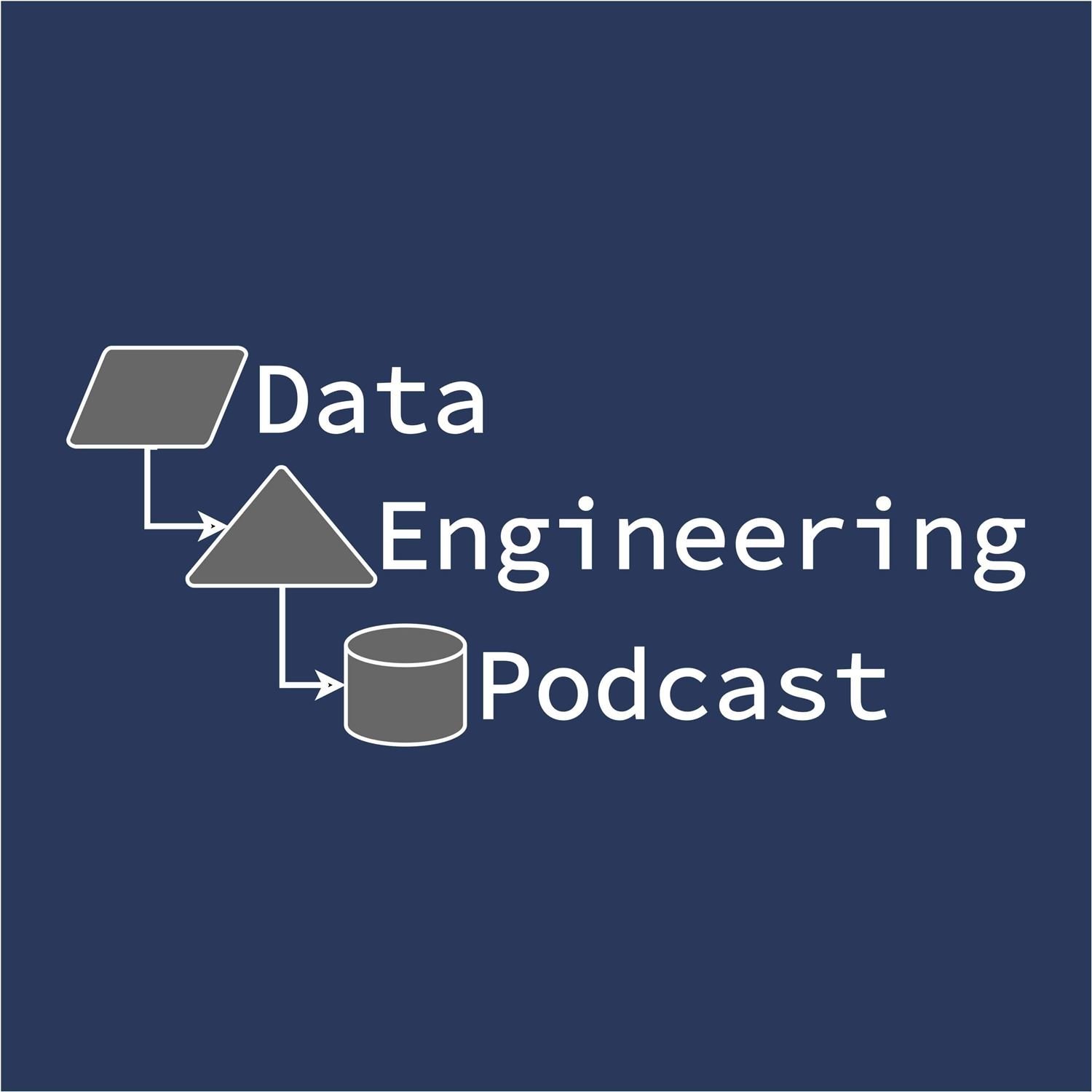
Education
Technology
Tobias Macey
This show goes behind the scenes for the tools, techniques, and difficulties associated with the discipline of data engineering. Databases, workflows, automation, and data manipulation are just some of the topics that you will find here.
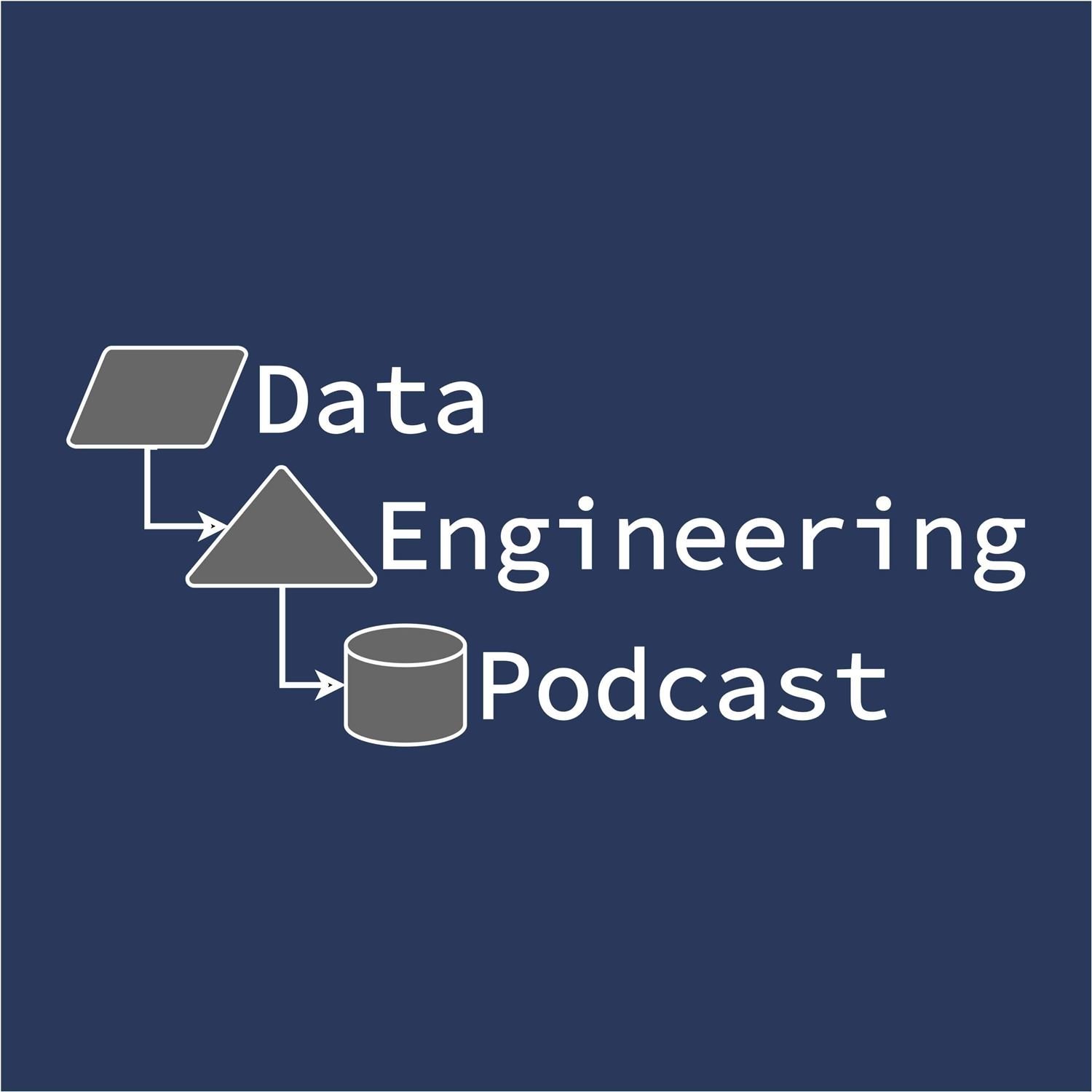
Bring Order To The Chaos Of Your Unstructured Data Assets With Unstruk
Summary
Working with unstructured data has typically been a motivation for a data lake. The challenge is imposing enough order on the platform to make it useful. Kirk Marple has spent years working with data systems and the media industry, which inspired him to build a platform for automatically organizing your unstructured assets to make them more valuable. In this episode he shares the goals of the Unstruk Data Warehouse, how it is architected to extract asset metadata and build a searchable knowledge graph from the information, and the myriad ways that the system can be used. If you are wondering how to deal with all of the information that doesn’t fit in your databases or data warehouses, then this episode is for you.
Announcements
Hello and welcome to the Data Engineering Podcast, the show about modern data management
When you’re ready to build your next pipeline, or want to test out the projects you hear about on the show, you’ll need somewhere to deploy it, so check out our friends at Linode. With their managed Kubernetes platform it’s now even easier to deploy and scale your workflows, or try out the latest Helm charts from tools like Pulsar and Pachyderm. With simple pricing, fast networking, object storage, and worldwide data centers, you’ve got everything you need to run a bulletproof data platform. Go to dataengineeringpodcast.com/linode today and get a $100 credit to try out a Kubernetes cluster of your own. And don’t forget to thank them for their continued support of this show!
Are you bored with writing scripts to move data into SaaS tools like Salesforce, Marketo, or Facebook Ads? Hightouch is the easiest way to sync data into the platforms that your business teams rely on. The data you’re looking for is already in your data warehouse and BI tools. Connect your warehouse to Hightouch, paste a SQL query, and use their visual mapper to specify how data should appear in your SaaS systems. No more scripts, just SQL. Supercharge your business teams with customer data using Hightouch for Reverse ETL today. Get started for free at dataengineeringpodcast.com/hightouch.
Atlan is a collaborative workspace for data-driven teams, like Github for engineering or Figma for design teams. By acting as a virtual hub for data assets ranging from tables and dashboards to SQL snippets & code, Atlan enables teams to create a single source of truth for all their data assets, and collaborate across the modern data stack through deep integrations with tools like Snowflake, Slack, Looker and more. Go to dataengineeringpodcast.com/atlan today and sign up for a free trial. If you’re a data engineering podcast listener, you get credits worth $3000 on an annual subscription
Your host is Tobias Macey and today I’m interviewing Kirk Marple about Unstruk Data, a company that is building a data warehouse for unstructured data that ofers automated data preparation via metadata enrichment, integrated compute, and graph-based search
Interview
Introduction
How did you get involved in the area of data management?
Can you describe what Unstruk Data is and the story behind it?
What would you classify as "unstructured data"?
What are some examples of industries that rely on large or varied sets of unstructured data?
What are the challenges for analytics that are posed by the different categories of unstructured data?
What is the current state of the industry for working with unstructured data?
What are the unique capabilities that Unstruk provides and how does it integrate with the rest of the ecosystem?
Where does it sit in the overall landscape of data tools?
Can you describe how the Unstruk data warehouse is implemented?
What are the assumptions that you had at the start of this project that have been challenged as you started working through the technical implementation and customer trials?
How has the design and architecture evolved or changed since you began working on it?
How do you handle versioning of data, given the potential for individual files to be quite large?
What are some of the considerations that users should have in mind when modeling their data in the warehouse?
Can you talk through the workflow of ingesting and analyzing data with Unstruk?
How do you manage data enrichment/integration with structured data sources?
What are the most interesting, innovative, or unexpected ways that you have seen the technology of Unstruk used?
What are the most interesting, unexpected, or challenging lessons that you have learned while working on and with the Unstruk platform?
When is Unstruk the wrong choice?
What do you have planned for the future of Unstruk?
Contact Info
LinkedIn
@KirkMarple on Twitter
Parting Question
From your perspective, what is the biggest gap in the tooling or technology for data management today?
Links
Unstruk Data
TIFF
ROSBag
HDF5
Media/Digital Asset Management
Data Mesh
SAN
NAS
Knowledge Graph
Entity Extraction
OCR (Optical Character Recognition)
Cloud Native
Cosmos DB
Azure Functions
Azure EventHub
Azure Cognitive Search
GraphQL
KNative
Schema.org
Pinecone Vector Database
Podcast Episode
Dublin Core Metadata Initiative
Knowledge Management
The intro and outro music is from The Hug by The Freak Fandango Orchestra / CC BY-SA
Support Data Engineering Podcast
40:4818/06/2021
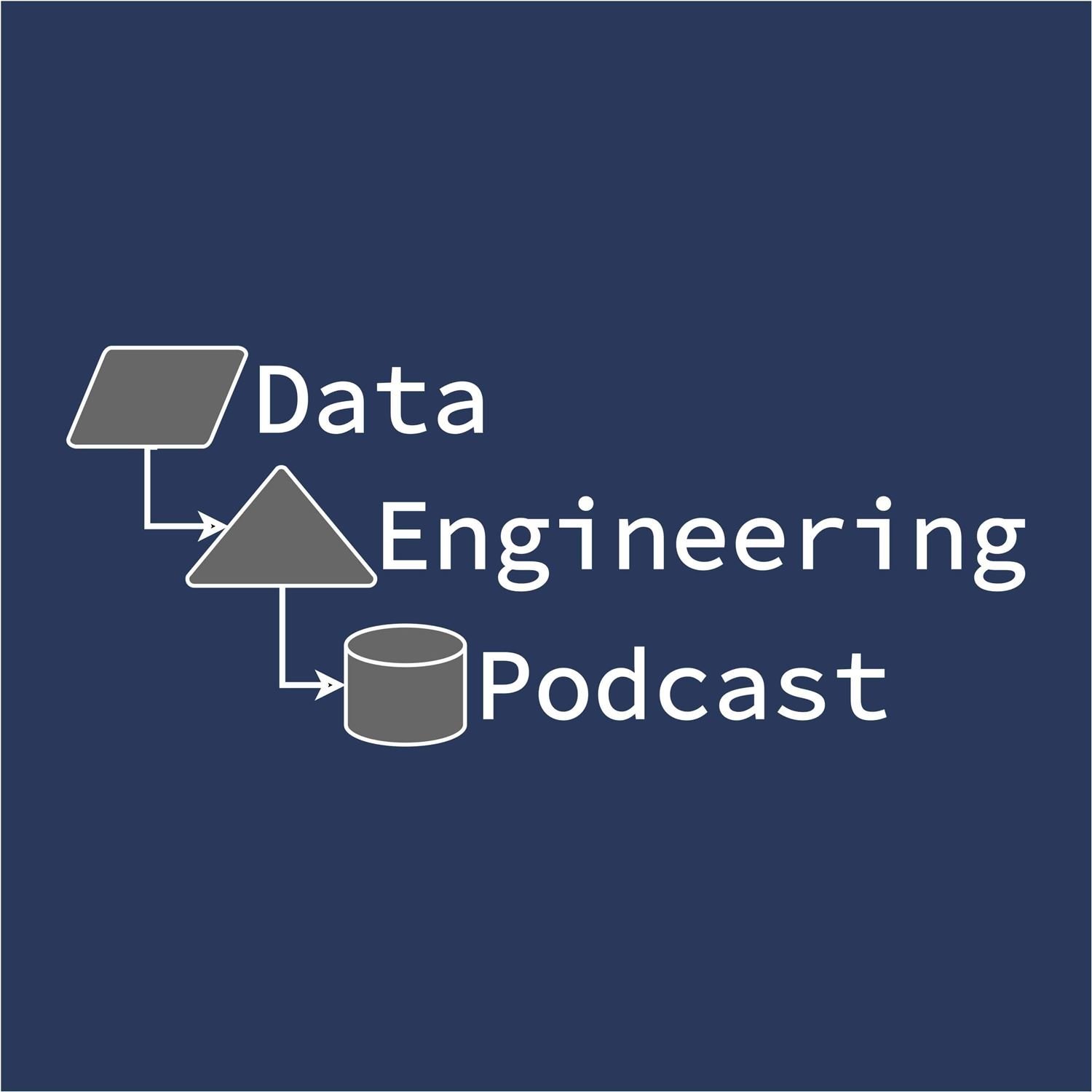
Accelerating ML Training And Delivery With In-Database Machine Learning
Summary
When you build a machine learning model, the first step is always to load your data. Typically this means downloading files from object storage, or querying a database. To speed up the process, why not build the model inside the database so that you don’t have to move the information? In this episode Paige Roberts explains the benefits of pushing the machine learning processing into the database layer and the approach that Vertica has taken for their implementation. If you are looking for a way to speed up your experimentation, or an easy way to apply AutoML then this conversation is for you.
Announcements
Hello and welcome to the Data Engineering Podcast, the show about modern data management
When you’re ready to build your next pipeline, or want to test out the projects you hear about on the show, you’ll need somewhere to deploy it, so check out our friends at Linode. With their managed Kubernetes platform it’s now even easier to deploy and scale your workflows, or try out the latest Helm charts from tools like Pulsar and Pachyderm. With simple pricing, fast networking, object storage, and worldwide data centers, you’ve got everything you need to run a bulletproof data platform. Go to dataengineeringpodcast.com/linode today and get a $100 credit to try out a Kubernetes cluster of your own. And don’t forget to thank them for their continued support of this show!
RudderStack’s smart customer data pipeline is warehouse-first. It builds your customer data warehouse and your identity graph on your data warehouse, with support for Snowflake, Google BigQuery, Amazon Redshift, and more. Their SDKs and plugins make event streaming easy, and their integrations with cloud applications like Salesforce and ZenDesk help you go beyond event streaming. With RudderStack you can use all of your customer data to answer more difficult questions and then send those insights to your whole customer data stack. Sign up free at dataengineeringpodcast.com/rudder today.
We’ve all been asked to help with an ad-hoc request for data by the sales and marketing team. Then it becomes a critical report that they need updated every week or every day. Then what do you do? Send a CSV via email? Write some Python scripts to automate it? But what about incremental sync, API quotas, error handling, and all of the other details that eat up your time? Today, there is a better way. With Census, just write SQL or plug in your dbt models and start syncing your cloud warehouse to SaaS applications like Salesforce, Marketo, Hubspot, and many more. Go to dataengineeringpodcast.com/census today to get a free 14-day trial.
Your host is Tobias Macey and today I’m interviewing Paige Roberts about machine learning workflows inside the database
Interview
Introduction
How did you get involved in the area of data management?
Can you start by giving an overview of the current state of the market for databases that support in-process machine learning?
What are the motivating factors for running a machine learning workflow inside the database?
What styles of ML are feasible to do inside the database? (e.g. bayesian inference, deep learning, etc.)
What are the performance implications of running a model training pipeline within the database runtime? (both in terms of training performance boosts, and database performance impacts)
Can you describe the architecture of how the machine learning process is managed by the database engine?
How do you manage interacting with Python/R/Jupyter/etc. when working within the database?
What is the impact on data pipeline and MLOps architectures when using the database to manage the machine learning workflow?
What are the most interesting, innovative, or unexpected ways that you have seen in-database ML used?
What are the most interesting, unexpected, or challenging lessons that you have learned while working on machine learning inside the database?
When is in-database ML the wrong choice?
What are the recent trends/changes in machine learning for the database that you are excited for?
Contact Info
LinkedIn
Blog
@RobertsPaige on Twitter
@PaigeEwing on Twitter
Parting Question
From your perspective, what is the biggest gap in the tooling or technology for data management today?
Closing Announcements
Thank you for listening! Don’t forget to check out our other show, Podcast.__init__ to learn about the Python language, its community, and the innovative ways it is being used.
Visit the site to subscribe to the show, sign up for the mailing list, and read the show notes.
If you’ve learned something or tried out a project from the show then tell us about it! Email [email protected]) with your story.
To help other people find the show please leave a review on iTunes and tell your friends and co-workers
Join the community in the new Zulip chat workspace at dataengineeringpodcast.com/chat
Links
Vertica
SyncSort
Hortonworks
Infoworld – 8 databases supporting in-database machine learning
Power BI
Podcast Episode
Grafana
Tableau
K-Means Clustering
MPP == Massively Parallel Processing
AutoML
Random Forest
PMML == Predictive Model Markup Language
SVM == Support Vector Machine
Naive Bayes
XGBoost
Pytorch
Tensorflow
Neural Magic
Tensorflow Frozen Graph
Parquet
ORC
Avro
CNCF == Cloud Native Computing Foundation
Hotel California
VerticaPy
Pandas
Podcast.__init__ Episode
Jupyter Notebook
UDX
Unifying Analytics Presentation
Hadoop
Yarn
Holden Karau
Spark
Vertica Academy
The intro and outro music is from The Hug by The Freak Fandango Orchestra / CC BY-SA
Support Data Engineering Podcast
01:05:3315/06/2021
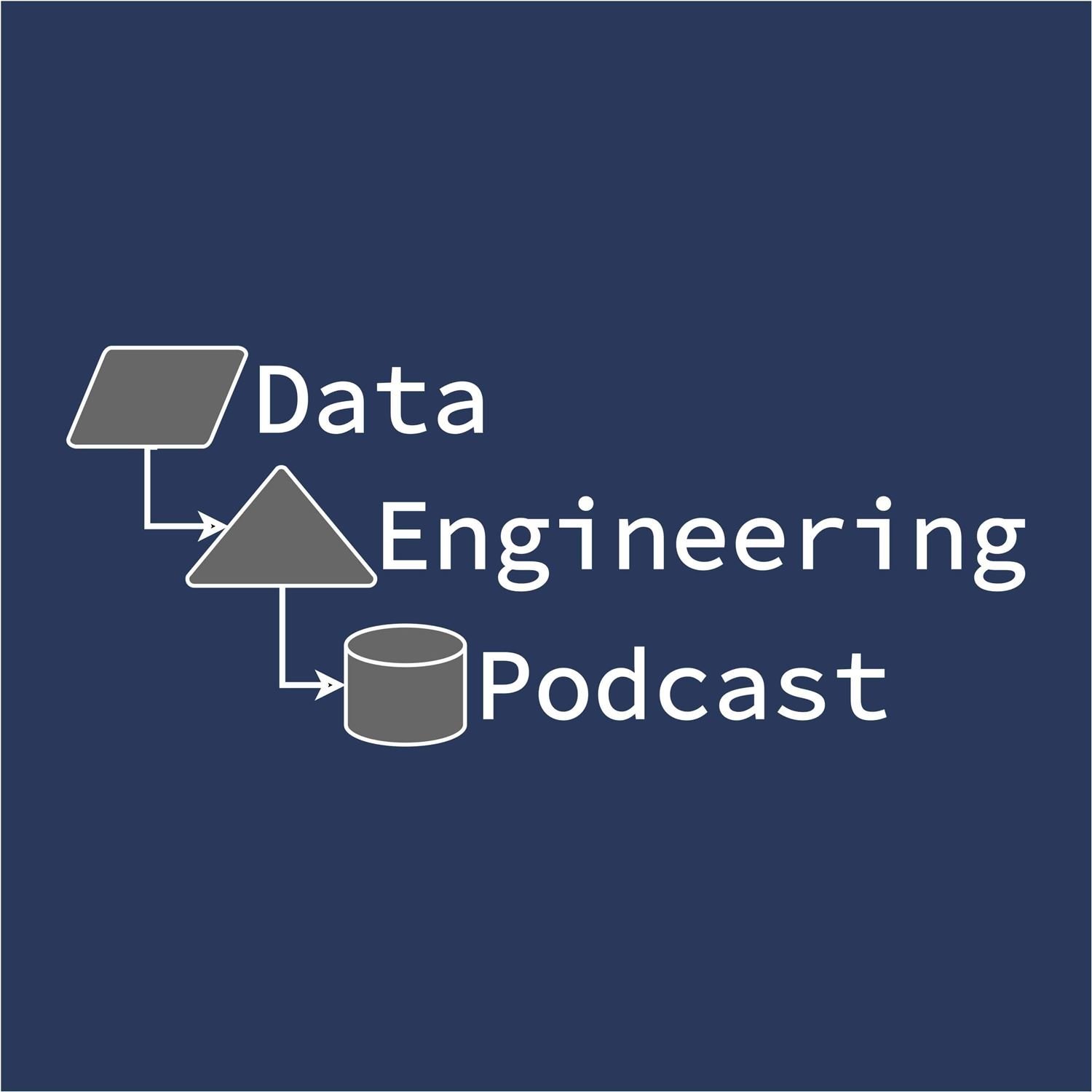
Taking A Tour Of The Google Cloud Platform For Data And Analytics
Summary
Google pioneered an impressive number of the architectural underpinnings of the broader big data ecosystem. Now they offer the technologies that they run internally to external users of their cloud platform. In this episode Lak Lakshmanan enumerates the variety of services that are available for building your various data processing and analytical systems. He shares some of the common patterns for building pipelines to power business intelligence dashboards, machine learning applications, and data warehouses. If you’ve ever been overwhelmed or confused by the array of services available in the Google Cloud Platform then this episode is for you.
Announcements
Hello and welcome to the Data Engineering Podcast, the show about modern data management
When you’re ready to build your next pipeline, or want to test out the projects you hear about on the show, you’ll need somewhere to deploy it, so check out our friends at Linode. With their managed Kubernetes platform it’s now even easier to deploy and scale your workflows, or try out the latest Helm charts from tools like Pulsar and Pachyderm. With simple pricing, fast networking, object storage, and worldwide data centers, you’ve got everything you need to run a bulletproof data platform. Go to dataengineeringpodcast.com/linode today and get a $100 credit to try out a Kubernetes cluster of your own. And don’t forget to thank them for their continued support of this show!
Are you bored with writing scripts to move data into SaaS tools like Salesforce, Marketo, or Facebook Ads? Hightouch is the easiest way to sync data into the platforms that your business teams rely on. The data you’re looking for is already in your data warehouse and BI tools. Connect your warehouse to Hightouch, paste a SQL query, and use their visual mapper to specify how data should appear in your SaaS systems. No more scripts, just SQL. Supercharge your business teams with customer data using Hightouch for Reverse ETL today. Get started for free at dataengineeringpodcast.com/hightouch.
Atlan is a collaborative workspace for data-driven teams, like Github for engineering or Figma for design teams. By acting as a virtual hub for data assets ranging from tables and dashboards to SQL snippets & code, Atlan enables teams to create a single source of truth for all their data assets, and collaborate across the modern data stack through deep integrations with tools like Snowflake, Slack, Looker and more. Go to dataengineeringpodcast.com/atlan today and sign up for a free trial. If you’re a data engineering podcast listener, you get credits worth $3000 on an annual subscription
Your host is Tobias Macey and today I’m interviewing Lak Lakshmanan about the suite of services for data and analytics in Google Cloud Platform.
Interview
Introduction
How did you get involved in the area of data management?
Can you start by giving an overview of the tools and products that are offered as part of Google Cloud for data and analytics?
How do the various systems relate to each other for building a full workflow?
How do you balance the need for clean integration between services with the need to make them useful in isolation when used as a single component of a data platform?
What have you found to be the primary motivators for customers who are adopting GCP for some or all of their data workloads?
What are some of the challenges that new users of GCP encounter when working with the data and analytics products that it offers?
What are the systems that you have found to be easiest to work with?
Which are the most challenging to work with, whether due to the kinds of problems that they are solving for, or due to their user experience design?
How has your work with customers fed back into the products that you are building on top of?
What are some examples of architectural or software patterns that are unique to the GCP product suite?
What are the most interesting, innovative, or unexpected ways that you have seen Google Cloud’s data and analytics services used?
What are the most interesting, unexpected, or challenging lessons that you have learned while working at Google and helping customers succeed in their data and analytics efforts?
What are some of the new capabilities, new services, or industry trends that you are most excited for?
Contact Info
LinkedIn
@lak_gcp on Twitter
Website
Parting Question
From your perspective, what is the biggest gap in the tooling or technology for data management today?
Links
Google Cloud
Data and Analytics Services
Forrester Wave
Dremel
BigQuery
MapReduce
Cloud Spanner
Spanner Paper
Hadoop
Tensorflow
Google Cloud SQL
Apache Spark
Dataproc
Dataflow
Apache Beam
Databricks
Mixpanel
Avalanche data warehouse
Kubernetes
GKE (Google Kubernetes Engine)
Google Cloud Run
Android
Youtube
Google Translate
Teradata
Power BI
Podcast Episode
AI Platform Notebooks
GitHub Data Repository
Stack Overflow Questions Data Repository
PyPI Download Statistics
Recommendations AI
Pub/Sub
Bigtable
Datastream
Change Data Capture
Podcast Episode About Debezium for CDC
Podcast Episode About CDC with Datacoral
Document AI
Google Meet
Data Governance
Podcast Episodes
The intro and outro music is from The Hug by The Freak Fandango Orchestra / CC BY-SA
Support Data Engineering Podcast
53:1712/06/2021
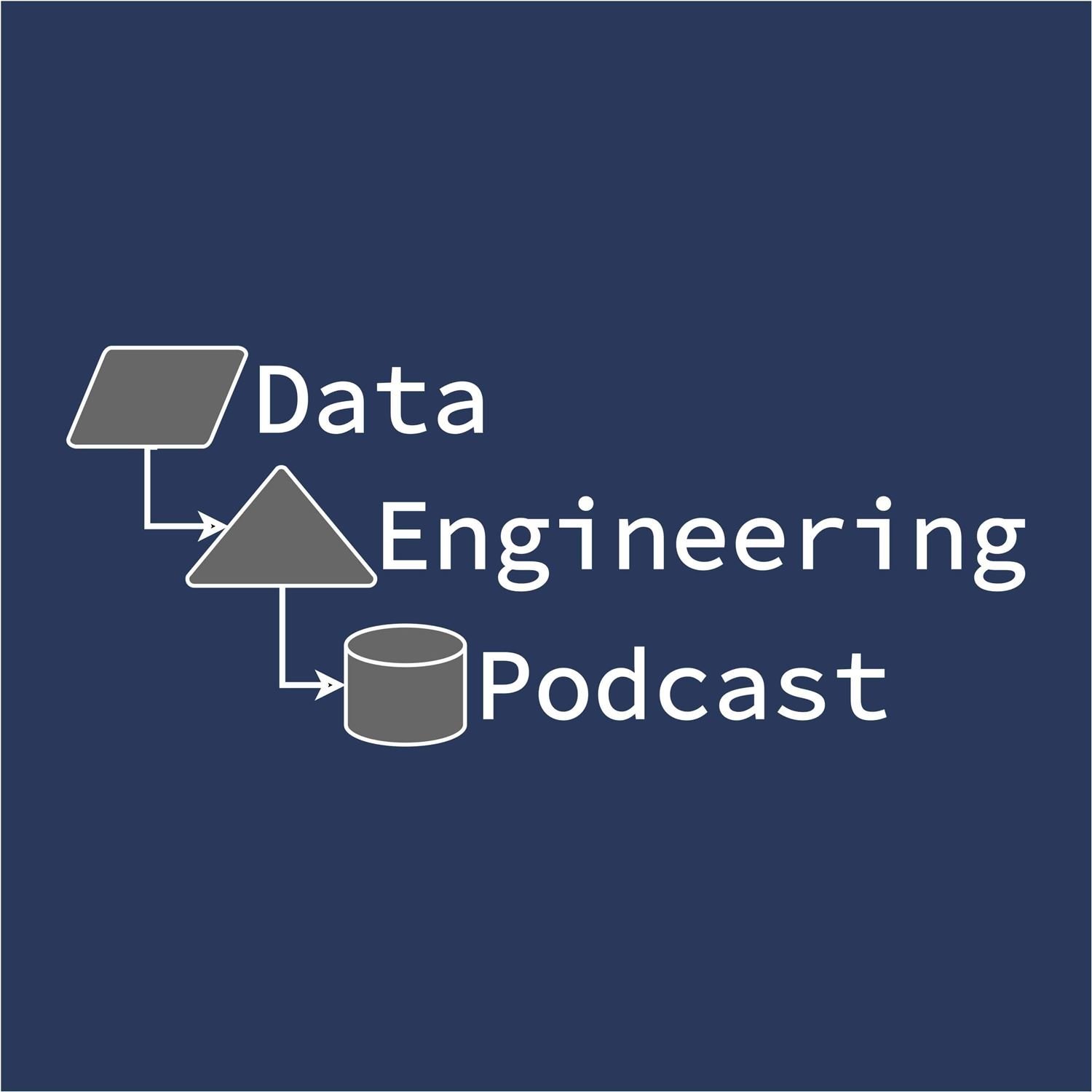
Make Sure Your Records Are Reliable With The BookKeeper Distributed Storage Layer
Summary
The way to build maintainable software and systems is through composition of individual pieces. By making those pieces high quality and flexible they can be used in surprising ways that the original creators couldn’t have imagined. One such component that has gone above and beyond its originally envisioned use case is BookKeeper, a distributed storage system that is optimized for durability and speed. In this episode Matteo Merli shares the story behind the creation of BookKeeper, the various ways that it is being used today, and the architectural aspects that make it such a strong building block for projects such as Pulsar. He also shares some of the other interesting systems that have been built on top of it and an amusing war story of running it at scale in its early years.
Announcements
Hello and welcome to the Data Engineering Podcast, the show about modern data management
When you’re ready to build your next pipeline, or want to test out the projects you hear about on the show, you’ll need somewhere to deploy it, so check out our friends at Linode. With their managed Kubernetes platform it’s now even easier to deploy and scale your workflows, or try out the latest Helm charts from tools like Pulsar and Pachyderm. With simple pricing, fast networking, object storage, and worldwide data centers, you’ve got everything you need to run a bulletproof data platform. Go to dataengineeringpodcast.com/linode today and get a $100 credit to try out a Kubernetes cluster of your own. And don’t forget to thank them for their continued support of this show!
RudderStack’s smart customer data pipeline is warehouse-first. It builds your customer data warehouse and your identity graph on your data warehouse, with support for Snowflake, Google BigQuery, Amazon Redshift, and more. Their SDKs and plugins make event streaming easy, and their integrations with cloud applications like Salesforce and ZenDesk help you go beyond event streaming. With RudderStack you can use all of your customer data to answer more difficult questions and then send those insights to your whole customer data stack. Sign up free at dataengineeringpodcast.com/rudder today.
We’ve all been asked to help with an ad-hoc request for data by the sales and marketing team. Then it becomes a critical report that they need updated every week or every day. Then what do you do? Send a CSV via email? Write some Python scripts to automate it? But what about incremental sync, API quotas, error handling, and all of the other details that eat up your time? Today, there is a better way. With Census, just write SQL or plug in your dbt models and start syncing your cloud warehouse to SaaS applications like Salesforce, Marketo, Hubspot, and many more. Go to dataengineeringpodcast.com/census today to get a free 14-day trial.
Your host is Tobias Macey and today I’m interviewing Matteo Merli about Apache BookKeeper, a scalable, fault-tolerant, and low-latency storage service optimized for real-time workloads
Interview
Introduction
How did you get involved in the area of data management?
Can you describe what BookKeeper is and the story behind it?
What are the most notable features/capabilities of BookKeeper?
What are some of the ways that BookKeeper is being used?
How has your work on Pulsar influenced the features and product direction of BookKeeper?
Can you describe the architecture of a BookKeeper cluster?
How have the design and goals of BookKeeper changed or evolved over time?
What is the impact of record-oriented storage on data distribution/allocation within the cluster when working with variable record sizes?
What are some of the operational considerations that users should be aware of?
What are some of the most interesting/compelling features from your perspective?
What are some of the most often overlooked or misunderstood capabilities of BookKeeper?
What are the most interesting, innovative, or unexpected ways that you have seen BookKeeper used?
What are the most interesting, unexpected, or challenging lessons that you have learned while working on BookKeeper?
When is BookKeeper the wrong choice?
What do you have planned for the future of BookKeeper?
Contact Info
LinkedIn
@merlimat on Twitter
merlimat on GitHub
Parting Question
From your perspective, what is the biggest gap in the tooling or technology for data management today?
Closing Announcements
Thank you for listening! Don’t forget to check out our other show, Podcast.__init__ to learn about the Python language, its community, and the innovative ways it is being used.
Visit the site to subscribe to the show, sign up for the mailing list, and read the show notes.
If you’ve learned something or tried out a project from the show then tell us about it! Email [email protected]) with your story.
