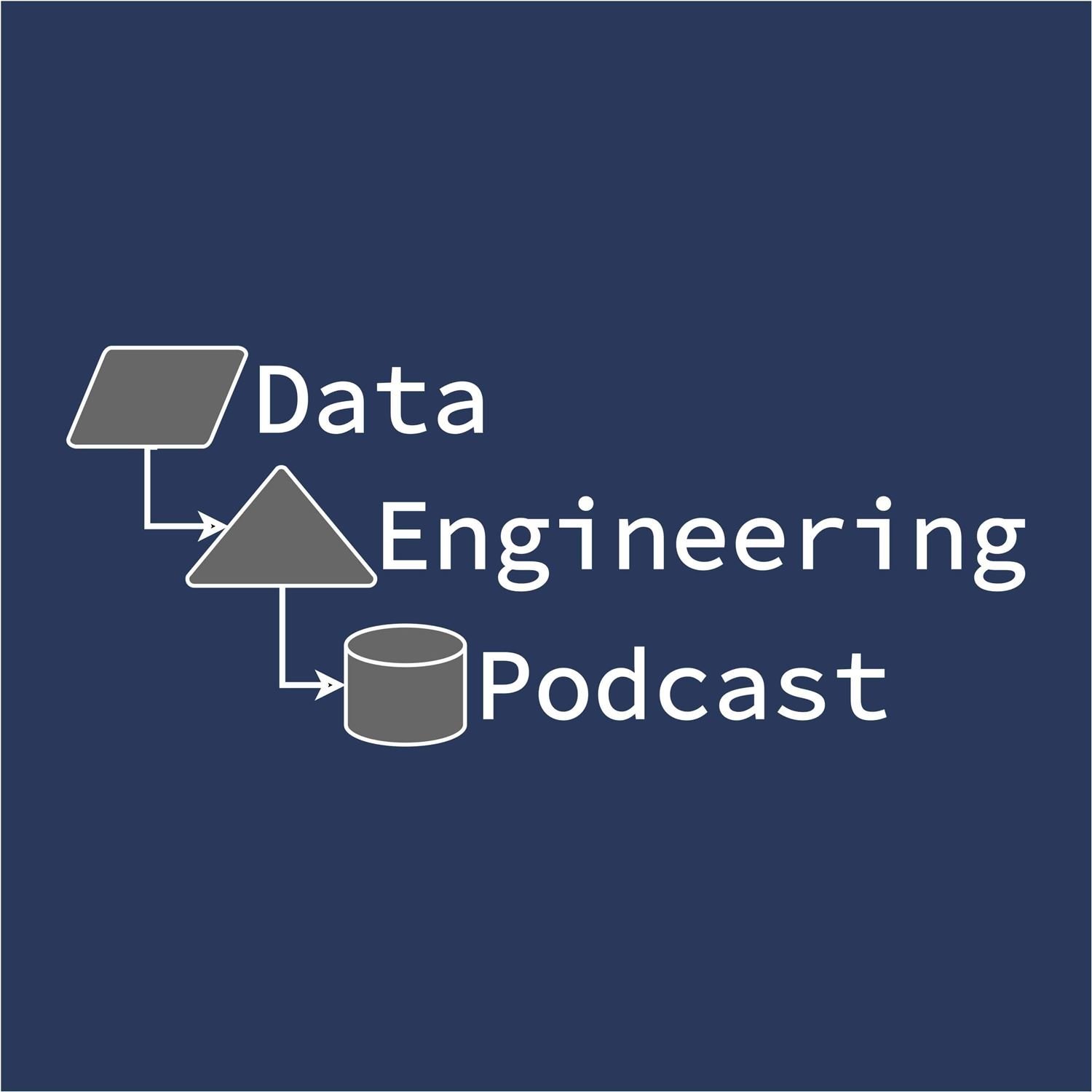
Defining A Strategy For Your Data Products
Summary
The primary application of data has moved beyond analytics. With the broader audience comes the need to present data in a more approachable format. This has led to the broad adoption of data products being the delivery mechanism for information. In this episode Ranjith Raghunath shares his thoughts on how to build a strategy for the development, delivery, and evolution of data products.