To help other people find the show please leave a review on iTunes and tell your friends and co-workers
Join the community in the new Zulip chat workspace at dataengineeringpodcast.com/chat
Links
Apache BookKeeper
Apache Pulsar
Podcast Episode
StreamNative
Podcast Episode
Hadoop NameNode
Apache Zookeeper
Podcast Episode
ActiveMQ
Write Ahead Log (WAL)
BookKeeper Architecture
RocksDB
LSM == Log-Structured Merge-Tree
RAID Controller
Pravega
Podcast Episode
BookKeeper etcd Metadata Storage
LevelDB
Ceph
Podcast Episode
Direct IO
Page Cache
The intro and outro music is from The Hug by The Freak Fandango Orchestra / CC BY-SA
Support Data Engineering Podcast
42:0109/06/2021
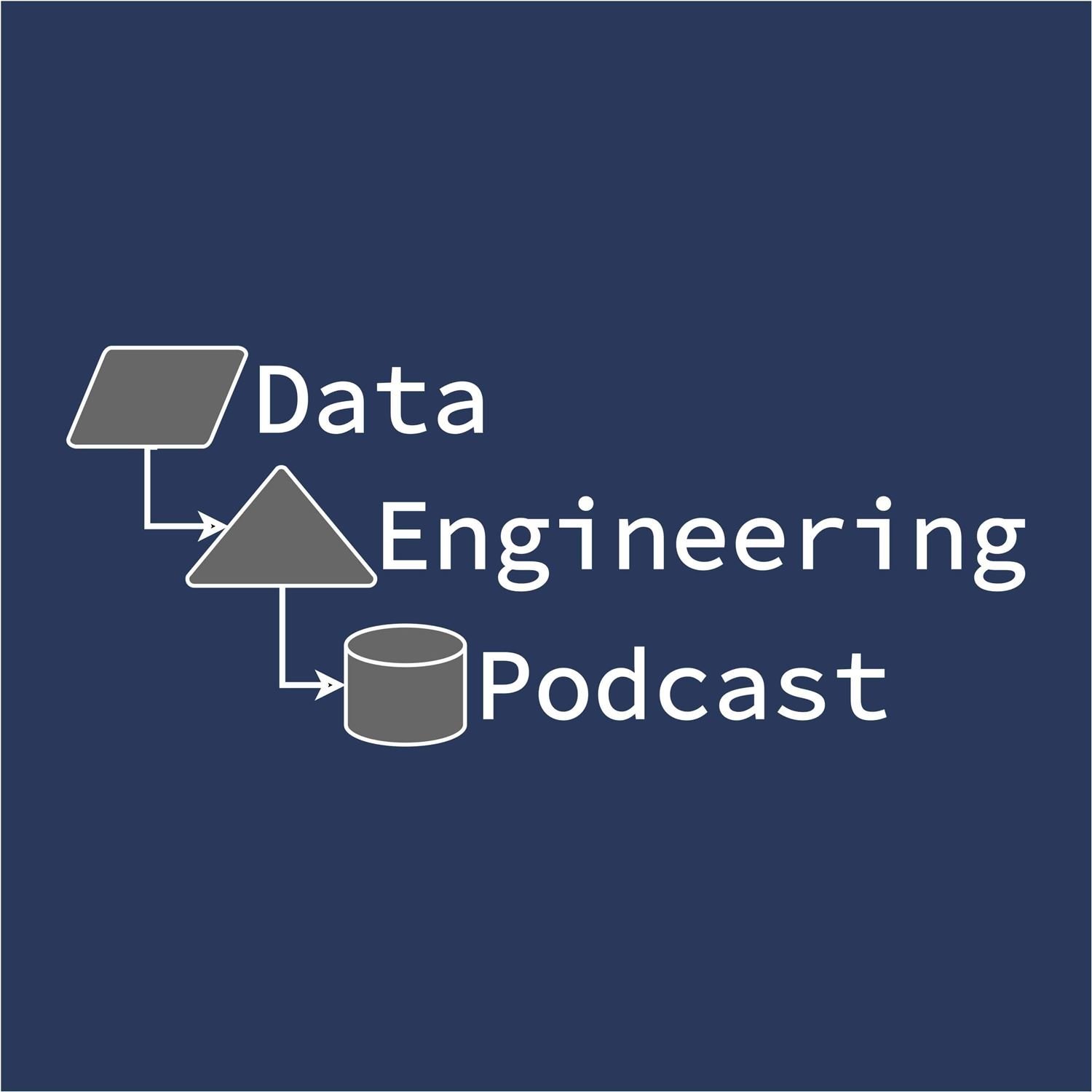
Build Your Analytics With A Collaborative And Expressive SQL IDE Using Querybook
Summary
SQL is the most widely used language for working with data, and yet the tools available for writing and collaborating on it are still clunky and inefficient. Frustrated with the lack of a modern IDE and collaborative workflow for managing the SQL queries and analysis of their big data environments, the team at Pinterest created Querybook. In this episode Justin Mejorada-Pier and Charlie Gu share the story of how the initial prototype for a data catalog ended up as one of their most widely used interfaces to their analytical data. They also discuss the unique combination of features that it offers, how it is implemented, and the path to releasing it as open source. Querybook is an impressive and unique piece of technology that is well worth exploring, so listen and try it out today.
Announcements
Hello and welcome to the Data Engineering Podcast, the show about modern data management
When you’re ready to build your next pipeline, or want to test out the projects you hear about on the show, you’ll need somewhere to deploy it, so check out our friends at Linode. With their managed Kubernetes platform it’s now even easier to deploy and scale your workflows, or try out the latest Helm charts from tools like Pulsar and Pachyderm. With simple pricing, fast networking, object storage, and worldwide data centers, you’ve got everything you need to run a bulletproof data platform. Go to dataengineeringpodcast.com/linode today and get a $100 credit to try out a Kubernetes cluster of your own. And don’t forget to thank them for their continued support of this show!
Firebolt is the fastest cloud data warehouse. Visit dataengineeringpodcast.com/firebolt to get started. The first 25 visitors will receive a Firebolt t-shirt.
Atlan is a collaborative workspace for data-driven teams, like Github for engineering or Figma for design teams. By acting as a virtual hub for data assets ranging from tables and dashboards to SQL snippets & code, Atlan enables teams to create a single source of truth for all their data assets, and collaborate across the modern data stack through deep integrations with tools like Snowflake, Slack, Looker and more. Go to dataengineeringpodcast.com/atlan today and sign up for a free trial. If you’re a data engineering podcast listener, you get credits worth $3000 on an annual subscription
Your host is Tobias Macey and today I’m interviewing Justin Mejorada-Pier and Charlie Gu about Querybook, an open source IDE for your big data projects
Interview
Introduction
How did you get involved in the area of data management?
Can you describe what Querybook is and the story behind it?
What are the main use cases or workflows that Querybook is designed for?
What are the shortcomings of dashboarding/BI tools that make something like Querybook necessary?
The tag line calls out the fact that Querybook is an IDE for "big data". What are the manifestations of that focus in the feature set and user experience?
Who are the target users of Querybook and how does that inform the feature priorities and user experience?
Can you describe how Querybook is architected?
How have the goals and design changed or evolved since you first began working on it?
What were some of the assumptions or design choices that you had to unwind in the process of open sourcing it?
What is the workflow for someone building a DataDoc with Querybook?
What is the experience of working as a collaborator on an analysis?
How do you handle lifecycle management of query results?
What are your thoughts on the potential for extending Querybook beyond SQL-oriented analysis and integrating something like Jupyter kernels?
What are the most interesting, innovative, or unexpected ways that you have seen Querybook used?
What are the most interesting, unexpected, or challenging lessons that you have learned while working on Querybook?
When is Querybook the wrong choice?
What do you have planned for the future of Querybook?
Contact Info
Justin
LinkedIn
Website
Charlie
czgu on GitHub
Parting Question
From your perspective, what is the biggest gap in the tooling or technology for data management today?
Closing Announcements
Thank you for listening! Don’t forget to check out our other show, Podcast.__init__ to learn about the Python language, its community, and the innovative ways it is being used.
Visit the site to subscribe to the show, sign up for the mailing list, and read the show notes.
If you’ve learned something or tried out a project from the show then tell us about it! Email [email protected]) with your story.
To help other people find the show please leave a review on iTunes and tell your friends and co-workers
Join the community in the new Zulip chat workspace at dataengineeringpodcast.com/chat
Links
Querybook
Announcing Querybook as Open Source
Pinterest
University of Waterloo
Superset
Podcast Episode
Podcast.__init__ Episode
Sequel Pro
Presto
Trino
Podcast Episode
Flask
uWSGI
Podcast.__init__ Episode
Celery
Redis
SocketIO
Elasticsearch
Podcast Episode
Amundsen
Podcast Episode
Apache Atlas
DataHub
Podcast Episode
Okta
LDAP (Lightweight Directory Access Protocol)
Grand Rounds
The intro and outro music is from The Hug by The Freak Fandango Orchestra / CC BY-SA
Support Data Engineering Podcast
52:3603/06/2021
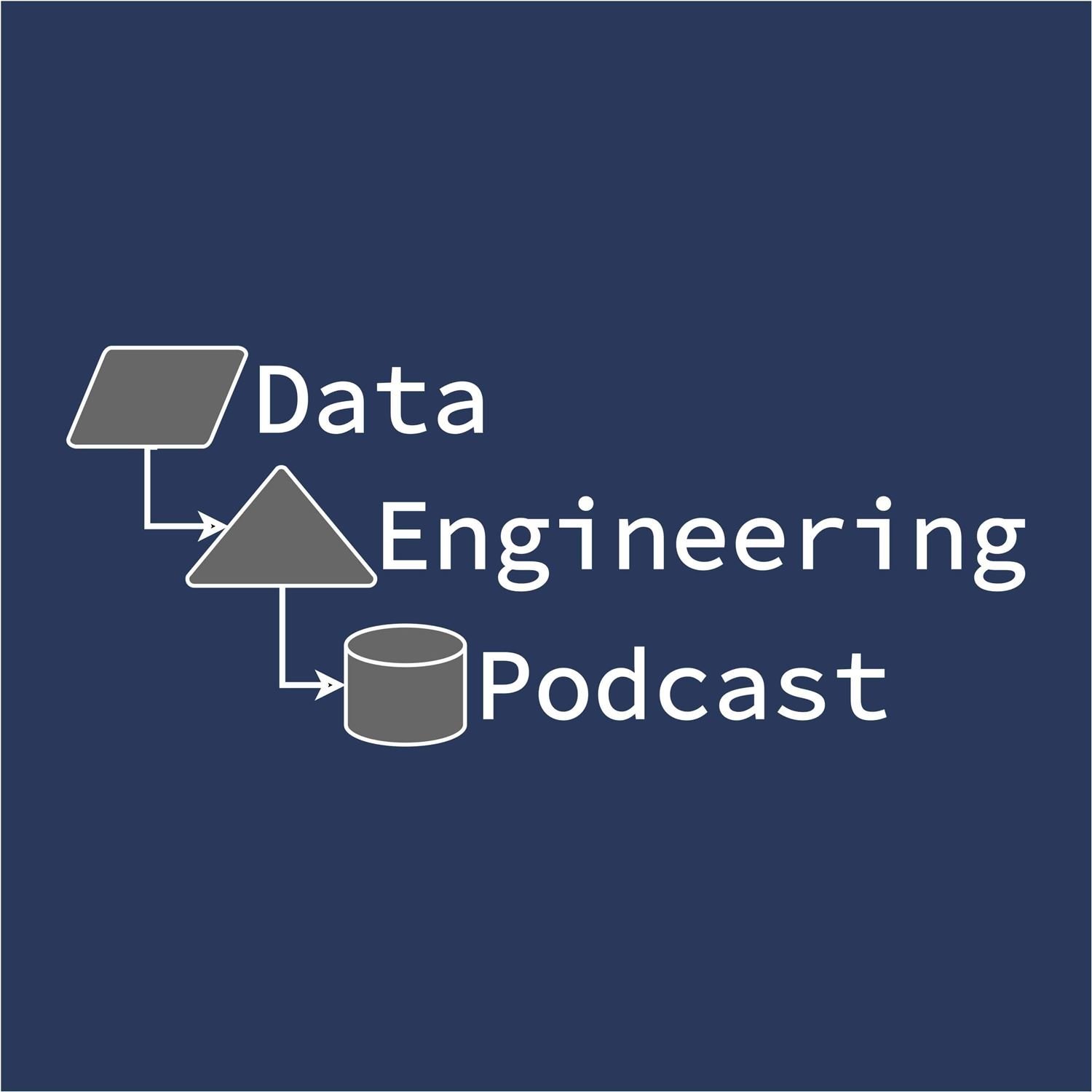
Making Data Pipelines Self-Serve For Everyone With Shipyard
Summary
Every part of the business relies on data, yet only a small team has the context and expertise to build and maintain workflows and data pipelines to transform, clean, and integrate it. In order for the true value of your data to be realized without burning out your engineers you need a way for everyone to get access to the information they care about. To help make that a more tractable problem Blake Burch co-founded Shipyard. In this episode he explains the utility of a low code solution that lets non engineers create their own self-serve pipelines, how the Shipyard platform is designed to make that possible, and how it allows engineers to create reusable tasks to satisfy the specific needs of the business. This is an interesting conversation about how to make data more accessible and more useful by improving the user experience of the tools that we create.
Announcements
Hello and welcome to the Data Engineering Podcast, the show about modern data management
When you’re ready to build your next pipeline, or want to test out the projects you hear about on the show, you’ll need somewhere to deploy it, so check out our friends at Linode. With their managed Kubernetes platform it’s now even easier to deploy and scale your workflows, or try out the latest Helm charts from tools like Pulsar and Pachyderm. With simple pricing, fast networking, object storage, and worldwide data centers, you’ve got everything you need to run a bulletproof data platform. Go to dataengineeringpodcast.com/linode today and get a $100 credit to try out a Kubernetes cluster of your own. And don’t forget to thank them for their continued support of this show!
RudderStack’s smart customer data pipeline is warehouse-first. It builds your customer data warehouse and your identity graph on your data warehouse, with support for Snowflake, Google BigQuery, Amazon Redshift, and more. Their SDKs and plugins make event streaming easy, and their integrations with cloud applications like Salesforce and ZenDesk help you go beyond event streaming. With RudderStack you can use all of your customer data to answer more difficult questions and then send those insights to your whole customer data stack. Sign up free at dataengineeringpodcast.com/rudder today.
When it comes to serving data for AI and ML projects, do you feel like you have to rebuild the plane while you’re flying it across the ocean? Molecula is an enterprise feature store that operationalizes advanced analytics and AI in a format designed for massive machine-scale projects without having to manage endless one-off information requests. With Molecula, data engineers manage one single feature store that serves the entire organization with millisecond query performance whether in the cloud or at your data center. And since it is implemented as an overlay, Molecula doesn’t disrupt legacy systems. High-growth startups use Molecula’s feature store because of its unprecedented speed, cost savings, and simplified access to all enterprise data. From feature extraction to model training to production, the Molecula feature store provides continuously updated feature access, reuse, and sharing without the need to pre-process data. If you need to deliver unprecedented speed, cost savings, and simplified access to large scale, real-time data, visit dataengineeringpodcast.com/molecula and request a demo. Mention that you’re a Data Engineering Podcast listener, and they’ll send you a free t-shirt.
Your host is Tobias Macey and today I’m interviewing Blake Burch about Shipyard, and his mission to create the easiest way for data teams to launch, monitor, and share resilient pipelines with less engineering
Interview
Introduction
How did you get involved in the area of data management?
Can you describe what you are building at Shipyard and the story behind it?
What are the main goals that you have for Shipyard?
How does it compare to other data orchestration frameworks in the market?
Who are the target users of Shipyard and how does that influence the features and design of the product?
What are your thoughts on the role of data orchestration in the business?
How is the Shipyard platform implemented?
What was your process for identifying the core requirements of the platform?
How have the design and goals of the system evolved since you first began working on it?
Can you describe the workflow of building a data workflow with Shipyard?
How do you manage the dependency chain across tasks in the execution graph? (e.g. task-based, data assets, etc.)
How do you handle testing and data quality management in your workflows?
What is the interface for creating custom task definitions?
How do you address dependencies and sandboxing for custom code?
What is your approach to developing templates?
What are the operational challenges that you have had to address to manage scaling and multi-tenancy in your platform?
What are the most interesting, innovative, or unexpected ways that you have seen Shipyard used?
What are the most interesting, unexpected, or challenging lessons that you have learned while working on Shipyard?
When is Shipyard the wrong choice?
What do you have planned for the future of Shipyard?
Contact Info
LinkedIn
@BlakeBurch_ on Twitter
Website
blakeburch on GitHub
Parting Question
From your perspective, what is the biggest gap in the tooling or technology for data management today?
Closing Announcements
Thank you for listening! Don’t forget to check out our other show, Podcast.__init__ to learn about the Python language, its community, and the innovative ways it is being used.
Visit the site to subscribe to the show, sign up for the mailing list, and read the show notes.
If you’ve learned something or tried out a project from the show then tell us about it! Email [email protected]) with your story.
To help other people find the show please leave a review on iTunes and tell your friends and co-workers
Join the community in the new Zulip chat workspace at dataengineeringpodcast.com/chat
Links
Shipyard
Zapier
Airtable
BigQuery
Snowflake
Podcast Episode
Docker
ECS == Elastic Container Service
Great Expectations
Podcast Episode
Monte Carlo
Podcast Episode
Soda Data
Podcast Episode
The intro and outro music is from The Hug by The Freak Fandango Orchestra / CC BY-SA
Support Data Engineering Podcast
51:2302/06/2021
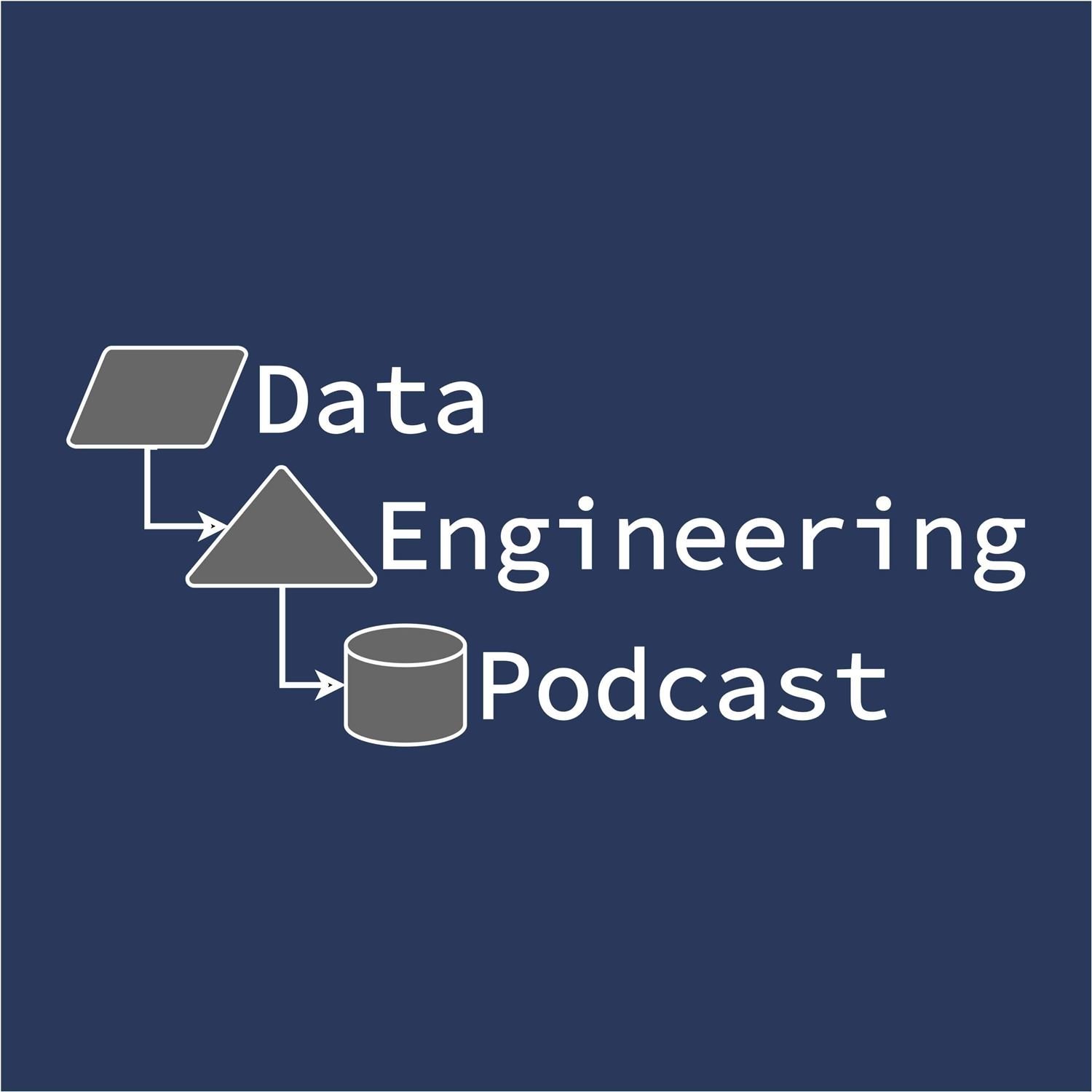
Paving The Road For Fast Analytics On Distributed Clouds With The Yellowbrick Data Warehouse
Summary
The data warehouse has become the focal point of the modern data platform. With increased usage of data across businesses, and a diversity of locations and environments where data needs to be managed, the warehouse engine needs to be fast and easy to manage. Yellowbrick is a data warehouse platform that was built from the ground up for speed, and can work across clouds and all the way to the edge. In this episode CTO Mark Cusack explains how the engine is architected, the benefits that speed and predictable pricing has for the organization, and how you can simplify your platform by putting the warehouse close to the data, instead of the other way around.
Announcements
Hello and welcome to the Data Engineering Podcast, the show about modern data management
When you’re ready to build your next pipeline, or want to test out the projects you hear about on the show, you’ll need somewhere to deploy it, so check out our friends at Linode. With their managed Kubernetes platform it’s now even easier to deploy and scale your workflows, or try out the latest Helm charts from tools like Pulsar and Pachyderm. With simple pricing, fast networking, object storage, and worldwide data centers, you’ve got everything you need to run a bulletproof data platform. Go to dataengineeringpodcast.com/linode today and get a $100 credit to try out a Kubernetes cluster of your own. And don’t forget to thank them for their continued support of this show!
Firebolt is the fastest cloud data warehouse. Visit dataengineeringpodcast.com/firebolt to get started. The first 25 visitors will receive a Firebolt t-shirt.
Atlan is a collaborative workspace for data-driven teams, like Github for engineering or Figma for design teams. By acting as a virtual hub for data assets ranging from tables and dashboards to SQL snippets & code, Atlan enables teams to create a single source of truth for all their data assets, and collaborate across the modern data stack through deep integrations with tools like Snowflake, Slack, Looker and more. Go to dataengineeringpodcast.com/atlan today and sign up for a free trial. If you’re a data engineering podcast listener, you get credits worth $3000 on an annual subscription
Your host is Tobias Macey and today I’m interviewing Mark Cusack about Yellowbrick, a data warehouse designed for distributed clouds
Interview
Introduction
How did you get involved in the area of data management?
Can you start by describing what Yellowbrick is and some of the story behind it?
What does the term "distributed cloud" signify and what challenges are associated with it?
How would you characterize Yellowbrick’s position in the database/DWH market?
How is Yellowbrick architected?
How have the goals and design of the platform changed or evolved over time?
How does Yellowbrick maintain visibility across the different data locations that it is responsible for?
What capabilities does it offer for being able to join across the disparate "clouds"?
What are some data modeling strategies that users should consider when designing their deployment of Yellowbrick?
What are some of the capabilities of Yellowbrick that you find most useful or technically interesting?
For someone who is adopting Yellowbrick, what is the process for getting it integrated into their data systems?
What are the most underutilized, overlooked, or misunderstood features of Yellowbrick?
What are the most interesting, innovative, or unexpected ways that you have seen Yellowbrick used?
What are the most interesting, unexpected, or challenging lessons that you have learned while working on and with Yellowbrick?
When is Yellowbrick the wrong choice?
What do you have planned for the future of the product?
Contact Info
LinkedIn
@markcusack on Twitter
Parting Question
From your perspective, what is the biggest gap in the tooling or technology for data management today?
Links
Yellowbrick
Teradata
Rainstor
Distributed Cloud
Hybrid Cloud
SwimOS
Podcast Episode
Kafka
Pulsar
Podcast Episode
Snowflake
Podcast Episode
AWS Redshift
MPP == Massively Parallel Processing
Presto
Trino
Podcast Episode
L3 Cache
NVMe
Reactive Programming
Coroutine
Star Schema
Denodo
Lexis Nexis
Vertica
Netezza
Grenplum
PostgreSQL
Podcast Episode
Clickhouse
Podcast Episode
Erasure Coding
The intro and outro music is from The Hug by The Freak Fandango Orchestra / CC BY-SA
Support Data Engineering Podcast
52:4128/05/2021
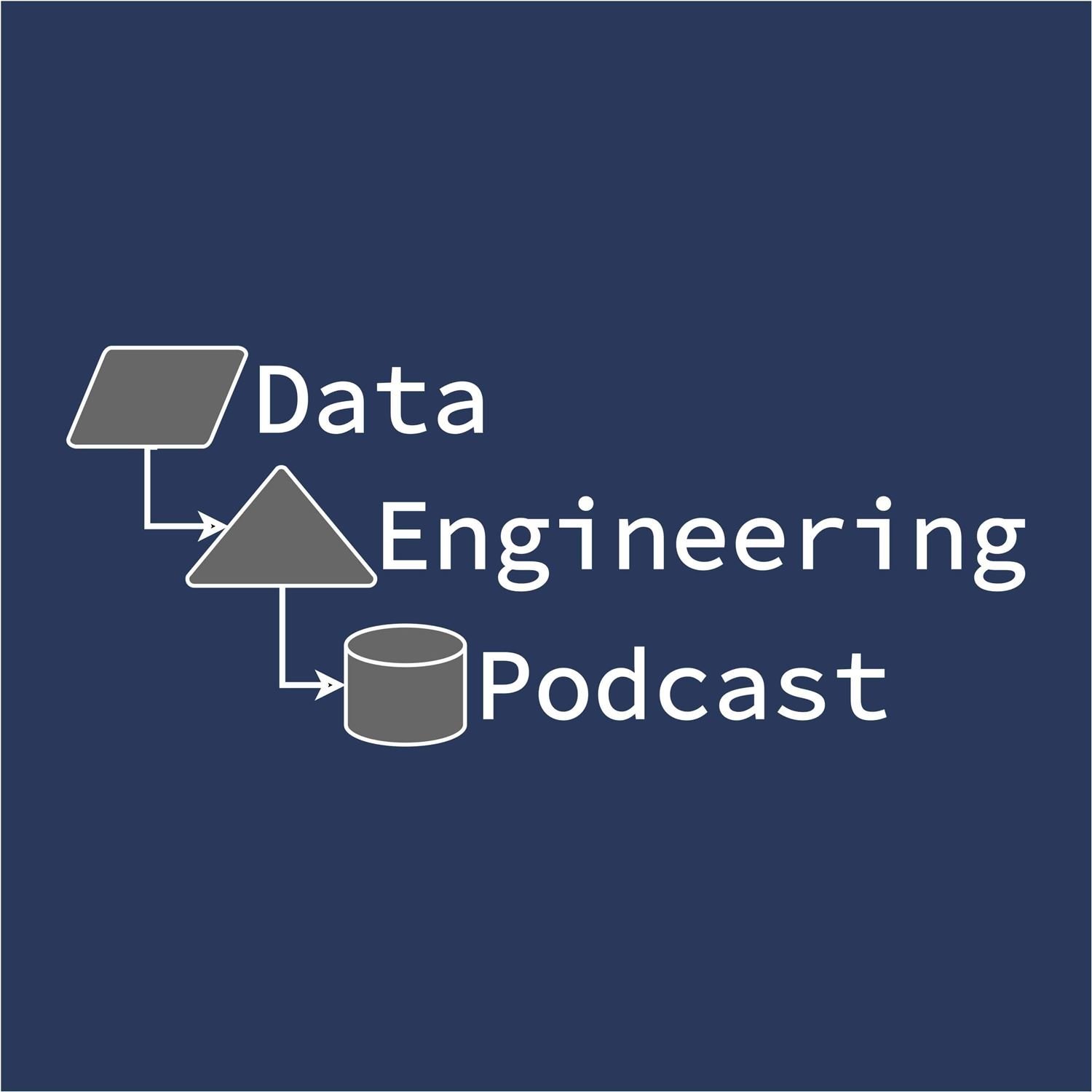
Easily Build Advanced Similarity Search With The Pinecone Vector Database
Summary
Machine learning models use vectors as the natural mechanism for representing their internal state. The problem is that in order for the models to integrate with external systems their internal state has to be translated into a lower dimension. To eliminate this impedance mismatch Edo Liberty founded Pinecone to build database that works natively with vectors. In this episode he explains how this technology will allow teams to accelerate the speed of innovation, how vectors make it possible to build more advanced search functionality, and how Pinecone is architected. This is an interesting conversation about how reconsidering the architecture of your systems can unlock impressive new capabilities.
Announcements
Hello and welcome to the Data Engineering Podcast, the show about modern data management
When you’re ready to build your next pipeline, or want to test out the projects you hear about on the show, you’ll need somewhere to deploy it, so check out our friends at Linode. With their managed Kubernetes platform it’s now even easier to deploy and scale your workflows, or try out the latest Helm charts from tools like Pulsar and Pachyderm. With simple pricing, fast networking, object storage, and worldwide data centers, you’ve got everything you need to run a bulletproof data platform. Go to dataengineeringpodcast.com/linode today and get a $100 credit to try out a Kubernetes cluster of your own. And don’t forget to thank them for their continued support of this show!
RudderStack’s smart customer data pipeline is warehouse-first. It builds your customer data warehouse and your identity graph on your data warehouse, with support for Snowflake, Google BigQuery, Amazon Redshift, and more. Their SDKs and plugins make event streaming easy, and their integrations with cloud applications like Salesforce and ZenDesk help you go beyond event streaming. With RudderStack you can use all of your customer data to answer more difficult questions and then send those insights to your whole customer data stack. Sign up free at dataengineeringpodcast.com/rudder today.
When it comes to serving data for AI and ML projects, do you feel like you have to rebuild the plane while you’re flying it across the ocean? Molecula is an enterprise feature store that operationalizes advanced analytics and AI in a format designed for massive machine-scale projects without having to manage endless one-off information requests. With Molecula, data engineers manage one single feature store that serves the entire organization with millisecond query performance whether in the cloud or at your data center. And since it is implemented as an overlay, Molecula doesn’t disrupt legacy systems. High-growth startups use Molecula’s feature store because of its unprecedented speed, cost savings, and simplified access to all enterprise data. From feature extraction to model training to production, the Molecula feature store provides continuously updated feature access, reuse, and sharing without the need to pre-process data. If you need to deliver unprecedented speed, cost savings, and simplified access to large scale, real-time data, visit dataengineeringpodcast.com/molecula and request a demo. Mention that you’re a Data Engineering Podcast listener, and they’ll send you a free t-shirt.
Your host is Tobias Macey and today I’m interviewing Edo Liberty about Pinecone, a vector database for powering machine learning and similarity search
Interview
Introduction
How did you get involved in the area of data management?
Can you start by describing what Pinecone is and the story behind it?
What are some of the contexts where someone would want to perform a similarity search?
What are the considerations that someone should be aware of when deciding between Pinecone and Solr/Lucene for a search oriented use case?
What are some of the other use cases that Pinecone enables?
In the absence of Pinecone, what kinds of systems and solutions are people building to address those use cases?
Where does Pinecone sit in the lifecycle of data and how does it integrate with the broader data management ecosystem?
What are some of the systems, tools, or frameworks that Pinecone might replace?
How is Pinecone implemented?
How has the architecture evolved since you first began working on it?
What are the most complex or difficult aspects of building Pinecone?
Who is your target user and how does that inform the user experience design and product development priorities?
For someone who wants to start using Pinecone, what is involved in populating it with data building an analysis or service with it?
What are some of the data modeling considerations when building a set of vectors in Pinecone?
What are some of the most interesting, unexpected, or innovative ways that you have seen Pinecone used?
What are the most interesting, unexpected, or challenging lessons that you have learned while building and growing the Pinecone technology and business?
When is Pinecone the wrong choice?
What do you have planned for the future of Pinecone?
Contact Info
Website
LinkedIn
Parting Question
From your perspective, what is the biggest gap in the tooling or technology for data management today?
Closing Announcements
Thank you for listening! Don’t forget to check out our other show, Podcast.__init__ to learn about the Python language, its community, and the innovative ways it is being used.
Visit the site to subscribe to the show, sign up for the mailing list, and read the show notes.
If you’ve learned something or tried out a project from the show then tell us about it! Email [email protected]) with your story.
To help other people find the show please leave a review on iTunes and tell your friends and co-workers
Join the community in the new Zulip chat workspace at dataengineeringpodcast.com/chat
Links
Pinecone
Theoretical Physics
High Dimensional Geometry
AWS Sagemaker
Visual Cortex
Temporal Lobe
Inverted Index
Elasticsearch
Podcast Episode
Solr
Lucene
NMSLib
Johnson-Lindenstrauss Lemma
The intro and outro music is from The Hug by The Freak Fandango Orchestra / CC BY-SA
Support Data Engineering Podcast
46:4825/05/2021
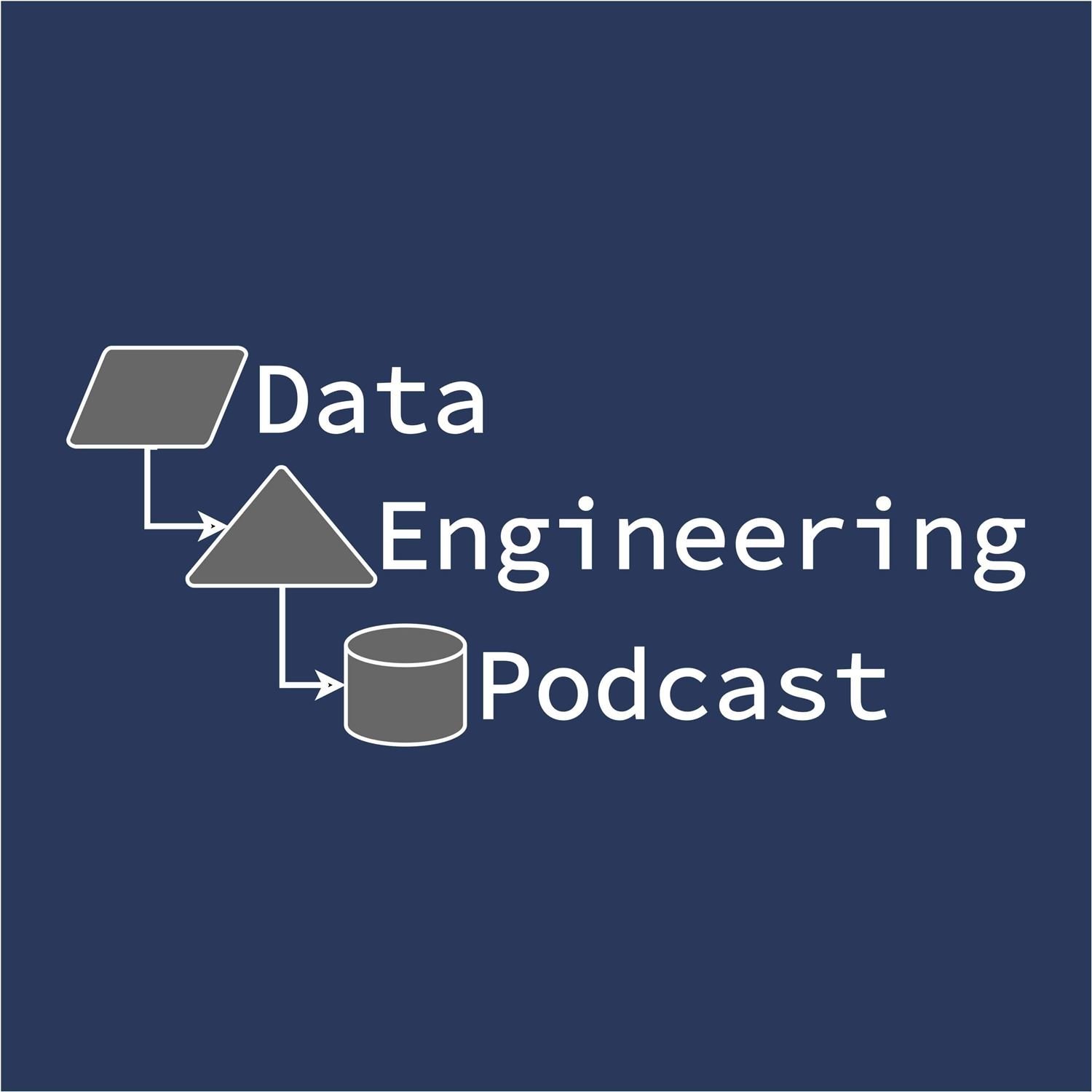
A Holistic Approach To Data Governance Through Self Reflection At Collibra
Summary
Data governance is a phrase that means many different things to many different people. This is because it is actually a concept that encompasses the entire lifecycle of data, across all of the people in an organization who interact with it. Stijn Christiaens co-founded Collibra with the goal of addressing the wide variety of technological aspects that are necessary to realize such an important and expansive process. In this episode he shares his thoughts on the balance between human and technological processes that are necessary for a well-managed data governance strategy, how Collibra is designed to aid in that endeavor, and his experiences using the platform that his company is building to help power the company. This is an excellent conversation that spans the engineering and philosophical complexities of an important and ever-present aspect of working with data.
Announcements
Hello and welcome to the Data Engineering Podcast, the show about modern data management
When you’re ready to build your next pipeline, or want to test out the projects you hear about on the show, you’ll need somewhere to deploy it, so check out our friends at Linode. With their managed Kubernetes platform it’s now even easier to deploy and scale your workflows, or try out the latest Helm charts from tools like Pulsar and Pachyderm. With simple pricing, fast networking, object storage, and worldwide data centers, you’ve got everything you need to run a bulletproof data platform. Go to dataengineeringpodcast.com/linode today and get a $100 credit to try out a Kubernetes cluster of your own. And don’t forget to thank them for their continued support of this show!
RudderStack’s smart customer data pipeline is warehouse-first. It builds your customer data warehouse and your identity graph on your data warehouse, with support for Snowflake, Google BigQuery, Amazon Redshift, and more. Their SDKs and plugins make event streaming easy, and their integrations with cloud applications like Salesforce and ZenDesk help you go beyond event streaming. With RudderStack you can use all of your customer data to answer more difficult questions and then send those insights to your whole customer data stack. Sign up free at dataengineeringpodcast.com/rudder today.
Your host is Tobias Macey and today I’m interviewing Stijn Christiaens about data governance in the enterprise and how Collibra applies the lessons learned from their customers to their own business
Interview
Introduction
How did you get involved in the area of data management?
Can you start by describing what you are building at Collibra and the story behind the company?
Wat does "data governance" mean to you, and how does that definition inform your work at Collibra?
How would you characterize the current landscape of "data governance" offerings and Collibra’s position within it?
What are the elements of governance that are often ignored in small/medium businesses but which are essential for the enterprise? (e.g. data stewards, business glossaries, etc.)
One of the most important tasks as a data professional is to establish and maintain trust in the information you are curating. What are the biggest obstacles to overcome in that mission?
What are some of the data problems that you will only find at large or complex organizations?
How does Collibra help to tame that complexity?
Who are the end users of Collibra within an organization?
Can you talk through the workflow and various interactions that your customers have as it relates to the overall flow of data through an organization?
Can you describe how the Collibra platform is implemented?
How has the scope and design of the system evolved since you first began working on it?
You are currently leading a team that uses Collibra to manage the operations of the business. What are some of the most notable surprises that you have learned from being your own customer?
What are some of the weak points that you have been able to identify and resolve?
How have you been able to use those lessons to help your customers?
What are the activities that are resistant to automation?
How do you design the system to allow for a smooth handoff between mechanistic and humanistic processes?
What are some of the most interesting, innovative, or unexpected ways that you have seen Collibra used?
What are the most interesting, unexpected, or challenging lessons that you have learned while building and growing Collibra, and running the internal data office?
When is Collibra the wrong choice?
What do you have planned for the future of the platform?
Contact Info
LinkedIn
@stichris on Twitter
Parting Question
From your perspective, what is the biggest gap in the tooling or technology for data management today?
Links
Collibra
Collibra Data Office
Electrical Engineering
Resistor Color Codes
STAR Lab (semantics, technology, and research)
Microsoft Azure
Data Governance
GDPR
Chief Data Officer
Dunbar’s Number
Business Glossary
Data Steward
ERP == Enterprise Resource Planning
CRM == Customer Relationship Management
Data Ownership
Data Mesh
Podcast Episode
The intro and outro music is from The Hug by The Freak Fandango Orchestra / CC BY-SA
Support Data Engineering Podcast
55:5321/05/2021
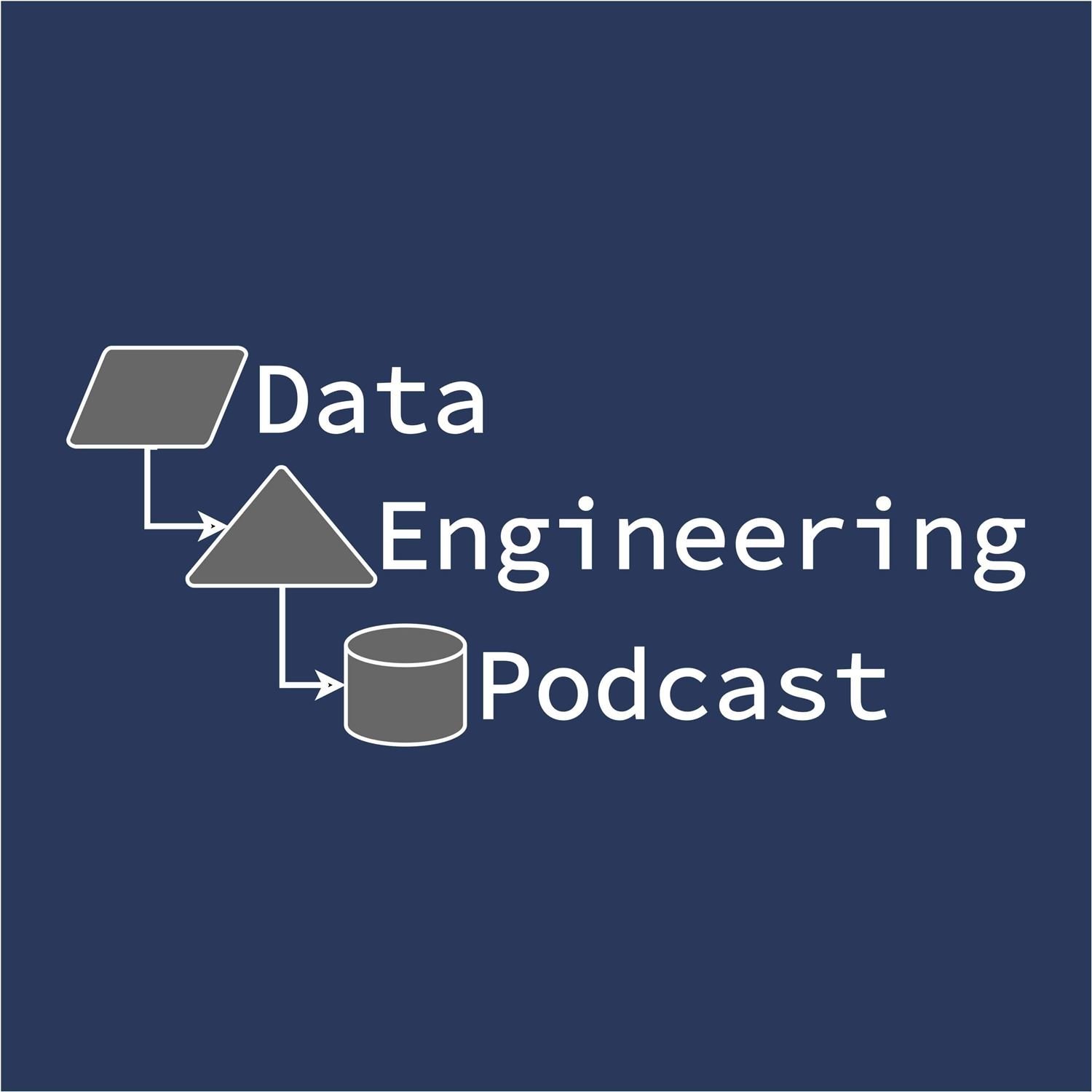
Unlocking The Power of Data Lineage In Your Platform with OpenLineage
Summary
Data lineage is the common thread that ties together all of your data pipelines, workflows, and systems. In order to get a holistic understanding of your data quality, where errors are occurring, or how a report was constructed you need to track the lineage of the data from beginning to end. The complicating factor is that every framework, platform, and product has its own concepts of how to store, represent, and expose that information. In order to eliminate the wasted effort of building custom integrations every time you want to combine lineage information across systems Julien Le Dem introduced the OpenLineage specification. In this episode he explains his motivations for starting the effort, the far-reaching benefits that it can provide to the industry, and how you can start integrating it into your data platform today. This is an excellent conversation about how competing companies can still find mutual benefit in co-operating on open standards.
Announcements
Hello and welcome to the Data Engineering Podcast, the show about modern data management
When you’re ready to build your next pipeline, or want to test out the projects you hear about on the show, you’ll need somewhere to deploy it, so check out our friends at Linode. With their managed Kubernetes platform it’s now even easier to deploy and scale your workflows, or try out the latest Helm charts from tools like Pulsar and Pachyderm. With simple pricing, fast networking, object storage, and worldwide data centers, you’ve got everything you need to run a bulletproof data platform. Go to dataengineeringpodcast.com/linode today and get a $100 credit to try out a Kubernetes cluster of your own. And don’t forget to thank them for their continued support of this show!
RudderStack’s smart customer data pipeline is warehouse-first. It builds your customer data warehouse and your identity graph on your data warehouse, with support for Snowflake, Google BigQuery, Amazon Redshift, and more. Their SDKs and plugins make event streaming easy, and their integrations with cloud applications like Salesforce and ZenDesk help you go beyond event streaming. With RudderStack you can use all of your customer data to answer more difficult questions and then send those insights to your whole customer data stack. Sign up free at dataengineeringpodcast.com/rudder today.
When it comes to serving data for AI and ML projects, do you feel like you have to rebuild the plane while you’re flying it across the ocean? Molecula is an enterprise feature store that operationalizes advanced analytics and AI in a format designed for massive machine-scale projects without having to manage endless one-off information requests. With Molecula, data engineers manage one single feature store that serves the entire organization with millisecond query performance whether in the cloud or at your data center. And since it is implemented as an overlay, Molecula doesn’t disrupt legacy systems. High-growth startups use Molecula’s feature store because of its unprecedented speed, cost savings, and simplified access to all enterprise data. From feature extraction to model training to production, the Molecula feature store provides continuously updated feature access, reuse, and sharing without the need to pre-process data. If you need to deliver unprecedented speed, cost savings, and simplified access to large scale, real-time data, visit dataengineeringpodcast.com/molecula and request a demo. Mention that you’re a Data Engineering Podcast listener, and they’ll send you a free t-shirt.
Your host is Tobias Macey and today I’m interviewing Julien Le Dem about Open Lineage, a new standard for structuring metadata to enable interoperability across the ecosystem of data management tools.
Interview
Introduction
How did you get involved in the area of data management?
Can you start by giving an overview of what the Open Lineage project is and the story behind it?
What is the current state of the ecosystem for generating and sharing metadata between systems?
What are your goals for the OpenLineage effort?
What are the biggest conceptual or consistency challenges that you are facing in defining a metadata model that is broad and flexible enough to be widely used while still being prescriptive enough to be useful?
What is the current state of the project? (e.g. code available, maturity of the specification, etc.)
What are some of the ideas or assumptions that you had at the beginning of this project that have had to be revisited as you iterate on the definition and implementation?
What are some of the projects/organizations/etc. that have committed to supporting or adopting OpenLineage?
What problem domain(s) are best suited to adopting OpenLineage?
What are some of the problems or use cases that you are explicitly not including in scope for OpenLineage?
For someone who already has a lineage and/or metadata catalog, what is involved in evolving that system to work well with OpenLineage?
What are some of the downstream/long-term impacts that you anticipate or hope that this standardization effort will generate?
What are some of the most interesting, unexpected, or challenging lessons that you have learned while working on the OpenLineage effort?
What do you have planned for the future of the project?
Contact Info
LinkedIn
@J_ on Twitter
julienledem on GitHub
Parting Question
From your perspective, what is the biggest gap in the tooling or technology for data management today?
Closing Announcements
Thank you for listening! Don’t forget to check out our other show, Podcast.__init__ to learn about the Python language, its community, and the innovative ways it is being used.
Visit the site to subscribe to the show, sign up for the mailing list, and read the show notes.
If you’ve learned something or tried out a project from the show then tell us about it! Email [email protected]) with your story.
To help other people find the show please leave a review on iTunes and tell your friends and co-workers
Join the community in the new Zulip chat workspace at dataengineeringpodcast.com/chat
Links
OpenLineage
Marquez
Podcast Episode
Hadoop
Pig
Apache Parquet
Podcast Episode
Doug Cutting
Avro
Apache Arrow
Service Oriented Architecture
Data Lineage
Apache Atlas
DataHub
Podcast Episode
Amundsen
Podcast Episode
Egeria
Pandas
Podcast.__init__ Episode
Apache Spark
EXIF
JSON Schema
OpenTelemetry
Podcast.__init__ Episode
OpenTracing
Superset
Podcast.__init__ Episode
Data Engineering Podcast Episode
Iceberg
Podcast Episode
Great Expectations
Podcast Episode
dbt
Podcast Episode
Data Mesh
Podcast Episode
The map is not the territory
Kafka
Apache Flink
Apache Storm
Kafka Streams
Stone Soup
Apache Beam
Linux Foundation AI & Data
The intro and outro music is from The Hug by The Freak Fandango Orchestra / CC BY-SA
Support Data Engineering Podcast
57:3918/05/2021
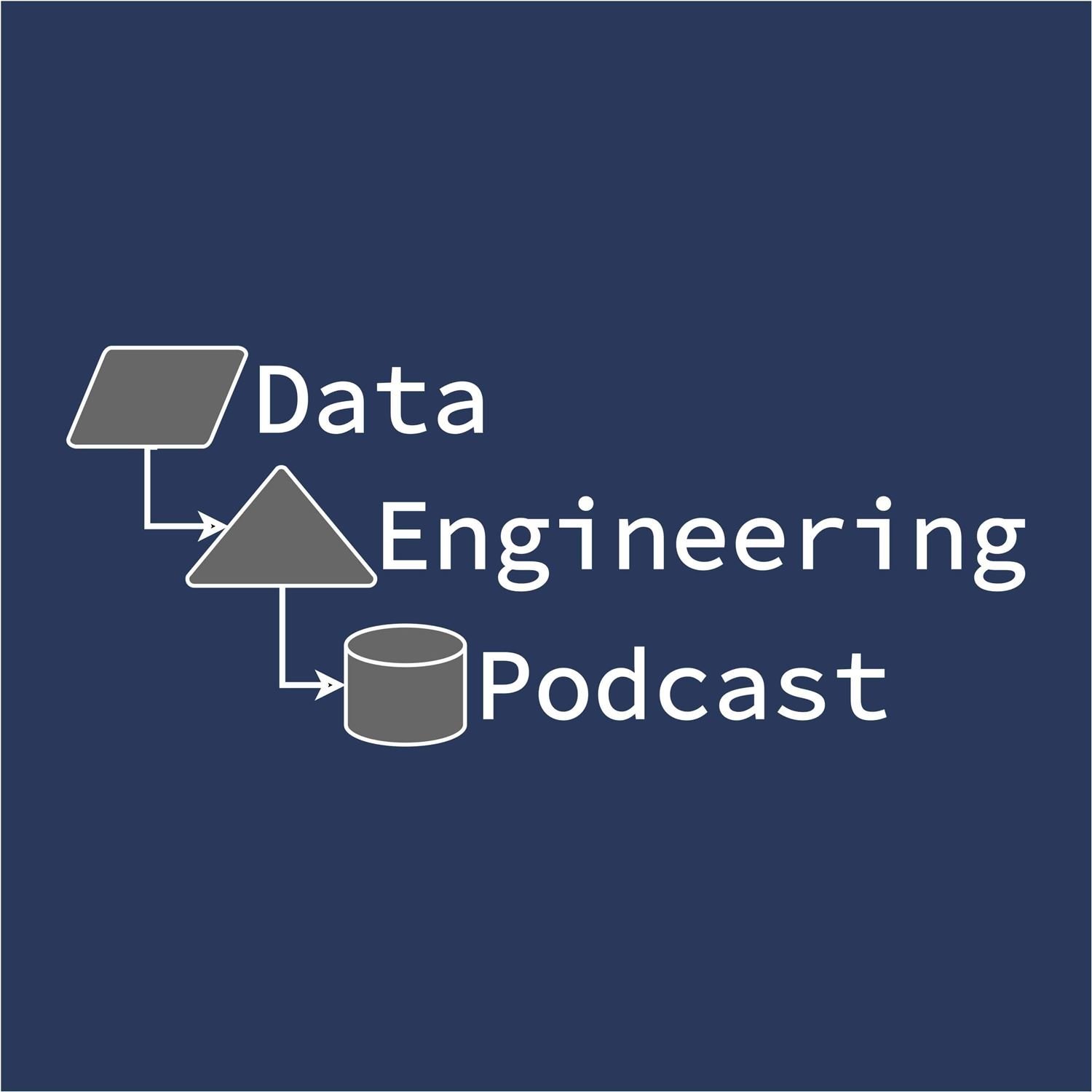
Building Your Data Warehouse On Top Of PostgreSQL
Summary
There is a lot of attention on the database market and cloud data warehouses. While they provide a measure of convenience, they also require you to sacrifice a certain amount of control over your data. If you want to build a warehouse that gives you both control and flexibility then you might consider building on top of the venerable PostgreSQL project. In this episode Thomas Richter and Joshua Drake share their advice on how to build a production ready data warehouse with Postgres.
Announcements
Hello and welcome to the Data Engineering Podcast, the show about modern data management
When you’re ready to build your next pipeline, or want to test out the projects you hear about on the show, you’ll need somewhere to deploy it, so check out our friends at Linode. With their managed Kubernetes platform it’s now even easier to deploy and scale your workflows, or try out the latest Helm charts from tools like Pulsar and Pachyderm. With simple pricing, fast networking, object storage, and worldwide data centers, you’ve got everything you need to run a bulletproof data platform. Go to dataengineeringpodcast.com/linode today and get a $100 credit to try out a Kubernetes cluster of your own. And don’t forget to thank them for their continued support of this show!
Firebolt is the fastest cloud data warehouse. Visit dataengineeringpodcast.com/firebolt to get started. The first 25 visitors will receive a Firebolt t-shirt.
Atlan is a collaborative workspace for data-driven teams, like Github for engineering or Figma for design teams. By acting as a virtual hub for data assets ranging from tables and dashboards to SQL snippets & code, Atlan enables teams to create a single source of truth for all their data assets, and collaborate across the modern data stack through deep integrations with tools like Snowflake, Slack, Looker and more. Go to dataengineeringpodcast.com/atlan today and sign up for a free trial. If you’re a data engineering podcast listener, you get credits worth $3000 on an annual subscription
Your host is Tobias Macey and today I’m interviewing Thomas Richter and Joshua Drake about using Postgres as your data warehouse
Interview
Introduction
How did you get involved in the area of data management?
Can you start by establishing a working definition of what constitutes a data warehouse for the purpose of this discussion?
What are the limitations for out-of-the-box Postgres when trying to use it for these workloads?
There are a large and growing number of options for data warehouse style workloads. How would you categorize the different systems and what is PostgreSQL’s position in that ecosystem?
What do you see as the motivating factors for a team or organization to select from among those categories?
Why would someone want to use Postgres as their data warehouse platform rather than using a purpose-built engine?
What is the cost/performance equation for Postgres as compared to other data warehouse solutions?
For someone who wants to turn Postgres into a data warehouse engine, what are their options?
What are the relative tradeoffs of the different open source and commercial offerings? (e.g. Citus, cstore_fdw, zedstore, Swarm64, Greenplum, etc.)
One of the biggest areas of growth right now is in the "cloud data warehouse" market where storage and compute are decoupled. What are the options for making that possible with Postgres? (e.g. using foreign data wrappers for interacting with data lake storage (S3, HDFS, Alluxio, etc.))
What areas of work are happening in the Postgres community for upcoming releases to make it more easily suited to data warehouse/analytical workloads?
What are some of the most interesting, innovative, or unexpected ways that you have seen Postgres used in analytical contexts?
What are the most interesting, unexpected, or challenging lessons that you have learned from your own experiences of building analytical systems with Postgres?
When is Postgres the wrong choice for a data warehouse?
What are you most excited for/what are you keeping an eye on in upcoming releases of Postgres and its ecosystem?
Contact Info
Thomas
LinkedIn
JD
LinkedIn
@linuxhiker on Twitter
Parting Question
From your perspective, what is the biggest gap in the tooling or technology for data management today?
Links
PostgreSQL
Podcast Episode
Swarm64
Podcast Episode
Command Prompt Inc.
IBM
Cognos
OLAP Cube
MariaDB
MySQL
Powell’s Books
DBase
Practical PostgreSQL
Netezza
Presto
Trino
Apache Drill
Parquet
Parquet Foreign Data Wrapper
Snowflake
Podcast Episode
Amazon RDS
Amazon Aurora
Hyperscale
Citus
TimescaleDB
Podcast Episode
Followup Podcast Episode
Greenplum
zedstore
Redshift
Microsoft SQL Server
Postgres Tablespaces
Debezium
Podcast Episode
EDI == Enterprise Data Integration
Change Data Capture
Podcast Episode
The intro and outro music is from The Hug by The Freak Fandango Orchestra / CC BY-SA
Support Data Engineering Podcast
01:15:0714/05/2021
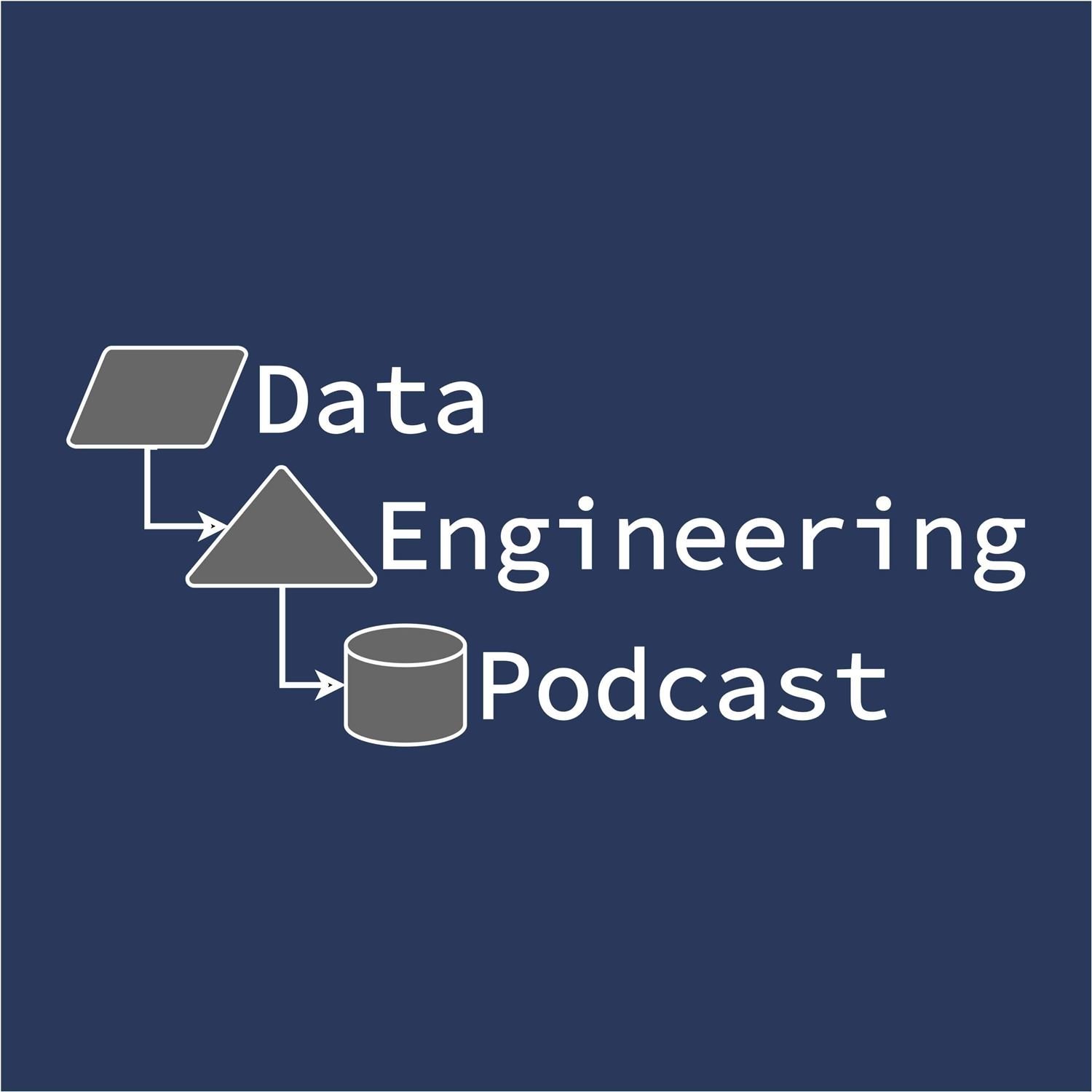
Making Analytical APIs Fast With Tinybird
Summary
Building an API for real-time data is a challenging project. Making it robust, scalable, and fast is a full time job. The team at Tinybird wants to make it easy to turn a continuous stream of data into a production ready API or data product. In this episode CEO Jorge Sancha explains how they have architected their system to handle high data throughput and fast response times, and why they have invested heavily in Clickhouse as the core of their platform. This is a great conversation about the challenges of building a maintainable business from a technical and product perspective.
Announcements
Hello and welcome to the Data Engineering Podcast, the show about modern data management
When you’re ready to build your next pipeline, or want to test out the projects you hear about on the show, you’ll need somewhere to deploy it, so check out our friends at Linode. With their managed Kubernetes platform it’s now even easier to deploy and scale your workflows, or try out the latest Helm charts from tools like Pulsar and Pachyderm. With simple pricing, fast networking, object storage, and worldwide data centers, you’ve got everything you need to run a bulletproof data platform. Go to dataengineeringpodcast.com/linode today and get a $100 credit to try out a Kubernetes cluster of your own. And don’t forget to thank them for their continued support of this show!
RudderStack’s smart customer data pipeline is warehouse-first. It builds your customer data warehouse and your identity graph on your data warehouse, with support for Snowflake, Google BigQuery, Amazon Redshift, and more. Their SDKs and plugins make event streaming easy, and their integrations with cloud applications like Salesforce and ZenDesk help you go beyond event streaming. With RudderStack you can use all of your customer data to answer more difficult questions and then send those insights to your whole customer data stack. Sign up free at dataengineeringpodcast.com/rudder today.
Ascend.io — recognized as a 2021 Gartner Cool Vendor in Enterprise AI Operationalization and Engineering—empowers data teams to to build, scale, and operate declarative data pipelines with 95% less code and zero maintenance. Connect to any data source using Ascend’s new flex code data connectors, rapidly iterate on transformations and send data to any destination in a fraction of the time it traditionally takes—just ask companies like Harry’s, HNI, and Mayvenn. Sound exciting? Come join the team! We’re hiring data engineers, so head on over to dataengineeringpodcast.com/ascend and check out our careers page to learn more.
Your host is Tobias Macey and today I’m interviewing Jorge Sancha about Tinybird, a platform to easily build analytical APIs for real-time data
Interview
Introduction
How did you get involved in the area of data management?
Can you start by describing what you are building at Tinybird and the story behind it?
What are some of the types of use cases that your customers are focused on?
What are the areas of complexity that come up when building analytical APIs that are often overlooked when first designing a system to operate on and expose real-time data?
What are the supporting systems that are necessary and useful for operating this kind of system which contribute to the overall time and engineering cost beyond the baseline functionality?
How is the Tinybird platform architected?
How have the goals and implementation of Tinybird changed or evolved since you first began building it?
What was your criteria for selecting the core building block of your platform, and how did that lead to your choice to build on top of Clickhouse?
What are some of the sharp edges that you have run into while operating Clickhouse?
What are some of the custom tools or systems that you have built to help deal with them?
What are some of the performance challenges that an API built with Tinybird might run into?
What are the considerations that users should be aware of to avoid introducing performance issues?
How do you handle multi-tenancy in your platform? (e.g. separate clusters, in-database quotas, etc.)
For users of Tinybird, can you talk through the workflow of getting it integrated into their platform and designing an API from their data?
What are some of the most interesting, innovative, or unexpected ways that you have seen Tinybird used?
What are the most interesting, unexpected, or challenging lessons that you have learned while building and growing Tinybird?
When is Tinybird the wrong choice?
What do you have planned for the future of the product and business?
Contact Info
@jorgesancha on Twitter
LinkedIn
jorgesancha on GitHub
Parting Question
From your perspective, what is the biggest gap in the tooling or technology for data management today?
Links
Tinybird
Carto
PostgreSQL
Podcast Episode
PostGIS
Clickhouse
Podcast Episode
Kafka
Tornado
Podcast.__init__ Episode
Redis
Formula 1
Web Application Firewall
The intro and outro music is from The Hug by The Freak Fandango Orchestra / CC BY-SA
Support Data Engineering Podcast
54:2411/05/2021
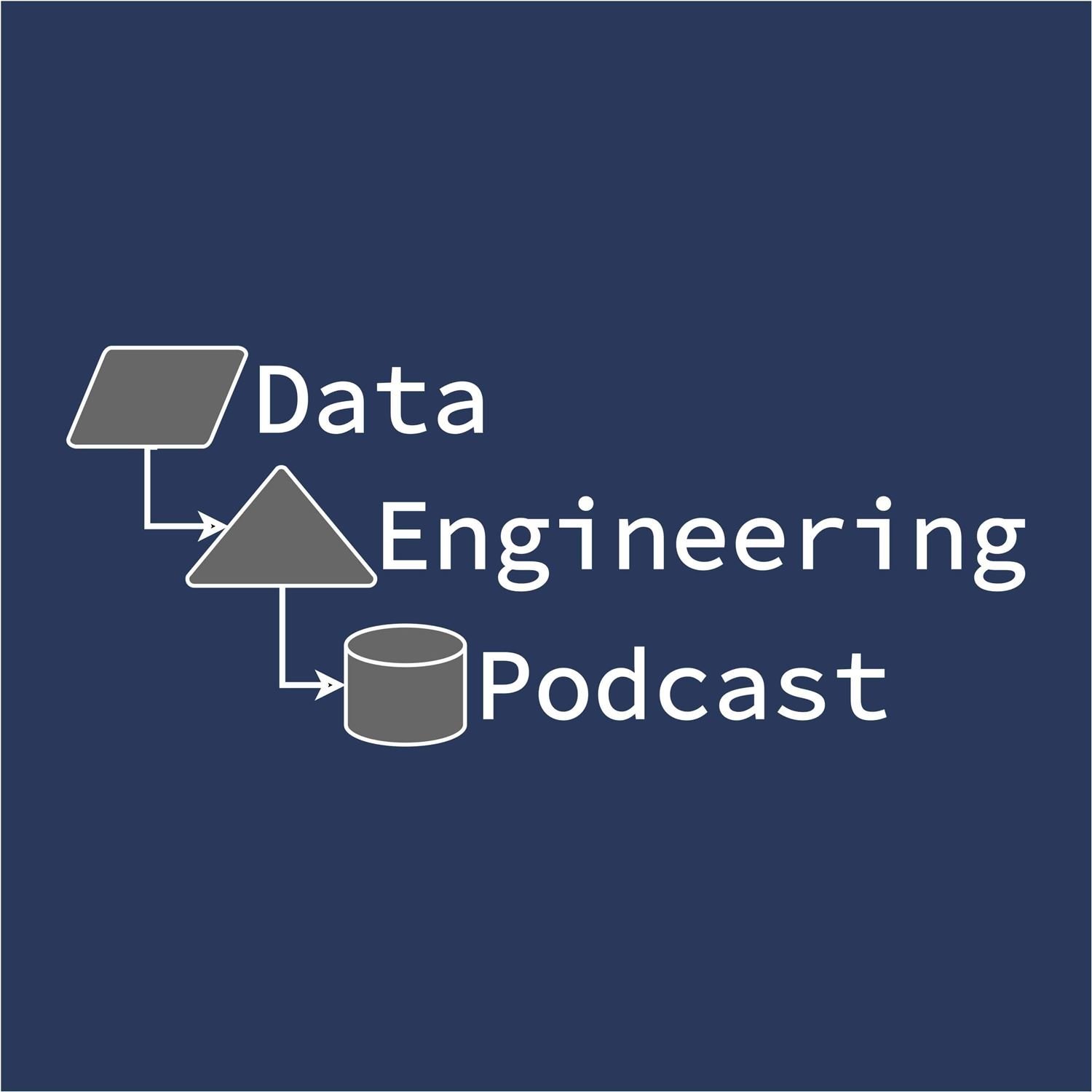
Making Spark Cloud Native At Data Mechanics
Summary
Spark is one of the most well-known frameworks for data processing, whether for batch or streaming, ETL or ML, and at any scale. Because of its popularity it has been deployed on every kind of platform you can think of. In this episode Jean-Yves Stephan shares the work that he is doing at Data Mechanics to make it sing on Kubernetes. He explains how operating in a cloud-native context simplifies some aspects of running the system while complicating others, how it simplifies the development and experimentation cycle, and how you can get a head start using their pre-built Spark container. This is a great conversation for understanding how new ways of operating systems can have broader impacts on how they are being used.
Announcements
Hello and welcome to the Data Engineering Podcast, the show about modern data management
When you’re ready to build your next pipeline, or want to test out the projects you hear about on the show, you’ll need somewhere to deploy it, so check out our friends at Linode. With their managed Kubernetes platform it’s now even easier to deploy and scale your workflows, or try out the latest Helm charts from tools like Pulsar and Pachyderm. With simple pricing, fast networking, object storage, and worldwide data centers, you’ve got everything you need to run a bulletproof data platform. Go to dataengineeringpodcast.com/linode today and get a $100 credit to try out a Kubernetes cluster of your own. And don’t forget to thank them for their continued support of this show!
Firebolt is the fastest cloud data warehouse. Visit dataengineeringpodcast.com/firebolt to get started. The first 25 visitors will receive a Firebolt t-shirt.
Atlan is a collaborative workspace for data-driven teams, like Github for engineering or Figma for design teams. By acting as a virtual hub for data assets ranging from tables and dashboards to SQL snippets & code, Atlan enables teams to create a single source of truth for all their data assets, and collaborate across the modern data stack through deep integrations with tools like Snowflake, Slack, Looker and more. Go to dataengineeringpodcast.com/atlan today and sign up for a free trial. If you’re a data engineering podcast listener, you get credits worth $3000 on an annual subscription
Your host is Tobias Macey and today I’m interviewing Jean-Yves Stephan about Data Mechanics, a cloud-native Spark platform for data engineers
Interview
Introduction
How did you get involved in the area of data management?
Can you start by giving an overview of what you are building at Data Mechanics and the story behind it?
What are the operational characteristics of Spark that make it difficult to run in a cloud-optimized environment?
How do you handle retries, state redistribution, etc. when instances get pre-empted during the middle of a job execution?
What are some of the tactics that you have found useful when designing jobs to make them more resilient to interruptions?
What are the customizations that you have had to make to Spark itself?
What are some of the supporting tools that you have built to allow for running Spark in a Kubernetes environment?
How is the Data Mechanics platform implemented?
How have the goals and design of the platform changed or evolved since you first began working on it?
How does running Spark in a container/Kubernetes environment change the ways that you and your customers think about how and where to use it?
How does it impact the development workflow for data engineers and data scientists?
What are some of the most interesting, unexpected, or challenging lessons that you have learned while building the Data Mechanics product?
When is Spark/Data Mechanics the wrong choice?
What do you have planned for the future of the platform?
Contact Info
LinkedIn
Parting Question
From your perspective, what is the biggest gap in the tooling or technology for data management today?