Announcements
Hello and welcome to the Data Engineering Podcast, the show about modern data management
Introducing RudderStack Profiles. RudderStack Profiles takes the SaaS guesswork and SQL grunt work out of building complete customer profiles so you can quickly ship actionable, enriched data to every downstream team. You specify the customer traits, then Profiles runs the joins and computations for you to create complete customer profiles. Get all of the details and try the new product today at dataengineeringpodcast.com/rudderstack
You shouldn't have to throw away the database to build with fast-changing data. You should be able to keep the familiarity of SQL and the proven architecture of cloud warehouses, but swap the decades-old batch computation model for an efficient incremental engine to get complex queries that are always up-to-date. With Materialize, you can! It’s the only true SQL streaming database built from the ground up to meet the needs of modern data products. Whether it’s real-time dashboarding and analytics, personalization and segmentation or automation and alerting, Materialize gives you the ability to work with fresh, correct, and scalable results — all in a familiar SQL interface. Go to dataengineeringpodcast.com/materialize today to get 2 weeks free!
As more people start using AI for projects, two things are clear: It’s a rapidly advancing field, but it’s tough to navigate. How can you get the best results for your use case? Instead of being subjected to a bunch of buzzword bingo, hear directly from pioneers in the developer and data science space on how they use graph tech to build AI-powered apps. . Attend the dev and ML talks at NODES 2023, a free online conference on October 26 featuring some of the brightest minds in tech. Check out the agenda and register today at Neo4j.com/NODES.
This episode is brought to you by Datafold – a testing automation platform for data engineers that finds data quality issues before the code and data are deployed to production. Datafold leverages data-diffing to compare production and development environments and column-level lineage to show you the exact impact of every code change on data, metrics, and BI tools, keeping your team productive and stakeholders happy. Datafold integrates with dbt, the modern data stack, and seamlessly plugs in your data CI for team-wide and automated testing. If you are migrating to a modern data stack, Datafold can also help you automate data and code validation to speed up the migration. Learn more about Datafold by visiting dataengineeringpodcast.com/datafold
Your host is Tobias Macey and today I'm interviewing Ranjith Raghunath about tactical elements of a data product strategy
Interview
Introduction
How did you get involved in the area of data management?
Can you describe what is encompassed by the idea of a data product strategy?
Which roles in an organization need to be involved in the planning and implementation of that strategy?
order of operations:
strategy -> platform design -> implementation/adoption
platform implementation -> product strategy -> interface development
managing grain of data in products
team organization to support product development/deployment
customer communications - what questions to ask? requirements gathering, helping to understand "the art of the possible"
What are the most interesting, innovative, or unexpected ways that you have seen organizations approach data product strategies?
What are the most interesting, unexpected, or challenging lessons that you have learned while working on defining and implementing data product strategies?
When is a data product strategy overkill?
What are some additional resources that you recommend for listeners to direct their thinking and learning about data product strategy?
Contact Info
LinkedIn
Parting Question
From your perspective, what is the biggest gap in the tooling or technology for data management today?
Closing Announcements
Thank you for listening! Don't forget to check out our other shows. Podcast.__init__ covers the Python language, its community, and the innovative ways it is being used. The Machine Learning Podcast helps you go from idea to production with machine learning.
Visit the site to subscribe to the show, sign up for the mailing list, and read the show notes.
If you've learned something or tried out a project from the show then tell us about it! Email [email protected]) with your story.