Links
Data Mechanics
Databricks
Stanford
Andrew Ng
Mining Massive Datasets
Spark
Kubernetes
Spot Instances
Infiniband
Data Mechanics Spark Container Image
Delight – Spark monitoring utility
Terraform
Blue/Green Deployment
Spark Operator for Kubernetes
JupyterHub
Jupyter Enterprise Gateway
The intro and outro music is from The Hug by The Freak Fandango Orchestra / CC BY-SA
Support Data Engineering Podcast
40:1607/05/2021
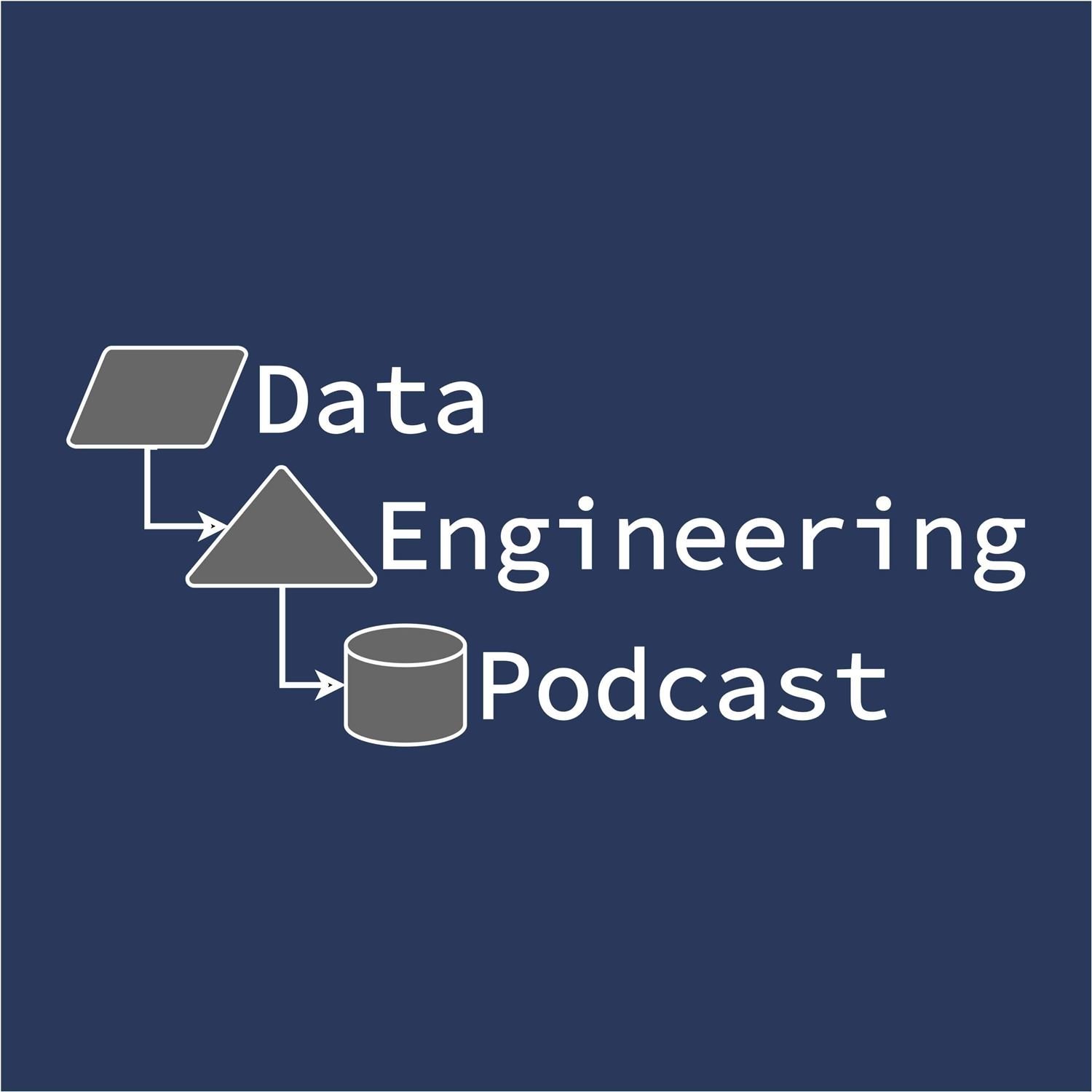
The Grand Vision And Present Reality of DataOps
Summary
The Data industry is changing rapidly, and one of the most active areas of growth is automation of data workflows. Taking cues from the DevOps movement of the past decade data professionals are orienting around the concept of DataOps. More than just a collection of tools, there are a number of organizational and conceptual changes that a proper DataOps approach depends on. In this episode Kevin Stumpf, CTO of Tecton, Maxime Beauchemin, CEO of Preset, and Lior Gavish, CTO of Monte Carlo, discuss the grand vision and present realities of DataOps. They explain how to think about your data systems in a holistic and maintainable fashion, the security challenges that threaten to derail your efforts, and the power of using metadata as the foundation of everything that you do. If you are wondering how to get control of your data platforms and bring all of your stakeholders onto the same page then this conversation is for you.
Announcements
Hello and welcome to the Data Engineering Podcast, the show about modern data management
When you’re ready to build your next pipeline, or want to test out the projects you hear about on the show, you’ll need somewhere to deploy it, so check out our friends at Linode. With their managed Kubernetes platform it’s now even easier to deploy and scale your workflows, or try out the latest Helm charts from tools like Pulsar and Pachyderm. With simple pricing, fast networking, object storage, and worldwide data centers, you’ve got everything you need to run a bulletproof data platform. Go to dataengineeringpodcast.com/linode today and get a $100 credit to try out a Kubernetes cluster of your own. And don’t forget to thank them for their continued support of this show!
Modern Data teams are dealing with a lot of complexity in their data pipelines and analytical code. Monitoring data quality, tracing incidents, and testing changes can be daunting and often takes hours to days. Datafold helps Data teams gain visibility and confidence in the quality of their analytical data through data profiling, column-level lineage and intelligent anomaly detection. Datafold also helps automate regression testing of ETL code with its Data Diff feature that instantly shows how a change in ETL or BI code affects the produced data, both on a statistical level and down to individual rows and values. Datafold integrates with all major data warehouses as well as frameworks such as Airflow & dbt and seamlessly plugs into CI workflows. Go to dataengineeringpodcast.com/datafold today to start a 30-day trial of Datafold. Once you sign up and create an alert in Datafold for your company data, they will send you a cool water flask.
RudderStack’s smart customer data pipeline is warehouse-first. It builds your customer data warehouse and your identity graph on your data warehouse, with support for Snowflake, Google BigQuery, Amazon Redshift, and more. Their SDKs and plugins make event streaming easy, and their integrations with cloud applications like Salesforce and ZenDesk help you go beyond event streaming. With RudderStack you can use all of your customer data to answer more difficult questions and then send those insights to your whole customer data stack. Sign up free at dataengineeringpodcast.com/rudder today.
Your host is Tobias Macey and today I’m interviewing Max Beauchemin, Lior Gavish, and Kevin Stumpf about the real world challenges of embracing DataOps practices and systems, and how to keep things secure as you scale
Interview
Introduction
How did you get involved in the area of data management?
Before we get started, can you each give your definition of what "DataOps" means to you?
How does this differ from "business as usual" in the data industry?
What are some of the things that DataOps isn’t (despite what marketers might say)?
What are the biggest difficulties that you have faced in going from concept to production with a workflow or system intended to power self-serve access to other members of the organization?
What are the weak points in the current state of the industry, whether technological or social, that contribute to your greatest sense of unease from a security perspective?
As founders of companies that aim to facilitate adoption of various aspects of DataOps, how are you applying the products that you are building to your own internal systems?
How does security factor into the design of robust DataOps systems? What are some of the biggest challenges related to security when it comes to putting these systems into production?
What are the biggest differences between DevOps and DataOps, particularly when it concerns designing distributed systems?
What areas of the DataOps landscape do you think are ripe for innovation?
Nowadays, it seems like new DataOps companies are cropping up every day to try and solve some of these problems. Why do you think DataOps is becoming such an important component of the modern data stack?
There’s been a lot of conversation recently around the "rise of the data engineer" versus other roles in the data ecosystem (i.e. data scientist or data analyst). Why do you think that is?
What are some of the most valuable lessons that you have learned from working with your customers about how to apply DataOps principles?
What are some of the most interesting, unexpected, or challenging lessons that you have learned while building your respective platforms and businesses?
What are the industry trends that you are each keeping an eye on to inform you future product direction?
Contact Info
Kevin
LinkedIn
kevinstumpf on GitHub
@kevinstumpf on Twitter
Maxime
LinkedIn
@mistercrunch on Twitter
mistercrunch on GitHub
Lior
LinkedIn
@lgavish on Twitter
Parting Question
From your perspective, what is the biggest gap in the tooling or technology for data management today?
Closing Announcements
Thank you for listening! Don’t forget to check out our other show, Podcast.__init__ to learn about the Python language, its community, and the innovative ways it is being used.
Visit the site to subscribe to the show, sign up for the mailing list, and read the show notes.
If you’ve learned something or tried out a project from the show then tell us about it! Email [email protected]) with your story.
To help other people find the show please leave a review on iTunes and tell your friends and co-workers
Join the community in the new Zulip chat workspace at dataengineeringpodcast.com/chat
Links
Tecton
Monte Carlo
Superset
Preset
Barracuda Networks
Feature Store
DataOps
DevOps
Data Catalog
Amundsen
OpenLineage
The Downfall of the Data Engineer
Hashicorp Vault
Reverse ELT
The intro and outro music is from The Hug by The Freak Fandango Orchestra / CC BY-SA
Support Data Engineering Podcast
57:0804/05/2021
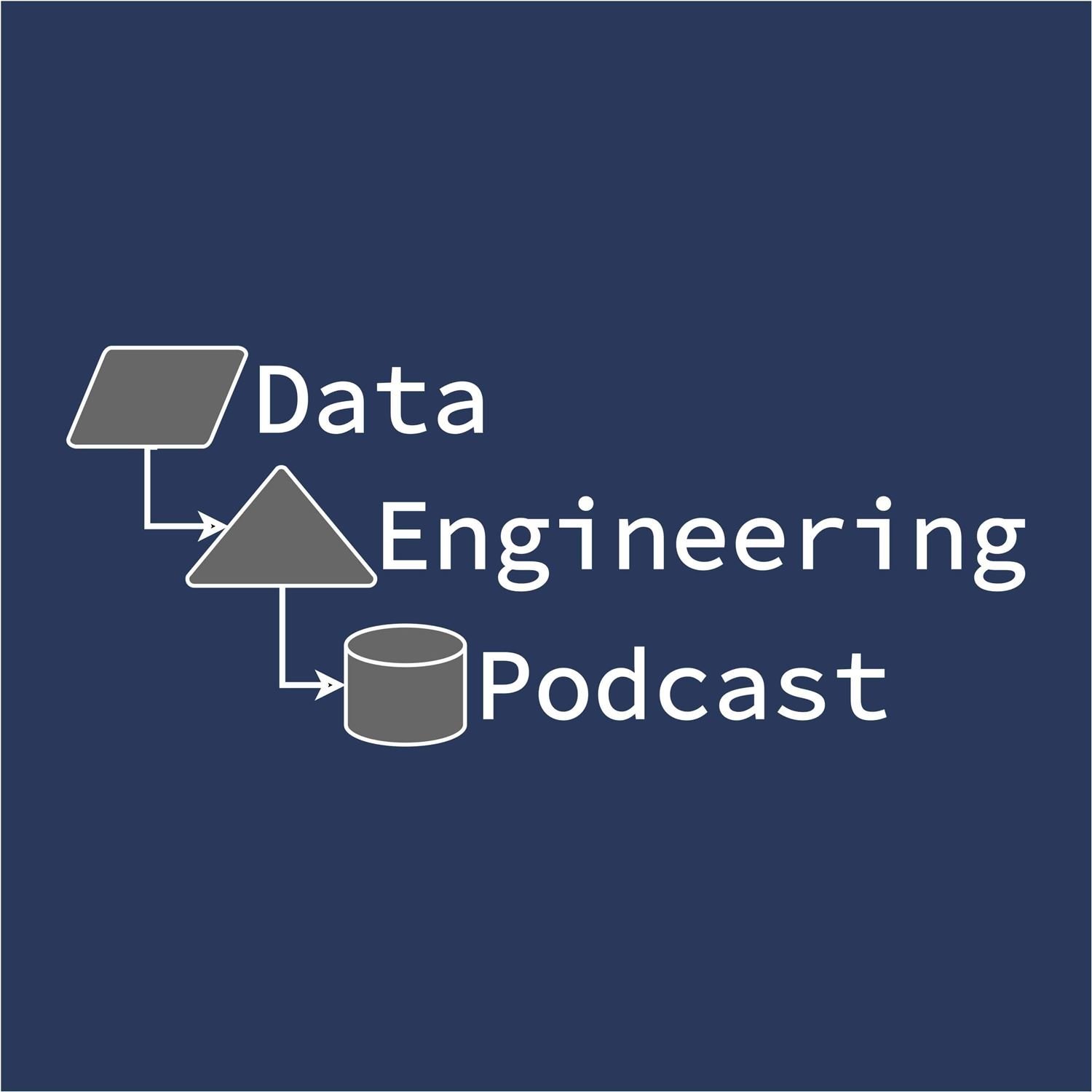
Self Service Data Exploration And Dashboarding With Superset
Summary
The reason for collecting, cleaning, and organizing data is to make it usable by the organization. One of the most common and widely used methods of access is through a business intelligence dashboard. Superset is an open source option that has been gaining popularity due to its flexibility and extensible feature set. In this episode Maxime Beauchemin discusses how data engineers can use Superset to provide self service access to data and deliver analytics. He digs into how it integrates with your data stack, how you can extend it to fit your use case, and why open source systems are a good choice for your business intelligence. If you haven’t already tried out Superset then this conversation is well worth your time. Give it a listen and then take it for a test drive today.
Announcements
Hello and welcome to the Data Engineering Podcast, the show about modern data management
When you’re ready to build your next pipeline, or want to test out the projects you hear about on the show, you’ll need somewhere to deploy it, so check out our friends at Linode. With their managed Kubernetes platform it’s now even easier to deploy and scale your workflows, or try out the latest Helm charts from tools like Pulsar and Pachyderm. With simple pricing, fast networking, object storage, and worldwide data centers, you’ve got everything you need to run a bulletproof data platform. Go to dataengineeringpodcast.com/linode today and get a $100 credit to try out a Kubernetes cluster of your own. And don’t forget to thank them for their continued support of this show!
Modern Data teams are dealing with a lot of complexity in their data pipelines and analytical code. Monitoring data quality, tracing incidents, and testing changes can be daunting and often takes hours to days. Datafold helps Data teams gain visibility and confidence in the quality of their analytical data through data profiling, column-level lineage and intelligent anomaly detection. Datafold also helps automate regression testing of ETL code with its Data Diff feature that instantly shows how a change in ETL or BI code affects the produced data, both on a statistical level and down to individual rows and values. Datafold integrates with all major data warehouses as well as frameworks such as Airflow & dbt and seamlessly plugs into CI workflows. Go to dataengineeringpodcast.com/datafold today to start a 30-day trial of Datafold. Once you sign up and create an alert in Datafold for your company data, they will send you a cool water flask.
RudderStack’s smart customer data pipeline is warehouse-first. It builds your customer data warehouse and your identity graph on your data warehouse, with support for Snowflake, Google BigQuery, Amazon Redshift, and more. Their SDKs and plugins make event streaming easy, and their integrations with cloud applications like Salesforce and ZenDesk help you go beyond event streaming. With RudderStack you can use all of your customer data to answer more difficult questions and then send those insights to your whole customer data stack. Sign up free at dataengineeringpodcast.com/rudder today.
Your host is Tobias Macey and today I’m interviewing Max Beauchemin about Superset, an open source platform for data exploration, dashboards, and business intelligence
Interview
Introduction
How did you get involved in the area of data management?
Can you start by describing what Superset is?
Superset is becoming part of the reference architecture for a modern data stack. What are the factors that have contributed to its popularity over other tools such as Redash, Metabase, Looker, etc.?
Where do dashboarding and exploration tools like Superset fit in the responsibilities and workflow of a data engineer?
What are some of the challenges that Superset faces in being performant when working with large data sources?
Which data sources have you found to be the most challenging to work with?
What are some anti-patterns that users of Superset might run into when building out a dashboard?
What are some of the ways that users can surface data quality indicators (e.g. freshness, lineage, check results, etc.) in a Superset dashboard?
Another trend in analytics and dashboard tools is providing actionable insights. How can Superset support those use cases where a business user or analyst wants to perform an action based on the data that they are being shown?
How can Superset factor into a data governance strategy for the business?
What are some of the most interesting, innovative, or unexpected ways that you have seen Superset used?
dogfooding
What are the most interesting, unexpected, or challenging lessons that you have learned from working on Superset and founding Preset?
When is Superset the wrong choice?
What do you have planned for the future of Superset and Preset?
Contact Info
LinkedIn
@mistercrunch on Twitter
mistercrunch on GitHub
Parting Question
From your perspective, what is the biggest gap in the tooling or technology for data management today?
Closing Announcements
Thank you for listening! Don’t forget to check out our other show, Podcast.__init__ to learn about the Python language, its community, and the innovative ways it is being used.
Visit the site to subscribe to the show, sign up for the mailing list, and read the show notes.
If you’ve learned something or tried out a project from the show then tell us about it! Email [email protected]) with your story.
To help other people find the show please leave a review on iTunes and tell your friends and co-workers
Join the community in the new Zulip chat workspace at dataengineeringpodcast.com/chat
Links
Superset
Podcast.__init__ Episode
Preset
ASP (Active Server Pages)
VBScript
Data Warehouse Institute
Ralph Kimball
Bill Inmon
Ubisoft
Hadoop
Tableau
Looker
Podcast Episode
The Future of Business Intelligence Is Open Source
Supercharging Apache Superset
Redash
Podcast.__init__ Episode
Metabase
Podcast Episode
The Rise Of The Data Engineer
AirBnB Data University
Python DBAPI
SQLAlchemy
Druid
SQL Common Table Expressions
SQL Window Functions
Data Warehouse Semantic Layer
Amundsen
Podcast Episode
Open Lineage
Datakin
Marquez
Podcast Episode
Apache Arrow
Podcast.__init__ Episode with Wes McKinney
Apache Parquet
DataHub
Podcast Episode
The intro and outro music is from The Hug by The Freak Fandango Orchestra / CC BY-SA
Support Data Engineering Podcast
47:2527/04/2021
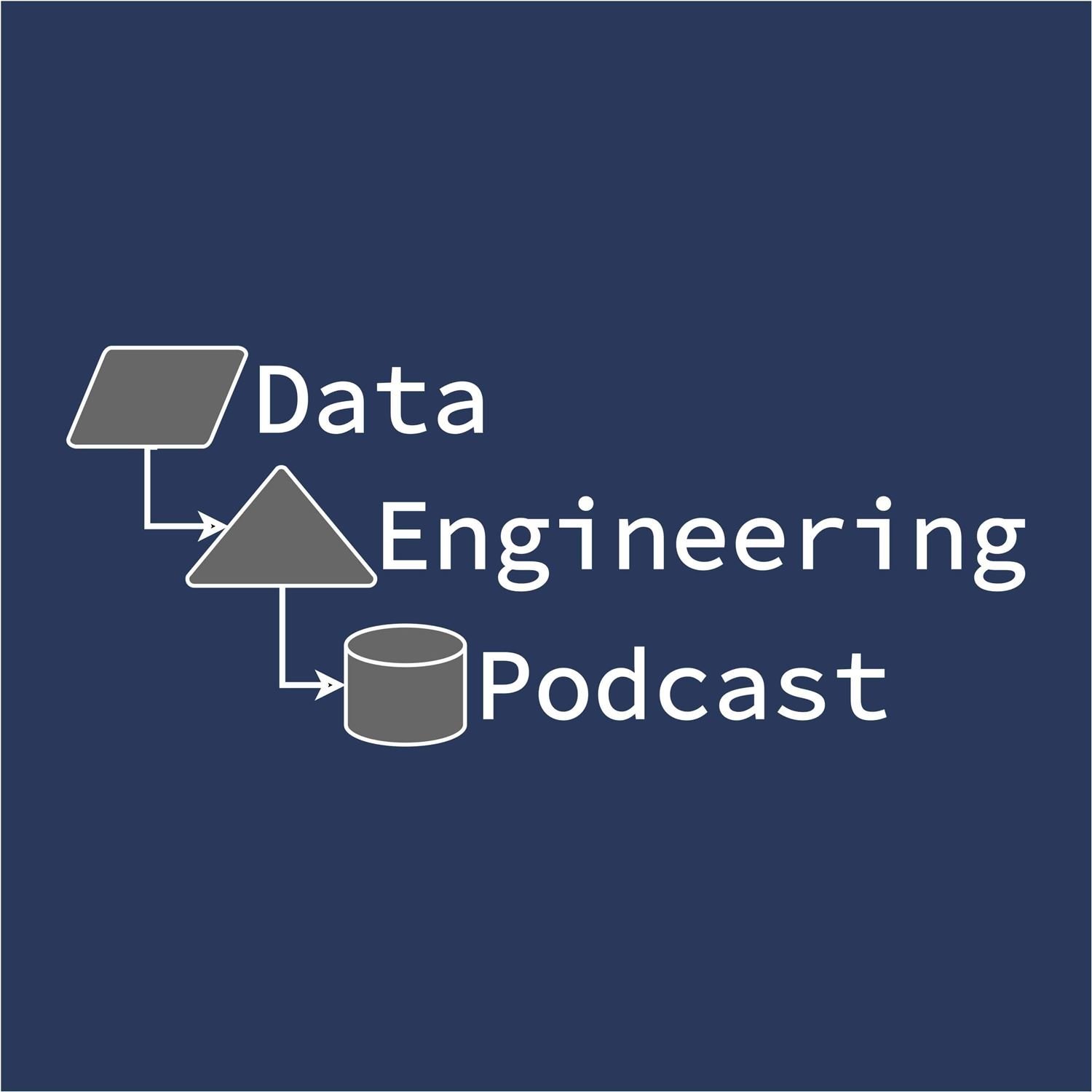
Moving Machine Learning Into The Data Pipeline at Cherre
Summary
Most of the time when you think about a data pipeline or ETL job what comes to mind is a purely mechanistic progression of functions that move data from point A to point B. Sometimes, however, one of those transformations is actually a full-fledged machine learning project in its own right. In this episode Tal Galfsky explains how he and the team at Cherre tackled the problem of messy data for Addresses by building a natural language processing and entity resolution system that is served as an API to the rest of their pipelines. He discusses the myriad ways that addresses are incomplete, poorly formed, and just plain wrong, why it was a big enough pain point to invest in building an industrial strength solution for it, and how it actually works under the hood. After listening to this you’ll look at your data pipelines in a new light and start to wonder how you can bring more advanced strategies into the cleaning and transformation process.
Announcements
Hello and welcome to the Data Engineering Podcast, the show about modern data management
When you’re ready to build your next pipeline, or want to test out the projects you hear about on the show, you’ll need somewhere to deploy it, so check out our friends at Linode. With their managed Kubernetes platform it’s now even easier to deploy and scale your workflows, or try out the latest Helm charts from tools like Pulsar and Pachyderm. With simple pricing, fast networking, object storage, and worldwide data centers, you’ve got everything you need to run a bulletproof data platform. Go to dataengineeringpodcast.com/linode today and get a $100 credit to try out a Kubernetes cluster of your own. And don’t forget to thank them for their continued support of this show!
Modern Data teams are dealing with a lot of complexity in their data pipelines and analytical code. Monitoring data quality, tracing incidents, and testing changes can be daunting and often takes hours to days. Datafold helps Data teams gain visibility and confidence in the quality of their analytical data through data profiling, column-level lineage and intelligent anomaly detection. Datafold also helps automate regression testing of ETL code with its Data Diff feature that instantly shows how a change in ETL or BI code affects the produced data, both on a statistical level and down to individual rows and values. Datafold integrates with all major data warehouses as well as frameworks such as Airflow & dbt and seamlessly plugs into CI workflows. Go to dataengineeringpodcast.com/datafold today to start a 30-day trial of Datafold. Once you sign up and create an alert in Datafold for your company data, they will send you a cool water flask.
RudderStack’s smart customer data pipeline is warehouse-first. It builds your customer data warehouse and your identity graph on your data warehouse, with support for Snowflake, Google BigQuery, Amazon Redshift, and more. Their SDKs and plugins make event streaming easy, and their integrations with cloud applications like Salesforce and ZenDesk help you go beyond event streaming. With RudderStack you can use all of your customer data to answer more difficult questions and then send those insights to your whole customer data stack. Sign up free at dataengineeringpodcast.com/rudder today.
Your host is Tobias Macey and today I’m interviewing Tal Galfsky about how Cherre is bringing order to the messy problem of physical addresses and entity resolution in their data pipelines.
Interview
Introduction
How did you get involved in the area of data management?
Started as physicist and evolved into Data Science
Can you start by giving a brief recap of what Cherre is and the types of data that you deal with?
Cherre is a company that connects data
We’re not a data vendor, in that we don’t sell data, primarily
We help companies connect and make sense of their data
The real estate market is historically closed, gut let, behind on tech
What are the biggest challenges that you deal with in your role when working with real estate data?
Lack of a standard domain model in real estate.
Ontology. What is a property? Each data source, thinks about properties in a very different way. Therefore, yielding similar, but completely different data.
QUALITY (Even if the dataset are talking about the same thing, there are different levels of accuracy, freshness).
HIREARCHY. When is one source better than another
What are the teams and systems that rely on address information?
Any company that needs to clean or organize (make sense) their data, need to identify, people, companies, and properties.
Our clients use Address resolution in multiple ways. Via the UI or via an API. Our service is both external and internal so what I build has to be good enough for the demanding needs of our data science team, robust enough for our engineers, and simple enough that non-expert clients can use it.
Can you give an example for the problems involved in entity resolution
Known entity example.
Empire state buidling.
To resolve addresses in a way that makes sense for the client you need to capture the real world entities. Lots, buildings, units.
Identify the type of the object (lot, building, unit)
Tag the object with all the relevant addresses
Relations to other objects (lot, building, unit)
What are some examples of the kinds of edge cases or messiness that you encounter in addresses?
First class is string problems.
Second class component problems.
third class is geocoding.
I understand that you have developed a service for normalizing addresses and performing entity resolution to provide canonical references for downstream analyses. Can you give an overview of what is involved?
What is the need for the service. The main requirement here is connecting an address to lot, building, unit with latitude and longitude coordinates
How were you satisfying this requirement previously?
Before we built our model and dedicated service we had a basic prototype for pipeline only to handle NYC addresses.
What were the motivations for designing and implementing this as a service?
Need to expand nationwide and to deal with client queries in real time.
What are some of the other data sources that you rely on to be able to perform this normalization and resolution?
Lot data, building data, unit data, Footprints and address points datasets.
What challenges do you face in managing these other sources of information?
Accuracy, hirearchy, standardization, unified solution, persistant ids and primary keys
Digging into the specifics of your solution, can you talk through the full lifecycle of a request to resolve an address and the various manipulations that are performed on it?
String cleaning, Parse and tokenize, standardize, Match
What are some of the other pieces of information in your system that you would like to see addressed in a similar fashion?
Our named entity solution with connection to knowledge graph and owner unmasking.
What are some of the most interesting, unexpected, or challenging lessons that you learned while building this address resolution system?
Scaling nyc geocode example. The NYC model was exploding a subset of the options for messing up an address. Flexibility. Dependencies. Client exposure.
Now that you have this system running in production, if you were to start over today what would you do differently?
a lot but at this point the module boundaries and client interface are defined in such way that we are able to make changes or completely replace any given part of it without breaking anything client facing
What are some of the other projects that you are excited to work on going forward?
Named entity resolution and Knowledge Graph
Contact Info
LinkedIn
Parting Question
From your perspective, what is the biggest gap in the tooling or technology for data management today?
BigQuery is huge asset and in particular UDFs but they don’t support API calls or python script
Closing Announcements
Thank you for listening! Don’t forget to check out our other show, Podcast.__init__ to learn about the Python language, its community, and the innovative ways it is being used.
Visit the site to subscribe to the show, sign up for the mailing list, and read the show notes.
If you’ve learned something or tried out a project from the show then tell us about it! Email [email protected]) with your story.
To help other people find the show please leave a review on iTunes and tell your friends and co-workers
Join the community in the new Zulip chat workspace at dataengineeringpodcast.com/chat
Links
Cherre
Podcast Episode
Photonics
Knowledge Graph
Entity Resolution
BigQuery
NLP == Natural Language Processing
dbt
Podcast Episode
Airflow
Podcast.__init__ Episode
Datadog
Podcast Episode
The intro and outro music is from The Hug by The Freak Fandango Orchestra / CC BY-SA
Support Data Engineering Podcast
48:0520/04/2021
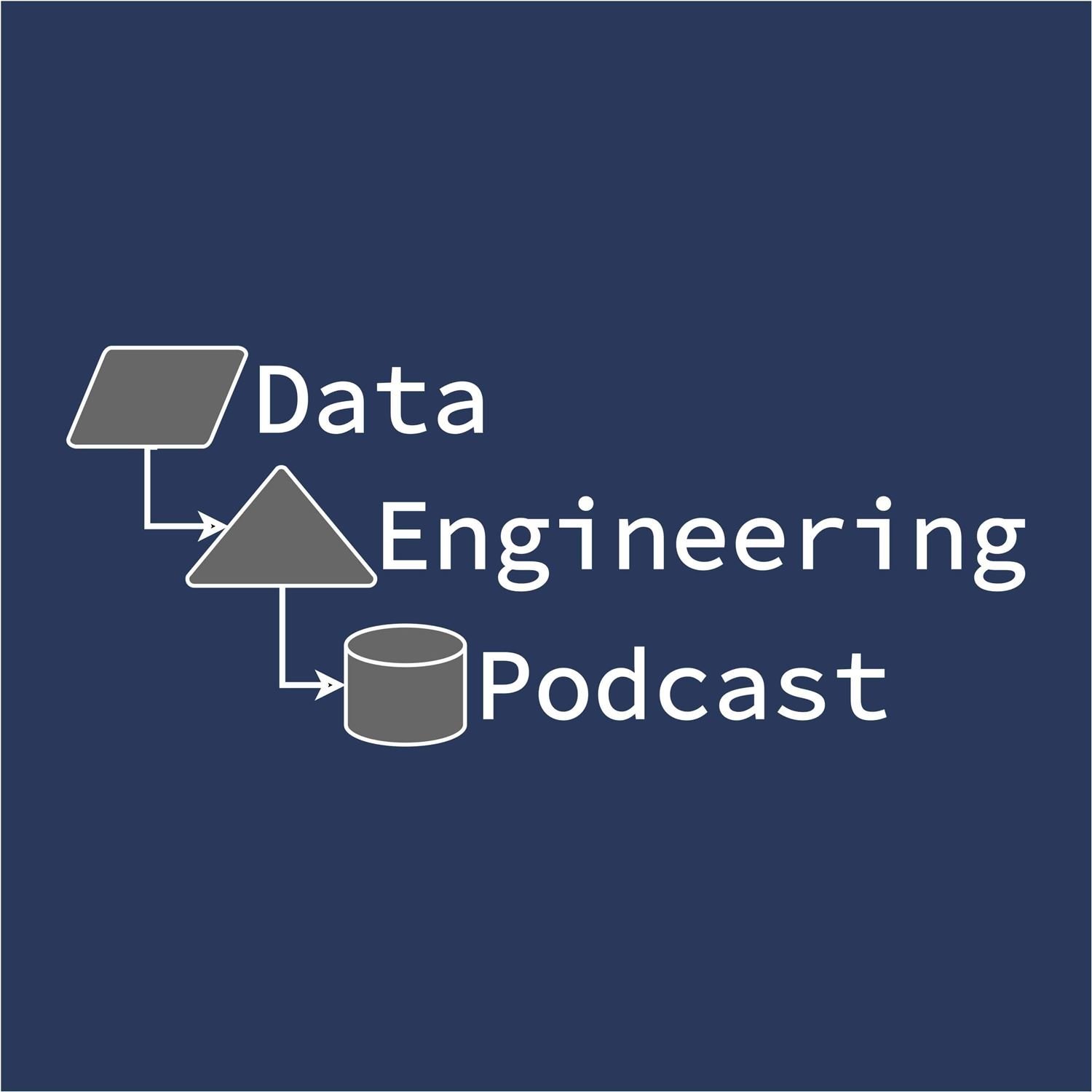
Exploring The Expanding Landscape Of Data Professions with Josh Benamram of Databand
Summary
"Business as usual" is changing, with more companies investing in data as a first class concern. As a result, the data team is growing and introducing more specialized roles. In this episode Josh Benamram, CEO and co-founder of Databand, describes the motivations for these emerging roles, how these positions affect the team dynamics, and the types of visibility that they need into the data platform to do their jobs effectively. He also talks about how his experience working with these teams informs his work at Databand. If you are wondering how to apply your talents and interests to working with data then this episode is a must listen.
Announcements
Hello and welcome to the Data Engineering Podcast, the show about modern data management
When you’re ready to build your next pipeline, or want to test out the projects you hear about on the show, you’ll need somewhere to deploy it, so check out our friends at Linode. With their managed Kubernetes platform it’s now even easier to deploy and scale your workflows, or try out the latest Helm charts from tools like Pulsar and Pachyderm. With simple pricing, fast networking, object storage, and worldwide data centers, you’ve got everything you need to run a bulletproof data platform. Go to dataengineeringpodcast.com/linode today and get a $100 credit to try out a Kubernetes cluster of your own. And don’t forget to thank them for their continued support of this show!
Modern Data teams are dealing with a lot of complexity in their data pipelines and analytical code. Monitoring data quality, tracing incidents, and testing changes can be daunting and often takes hours to days. Datafold helps Data teams gain visibility and confidence in the quality of their analytical data through data profiling, column-level lineage and intelligent anomaly detection. Datafold also helps automate regression testing of ETL code with its Data Diff feature that instantly shows how a change in ETL or BI code affects the produced data, both on a statistical level and down to individual rows and values. Datafold integrates with all major data warehouses as well as frameworks such as Airflow & dbt and seamlessly plugs into CI workflows. Go to dataengineeringpodcast.com/datafold today to start a 30-day trial of Datafold. Once you sign up and create an alert in Datafold for your company data, they will send you a cool water flask.
RudderStack’s smart customer data pipeline is warehouse-first. It builds your customer data warehouse and your identity graph on your data warehouse, with support for Snowflake, Google BigQuery, Amazon Redshift, and more. Their SDKs and plugins make event streaming easy, and their integrations with cloud applications like Salesforce and ZenDesk help you go beyond event streaming. With RudderStack you can use all of your customer data to answer more difficult questions and then send those insights to your whole customer data stack. Sign up free at dataengineeringpodcast.com/rudder today.
Your host is Tobias Macey and today I’m interviewing Josh Benamram about the continued evolution of roles and responsibilities in data teams and their varied requirements for visibility into the data stack
Interview
Introduction
How did you get involved in the area of data management?
Can you start by discussing the set of roles that you see in a majority of data teams?
What new roles do you see emerging, and what are the motivating factors?
Which of the more established positions are fracturing or merging to create these new responsibilities?
What are the contexts in which you are seeing these role definitions used? (e.g. small teams, large orgs, etc.)
How do the increased granularity/specialization of responsibilities across data teams change the ways that data and platform architects need to think about technology investment?
What are the organizational impacts of these new types of data work?
How do these shifts in role definition change the ways that the individuals in the position interact with the data platform?
What are the types of questions that practitioners in different roles are asking of the data that they are working with? (e.g. what is the lineage of this asset vs. what is the distribution of values in this column, etc.)
How can metrics and observability data about pipelines and data systems help to support these various roles?
What are the different ways of measuring data quality for the needs of these roles?
How is the work you are doing at Databand informed by these changing needs?
One of the big challenges caused by data systems is the varying modes of access and interaction across the different stakeholders and activities. How can data platform teams and vendors help to surface useful metrics and information across these various interfaces without forcing users into a new or unfamiliar workflow?
What are some of the long-term impacts that you foresee in the data ecosystem and ways of interacting with data as a result of the current trend toward more specialized tasks?
As a vendor working to provide useful context to these practitioners what are some of the most interesting, unexpected, or challenging lessons that you have learned?
What do you have planned for the future of Databand?
Contact Info
Email
Parting Question
From your perspective, what is the biggest gap in the tooling or technology for data management today?
Closing Announcements
Thank you for listening! Don’t forget to check out our other show, Podcast.__init__ to learn about the Python language, its community, and the innovative ways it is being used.
Visit the site to subscribe to the show, sign up for the mailing list, and read the show notes.
If you’ve learned something or tried out a project from the show then tell us about it! Email [email protected]) with your story.
To help other people find the show please leave a review on iTunes and tell your friends and co-workers
Join the community in the new Zulip chat workspace at dataengineeringpodcast.com/chat
Links
Databand
Website
Platform
Open Core
More data engineering stories & best practices
Atlassian
Chartio
Data Mesh Article
Podcast Episode
Grafana
Metabase
Superset
Podcast.__init__ Episode
Snowflake
Podcast Episode
Spark
Airflow
Podcast.__init__ Episode
The intro and outro music is from The Hug by The Freak Fandango Orchestra / CC BY-SA
Support Data Engineering Podcast
01:08:3613/04/2021
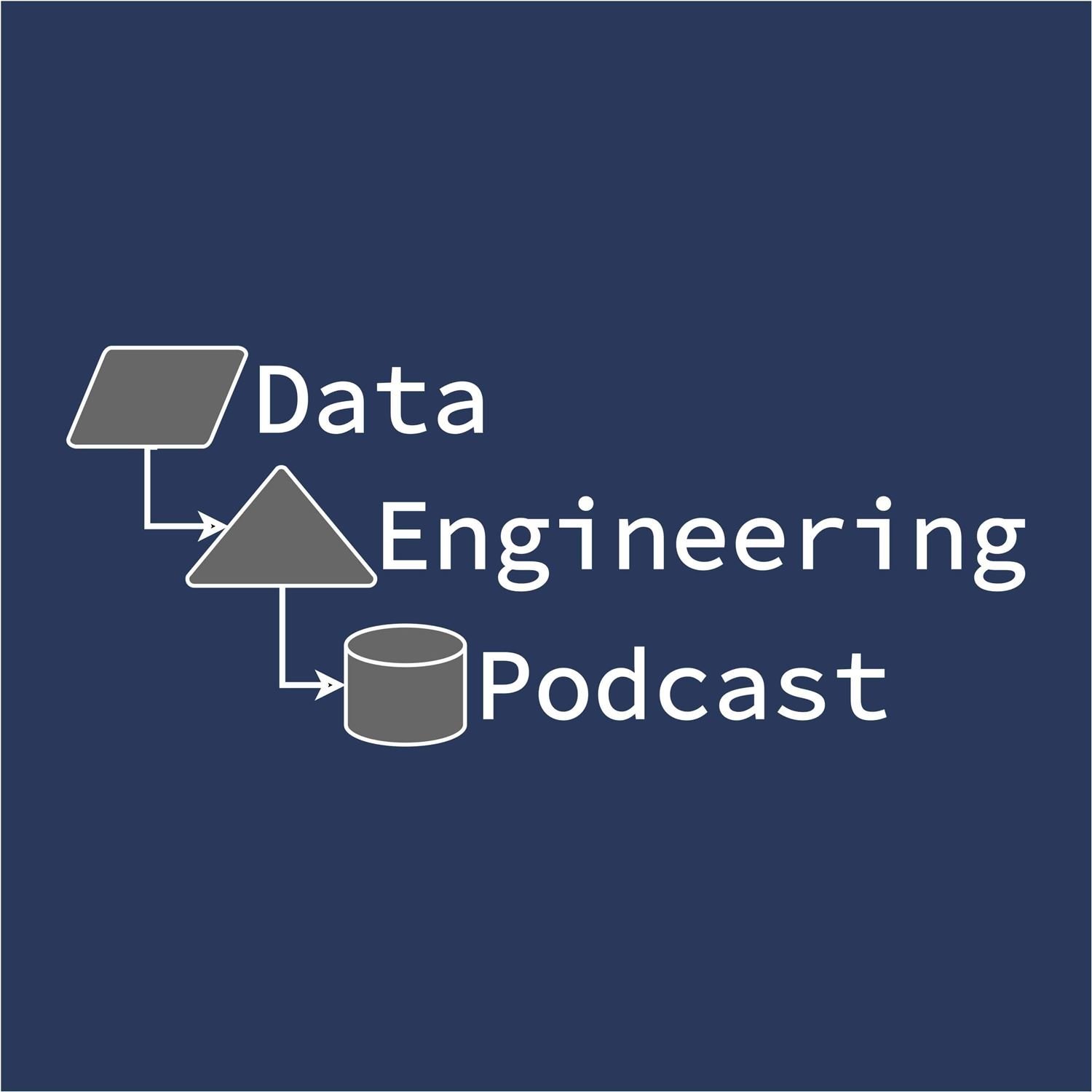
Put Your Whole Data Team On The Same Page With Atlan
Summary
One of the biggest obstacles to success in delivering data products is cross-team collaboration. Part of the problem is the difference in the information that each role requires to do their job and where they expect to find it. This introduces a barrier to communication that is difficult to overcome, particularly in teams that have not reached a significant level of maturity in their data journey. In this episode Prukalpa Sankar shares her experiences across multiple attempts at building a system that brings everyone onto the same page, ultimately bringing her to found Atlan. She explains how the design of the platform is informed by the needs of managing data projects for large and small teams across her previous roles, how it integrates with your existing systems, and how it can work to bring everyone onto the same page.
Announcements
Hello and welcome to the Data Engineering Podcast, the show about modern data management
When you’re ready to build your next pipeline, or want to test out the projects you hear about on the show, you’ll need somewhere to deploy it, so check out our friends at Linode. With their managed Kubernetes platform it’s now even easier to deploy and scale your workflows, or try out the latest Helm charts from tools like Pulsar and Pachyderm. With simple pricing, fast networking, object storage, and worldwide data centers, you’ve got everything you need to run a bulletproof data platform. Go to dataengineeringpodcast.com/linode today and get a $100 credit to try out a Kubernetes cluster of your own. And don’t forget to thank them for their continued support of this show!
Modern Data teams are dealing with a lot of complexity in their data pipelines and analytical code. Monitoring data quality, tracing incidents, and testing changes can be daunting and often takes hours to days. Datafold helps Data teams gain visibility and confidence in the quality of their analytical data through data profiling, column-level lineage and intelligent anomaly detection. Datafold also helps automate regression testing of ETL code with its Data Diff feature that instantly shows how a change in ETL or BI code affects the produced data, both on a statistical level and down to individual rows and values. Datafold integrates with all major data warehouses as well as frameworks such as Airflow & dbt and seamlessly plugs into CI workflows. Go to dataengineeringpodcast.com/datafold today to start a 30-day trial of Datafold. Once you sign up and create an alert in Datafold for your company data, they will send you a cool water flask.
RudderStack’s smart customer data pipeline is warehouse-first. It builds your customer data warehouse and your identity graph on your data warehouse, with support for Snowflake, Google BigQuery, Amazon Redshift, and more. Their SDKs and plugins make event streaming easy, and their integrations with cloud applications like Salesforce and ZenDesk help you go beyond event streaming. With RudderStack you can use all of your customer data to answer more difficult questions and then send those insights to your whole customer data stack. Sign up free at dataengineeringpodcast.com/rudder today.
Your host is Tobias Macey and today I’m interviewing Prukalpa Sankar about Atlan, a modern data workspace that makes collaboration among data stakeholders easier, increasing efficiency and agility in data projects
Interview
Introduction
How did you get involved in the area of data management?
Can you start by giving an overview of what you are building at Atlan and some of the story behind it?
Who are the target users of Atlan?
What portions of the data workflow is Atlan responsible for?
What components of the data stack might Atlan replace?
How would you characterize Atlan’s position in the current data ecosystem?
What makes Atlan stand out from other systems for data cataloguing, metadata management, or data governance?
What types of data assets (e.g. structured vs unstructured, textual vs binary, etc.) is Atlan designed to understand?
Can you talk through how Atlan is implemented?
How have the goals and design of the platform changed or evolved since you first began working on it?
What are some of the early assumptions that you have had to revisit or reconsider?
What is involved in getting Atlan deployed and integrated into an existing data platform?
Beyond the technical aspects, what are the business processes that teams need to implement to be successful when incorporating Atlan into their systems?
Once Atlan is set up, what is a typical workflow for an individual and their team to collaborate on a set of data assets, or building out a new processing pipeline?
What are some useful steps for introducing all of the stakeholders to the system and workflow?
What are the available extension points for managing data in systems that aren’t supported by Atlan out of the box?
What are some of the most interesting, innovative, or unexpected ways that you have seen Atlan used?
What are the most interesting, unexpected, or challenging lessons that you have learned while building Atlan?
When is Atlan the wrong choice?
What do you have planned for the future of the product?
Contact Info
LinkedIn
@prukalpa on Twitter
Parting Question
From your perspective, what is the biggest gap in the tooling or technology for data management today?
Closing Announcements
Thank you for listening! Don’t forget to check out our other show, Podcast.__init__ to learn about the Python language, its community, and the innovative ways it is being used.
Visit the site to subscribe to the show, sign up for the mailing list, and read the show notes.
If you’ve learned something or tried out a project from the show then tell us about it! Email [email protected]) with your story.
To help other people find the show please leave a review on iTunes and tell your friends and co-workers
Join the community in the new Zulip chat workspace at dataengineeringpodcast.com/chat
Links
Atlan
India’s National Data Platform
World Economic Forum
UN
Gates Foundation
GitHub
Figma
Snowflake
Redshift
Databricks
DBT
Sisense
Looker
Apache Atlas
Immuta
DataHub
Datakin
Aapache Ranger
Great Expectations
Trino
Airflow
Dagster
Privacera
Databand
Cloudformation
Grafana
Deequ
We Failed to Set Up a Data Catalog 3x. Here’s Why.
Analysing the analysers book
OpenAPI
The intro and outro music is from The Hug by The Freak Fandango Orchestra / CC BY-SA
Support Data Engineering Podcast
57:3706/04/2021
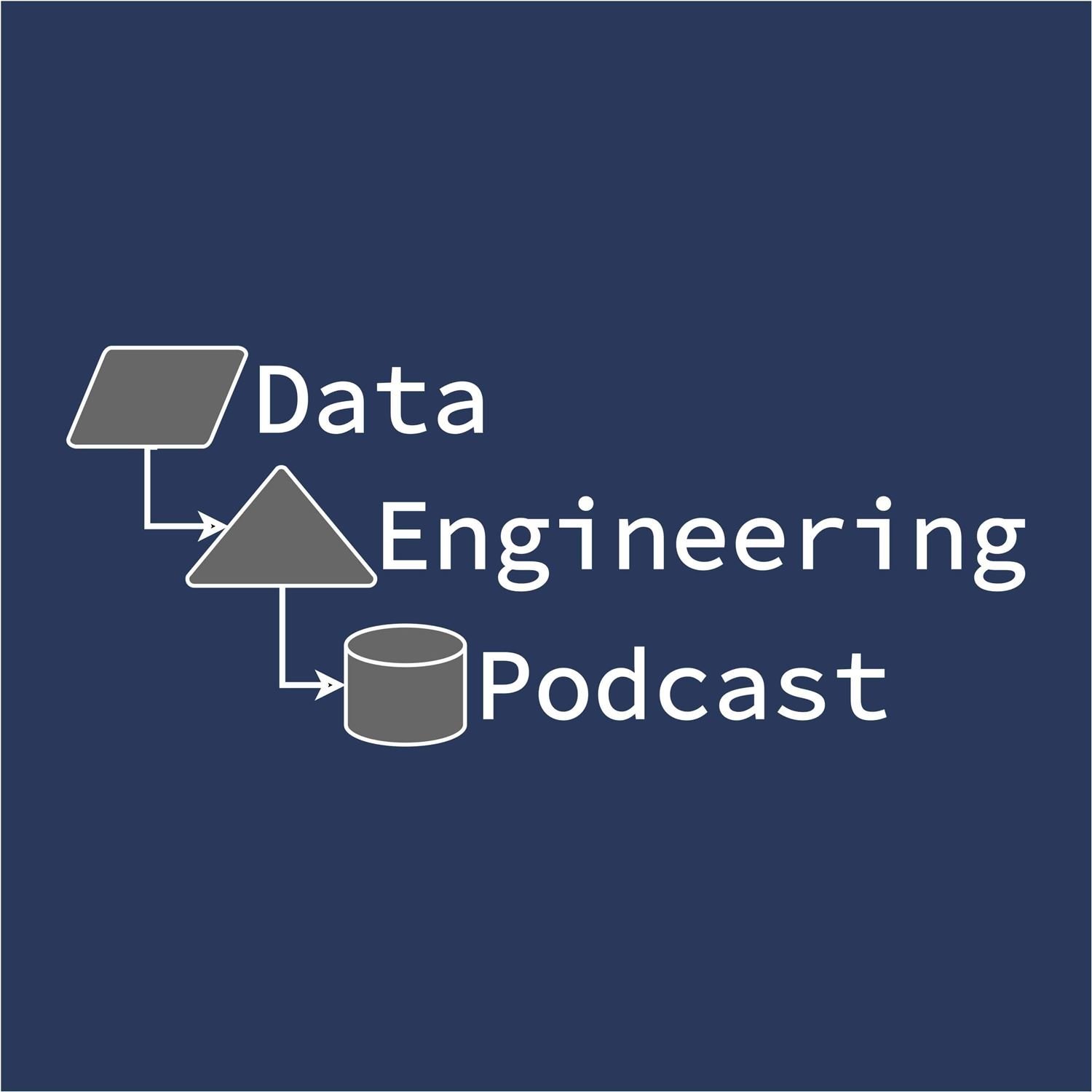
Data Quality Management For The Whole Team With Soda Data
Summary
Data quality is on the top of everyone’s mind recently, but getting it right is as challenging as ever. One of the contributing factors is the number of people who are involved in the process and the potential impact on the business if something goes wrong. In this episode Maarten Masschelein and Tom Baeyens share the work they are doing at Soda to bring everyone on board to make your data clean and reliable. They explain how they started down the path of building a solution for managing data quality, their philosophy of how to empower data engineers with well engineered open source tools that integrate with the rest of the platform, and how to bring all of the stakeholders onto the same page to make your data great. There are many aspects of data quality management and it’s always a treat to learn from people who are dedicating their time and energy to solving it for everyone.
Announcements
Hello and welcome to the Data Engineering Podcast, the show about modern data management
When you’re ready to build your next pipeline, or want to test out the projects you hear about on the show, you’ll need somewhere to deploy it, so check out our friends at Linode. With their managed Kubernetes platform it’s now even easier to deploy and scale your workflows, or try out the latest Helm charts from tools like Pulsar and Pachyderm. With simple pricing, fast networking, object storage, and worldwide data centers, you’ve got everything you need to run a bulletproof data platform. Go to dataengineeringpodcast.com/linode today and get a $100 credit to try out a Kubernetes cluster of your own. And don’t forget to thank them for their continued support of this show!
Modern Data teams are dealing with a lot of complexity in their data pipelines and analytical code. Monitoring data quality, tracing incidents, and testing changes can be daunting and often takes hours to days. Datafold helps Data teams gain visibility and confidence in the quality of their analytical data through data profiling, column-level lineage and intelligent anomaly detection. Datafold also helps automate regression testing of ETL code with its Data Diff feature that instantly shows how a change in ETL or BI code affects the produced data, both on a statistical level and down to individual rows and values. Datafold integrates with all major data warehouses as well as frameworks such as Airflow & dbt and seamlessly plugs into CI workflows. Go to dataengineeringpodcast.com/datafold today to start a 30-day trial of Datafold. Once you sign up and create an alert in Datafold for your company data, they will send you a cool water flask.
RudderStack’s smart customer data pipeline is warehouse-first. It builds your customer data warehouse and your identity graph on your data warehouse, with support for Snowflake, Google BigQuery, Amazon Redshift, and more. Their SDKs and plugins make event streaming easy, and their integrations with cloud applications like Salesforce and ZenDesk help you go beyond event streaming. With RudderStack you can use all of your customer data to answer more difficult questions and then send those insights to your whole customer data stack. Sign up free at dataengineeringpodcast.com/rudder today.
Your host is Tobias Macey and today I’m interviewing Maarten Masschelein and Tom Baeyens about the work are doing at Soda to power data quality management
Interview
Introduction
How did you get involved in the area of data management?
Can you start by giving an overview of what you are building at Soda?
What problem are you trying to solve?
And how are you solving that problem?
What motivated you to start a business focused on data monitoring and data quality?
The data monitoring and broader data quality space is a segment of the industry that is seeing a huge increase in attention recently. Can you share your perspective on the current state of the ecosystem and how your approach compares to other tools and products?
who have you created Soda for (e.g platform engineers, data engineers, data product owners etc) and what is a typical workflow for each of them?
How do you go about integrating Soda into your data infrastructure?
How has the Soda platform been architected?
Why is this architecture important?
How have the goals and design of the system changed or evolved as you worked with early customers and iterated toward your current state?
What are some of the challenges associated with the ongoing monitoring and testing of data?
what are some of the tools or techniques for data testing used in conjunction with Soda?
What are some of the most interesting, innovative, or unexpected ways that you have seen Soda being used?
What are the most interesting, unexpected, or challenging lessons that you have learned while building the technology and business for Soda?
When is Soda the wrong choice?
What do you have planned for the future?
Contact Info
Maarten
LinkedIn
@masscheleinm on Twitter
Tom
LinkedIn
@tombaeyens on Twitter
Parting Question
From your perspective, what is the biggest gap in the tooling or technology for data management today?
Closing Announcements
Thank you for listening! Don’t forget to check out our other show, Podcast.__init__ to learn about the Python language, its community, and the innovative ways it is being used.
Visit the site to subscribe to the show, sign up for the mailing list, and read the show notes.
If you’ve learned something or tried out a project from the show then tell us about it! Email [email protected]) with your story.
To help other people find the show please leave a review on iTunes and tell your friends and co-workers
Join the community in the new Zulip chat workspace at dataengineeringpodcast.com/chat
Links
Soda Data
Soda SQL
RedHat
Collibra
Spark
Getting Things Done by David Allen (affiliate link)
Slack
OpsGenie
DBT
Airflow
The intro and outro music is from The Hug by The Freak Fandango Orchestra / CC BY-SA
Support Data Engineering Podcast
58:0030/03/2021
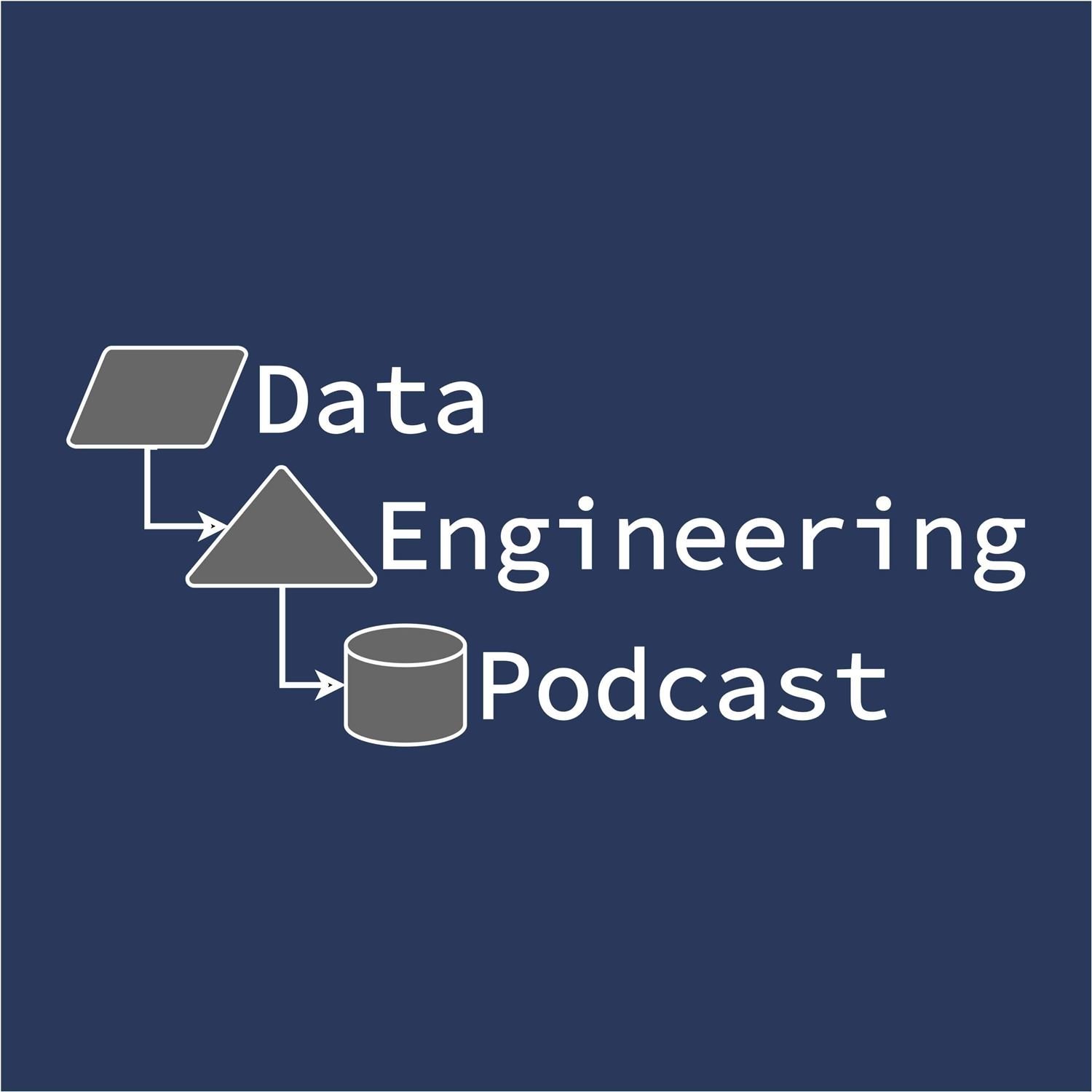
Real World Change Data Capture At Datacoral
Summary
The world of business is becoming increasingly dependent on information that is accurate up to the minute. For analytical systems, the only way to provide this reliably is by implementing change data capture (CDC). Unfortunately, this is a non-trivial undertaking, particularly for teams that don’t have extensive experience working with streaming data and complex distributed systems. In this episode Raghu Murthy, founder and CEO of Datacoral, does a deep dive on how he and his team manage change data capture pipelines in production.
Announcements
Hello and welcome to the Data Engineering Podcast, the show about modern data management
When you’re ready to build your next pipeline, or want to test out the projects you hear about on the show, you’ll need somewhere to deploy it, so check out our friends at Linode. With their managed Kubernetes platform it’s now even easier to deploy and scale your workflows, or try out the latest Helm charts from tools like Pulsar and Pachyderm. With simple pricing, fast networking, object storage, and worldwide data centers, you’ve got everything you need to run a bulletproof data platform. Go to dataengineeringpodcast.com/linode today and get a $100 credit to try out a Kubernetes cluster of your own. And don’t forget to thank them for their continued support of this show!
Modern Data teams are dealing with a lot of complexity in their data pipelines and analytical code. Monitoring data quality, tracing incidents, and testing changes can be daunting and often takes hours to days. Datafold helps Data teams gain visibility and confidence in the quality of their analytical data through data profiling, column-level lineage and intelligent anomaly detection. Datafold also helps automate regression testing of ETL code with its Data Diff feature that instantly shows how a change in ETL or BI code affects the produced data, both on a statistical level and down to individual rows and values. Datafold integrates with all major data warehouses as well as frameworks such as Airflow & dbt and seamlessly plugs into CI workflows. Go to dataengineeringpodcast.com/datafold today to start a 30-day trial of Datafold. Once you sign up and create an alert in Datafold for your company data, they will send you a cool water flask.
RudderStack’s smart customer data pipeline is warehouse-first. It builds your customer data warehouse and your identity graph on your data warehouse, with support for Snowflake, Google BigQuery, Amazon Redshift, and more. Their SDKs and plugins make event streaming easy, and their integrations with cloud applications like Salesforce and ZenDesk help you go beyond event streaming. With RudderStack you can use all of your customer data to answer more difficult questions and then send those insights to your whole customer data stack. Sign up free at dataengineeringpodcast.com/rudder today.
Your host is Tobias Macey and today I’m interviewing Raghu Murthy about his recent work of making change data capture more accessible and maintainable
Interview
Introduction
How did you get involved in the area of data management?
Can you start by giving an overview of what CDC is and when it is useful?
What are the alternatives to CDC?
What are the cases where a more batch-oriented approach would be preferable?
What are the factors that you need to consider when deciding whether to implement a CDC system for a given data integration?
What are the barriers to entry?
What are some of the common mistakes or misconceptions about CDC that you have encountered in your own work and while working with customers?
How does CDC fit into a broader data platform, particularly where there are likely to be other data integration pipelines in operation? (e.g. Fivetran/Airbyte/Meltano/custom scripts)
What are the moving pieces in a CDC workflow that need to be considered as you are designing the system?
What are some examples of the configuration changes necessary in source systems to provide the needed log data?
How would you characterize the current landscape of tools available off the shelf for building a CDC pipeline?
What are your predictions about the potential for a unified abstraction layer for log-based CDC across databases?
What are some of the potential performance/uptime impacts on source databases, both during the initial historical sync and once you hit a steady state?
How can you mitigate the impacts of the CDC pipeline on the source databases?
What are some of the implementation details that application developers DBAs need to be aware of for data modeling in the source systems to allow for proper replication via CDC?
Are there any performance challenges that need to be addressed in the consumers or destination systems? e.g. parallelism
Can you describe the technical implementation and architecture that you use for implementing CDC?
How has the design evolved as you have grown the scale and sophistication of your system?
In the destination system, what data modeling decisions need to be made to ensure that the replicated information is usable for anlytics?
What additional attributes need to be added to track things like row modifications, deletions, schema changes, etc.?
How do you approach treatment of data copies in the DWH? (e.g. ELT – keep all source tables and use DBT for converting relevant tables into star/snowflake/data vault/wide tables)
What are your thoughts on the viability of a data lake as the destination system? (e.g. S3/Parquet or Trino/Drill/etc.)
CDC is a topic that is generally reserved for coversations about databases, but what are some of the other systems that we could think about implementing CDC? e.g. APIs and third party data sources
How can we integrage CDC into metadata/lineage tooling?
How do you handle observability of CDC flows?
What is involved in debugging a replication flow?
How can we build data quality checks into CDC workflows?
What are some of the most interesting, innovative, or unexpected ways that you have seen CDC used?
What are the most interesting, unexpected, or challenging lessons that you have learned from digging deep into CDC implementation?
When is CDC the wrong choice?
What are some of the industry or technology trends around CDC that you are most excited by?
Contact Info
LinkedIn
Parting Question
From your perspective, what is the biggest gap in the tooling or technology for data management today?
Closing Announcements
Thank you for listening! Don’t forget to check out our other show, Podcast.__init__ to learn about the Python language, its community, and the innovative ways it is being used.
Visit the site to subscribe to the show, sign up for the mailing list, and read the show notes.
If you’ve learned something or tried out a project from the show then tell us about it! Email [email protected]) with your story.
To help other people find the show please leave a review on iTunes and tell your friends and co-workers
Join the community in the new Zulip chat workspace at dataengineeringpodcast.com/chat
Links
DataCoral
Podcast Episode
DataCoral Blog
3 Steps To Build A Modern Data Stack
Change Data Capture: Overview
Hive
Hadoop
DBT
Podcast Episode
FiveTran
Podcast Episode
Change Data Capture
Metadata First Blog Post
Debezium
Podcast Episode
UUID == Universally Unique Identifier
Airflow
Oracle Goldengate
Parquet
Trino
AWS Lambda
Data Mesh
Podcast Episode
Enterprise Message Bus
The intro and outro music is from The Hug by The Freak Fandango Orchestra / CC BY-SA
Support Data Engineering Podcast
49:5823/03/2021
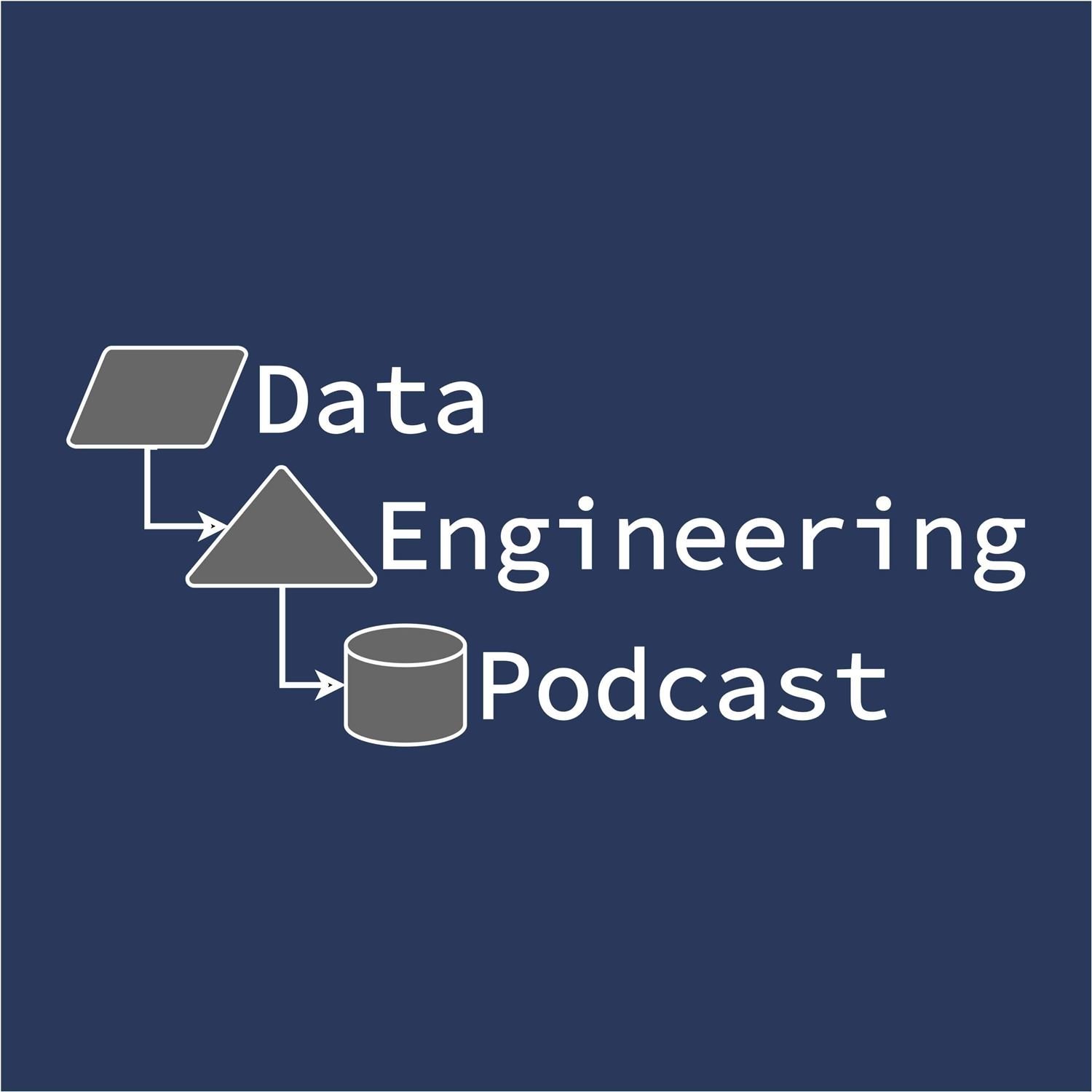
Managing The DoorDash Data Platform
Summary
The team at DoorDash has a complex set of optimization challenges to deal with using data that they collect from a multi-sided marketplace. In order to handle the volume and variety of information that they use to run and improve the business the data team has to build a platform that analysts and data scientists can use in a self-service manner. In this episode the head of data platform for DoorDash, Sudhir Tonse, discusses the technologies that they are using, the approach that they take to adding new systems, and how they think about priorities for what to support for the whole company vs what to leave as a specialized concern for a single team. This is a valuable look at how to manage a large and growing data platform with that supports a variety of teams with varied and evolving needs.
Announcements
Hello and welcome to the Data Engineering Podcast, the show about modern data management
When you’re ready to build your next pipeline, or want to test out the projects you hear about on the show, you’ll need somewhere to deploy it, so check out our friends at Linode. With their managed Kubernetes platform it’s now even easier to deploy and scale your workflows, or try out the latest Helm charts from tools like Pulsar and Pachyderm. With simple pricing, fast networking, object storage, and worldwide data centers, you’ve got everything you need to run a bulletproof data platform. Go to dataengineeringpodcast.com/linode today and get a $100 credit to try out a Kubernetes cluster of your own. And don’t forget to thank them for their continued support of this show!
Modern Data teams are dealing with a lot of complexity in their data pipelines and analytical code. Monitoring data quality, tracing incidents, and testing changes can be daunting and often takes hours to days. Datafold helps Data teams gain visibility and confidence in the quality of their analytical data through data profiling, column-level lineage and intelligent anomaly detection. Datafold also helps automate regression testing of ETL code with its Data Diff feature that instantly shows how a change in ETL or BI code affects the produced data, both on a statistical level and down to individual rows and values. Datafold integrates with all major data warehouses as well as frameworks such as Airflow & dbt and seamlessly plugs into CI workflows. Go to dataengineeringpodcast.com/datafold today to start a 30-day trial of Datafold. Once you sign up and create an alert in Datafold for your company data, they will send you a cool water flask.
RudderStack’s smart customer data pipeline is warehouse-first. It builds your customer data warehouse and your identity graph on your data warehouse, with support for Snowflake, Google BigQuery, Amazon Redshift, and more. Their SDKs and plugins make event streaming easy, and their integrations with cloud applications like Salesforce and ZenDesk help you go beyond event streaming. With RudderStack you can use all of your customer data to answer more difficult questions and then send those insights to your whole customer data stack. Sign up free at dataengineeringpodcast.com/rudder today.
Your host is Tobias Macey and today I’m interviewing Sudhir Tonse about how the team at DoorDash designed their data platform
Interview
Introduction
How did you get involved in the area of data management?
Can you start by giving a quick overview of what you do at DoorDash?
What are some of the ways that data is used to power the business?
How has the pandemic affected the scale and volatility of the data that you are working with?
Can you describe the type(s) of data that you are working with?
What are the primary sources of data that you collect?
What secondary or third party sources of information do you rely on?
Can you give an overview of the collection process for that data?
In selecting the technologies for the various components in your data stack, what are the primary factors that you consider when evaluating the build vs. buy decision?
In your recent post about how you are scaling the capabilities and capacity of your data platform you mentioned the concept of maintaining a "paved path" of supported technologies to simplify integration across teams. What are the technologies that you use and rely on for the "paved path"?
How are you managing quality and consistency of your data across its lifecycle?
What are some of the specific data quality solutions that you have integrated into the platform and "paved path"?
What are some of the technologies that were used early on at DoorDash that failed to keep up as the business scaled?
How do you manage the migration path for adopting new technologies or techniques?
In the same post you mentioned the tendency to allow for building point solutions before deciding whether to generalize a given use case into a generalized platform capability. Can you give some examples of cases where a point solution remains a one-off versus when it needs to be expanded into a widely used component?
How do you identify and tracking cost factors in the data platform?
What do you do with that information?
What is your approach for identifying and measuring useful OKRs (Objectives and Key Results)?
How do you quantify potentially subjective metrics such as reliability and quality?
How have you designed the organizational structure for your data teams?
What are the responsibilities and organizational interfaces for data engineers within the company?
How have the organizational structures/patterns shifted or changed at different levels of scale/maturity for the business?
What are some of the most interesting, useful, unexpected, or challenging lessons that you have learned during your time as a data professional at DoorDash?
What are some of the upcoming projects or changes that you anticipate in the near to medium future?
Contact Info
LinkedIn
@stonse on Twitter
Parting Question
From your perspective, what is the biggest gap in the tooling or technology for data management today?
Closing Announcements
Thank you for listening! Don’t forget to check out our other show, Podcast.__init__ to learn about the Python language, its community, and the innovative ways it is being used.
Visit the site to subscribe to the show, sign up for the mailing list, and read the show notes.
If you’ve learned something or tried out a project from the show then tell us about it! Email [email protected]) with your story.