To help other people find the show please leave a review on Apple Podcasts and tell your friends and co-workers
Links
CXData Labs
Dimensional Modeling
The intro and outro music is from The Hug by The Freak Fandango Orchestra / CC BY-SASponsored By:Neo4J: 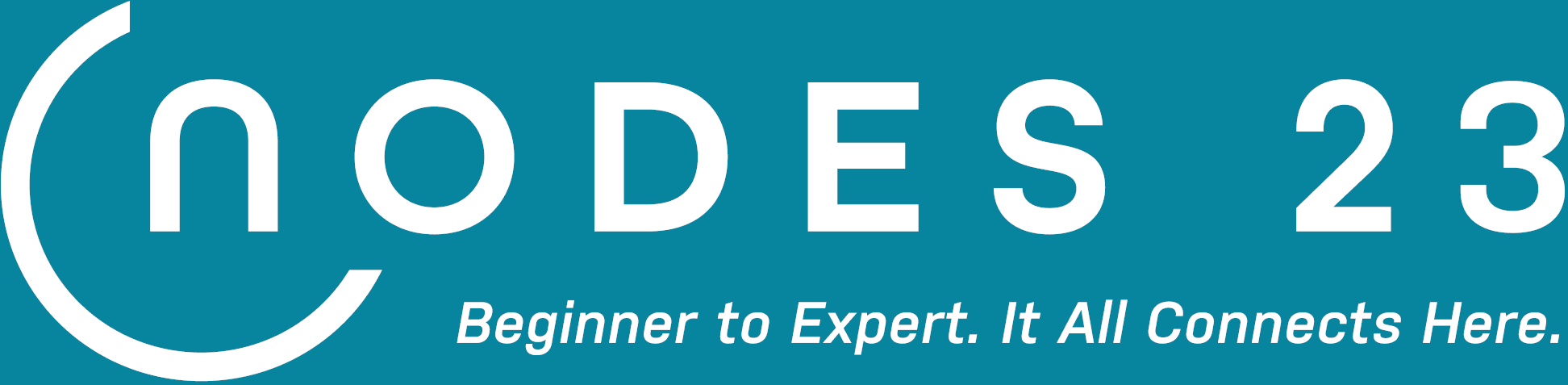
NODES 2023 is a free online conference focused on graph-driven innovations with content for all skill levels. Its 24 hours are packed with 90 interactive technical sessions from top developers and data scientists across the world covering a broad range of topics and use cases. The event tracks:
- Intelligent Applications: APIs, Libraries, and Frameworks – Tools and best practices for creating graph-powered applications and APIs with any software stack and programming language, including Java, Python, and JavaScript
- Machine Learning and AI – How graph technology provides context for your data and enhances the accuracy of your AI and ML projects (e.g.: graph neural networks, responsible AI)
- Visualization: Tools, Techniques, and Best Practices – Techniques and tools for exploring hidden and unknown patterns in your data and presenting complex relationships (knowledge graphs, ethical data practices, and data representation)
Don’t miss your chance to hear about the latest graph-powered implementations and best practices for free on October 26 at NODES 2023. Go to [Neo4j.com/NODES](https://Neo4j.com/NODES) today to see the full agenda and register!Rudderstack: 
Introducing RudderStack Profiles. RudderStack Profiles takes the SaaS guesswork and SQL grunt work out of building complete customer profiles so you can quickly ship actionable, enriched data to every downstream team. You specify the customer traits, then Profiles runs the joins and computations for you to create complete customer profiles. Get all of the details and try the new product today at [dataengineeringpodcast.com/rudderstack](https://www.dataengineeringpodcast.com/rudderstack)Materialize: 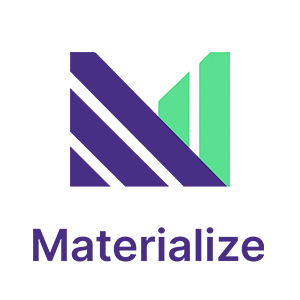
You shouldn't have to throw away the database to build with fast-changing data. Keep the familiar SQL, keep the proven architecture of cloud warehouses, but swap the decades-old batch computation model for an efficient incremental engine to get complex queries that are always up-to-date.
That is Materialize, the only true SQL streaming database built from the ground up to meet the needs of modern data products: Fresh, Correct, Scalable — all in a familiar SQL UI. Built on Timely Dataflow and Differential Dataflow, open source frameworks created by cofounder Frank McSherry at Microsoft Research, Materialize is trusted by data and engineering teams at Ramp, Pluralsight, Onward and more to build real-time data products without the cost, complexity, and development time of stream processing.
Go to [materialize.com](https://materialize.com/register/?utm_source=depodcast&utm_medium=paid&utm_campaign=early-access) today and get 2 weeks free!Datafold: 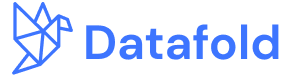
This episode is brought to you by Datafold – a testing automation platform for data engineers that finds data quality issues before the code and data are deployed to production. Datafold leverages data-diffing to compare production and development environments and column-level lineage to show you the exact impact of every code change on data, metrics, and BI tools, keeping your team productive and stakeholders happy. Datafold integrates with dbt, the modern data stack, and seamlessly plugs in your data CI for team-wide and automated testing. If you are migrating to a modern data stack, Datafold can also help you automate data and code validation to speed up the migration. Learn more about Datafold by visiting [dataengineeringpodcast.com/datafold](https://www.dataengineeringpodcast.com/datafold) today!Support Data Engineering Podcast
01:03:5023/10/2023