To help other people find the show please leave a review on iTunes and tell your friends and co-workers
Join the community in the new Zulip chat workspace at dataengineeringpodcast.com/chat
Links
How DoorDash is Scaling its Data Platform to Delight Customers and Meet our Growing Demand
DoorDash
Uber
Netscape
Netflix
Change Data Capture
Debezium
Podcast Episode
SnowflakeDB
Podcast Episode
Airflow
Podcast.__init__ Episode
Kafka
Flink
Podcast Episode
Pinot
GDPR
CCPA
Data Governance
AWS
LightGBM
XGBoost
Big Data Landscape
Kinesis
Kafka Connect
Cassandra
PostgreSQL
Podcast Episode
Amundsen
Podcast Episode
SQS
Feature Toggles
BigEye
Podcast Episode
The intro and outro music is from The Hug by The Freak Fandango Orchestra / CC BY-SA
Support Data Engineering Podcast
46:0516/03/2021
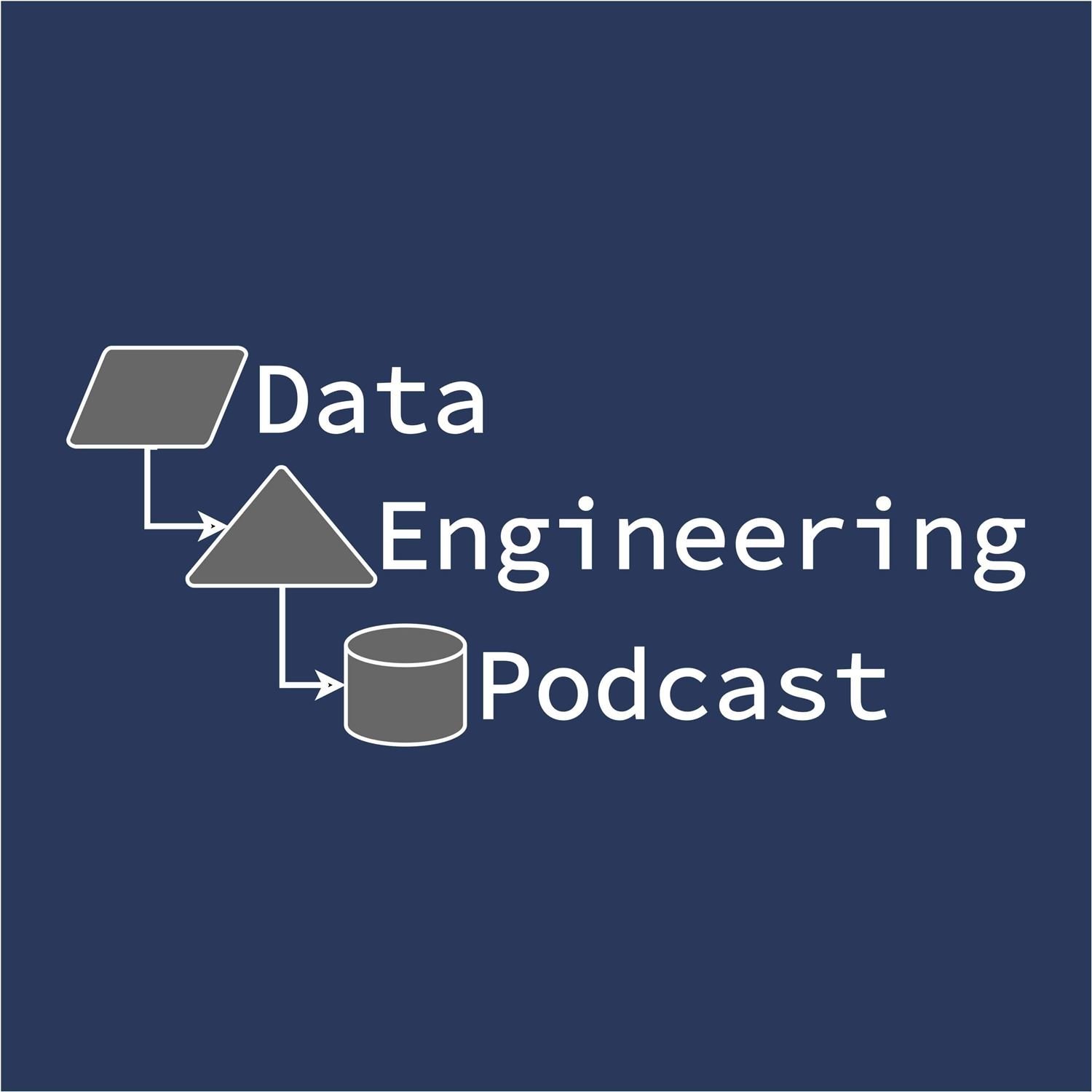
Leave Your Data Where It Is And Automate Feature Extraction With Molecula
Summary
A majority of the time spent in data engineering is copying data between systems to make the information available for different purposes. This introduces challenges such as keeping information synchronized, managing schema evolution, building transformations to match the expectations of the destination systems. H.O. Maycotte was faced with these same challenges but at a massive scale, leading him to question if there is a better way. After tasking some of his top engineers to consider the problem in a new light they created the Pilosa engine. In this episode H.O. explains how using Pilosa as the core he built the Molecula platform to eliminate the need to copy data between systems in able to make it accessible for analytical and machine learning purposes. He also discusses the challenges that he faces in helping potential users and customers understand the shift in thinking that this creates, and how the system is architected to make it possible. This is a fascinating conversation about what the future looks like when you revisit your assumptions about how systems are designed.
Announcements
Hello and welcome to the Data Engineering Podcast, the show about modern data management
When you’re ready to build your next pipeline, or want to test out the projects you hear about on the show, you’ll need somewhere to deploy it, so check out our friends at Linode. With their managed Kubernetes platform it’s now even easier to deploy and scale your workflows, or try out the latest Helm charts from tools like Pulsar and Pachyderm. With simple pricing, fast networking, object storage, and worldwide data centers, you’ve got everything you need to run a bulletproof data platform. Go to dataengineeringpodcast.com/linode today and get a $100 credit to try out a Kubernetes cluster of your own. And don’t forget to thank them for their continued support of this show!
Modern Data teams are dealing with a lot of complexity in their data pipelines and analytical code. Monitoring data quality, tracing incidents, and testing changes can be daunting and often takes hours to days. Datafold helps Data teams gain visibility and confidence in the quality of their analytical data through data profiling, column-level lineage and intelligent anomaly detection. Datafold also helps automate regression testing of ETL code with its Data Diff feature that instantly shows how a change in ETL or BI code affects the produced data, both on a statistical level and down to individual rows and values. Datafold integrates with all major data warehouses as well as frameworks such as Airflow & dbt and seamlessly plugs into CI workflows. Go to dataengineeringpodcast.com/datafold today to start a 30-day trial of Datafold. Once you sign up and create an alert in Datafold for your company data, they will send you a cool water flask.
RudderStack’s smart customer data pipeline is warehouse-first. It builds your customer data warehouse and your identity graph on your data warehouse, with support for Snowflake, Google BigQuery, Amazon Redshift, and more. Their SDKs and plugins make event streaming easy, and their integrations with cloud applications like Salesforce and ZenDesk help you go beyond event streaming. With RudderStack you can use all of your customer data to answer more difficult questions and then send those insights to your whole customer data stack. Sign up free at dataengineeringpodcast.com/rudder today.
Your host is Tobias Macey and today I’m interviewing H.O. Maycotte about Molecula, a cloud based feature store based on the open source Pilosa project
Interview
Introduction
How did you get involved in the area of data management?
Can you start by giving an overview of what you are building at Molecula and the story behind it?
What are the additional capabilities that Molecula offers on top of the open source Pilosa project?
What are the problems/use cases that Molecula solves for?
What are some of the technologies or architectural patterns that Molecula might replace in a companies data platform?
One of the use cases that is mentioned on the Molecula site is as a feature store for ML and AI. This is a category that has been seeing a lot of growth recently. Can you provide some context how Molecula fits in that market and how it compares to options such as Tecton, Iguazio, Feast, etc.?
What are the benefits of using a bitmap index for identifying and computing features?
Can you describe how the Molecula platform is architected?
How has the design and goal of Molecula changed or evolved since you first began working on it?
For someone who is using Molecula, can you describe the process of integrating it with their existing data sources?
Can you describe the internal data model of Pilosa/Molecula?
How should users think about data modeling and architecture as they are loading information into the platform?
Once a user has data in Pilosa, what are the available mechanisms for performing analyses or feature engineering?
What are some of the most underutilized or misunderstood capabilities of Molecula?
What are some of the most interesting, unexpected, or innovative ways that you have seen the Molecula platform used?
What are the most interesting, unexpected, or challenging lessons that you have learned from building and scaling Molecula?
When is Molecula the wrong choice?
What do you have planned for the future of the platform and business?
Contact Info
LinkedIn
@maycotte on Twitter
Parting Question
From your perspective, what is the biggest gap in the tooling or technology for data management today?
Closing Announcements
Thank you for listening! Don’t forget to check out our other show, Podcast.__init__ to learn about the Python language, its community, and the innovative ways it is being used.
Visit the site to subscribe to the show, sign up for the mailing list, and read the show notes.
If you’ve learned something or tried out a project from the show then tell us about it! Email [email protected]) with your story.
To help other people find the show please leave a review on iTunes and tell your friends and co-workers
Join the community in the new Zulip chat workspace at dataengineeringpodcast.com/chat
Links
Molecula
Pilosa
Podcast Episode
The Social Dilemma
Feature Store
Cassandra
Elasticsearch
Podcast Episode
Druid
MongoDB
SwimOS
Podcast Episode
Kafka
Kafka Schema Registry
Podcast Episode
Homomorphic Encryption
Lucene
Solr
The intro and outro music is from The Hug by The Freak Fandango Orchestra / CC BY-SA
Support Data Engineering Podcast
51:4009/03/2021
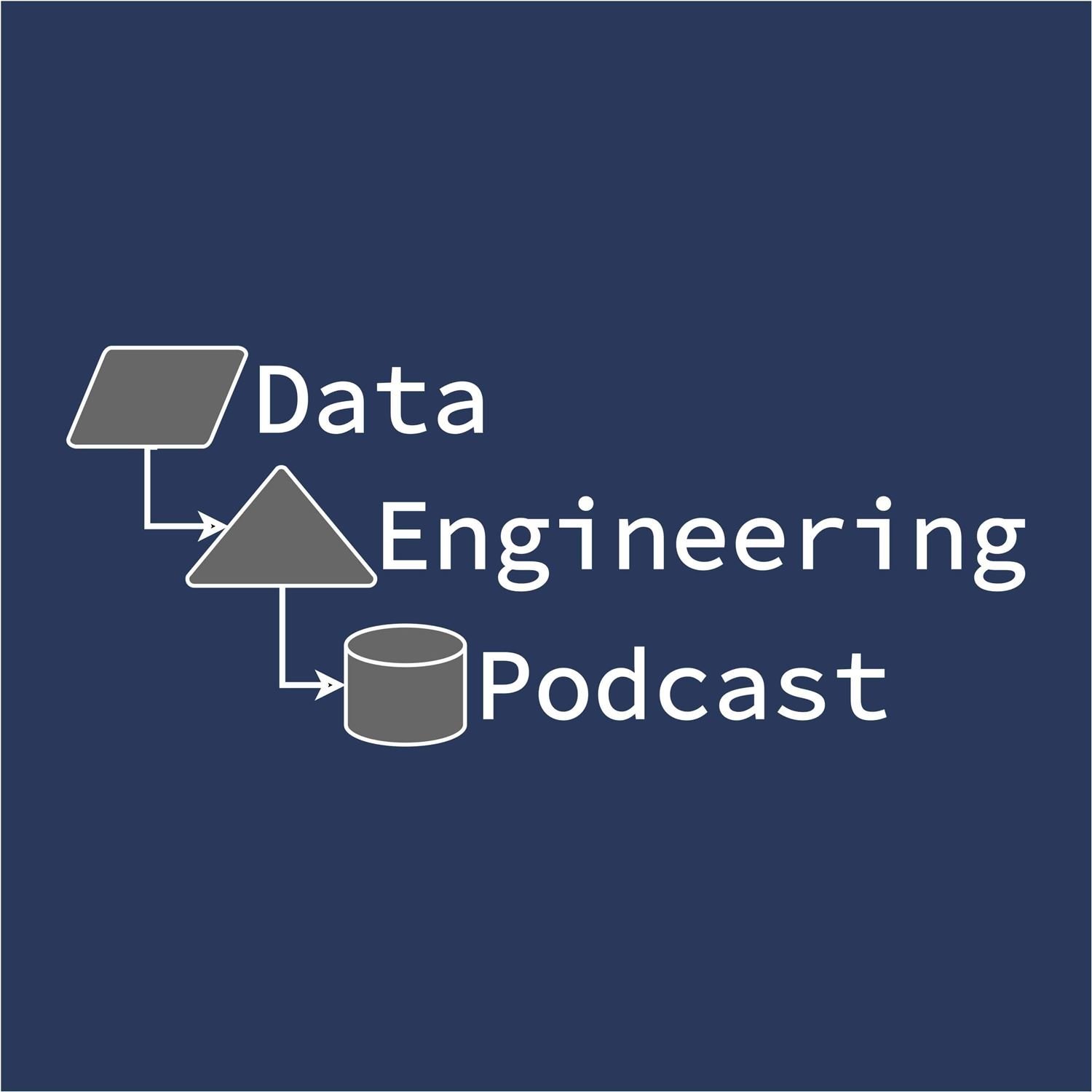
Bridging The Gap Between Machine Learning And Operations At Iguazio
Summary
The process of building and deploying machine learning projects requires a staggering number of systems and stakeholders to work in concert. In this episode Yaron Haviv, co-founder of Iguazio, discusses the complexities inherent to the process, as well as how he has worked to democratize the technologies necessary to make machine learning operations maintainable.
Announcements
Hello and welcome to the Data Engineering Podcast, the show about modern data management
When you’re ready to build your next pipeline, or want to test out the projects you hear about on the show, you’ll need somewhere to deploy it, so check out our friends at Linode. With their managed Kubernetes platform it’s now even easier to deploy and scale your workflows, or try out the latest Helm charts from tools like Pulsar and Pachyderm. With simple pricing, fast networking, object storage, and worldwide data centers, you’ve got everything you need to run a bulletproof data platform. Go to dataengineeringpodcast.com/linode today and get a $100 credit to try out a Kubernetes cluster of your own. And don’t forget to thank them for their continued support of this show!
Modern Data teams are dealing with a lot of complexity in their data pipelines and analytical code. Monitoring data quality, tracing incidents, and testing changes can be daunting and often takes hours to days. Datafold helps Data teams gain visibility and confidence in the quality of their analytical data through data profiling, column-level lineage and intelligent anomaly detection. Datafold also helps automate regression testing of ETL code with its Data Diff feature that instantly shows how a change in ETL or BI code affects the produced data, both on a statistical level and down to individual rows and values. Datafold integrates with all major data warehouses as well as frameworks such as Airflow & dbt and seamlessly plugs into CI workflows. Go to dataengineeringpodcast.com/datafold today to start a 30-day trial of Datafold. Once you sign up and create an alert in Datafold for your company data, they will send you a cool water flask.
RudderStack’s smart customer data pipeline is warehouse-first. It builds your customer data warehouse and your identity graph on your data warehouse, with support for Snowflake, Google BigQuery, Amazon Redshift, and more. Their SDKs and plugins make event streaming easy, and their integrations with cloud applications like Salesforce and ZenDesk help you go beyond event streaming. With RudderStack you can use all of your customer data to answer more difficult questions and then send those insights to your whole customer data stack. Sign up free at dataengineeringpodcast.com/rudder today.
Your host is Tobias Macey and today I’m interviewing Yaron Haviv about Iguazio, a platform for end to end automation of machine learning applications using MLOps principles.
Interview
Introduction
How did you get involved in the area of data science & analytics?
Can you start by giving an overview of what Iguazio is and the story of how it got started?
How would you characterize your target or typical customer?
What are the biggest challenges that you see around building production grade workflows for machine learning?
How does Iguazio help to address those complexities?
For customers who have already invested in the technical and organizational capacity for data science and data engineering, how does Iguazio integrate with their environments?
What are the responsibilities of a data engineer throughout the different stages of the lifecycle for a machine learning application?
Can you describe how the Iguazio platform is architected?
How has the design of the platform evolved since you first began working on it?
How have the industry best practices around bringing machine learning to production changed?
How do you approach testing/validation of machine learning applications and releasing them to production environments? (e.g. CI/CD)
Once a model is in production, what are the types and sources of information that you collect to monitor their performance?
What are the factors that contribute to model drift?
What are the remaining gaps in the tooling or processes available for managing the lifecycle of machine learning projects?
What are the most interesting, innovative, or unexpected ways that you have seen the Iguazio platform used?
What are the most interesting, unexpected, or challenging lessons that you have learned while building and scaling the Iguazio platform and business?
When is Iguazio the wrong choice?
What do you have planned for the future of the platform?
Contact Info
LinkedIn
@yaronhaviv on Twitter
Parting Question
From your perspective, what is the biggest gap in the tooling or technology for data management today?
Links
Iguazio
MLOps
Oracle Exadata
SAP HANA
Mellanox
NVIDIA
Multi-Model Database
Nuclio
MLRun
Jupyter Notebook
Pandas
Scala
Feature Imputing
Feature Store
Parquet
Spark
Apache Flink
Podcast Episode
Apache Beam
NLP (Natural Language Processing)
Deep Learning
BERT
Airflow
Podcast.__init__ Episode
Dagster
Data Engineering Podcast Episode
Podcast.__init__ Episode
Kubeflow
Argo
AWS Step Functions
Presto/Trino
Podcast Episode
Dask
Podcast Episode
Hadoop
Sagemaker
Tecton
Podcast Episode
Seldon
DataRobot
RapidMiner
H2O.ai
Grafana
Storey
The intro and outro music is from The Hug by The Freak Fandango Orchestra / CC BY-SA
Support Data Engineering Podcast
01:06:2802/03/2021
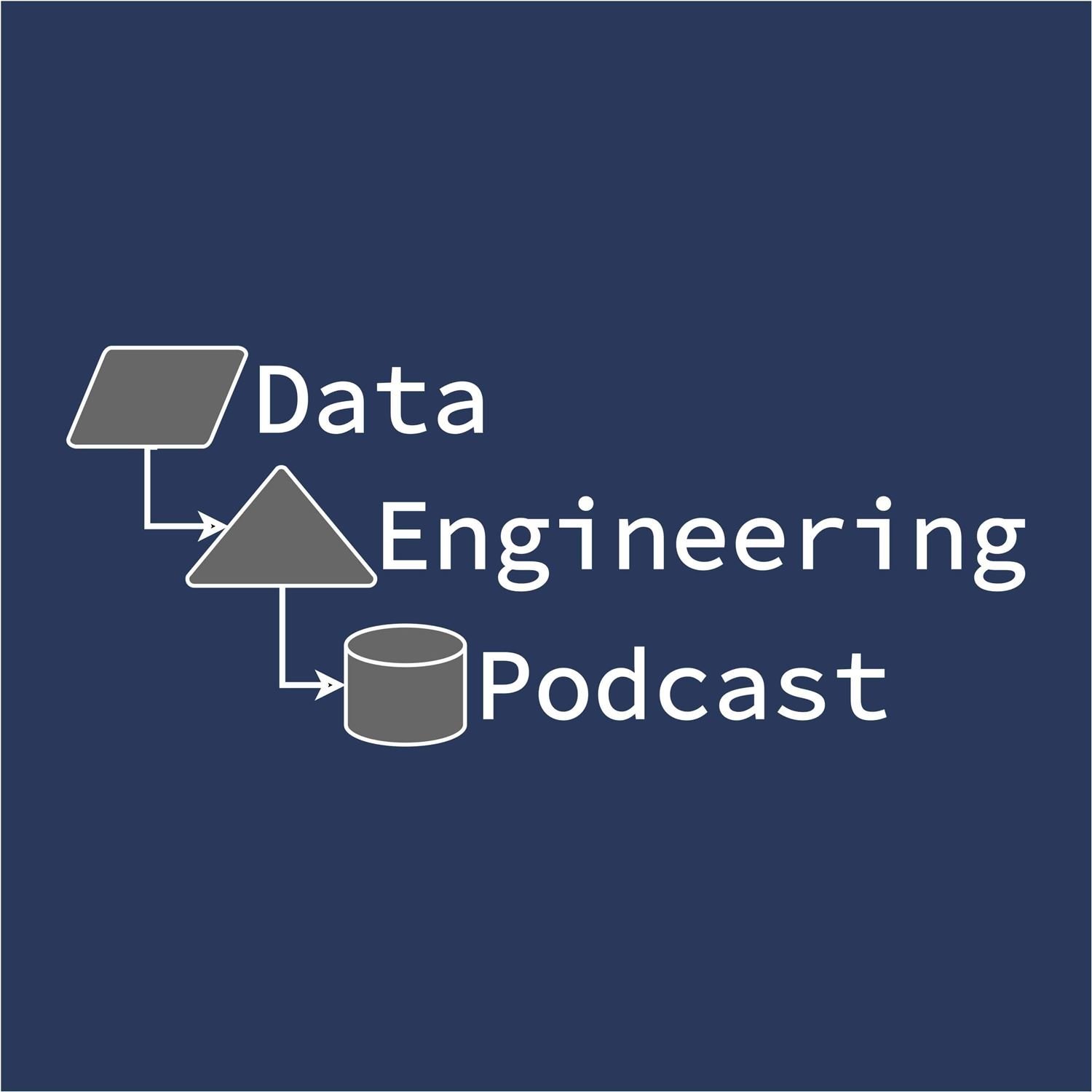
Self Service Open Source Data Integration With AirByte
Summary
Data integration is a critical piece of every data pipeline, yet it is still far from being a solved problem. There are a number of managed platforms available, but the list of options for an open source system that supports a large variety of sources and destinations is still embarrasingly short. The team at Airbyte is adding a new entry to that list with the goal of making robust and easy to use data integration more accessible to teams who want or need to maintain full control of their data. In this episode co-founders John Lafleur and Michel Tricot share the story of how and why they created Airbyte, discuss the project’s design and architecture, and explain their vision of what an open soure data integration platform should offer. If you are struggling to maintain your extract and load pipelines or spending time on integrating with a new system when you would prefer to be working on other projects then this is definitely a conversation worth listening to.
Announcements
Hello and welcome to the Data Engineering Podcast, the show about modern data management
When you’re ready to build your next pipeline, or want to test out the projects you hear about on the show, you’ll need somewhere to deploy it, so check out our friends at Linode. With their managed Kubernetes platform it’s now even easier to deploy and scale your workflows, or try out the latest Helm charts from tools like Pulsar and Pachyderm. With simple pricing, fast networking, object storage, and worldwide data centers, you’ve got everything you need to run a bulletproof data platform. Go to dataengineeringpodcast.com/linode today and get a $100 credit to try out a Kubernetes cluster of your own. And don’t forget to thank them for their continued support of this show!
Modern Data teams are dealing with a lot of complexity in their data pipelines and analytical code. Monitoring data quality, tracing incidents, and testing changes can be daunting and often takes hours to days. Datafold helps Data teams gain visibility and confidence in the quality of their analytical data through data profiling, column-level lineage and intelligent anomaly detection. Datafold also helps automate regression testing of ETL code with its Data Diff feature that instantly shows how a change in ETL or BI code affects the produced data, both on a statistical level and down to individual rows and values. Datafold integrates with all major data warehouses as well as frameworks such as Airflow & dbt and seamlessly plugs into CI workflows. Go to dataengineeringpodcast.com/datafold today to start a 30-day trial of Datafold. Once you sign up and create an alert in Datafold for your company data, they will send you a cool water flask.
RudderStack’s smart customer data pipeline is warehouse-first. It builds your customer data warehouse and your identity graph on your data warehouse, with support for Snowflake, Google BigQuery, Amazon Redshift, and more. Their SDKs and plugins make event streaming easy, and their integrations with cloud applications like Salesforce and ZenDesk help you go beyond event streaming. With RudderStack you can use all of your customer data to answer more difficult questions and then send those insights to your whole customer data stack. Sign up free at dataengineeringpodcast.com/rudder today.
Your host is Tobias Macey and today I’m interviewing Michel Tricot and John Lafleur about Airbyte, an open source framework for building data integration pipelines.
Interview
Introduction
How did you get involved in the area of data management?
Can you start by explaining what Airbyte is and the story behind it?
Businesses and data engineers have a variety of options for how to manage their data integration. How would you characterize the overall landscape and how does Airbyte distinguish itself in that space?
How would you characterize your target users?
How have those personas instructed the priorities and design of Airbyte?
What do you see as the benefits and tradeoffs of a UI oriented data integration platform as compared to a code first approach?
what are the complex/challenging elements of data integration that makes it such a slippery problem?
motivation for creating open source ELT as a business
Can you describe how the Airbyte platform is implemented?
What was your motivation for choosing Java as the primary language?
incidental complexity of forcing all connectors to be packaged as containers
shortcomings of the Singer specification/motivation for creating a backwards incompatible interface
perceived potential for community adoption of Airbyte specification
tradeoffs of using JSON as interchange format vs. e.g. protobuf/gRPC/Avro/etc.
information lost when converting records to JSON types/how to preserve that information (e.g. field constraints, valid enums, etc.)
interfaces/extension points for integrating with other tools, e.g. Dagster
abstraction layers for simplifying implementation of new connectors
tradeoffs of storing all connectors in a monorepo with the Airbyte core
impact of community adoption/contributions
What is involved in setting up an Airbyte installation?
What are the available axes for scaling an Airbyte deployment?
challenges of setting up and maintaining CI environment for Airbyte
How are you managing governance and long term sustainability of the project?
What are some of the most interesting, unexpected, or innovative ways that you have seen Airbyte used?
What are the most interesting, unexpected, or challenging lessons that you have learned while building Airbyte?
When is Airbyte the wrong choice?
What do you have planned for the future of the project?
Contact Info
Michel
LinkedIn
@MichelTricot on Twitter
michel-tricot on GitHub
John
LinkedIn
@JeanLafleur on Twitter
johnlafleur on GitHub
Parting Question
From your perspective, what is the biggest gap in the tooling or technology for data management today?
Links
Airbyte
Liveramp
Fivetran
Podcast Episode
Stitch Data
Matillion
DataCoral
Podcast Episode
Singer
Meltano
Podcast Episode
Airflow
Podcast.__init__ Episode
Kotlin
Docker
Monorepo
Airbyte Specification
Great Expectations
Podcast Episode
Dagster
Data Engineering Podcast Episode
Podcast.__init__ Episode
Prefect
Podcast Episode
DBT
Podcast Episode
Kubernetes
Snowflake
Podcast Episode
Redshift
Presto
Spark
Parquet
Podcast Episode
The intro and outro music is from The Hug by The Freak Fandango Orchestra / CC BY-SA
Support Data Engineering Podcast
52:1523/02/2021
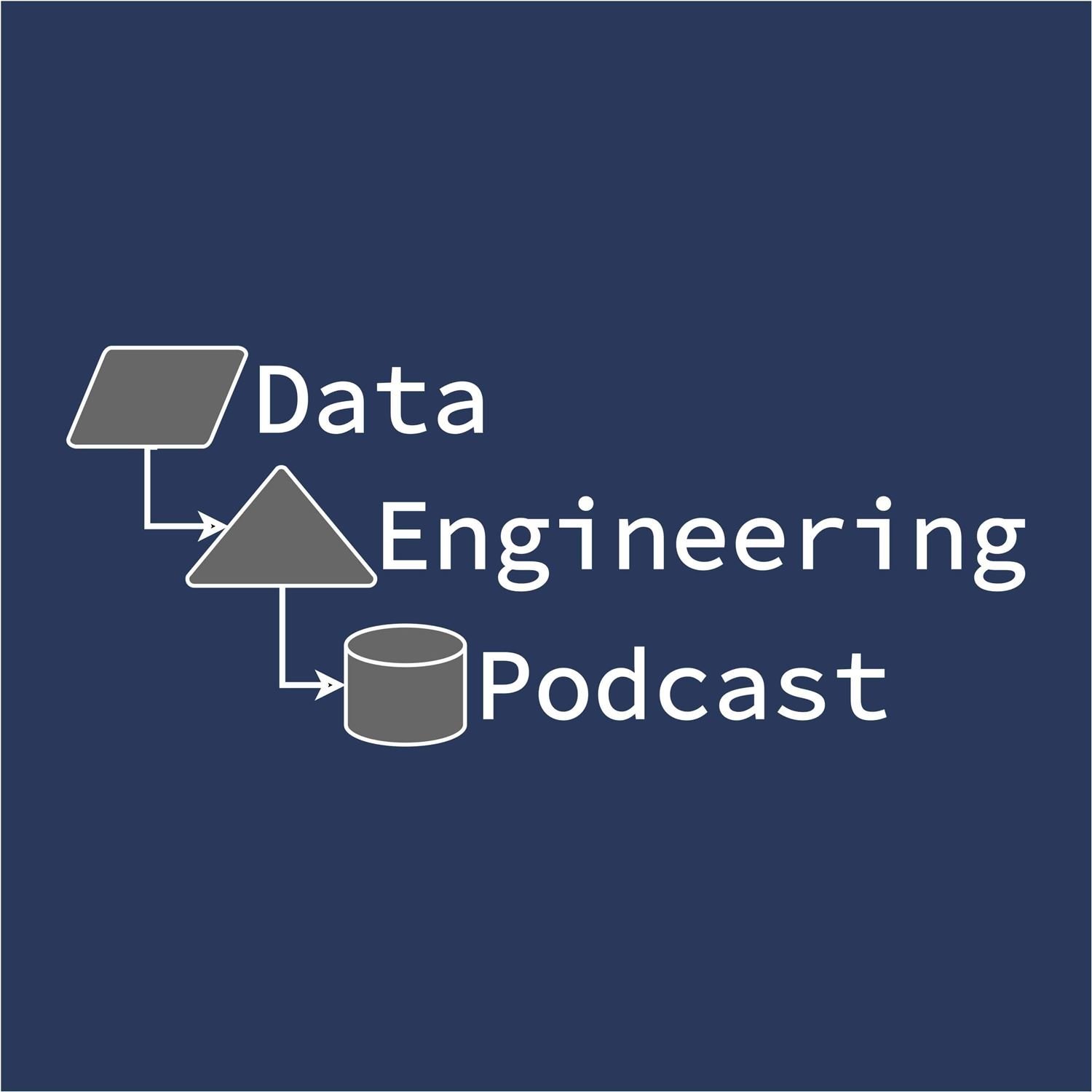
Building The Foundations For Data Driven Businesses at 5xData
Summary
Every business aims to be data driven, but not all of them succeed in that effort. In order to be able to truly derive insights from the data that an organization collects, there are certain foundational capabilities that they need to have capacity for. In order to help more businesses build those foundations, Tarush Aggarwal created 5xData, offering collaborative workshops to assist in setting up the technical and organizational systems that are necessary to succeed. In this episode he shares his thoughts on the core elements that are necessary for every business to be data driven, how he is helping companies incorporate those capabilities into their structure, and the ongoing support that he is providing through a network of mastermind groups. This is a great conversation about the initial steps that every group should be thinking of as they start down the road to making data informed decisions.
Announcements
Hello and welcome to the Data Engineering Podcast, the show about modern data management
When you’re ready to build your next pipeline, or want to test out the projects you hear about on the show, you’ll need somewhere to deploy it, so check out our friends at Linode. With their managed Kubernetes platform it’s now even easier to deploy and scale your workflows, or try out the latest Helm charts from tools like Pulsar and Pachyderm. With simple pricing, fast networking, object storage, and worldwide data centers, you’ve got everything you need to run a bulletproof data platform. Go to dataengineeringpodcast.com/linode today and get a $100 credit to try out a Kubernetes cluster of your own. And don’t forget to thank them for their continued support of this show!
Modern Data teams are dealing with a lot of complexity in their data pipelines and analytical code. Monitoring data quality, tracing incidents, and testing changes can be daunting and often takes hours to days. Datafold helps Data teams gain visibility and confidence in the quality of their analytical data through data profiling, column-level lineage and intelligent anomaly detection. Datafold also helps automate regression testing of ETL code with its Data Diff feature that instantly shows how a change in ETL or BI code affects the produced data, both on a statistical level and down to individual rows and values. Datafold integrates with all major data warehouses as well as frameworks such as Airflow & dbt and seamlessly plugs into CI workflows. Go to dataengineeringpodcast.com/datafold today to start a 30-day trial of Datafold. Once you sign up and create an alert in Datafold for your company data, they will send you a cool water flask.
RudderStack’s smart customer data pipeline is warehouse-first. It builds your customer data warehouse and your identity graph on your data warehouse, with support for Snowflake, Google BigQuery, Amazon Redshift, and more. Their SDKs and plugins make event streaming easy, and their integrations with cloud applications like Salesforce and ZenDesk help you go beyond event streaming. With RudderStack you can use all of your customer data to answer more difficult questions and then send those insights to your whole customer data stack. Sign up free at dataengineeringpodcast.com/rudder today.
Your host is Tobias Macey and today I’m interviewing Tarush Aggarwal about his mission at 5xData to teach companies how to build solid foundations for their data capabilities
Interview
Introduction
How did you get involved in the area of data management?
Can you start by giving an overview of what you are building at 5xData and the story behind it?
impact of industry on challenges in becoming data driven
profile of companies that you are trying to work with
common mistakes when designing data platform
misconceptions that the business has around how to invest in data
challenges in attracting/interviewing/hiring data talent
What are the core components that you have standardized on for building the foundational layers of the data platform?
providing context and training to business users in order to allow them to self-serve the answers to their questions
tooling/interfaces needed to allow them to ask and investigate questions
most high impact areas for data engineers to focus on in the initial stages of implementing the data platform
how to identify and prioritize areas of effort
useful structure of data team at different stages of maturity
What are the most interesting, unexpected, or challenging lessons that you have learned while building out the business and team of 5xData?
What do you have planned for the future of the business?
What are the industry trends or specific technologies that you are keeping a close watch on?
Contact Info
LinkedIn
@tarush on Twitter
Parting Question
From your perspective, what is the biggest gap in the tooling or technology for data management today?
Closing Announcements
Thank you for listening! Don’t forget to check out our other show, Podcast.__init__ to learn about the Python language, its community, and the innovative ways it is being used.
Visit the site to subscribe to the show, sign up for the mailing list, and read the show notes.
If you’ve learned something or tried out a project from the show then tell us about it! Email [email protected]) with your story.
To help other people find the show please leave a review on iTunes and tell your friends and co-workers
Join the community in the new Zulip chat workspace at dataengineeringpodcast.com/chat
Links
5xData
Looker
Podcast Episode
Snowflake
Podcast Episode
Fivetran
Podcast Episode
DBT
Podcast Episode
The intro and outro music is from The Hug by The Freak Fandango Orchestra / CC BY-SA
Support Data Engineering Podcast
52:1616/02/2021
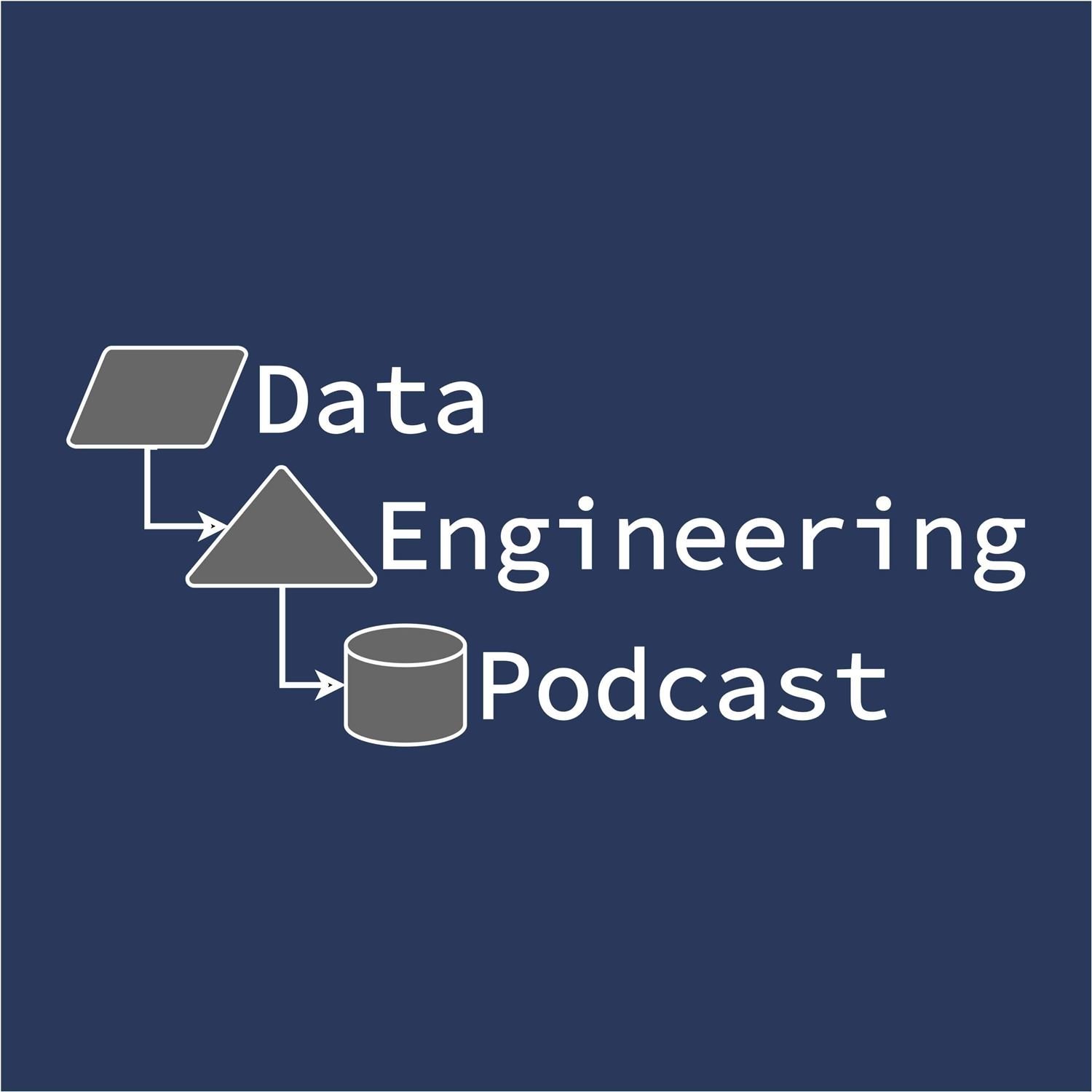
How Shopify Is Building Their Production Data Warehouse Using DBT
Summary
With all of the tools and services available for building a data platform it can be difficult to separate the signal from the noise. One of the best ways to get a true understanding of how a technology works in practice is to hear from people who are running it in production. In this episode Zeeshan Qureshi and Michelle Ark share their experiences using DBT to manage the data warehouse for Shopify. They explain how the structured the project to allow for multiple teams to collaborate in a scalable manner, the additional tooling that they added to address the edge cases that they have run into, and the optimizations that they baked into their continuous integration process to provide fast feedback and reduce costs. This is a great conversation about the lessons learned from real world use of a specific technology and how well it lives up to its promises.
Announcements
Hello and welcome to the Data Engineering Podcast, the show about modern data management
When you’re ready to build your next pipeline, or want to test out the projects you hear about on the show, you’ll need somewhere to deploy it, so check out our friends at Linode. With their managed Kubernetes platform it’s now even easier to deploy and scale your workflows, or try out the latest Helm charts from tools like Pulsar and Pachyderm. With simple pricing, fast networking, object storage, and worldwide data centers, you’ve got everything you need to run a bulletproof data platform. Go to dataengineeringpodcast.com/linode today and get a $100 credit to try out a Kubernetes cluster of your own. And don’t forget to thank them for their continued support of this show!
Modern Data teams are dealing with a lot of complexity in their data pipelines and analytical code. Monitoring data quality, tracing incidents, and testing changes can be daunting and often takes hours to days. Datafold helps Data teams gain visibility and confidence in the quality of their analytical data through data profiling, column-level lineage and intelligent anomaly detection. Datafold also helps automate regression testing of ETL code with its Data Diff feature that instantly shows how a change in ETL or BI code affects the produced data, both on a statistical level and down to individual rows and values. Datafold integrates with all major data warehouses as well as frameworks such as Airflow & dbt and seamlessly plugs into CI workflows. Go to dataengineeringpodcast.com/datafold today to start a 30-day trial of Datafold. Once you sign up and create an alert in Datafold for your company data, they will send you a cool water flask.
Today’s episode of Data Engineering Podcast is sponsored by Datadog, the monitoring and analytics platform for cloud-scale infrastructure and applications. Datadog’s machine-learning based alerts, customizable dashboards, and 400+ vendor-backed integrations makes it easy to unify disparate data sources and pivot between correlated metrics and events for faster troubleshooting. By combining metrics, traces, and logs in one place, you can easily improve your application performance. Try Datadog free by starting a your 14-day trial and receive a free t-shirt once you install the agent. Go to dataengineeringpodcast.com/datadog today see how you can unify your monitoring today.
Your host is Tobias Macey and today I’m interviewing Zeeshan Qureshi and Michelle Ark about how Shopify is building their production data warehouse platform with DBT
Interview
Introduction
How did you get involved in the area of data management?
Can you start by giving an overview of what the Shopify platform is?
What kinds of data sources are you working with?
Can you share some examples of the types of analysis, decisions, and products that you are building with the data that you manage?
How have you structured your data teams to be able to deliver those projects?
What are the systems that you have in place, technological or otherwise, to allow you to support the needs of the various data professionals and business users?
What was the tipping point that led you to reconsider your system design and start down the road of architecting a data warehouse?
What were your criteria when selecting a platform for your data warehouse?
What decision did that criteria lead you to make?
Once you decided to orient a large portion of your reporting around a data warehouse, what were the biggest unknowns that you were faced with while deciding how to structure the workflows and access policies?
What were your criteria for determining what toolchain to use for managing the data warehouse?
You ultimately decided to standardize on DBT. What were the other options that you explored and what were the requirements that you had for determining the candidates?
What was your process for onboarding users into the DBT toolchain and determining how to structure the project layout?
What are some of the shortcomings or edge cases that you ran into?
Rather than rely on the vanilla DBT workflow you created a wrapper project to add additional functionality. What were some of the features that you needed to add to suit your particular needs?
What has been your experience with extending and integrating with DBT to customize it for your environment?
Can you talk through how you manage testing of your DBT pipelines and the tables that it is responsible for?
How much of the testing are you able to do with out-of-the-box functionality from DBT?
What are the additional capabilities that you have bolted on to provide a more robust and scalable means of verifying your pipeline changes?
Can you share how you manage the CI/CD process for changes in your data warehouse?
What kinds of monitoring or metrics collection do you perform on the execution of your DBT pipelines?
How do you integrate the management of your data warehouse and DBT workflows with your broader data platform?
Now that you have been using DBT in production for a while, what are the challenges that you have encountered when using it at scale?
Are there any patterns that you and your team have found useful that are worth digging into for other teams who are considering DBT or are actively using it?
What are the opportunities and available mechanisms that you have found for introducing abstraction layers to reduce the maintenance burden for your data warehouse?
What is the data modeling approach that you are using? (e.g. Data Vault, Star/Snowflake Schema, wide tables, etc.)
As you continue to work with DBT and rely on the data warehouse for production use cases, what are some of the additional features/improvements that you have planned?
What are some of the unexpected/innovative/surprising use cases that you and your team have found for the Seamster tool or the data models that it generates?
What are the cases where you think that DBT or data warehousing is the wrong answer and teams should be looking to other solutions?
What are the most interesting, unexpected, or challenging lessons that you learned while working through the process of migrating a portion of your data workloads into the data warehouse and managing them with DBT?
Contact Info
Zeeshan
@zeeshanq on Twitter
Website
Michelle
@michellearky on Twitter
LinkedIn
Parting Question
From your perspective, what is the biggest gap in the tooling or technology for data management today?
Closing Announcements
Thank you for listening! Don’t forget to check out our other show, Podcast.__init__ to learn about the Python language, its community, and the innovative ways it is being used.
Visit the site to subscribe to the show, sign up for the mailing list, and read the show notes.
If you’ve learned something or tried out a project from the show then tell us about it! Email [email protected]) with your story.
To help other people find the show please leave a review on iTunes and tell your friends and co-workers
Join the community in the new Zulip chat workspace at dataengineeringpodcast.com/chat
Links
How to Build a Production Grade Workflow with SQL Modelling
Shopify
JRuby
PySpark
Druid
Amplitude
Mode
Snowflake Schema
Data Vault
Podcast Episode
BigQuery
Amazon Redshift
CI/CD
Great Expectations
Podcast Episode
Master Data Management
Podcast Episode
Flink SQL
The intro and outro music is from The Hug by The Freak Fandango Orchestra / CC BY-SA
Support Data Engineering Podcast
46:3109/02/2021
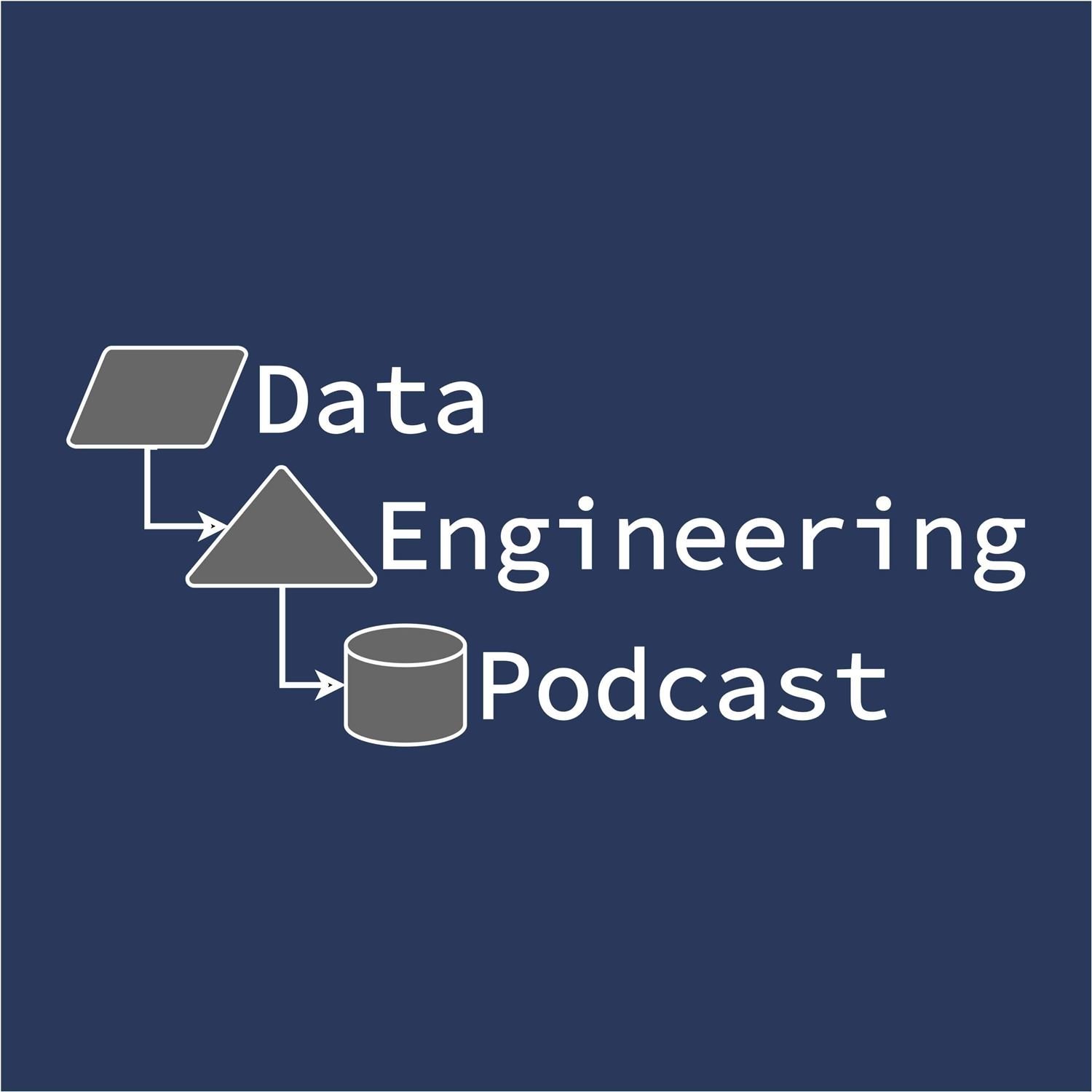
System Observability For The Cloud Native Era With Chronosphere
Summary
Collecting and processing metrics for monitoring use cases is an interesting data problem. It is eminently possible to generate millions or billions of data points per second, the information needs to be propagated to a central location, processed, and analyzed in timeframes on the order of milliseconds or single-digit seconds, and the consumers of the data need to be able to query the information quickly and flexibly. As the systems that we build continue to grow in scale and complexity the need for reliable and manageable monitoring platforms increases proportionately. In this episode Rob Skillington, CTO of Chronosphere, shares his experiences building metrics systems that provide observability to companies that are operating at extreme scale. He describes how the M3DB storage engine is designed to manage the pressures of a critical system component, the inherent complexities of working with telemetry data, and the motivating factors that are contributing to the growing need for flexibility in querying the collected metrics. This is a fascinating conversation about an area of data management that is often taken for granted.
Announcements
Hello and welcome to the Data Engineering Podcast, the show about modern data management
When you’re ready to build your next pipeline, or want to test out the projects you hear about on the show, you’ll need somewhere to deploy it, so check out our friends at Linode. With their managed Kubernetes platform it’s now even easier to deploy and scale your workflows, or try out the latest Helm charts from tools like Pulsar and Pachyderm. With simple pricing, fast networking, object storage, and worldwide data centers, you’ve got everything you need to run a bulletproof data platform. Go to dataengineeringpodcast.com/linode today and get a $100 credit to try out a Kubernetes cluster of your own. And don’t forget to thank them for their continued support of this show!
Modern Data teams are dealing with a lot of complexity in their data pipelines and analytical code. Monitoring data quality, tracing incidents, and testing changes can be daunting and often takes hours to days. Datafold helps Data teams gain visibility and confidence in the quality of their analytical data through data profiling, column-level lineage and intelligent anomaly detection. Datafold also helps automate regression testing of ETL code with its Data Diff feature that instantly shows how a change in ETL or BI code affects the produced data, both on a statistical level and down to individual rows and values. Datafold integrates with all major data warehouses as well as frameworks such as Airflow & dbt and seamlessly plugs into CI workflows. Go to dataengineeringpodcast.com/datafold today to start a 30-day trial of Datafold. Once you sign up and create an alert in Datafold for your company data, they will send you a cool water flask.
Today’s episode of Data Engineering Podcast is sponsored by Datadog, the monitoring and analytics platform for cloud-scale infrastructure and applications. Datadog’s machine-learning based alerts, customizable dashboards, and 400+ vendor-backed integrations makes it easy to unify disparate data sources and pivot between correlated metrics and events for faster troubleshooting. By combining metrics, traces, and logs in one place, you can easily improve your application performance. Try Datadog free by starting a your 14-day trial and receive a free t-shirt once you install the agent. Go to dataengineeringpodcast.com/datadog today see how you can unify your monitoring today.
Your host is Tobias Macey and today I’m interviewing Rob Skillington about Chronosphere, a scalable, reliable and customizable monitoring-as-a-service purpose built for cloud-native applications.
Interview
Introduction
How did you get involved in the area of data management?
Can you start by describing what you are building at Chronosphere and your motivation for turning it into a business?
What are the biggest challenges inherent to monitoring use cases?
How does the advent of cloud native environments complicate things further?
While you were at Uber you helped to create the M3 storage engine. There are a wide array of time series databases available, including many purpose built for metrics use cases. What were the missing pieces that made it necessary to create a new system?
How do you handle schema design/data modeling for metrics storage?
How do the usage patterns of metrics systems contribute to the complexity of building a storage layer to support them?
What are the optimizations that need to be made for the read and write paths in M3?
How do you handle high cardinality of metrics and ad-hoc queries to understand system behaviors?
What are the scaling factors for M3?
Can you describe how you have architected the Chronosphere platform?
What are the convenience features built on top of M3 that you are creating at Chronosphere?
How do you handle deployment and scaling of your infrastructure given the scale of the businesses that you are working with?
Beyond just server infrastructure and application behavior, what are some of the other sources of metrics that you and your users are sending into Chronosphere?
How do those alternative metrics sources complicate the work of generating useful insights from the data?
In addition to the read and write loads, metrics systems also need to be able to identify patterns, thresholds, and anomalies in the data to alert on it with minimal latency. How do you handle that in the Chronosphere platform?
What are some of the most interesting, innovative, or unexpected ways that you have seen Chronosphere/M3 used?
What are some of the most interesting, unexpected, or challenging lessons that you have learned while building Chronosphere?
When is Chronosphere the wrong choice?
What do you have planned for the future of the platform and business?
Contact Info
LinkedIn
@roskilli on Twitter
robskillington on GitHub
Parting Question
From your perspective, what is the biggest gap in the tooling or technology for data management today?
Closing Announcements
Thank you for listening! Don’t forget to check out our other show, Podcast.__init__ to learn about the Python language, its community, and the innovative ways it is being used.
Visit the site to subscribe to the show, sign up for the mailing list, and read the show notes.
If you’ve learned something or tried out a project from the show then tell us about it! Email [email protected]) with your story.
To help other people find the show please leave a review on iTunes and tell your friends and co-workers
Join the community in the new Zulip chat workspace at dataengineeringpodcast.com/chat
Links
Chronosphere
Lidar
Cloud Native
M3DB
OpenTracing
Metrics/Telemetry
Graphite
Podcast.__init__ Episode
InfluxDB
Clickhouse
Podcast Episode
Prometheus
Inverted Index
Druid
Cardinality
Apache Flink
Podcast Episode
HDFS
Avro
Podcast Episode
Grafana
Tecton
Podcast Episode
Datadog
Podcast Episode
Kubernetes
Sourcegraph
The intro and outro music is from The Hug by The Freak Fandango Orchestra / CC BY-SA
Support Data Engineering Podcast
01:04:5002/02/2021
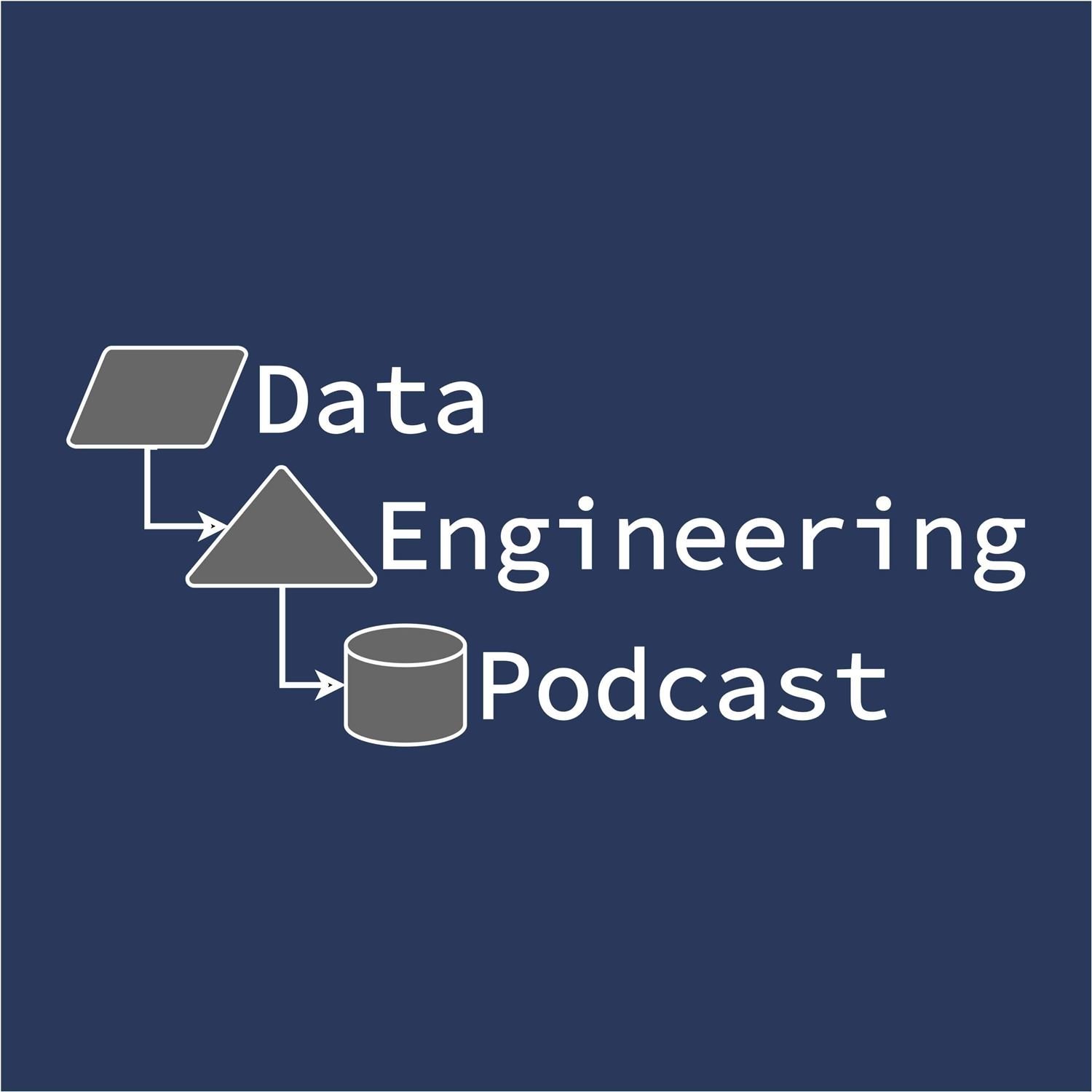
Making It Easier To Stick B2B Data Integration Pipelines Together With Hotglue
Summary
Businesses often need to be able to ingest data from their customers in order to power the services that they provide. For each new source that they need to integrate with it is another custom set of ETL tasks that they need to maintain. In order to reduce the friction involved in supporting new data transformations David Molot and Hassan Syyid built the Hotlue platform. In this episode they describe the data integration challenges facing many B2B companies, how their work on the Hotglue platform simplifies their efforts, and how they have designed the platform to make these ETL workloads embeddable and self service for end users.
Announcements
Hello and welcome to the Data Engineering Podcast, the show about modern data management
When you’re ready to build your next pipeline, or want to test out the projects you hear about on the show, you’ll need somewhere to deploy it, so check out our friends at Linode. With their managed Kubernetes platform it’s now even easier to deploy and scale your workflows, or try out the latest Helm charts from tools like Pulsar and Pachyderm. With simple pricing, fast networking, object storage, and worldwide data centers, you’ve got everything you need to run a bulletproof data platform. Go to dataengineeringpodcast.com/linode today and get a $60 credit to try out a Kubernetes cluster of your own. And don’t forget to thank them for their continued support of this show!
Modern Data teams are dealing with a lot of complexity in their data pipelines and analytical code. Monitoring data quality, tracing incidents, and testing changes can be daunting and often takes hours to days. Datafold helps Data teams gain visibility and confidence in the quality of their analytical data through data profiling, column-level lineage and intelligent anomaly detection. Datafold also helps automate regression testing of ETL code with its Data Diff feature that instantly shows how a change in ETL or BI code affects the produced data, both on a statistical level and down to individual rows and values. Datafold integrates with all major data warehouses as well as frameworks such as Airflow & dbt and seamlessly plugs into CI workflows. Go to dataengineeringpodcast.com/datafold today to start a 30-day trial of Datafold. Once you sign up and create an alert in Datafold for your company data, they will send you a cool water flask.
This episode of Data Engineering Podcast is sponsored by Datadog, a unified monitoring and analytics platform built for developers, IT operations teams, and businesses in the cloud age. Datadog provides customizable dashboards, log management, and machine-learning-based alerts in one fully-integrated platform so you can seamlessly navigate, pinpoint, and resolve performance issues in context. Monitor all your databases, cloud services, containers, and serverless functions in one place with Datadog’s 400+ vendor-backed integrations. If an outage occurs, Datadog provides seamless navigation between your logs, infrastructure metrics, and application traces in just a few clicks to minimize downtime. Try it yourself today by starting a free 14-day trial and receive a Datadog t-shirt after installing the agent. Go to dataengineeringpodcast.com/datadog today to see how you can enhance visibility into your stack with Datadog.
Your host is Tobias Macey and today I’m interviewing David Molot and Hassan Syyid about Hotglue, an embeddable data integration tool for B2B developers built on the Python ecosystem.
Interview
Introduction
How did you get involved in the area of data management?
Can you start by describing what you are building at Hotglue?
What was your motivation for starting a business to address this particular problem?
Who is the target user of Hotglue and what are their biggest data problems?
What are the types and sources of data that they are likely to be working with?
How are they currently handling solutions for those problems?
How does the introduction of Hotglue simplify or improve their work?
What is involved in getting Hotglue integrated into a given customer’s environment?
How is Hotglue itself implemented?
How has the design or goals of the platform evolved since you first began building it?
What were some of the initial assumptions that you had at the outset and how well have they held up as you progressed?
Once a customer has set up Hotglue what is their workflow for building and executing an ETL workflow?
What are their options for working with sources that aren’t supported out of the box?
What are the biggest design and implementation challenges that you are facing given the need for your product to be embedded in customer platforms and exposed to their end users?
What are some of the most interesting, innovative, or unexpected ways that you have seen Hotglue used?
What are the most interesting, unexpected, or challenging lessons that you have learned while building Hotglue?
When is Hotglue the wrong choice?
What do you have planned for the future of the product?
Contact Info
David
@davidmolot on Twitter
LinkedIn
Hassan
hsyyid on GitHub
LinkedIn
Parting Question
From your perspective, what is the biggest gap in the tooling or technology for data management today?
Closing Announcements
Thank you for listening! Don’t forget to check out our other show, Podcast.__init__ to learn about the Python language, its community, and the innovative ways it is being used.
Visit the site to subscribe to the show, sign up for the mailing list, and read the show notes.
If you’ve learned something or tried out a project from the show then tell us about it! Email [email protected]) with your story.
To help other people find the show please leave a review on iTunes and tell your friends and co-workers
Join the community in the new Zulip chat workspace at dataengineeringpodcast.com/chat
Links
Hotglue
Python
The Python Podcast.__init__
B2B == Business to Business
Meltano
Podcast Episode
Airbyte
Singer
The intro and outro music is from The Hug by The Freak Fandango Orchestra / CC BY-SA
Support Data Engineering Podcast
34:0526/01/2021
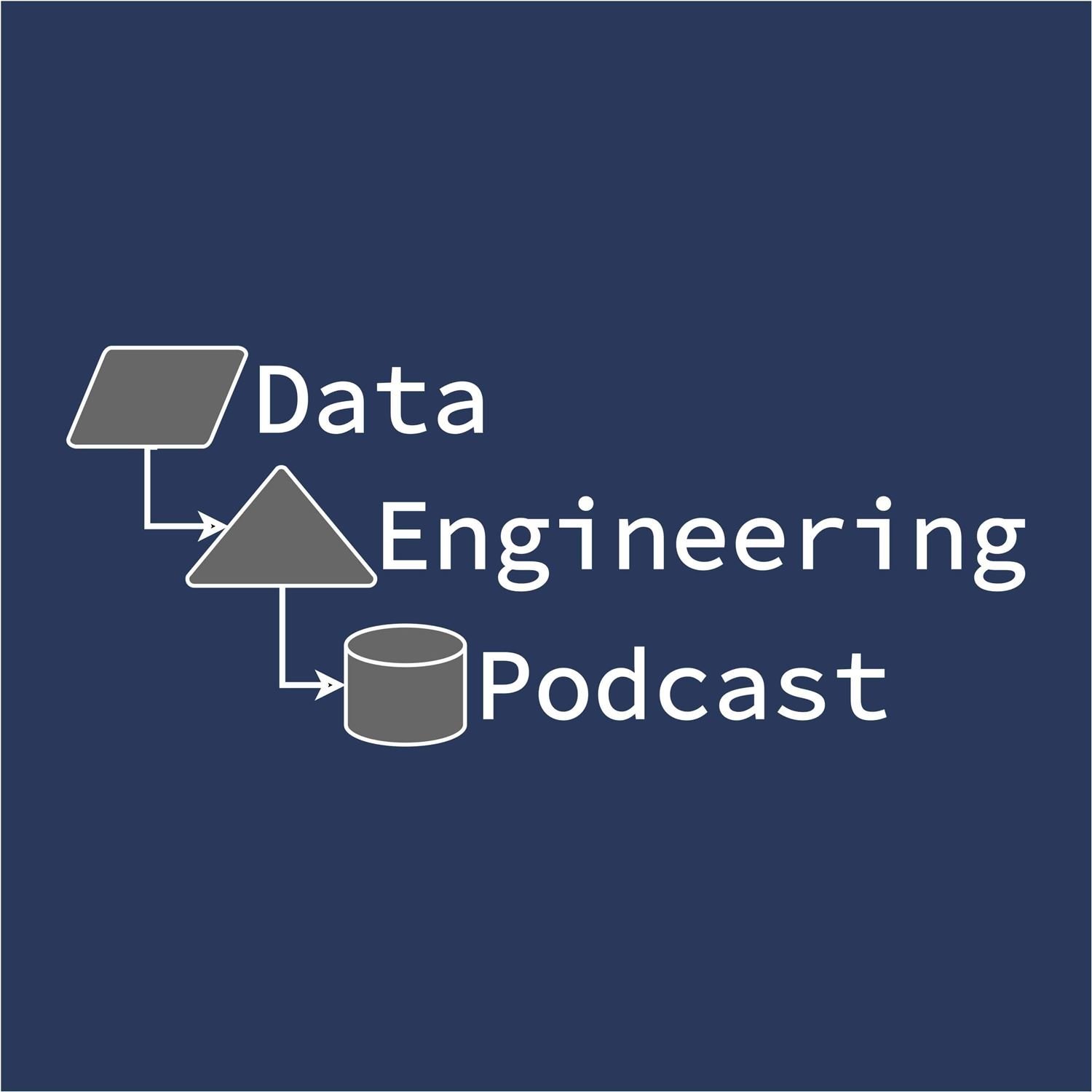
Using Your Data Warehouse As The Source Of Truth For Customer Data With Hightouch
Summary
The data warehouse has become the central component of the modern data stack. Building on this pattern, the team at Hightouch have created a platform that synchronizes information about your customers out to third party systems for use by marketing and sales teams. In this episode Tejas Manohar explains the benefits of sourcing customer data from one location for all of your organization to use, the technical challenges of synchronizing the data to external systems with varying APIs, and the workflow for enabling self-service access to your customer data by your marketing teams. This is an interesting conversation about the importance of the data warehouse and how it can be used beyond just internal analytics.
Announcements
Hello and welcome to the Data Engineering Podcast, the show about modern data management
When you’re ready to build your next pipeline, or want to test out the projects you hear about on the show, you’ll need somewhere to deploy it, so check out our friends at Linode. With their managed Kubernetes platform it’s now even easier to deploy and scale your workflows, or try out the latest Helm charts from tools like Pulsar and Pachyderm. With simple pricing, fast networking, object storage, and worldwide data centers, you’ve got everything you need to run a bulletproof data platform. Go to dataengineeringpodcast.com/linode today and get a $60 credit to try out a Kubernetes cluster of your own. And don’t forget to thank them for their continued support of this show!
Modern Data teams are dealing with a lot of complexity in their data pipelines and analytical code. Monitoring data quality, tracing incidents, and testing changes can be daunting and often takes hours to days. Datafold helps Data teams gain visibility and confidence in the quality of their analytical data through data profiling, column-level lineage and intelligent anomaly detection. Datafold also helps automate regression testing of ETL code with its Data Diff feature that instantly shows how a change in ETL or BI code affects the produced data, both on a statistical level and down to individual rows and values. Datafold integrates with all major data warehouses as well as frameworks such as Airflow & dbt and seamlessly plugs into CI workflows. Go to dataengineeringpodcast.com/datafold today to start a 30-day trial of Datafold. Once you sign up and create an alert in Datafold for your company data, they will send you a cool water flask.
This episode of Data Engineering Podcast is sponsored by Datadog, a unified monitoring and analytics platform built for developers, IT operations teams, and businesses in the cloud age. Datadog provides customizable dashboards, log management, and machine-learning-based alerts in one fully-integrated platform so you can seamlessly navigate, pinpoint, and resolve performance issues in context. Monitor all your databases, cloud services, containers, and serverless functions in one place with Datadog’s 400+ vendor-backed integrations. If an outage occurs, Datadog provides seamless navigation between your logs, infrastructure metrics, and application traces in just a few clicks to minimize downtime. Try it yourself today by starting a free 14-day trial and receive a Datadog t-shirt after installing the agent. Go to dataengineeringpodcast.com/datadog today to see how you can enhance visibility into your stack with Datadog.
Your host is Tobias Macey and today I’m interviewing Tejas Manohar about Hightouch, a data platform that helps you sync your customer data from your data warehouse to your CRM, marketing, and support tools
Interview
Introduction
How did you get involved in the area of data management?
Can you start by giving an overview of what you are building at Hightouch and your motivation for creating it?
What are the main points of friction for teams who are trying to make use of customer data?
Where is Hightouch positioned in the ecosystem of customer data tools such as Segment, Mixpanel, Amplitude, etc.?
Who are the target users of Hightouch?
How has that influenced the design of the platform?
What are the baseline attributes necessary for Hightouch to populate downstream systems?
What are the data modeling considerations that users need to be aware of when sending data to other platforms?
Can you describe how Hightouch is architected?
How has the design of the platform evolved since you first began working on it?
What goals or assumptions did you have when you first began building Hightouch that have been modified or invalidated once you began working with customers?
Can you talk through the workflow of using Hightouch to propagate data to other platforms?
How do you keep data up to date between the warehouse and downstream systems?
What are the upstream systems that users need to have in place to make Hightouch a viable and effective tool?
What are the benefits of using the data warehouse as the source of truth for downstream services?
What are the trends in data warehousing that you are keeping a close eye on?
What are you most excited for?
Are there any that you find worrisome?
What are some of the most interesting, unexpected, or innovative ways that you have seen Hightouch used?
What are the most interesting, unexpected, or challenging lessons that you have learned while building Hightouch?
When is Hightouch the wrong choice?
What do you have planned for the future of the platform?
Contact Info
LinkedIn
@tejasmanohar on Twitter
tejasmanoharon GitHub
Parting Question
From your perspective, what is the biggest gap in the tooling or technology for data management today?
Links
Hightouch
Segment
Podcast Episode
DBT
Podcast Episode
Looker
Podcast Episode
Change Data Capture
Podcast Episode
Database Trigger
Materialize
Podcast Episode
Flink
Podcast Episode
Zapier
The intro and outro music is from The Hug by The Freak Fandango Orchestra / CC BY-SA
Support Data Engineering Podcast
59:3419/01/2021
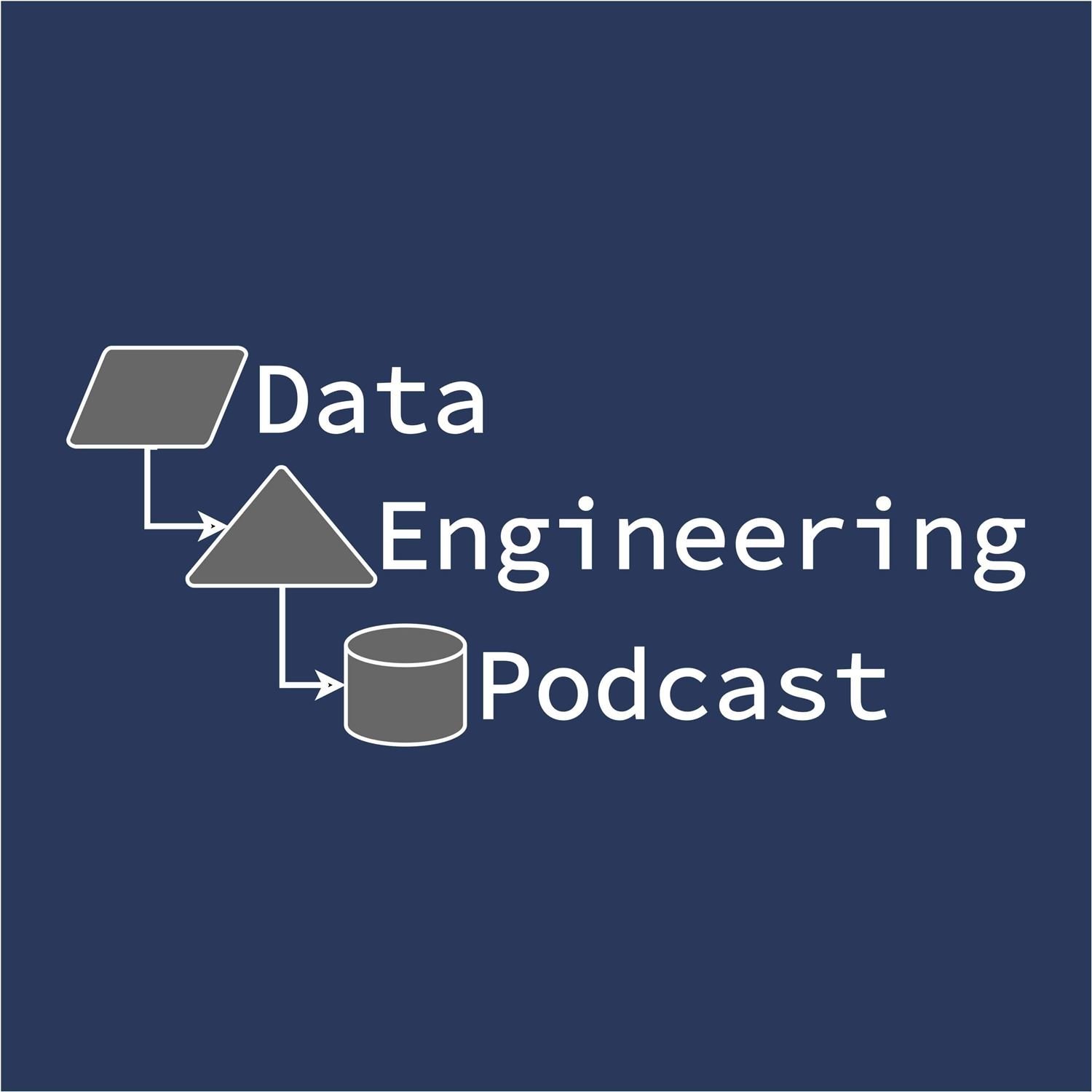
Enabling Version Controlled Data Collaboration With TerminusDB
Summary
As data professionals we have a number of tools available for storing, processing, and analyzing data. We also have tools for collaborating on software and analysis, but collaborating on data is still an underserved capability. Gavin Mendel-Gleason encountered this problem first hand while working on the Sesshat databank, leading him to create TerminusDB and TerminusHub. In this episode he explains how the TerminusDB system is architected to provide a versioned graph storage engine that allows for branching and merging of data sets, how that opens up new possibilities for individuals and teams to work together on building new data repositories. This is a fascinating conversation on the technical challenges involved, the opportunities that such as system provides, and the complexities inherent to building a successful business on open source.
Announcements
Hello and welcome to the Data Engineering Podcast, the show about modern data management
When you’re ready to build your next pipeline, or want to test out the projects you hear about on the show, you’ll need somewhere to deploy it, so check out our friends at Linode. With their managed Kubernetes platform it’s now even easier to deploy and scale your workflows, or try out the latest Helm charts from tools like Pulsar and Pachyderm. With simple pricing, fast networking, object storage, and worldwide data centers, you’ve got everything you need to run a bulletproof data platform. Go to dataengineeringpodcast.com/linode today and get a $60 credit to try out a Kubernetes cluster of your own. And don’t forget to thank them for their continued support of this show!
Do you want to get better at Python? Now is an excellent time to take an online course. Whether you’re just learning Python or you’re looking for deep dives on topics like APIs, memory mangement, async and await, and more, our friends at Talk Python Training have a top-notch course for you. If you’re just getting started, be sure to check out the Python for Absolute Beginners course. It’s like the first year of computer science that you never took compressed into 10 fun hours of Python coding and problem solving. Go to dataengineeringpodcast.com/talkpython today and get 10% off the course that will help you find your next level. That’s dataengineeringpodcast.com/talkpython, and don’t forget to thank them for supporting the show.
You invest so much in your data infrastructure – you simply can’t afford to settle for unreliable data. Fortunately, there’s hope: in the same way that New Relic, DataDog, and other Application Performance Management solutions ensure reliable software and keep application downtime at bay, Monte Carlo solves the costly problem of broken data pipelines. Monte Carlo’s end-to-end Data Observability Platform monitors and alerts for data issues across your data warehouses, data lakes, ETL, and business intelligence. The platform uses machine learning to infer and learn your data, proactively identify data issues, assess its impact through lineage, and notify those who need to know before it impacts the business. By empowering data teams with end-to-end data reliability, Monte Carlo helps organizations save time, increase revenue, and restore trust in their data. Visit dataengineeringpodcast.com/montecarlo today to request a demo and see how Monte Carlo delivers data observability across your data infrastructure. The first 25 will receive a free, limited edition Monte Carlo hat!
Your host is Tobias Macey and today I’m interviewing Gavin Mendel-Gleason about TerminusDB, an open source model driven graph database for knowledge graph representation
Interview
Introduction
How did you get involved in the area of data management?
Can you start by describing what TerminusDB is and what motivated you to build it?
What are the use cases that TerminusDB and TerminusHub are designed for?
There are a number of different reasons and methods for versioning data, such as the work being done with Datomic, LakeFS, DVC, etc. Where does TerminusDB fit in relation to those and other data versioning systems that are available today?
Can you describe how TerminusDB is implemented?
How has the design changed or evolved since you first began working on it?
What was the decision process and design considerations that led you to choose Prolog as the implementation language?
One of the challenges that have faced other knowledge engines built around RDF is that of scale and performance. How are you addressing those difficulties in TerminusDB?
What are the scaling factors and limitations for TerminusDB? (e.g. volumes of data, clustering, etc.)
How does the use of RDF triples and JSON-LD impact the audience for TerminusDB?
How much overhead is incurred by maintaining a long history of changes for a database?
How do you handle garbage collection/compaction of versions?
How does the availability of branching and merging strategies change the approach that data teams take when working on a project?
What are the edge cases in merging and conflict resolution, and what tools does TerminusDB/TerminusHub provide for working through those situations?
What are some useful strategies that teams should be aware of for working effectively with collaborative datasets in TerminusDB?
Another interesting element of the TerminusDB platform is the query language. What did you use as inspiration for designing it and how much of a learning curve is involved?
What are some of the most interesting, innovative, or unexpected ways that you have seen TerminusDB used?
https://en.wikipedia.org/wiki/Semantic_Web-?utm_source=rss&utm_medium=rss What are the most interesting, unexpected, or challenging lessons that you have learned while building TerminusDB and TerminusHub?
When is TerminusDB the wrong choice?
What do you have planned for the future of the project?
Contact Info
@GavinMGleason on Twitter
LinkedIn
GavinMendelGleason on GitHub
Parting Question
From your perspective, what is the biggest gap in the tooling or technology for data management today?
Links
TerminusDB
TerminusHub
Chem Informatics
Type Theory
Graph Database
Trinity College Dublin
Sesshat Databank analytics over civilizations in history
PostgreSQL
DGraph
Grakn
Neo4J
Datomic
LakeFS
DVC
Dolt
Persistent Succinct Data Structure
Currying
Prolog
WOQL TerminusDB query language
RDF
JSON-LD
Semantic Web
Property Graph
Hypergraph
Super Node
Bloom Filters
Data Curation
Podcast Episode
CRDT == Conflict-Free Replicated Data Types
Podcast Episode
SPARQL
Datalog
AST == Abstract Syntax Tree
The intro and outro music is from The Hug by The Freak Fandango Orchestra / CC BY-SA
Support Data Engineering Podcast
57:4811/01/2021
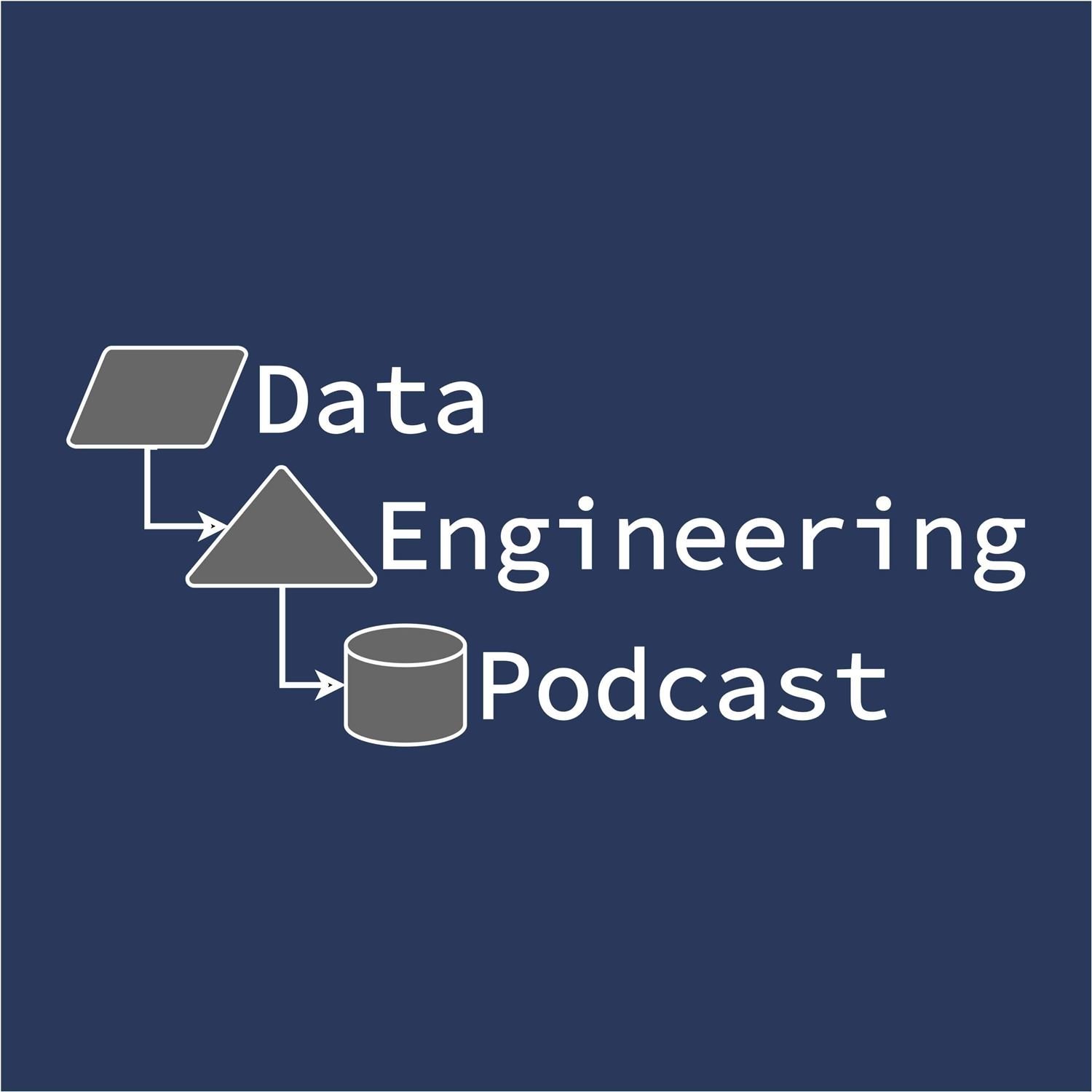
Bringing Feature Stores and MLOps to the Enterprise at Tecton
Summary
As more organizations are gaining experience with data management and incorporating analytics into their decision making, their next move is to adopt machine learning. In order to make those efforts sustainable, the core capability they need is for data scientists and analysts to be able to build and deploy features in a self service manner. As a result the feature store is becoming a required piece of the data platform. To fill that need Kevin Stumpf and the team at Tecton are building an enterprise feature store as a service. In this episode he explains how his experience building the Michelanagelo platform at Uber has informed the design and architecture of Tecton, how it integrates with your existing data systems, and the elements that are required for well engineered feature store.
Announcements
Hello and welcome to the Data Engineering Podcast, the show about modern data management
When you’re ready to build your next pipeline, or want to test out the projects you hear about on the show, you’ll need somewhere to deploy it, so check out our friends at Linode. With their managed Kubernetes platform it’s now even easier to deploy and scale your workflows, or try out the latest Helm charts from tools like Pulsar and Pachyderm. With simple pricing, fast networking, object storage, and worldwide data centers, you’ve got everything you need to run a bulletproof data platform. Go to dataengineeringpodcast.com/linode today and get a $60 credit to try out a Kubernetes cluster of your own. And don’t forget to thank them for their continued support of this show!
Do you want to get better at Python? Now is an excellent time to take an online course. Whether you’re just learning Python or you’re looking for deep dives on topics like APIs, memory mangement, async and await, and more, our friends at Talk Python Training have a top-notch course for you. If you’re just getting started, be sure to check out the Python for Absolute Beginners course. It’s like the first year of computer science that you never took compressed into 10 fun hours of Python coding and problem solving. Go to dataengineeringpodcast.com/talkpython today and get 10% off the course that will help you find your next level. That’s dataengineeringpodcast.com/talkpython, and don’t forget to thank them for supporting the show.
You invest so much in your data infrastructure – you simply can’t afford to settle for unreliable data. Fortunately, there’s hope: in the same way that New Relic, DataDog, and other Application Performance Management solutions ensure reliable software and keep application downtime at bay, Monte Carlo solves the costly problem of broken data pipelines. Monte Carlo’s end-to-end Data Observability Platform monitors and alerts for data issues across your data warehouses, data lakes, ETL, and business intelligence. The platform uses machine learning to infer and learn your data, proactively identify data issues, assess its impact through lineage, and notify those who need to know before it impacts the business. By empowering data teams with end-to-end data reliability, Monte Carlo helps organizations save time, increase revenue, and restore trust in their data. Visit dataengineeringpodcast.com/montecarlo today to request a demo and see how Monte Carlo delivers data observability across your data infrastructure. The first 25 will receive a free, limited edition Monte Carlo hat!
Your host is Tobias Macey and today I’m interviewing Kevin Stumpf about Tecton and the role that the feature store plays in a modern MLOps platform
Interview
Introduction
How did you get involved in the area of data management?
Can you start by describing what you are building at Tecton and your motivation for starting the business?
For anyone who isn’t familiar with the concept, what is an example of a feature?
How do you define what a feature store is?
What role does a feature store play in the overall lifecycle of a machine learning project?
How would you characterize the current landscape of feature stores?
What are the other components that are necessary for a complete ML operations platform?
At what points in the lifecycle of data does the feature store get integrated?
What types of data can feature stores manage? (e.g. text vs. image/binary vs. spatial, etc.)
How is the Tecton platform implemented?
How has the design evolved since you first began building it?
How did your work on Uber’s Michelangelo inform your work on Tecton?
What is the workflow and lifecycle of developing, testing, and deploying a feature to a feature store?
What aspects of a feature do you monitor to determine whether it has drifted?
How do you define drift in the context of a feature?
How does that differ from drift in an ML model?
How does Tecton handle versioning of features and associating those different versions with the models that are using them?
What are some of the most interesting, innovative, or unexpected projects that you have seen built with Tecton?
When is Tecton the wrong choice?
What do you have planned for the future of the product?
Contact Info
LinkedIn
kevinstumpf on GitHub
@kevinstumpf on Twitter
Parting Question
From your perspective, what is the biggest gap in the tooling or technology for data management today?
Closing Announcements
Thank you for listening! Don’t forget to check out our other show, Podcast.__init__ to learn about the Python language, its community, and the innovative ways it is being used.
Visit the site to subscribe to the show, sign up for the mailing list, and read the show notes.
If you’ve learned something or tried out a project from the show then tell us about it! Email [email protected]) with your story.
To help other people find the show please leave a review on iTunes and tell your friends and co-workers
Join the community in the new Zulip chat workspace at dataengineeringpodcast.com/chat
Links
Tecton
Uber Michelangelo
MLOps
Feature Store
Blog: What Is A Feature Store
StreamSQL
Podcast Episode
AWS Feature Store
Logical Clocks
EMR
Kotlin
DynamoDB
scikit-learn
Tensorflow
MLFlow
Algorithmia
SageMaker
Feast open source feature store
Jaeger
OpenTelemetry
The intro and outro music is from The Hug by The Freak Fandango Orchestra / CC BY-SA
Support Data Engineering Podcast
47:4105/01/2021
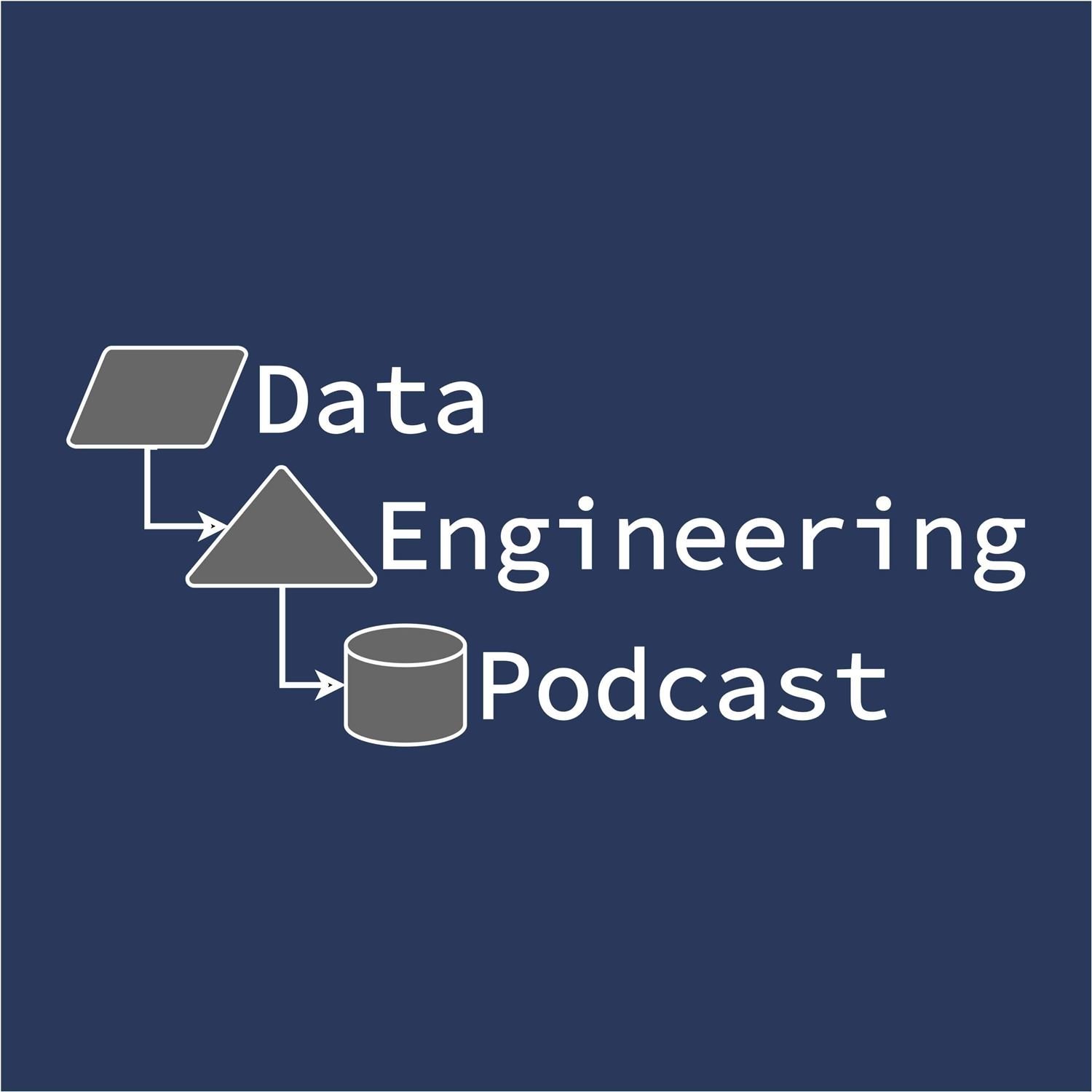
Off The Shelf Data Governance With Satori
Summary
One of the core responsibilities of data engineers is to manage the security of the information that they process. The team at Satori has a background in cybersecurity and they are using the lessons that they learned in that field to address the challenge of access control and auditing for data governance. In this episode co-founder and CTO Yoav Cohen explains how the Satori platform provides a proxy layer for your data, the challenges of managing security across disparate storage systems, and their approach to building a dynamic data catalog based on the records that your organization is actually using. This is an interesting conversation about the intersection of data and security and the lessons that can be learned in each direction.
Announcements
Hello and welcome to the Data Engineering Podcast, the show about modern data management
When you’re ready to build your next pipeline, or want to test out the projects you hear about on the show, you’ll need somewhere to deploy it, so check out our friends at Linode. With their managed Kubernetes platform it’s now even easier to deploy and scale your workflows, or try out the latest Helm charts from tools like Pulsar and Pachyderm. With simple pricing, fast networking, object storage, and worldwide data centers, you’ve got everything you need to run a bulletproof data platform. Go to dataengineeringpodcast.com/linode today and get a $60 credit to try out a Kubernetes cluster of your own. And don’t forget to thank them for their continued support of this show!
Your host is Tobias Macey and today I’m interviewing Yoav Cohen about Satori, a data access service to monitor, classify and control access to sensitive data
Interview
Introduction
How did you get involved in the area of data management?
Can you start by describing what you have built at Satori?
What is the story behind the product and company?
How does Satori compare to other tools and products for managing access control and governance for data assets?
What are the biggest challenges that organizations face in establishing and enforcing policies for their data?
What are the main goals for the Satori product and what use cases does it enable?
Can you describe how the Satori platform is architected?
How has the design of the platform evolved since you first began working on it?
How have your experiences working in cyber security informed your approach to data governance?
How does the design of the Satori platform simplify technical aspects of data governance?
What aspects of governance do you delegate to other systems or platforms?
What elements of data infrastructure does Satori integrate with?
For someone who is adopting Satori, what is involved in getting it deployed and set up with their existing data platforms?
What do you see as being the most complex or underserved aspects of data governance?
How much of that complexity is inherent to the problem vs. being a result of how the industry has evolved?
What are some of the most interesting, innovative, or unexpected ways that you have seen the Satori platform used?
What are the most interesting, unexpected, or challenging lessons that you have learned while building Satori?
When is Satori the wrong choice?
What do you have planned for the future of the platform?
Contact Info
LinkedIn
@yoavcohen on Twitter
Parting Question
From your perspective, what is the biggest gap in the tooling or technology for data management today?
Closing Announcements
Thank you for listening! Don’t forget to check out our other show, Podcast.__init__ to learn about the Python language, its community, and the innovative ways it is being used.
Visit the site to subscribe to the show, sign up for the mailing list, and read the show notes.
If you’ve learned something or tried out a project from the show then tell us about it! Email [email protected]) with your story.
To help other people find the show please leave a review on iTunes and tell your friends and co-workers
Join the community in the new Zulip chat workspace at dataengineeringpodcast.com/chat
Links
Satori
Data Governance
Data Masking
TLS == Transport Layer Security
The intro and outro music is from The Hug by The Freak Fandango Orchestra / CC BY-SA
Support Data Engineering Podcast
34:2428/12/2020
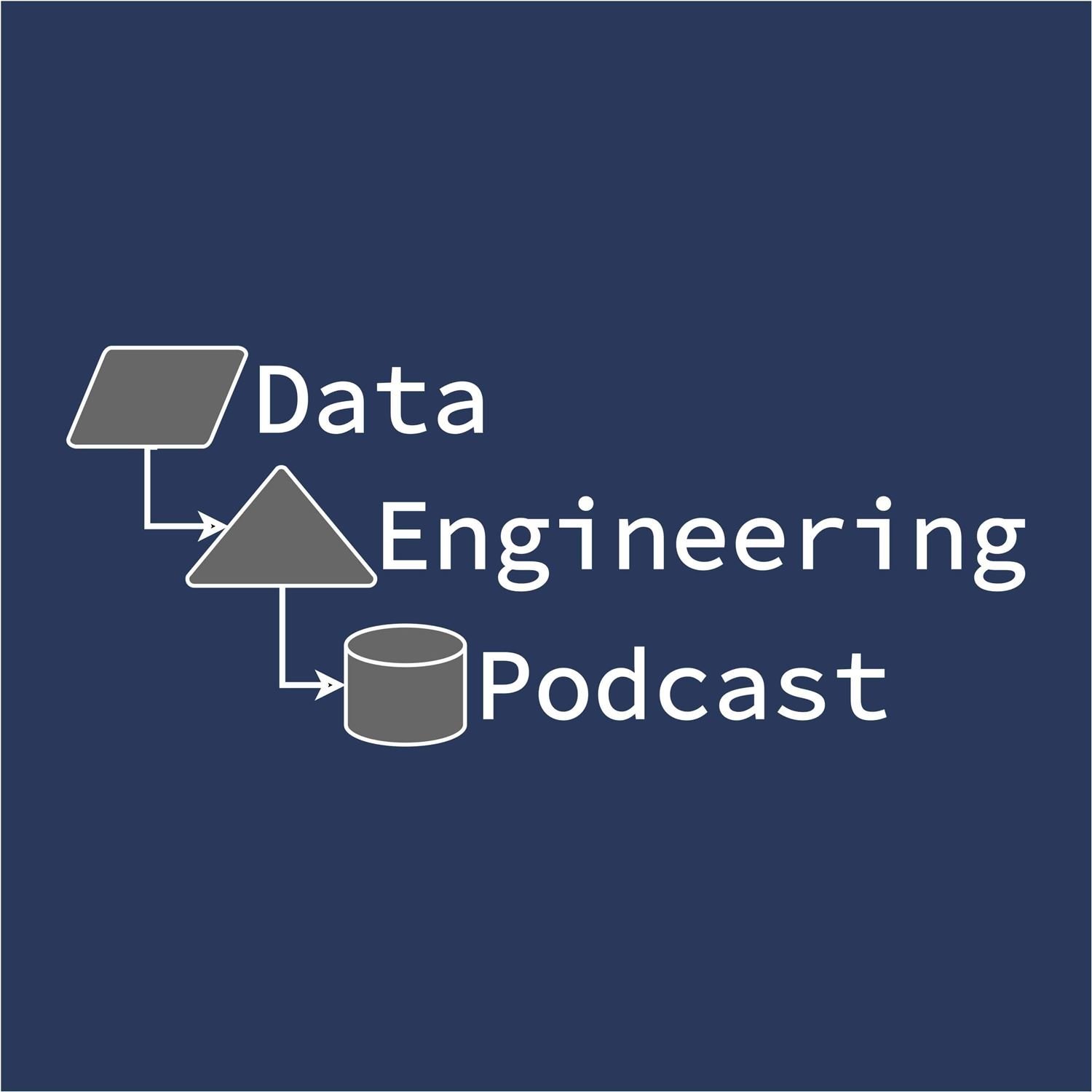
Low Friction Data Governance With Immuta
Summary
Data governance is a term that encompasses a wide range of responsibilities, both technical and process oriented. One of the more complex aspects is that of access control to the data assets that an organization is responsible for managing. The team at Immuta has built a platform that aims to tackle that problem in a flexible and maintainable fashion so that data teams can easily integrate authorization, data masking, and privacy enhancing technologies into their data infrastructure. In this episode Steve Touw and Stephen Bailey share what they have built at Immuta, how it is implemented, and how it streamlines the workflow for everyone involved in working with sensitive data. If you are starting down the path of implementing a data governance strategy then this episode will provide a great overview of what is involved.
Announcements
Hello and welcome to the Data Engineering Podcast, the show about modern data management
What are the pieces of advice that you wish you had received early in your career of data engineering? If you hand a book to a new data engineer, what wisdom would you add to it? I’m working with O’Reilly on a project to collect the 97 things that every data engineer should know, and I need your help. Go to dataengineeringpodcast.com/97things to add your voice and share your hard-earned expertise.
When you’re ready to build your next pipeline, or want to test out the projects you hear about on the show, you’ll need somewhere to deploy it, so check out our friends at Linode. With their managed Kubernetes platform it’s now even easier to deploy and scale your workflows, or try out the latest Helm charts from tools like Pulsar and Pachyderm. With simple pricing, fast networking, object storage, and worldwide data centers, you’ve got everything you need to run a bulletproof data platform. Go to dataengineeringpodcast.com/linode today and get a $60 credit to try out a Kubernetes cluster of your own. And don’t forget to thank them for their continued support of this show!
Feature flagging is a simple concept that enables you to ship faster, test in production, and do easy rollbacks without redeploying code. Teams using feature flags release new software with less risk, and release more often. ConfigCat is a feature flag service that lets you easily add flags to your Python code, and 9 other platforms. By adopting ConfigCat you and your manager can track and toggle your feature flags from their visual dashboard without redeploying any code or configuration, including granular targeting rules. You can roll out new features to a subset or your users for beta testing or canary deployments. With their simple API, clear documentation, and pricing that is independent of your team size you can get your first feature flags added in minutes without breaking the bank. Go to dataengineeringpodcast.com/configcat today to get 35% off any paid plan with code DATAENGINEERING or try out their free forever plan.
You invest so much in your data infrastructure – you simply can’t afford to settle for unreliable data. Fortunately, there’s hope: in the same way that New Relic, DataDog, and other Application Performance Management solutions ensure reliable software and keep application downtime at bay, Monte Carlo solves the costly problem of broken data pipelines. Monte Carlo’s end-to-end Data Observability Platform monitors and alerts for data issues across your data warehouses, data lakes, ETL, and business intelligence. The platform uses machine learning to infer and learn your data, proactively identify data issues, assess its impact through lineage, and notify those who need to know before it impacts the business. By empowering data teams with end-to-end data reliability, Monte Carlo helps organizations save time, increase revenue, and restore trust in their data. Visit dataengineeringpodcast.com/montecarlo today to request a demo and see how Monte Carlo delivers data observability across your data infrastructure. The first 25 will receive a free, limited edition Monte Carlo hat!
Your host is Tobias Macey and today I’m interviewing Steve Touw and Stephen Bailey about Immuta and how they work to automate data governance
Interview
Introduction
How did you get involved in the area of data management?
Can you start by describing what you have built at Immuta and your motivation for starting the company?
What is data governance?
How much of data governance can be solved with technology and how much is a matter of process and communication?
What does the current landscape of data governance solutions look like?
What are the motivating factors that would lead someone to choose Immuta as a component of their data governance strategy?
How does Immuta integrate with the broader ecosystem of data tools and platforms?
What other workflows or activities are necessary outside of Immuta to ensure a comprehensive governance/compliance strategy?
What are some of the common blind spots when it comes to data governance?
How is the Immuta platform architected?
How have the design and goals of the system evolved since you first started building it?
What is involved in adopting Immuta for an existing data platform?
Once an organization has integrated Immuta, what are the workflows for the different stakeholders of the data?
What are the biggest challenges in automated discovery/identification of sensitive data?
How does the evolution of what qualifies as sensitive complicate those efforts?
How do you approach the challenge of providing a unified interface for access control and auditing across different systems (e.g. BigQuery, Snowflake, RedShift, etc.)?
What are the complexities that creep into data masking?
What are some alternatives for obfuscating and managing access to sensitive information?
How do you handle managing access control/masking/tagging for derived data sets?
What are some of the most interesting, unexpected, or challenging lessons that you have learned while building Immuta?
When is Immuta the wrong choice?
What do you have planned for the future of the platform and business?
Contact Info
Steve
LinkedIn
@steve_touw on Twitter
Stephen
LinkedIn
Website
Parting Question
From your perspective, what is the biggest gap in the tooling or technology for data management today?
Closing Announcements
Thank you for listening! Don’t forget to check out our other show, Podcast.__init__ to learn about the Python language, its community, and the innovative ways it is being used.
Visit the site to subscribe to the show, sign up for the mailing list, and read the show notes.
If you’ve learned something or tried out a project from the show then tell us about it! Email [email protected]) with your story.
To help other people find the show please leave a review on iTunes and tell your friends and co-workers
Join the community in the new Zulip chat workspace at dataengineeringpodcast.com/chat
Links
Immuta
Data Governance
Data Catalog
Snowflake DB
Podcast Episode
Looker
Podcast Episode
Collibra
ABAC == Attribute Based Access Control
RBAC == Role Based Access Control
Paul Ohm: Broken Promises of Privacy
PET == Privacy Enhancing Technologies
K Anonymization
Differential Privacy
LDAP == Lightweight Directory Access Protocol
Active Directory
COVID Alliance
HIPAA
GDPR
CCPA
The intro and outro music is from The Hug by The Freak Fandango Orchestra / CC BY-SA
Support Data Engineering Podcast
53:3321/12/2020
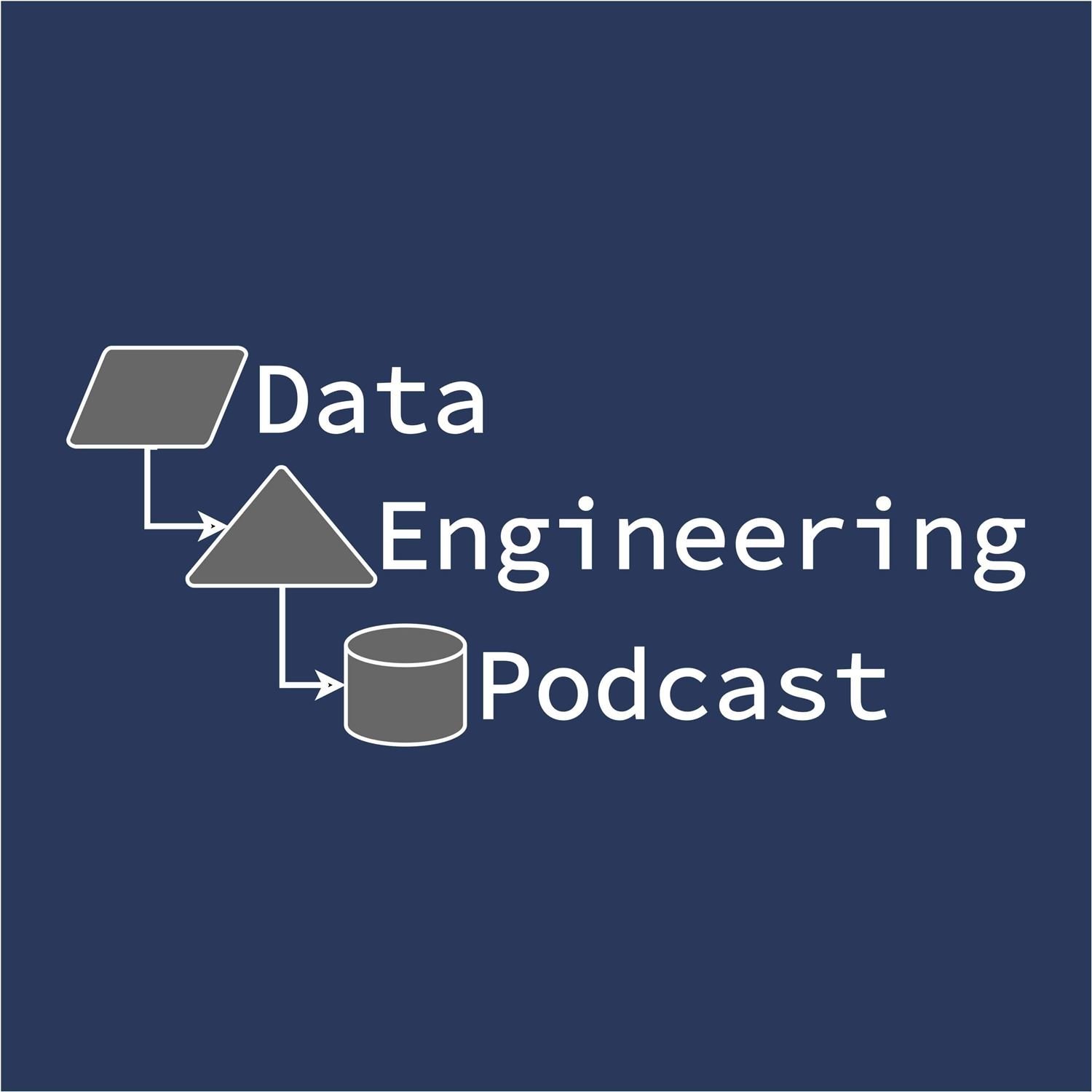
Building A Self Service Data Platform For Alternative Data Analytics At YipitData
Summary
As a data engineer you’re familiar with the process of collecting data from databases, customer data platforms, APIs, etc. At YipitData they rely on a variety of alternative data sources to inform investment decisions by hedge funds and businesses. In this episode Andrew Gross, Bobby Muldoon, and Anup Segu describe the self service data platform that they have built to allow data analysts to own the end-to-end delivery of data projects and how that has allowed them to scale their output. They share the journey that they went through to build a scalable and maintainable system for web scraping, how to make it reliable and resilient to errors, and the lessons that they learned in the process. This was a great conversation about real world experiences in building a successful data-oriented business.
Announcements
Hello and welcome to the Data Engineering Podcast, the show about modern data management
What are the pieces of advice that you wish you had received early in your career of data engineering? If you hand a book to a new data engineer, what wisdom would you add to it? I’m working with O’Reilly on a project to collect the 97 things that every data engineer should know, and I need your help. Go to dataengineeringpodcast.com/97things to add your voice and share your hard-earned expertise.
When you’re ready to build your next pipeline, or want to test out the projects you hear about on the show, you’ll need somewhere to deploy it, so check out our friends at Linode. With their managed Kubernetes platform it’s now even easier to deploy and scale your workflows, or try out the latest Helm charts from tools like Pulsar and Pachyderm. With simple pricing, fast networking, object storage, and worldwide data centers, you’ve got everything you need to run a bulletproof data platform. Go to dataengineeringpodcast.com/linode today and get a $60 credit to try out a Kubernetes cluster of your own. And don’t forget to thank them for their continued support of this show!
Are you bogged down by having to manually manage data access controls, repeatedly move and copy data, and create audit reports to prove compliance? How much time could you save if those tasks were automated across your cloud platforms? Immuta is an automated data governance solution that enables safe and easy data analytics in the cloud. Our comprehensive data-level security, auditing and de-identification features eliminate the need for time-consuming manual processes and our focus on data and compliance team collaboration empowers you to deliver quick and valuable data analytics on the most sensitive data to unlock the full potential of your cloud data platforms. Learn how we streamline and accelerate manual processes to help you derive real results from your data at dataengineeringpodcast.com/immuta.
Today’s episode of the Data Engineering Podcast is sponsored by Datadog, a SaaS-based monitoring and analytics platform for cloud-scale infrastructure, applications, logs, and more. Datadog uses machine-learning based algorithms to detect errors and anomalies across your entire stack—which reduces the time it takes to detect and address outages and helps promote collaboration between Data Engineering, Operations, and the rest of the company. Go to dataengineeringpodcast.com/datadog today to start your free 14 day trial. If you start a trial and install Datadog’s agent, Datadog will send you a free T-shirt.
Your host is Tobias Macey and today I’m interviewing Andrew Gross, Bobby Muldoon, and Anup Segu about they are building pipelines at Yipit Data
Interview
Introduction
How did you get involved in the area of data management?
Can you start by giving an overview of what YipitData does?
What kinds of data sources and data assets are you working with?
What is the composition of your data teams and how are they structured?
Given the use of your data products in the financial sector how do you handle monitoring and alerting around data quality?
For web scraping in particular, given how fragile it can be, what have you done to make it a reliable and repeatable part of the data pipeline?
Can you describe how your data platform is implemented?
How has the design of your platform and its goals evolved or changed?
What is your guiding principle for providing an approachable interface to analysts?
How much knowledge do your analysts require about the guarantees offered, and edge cases to be aware of in the underlying data and its processing?
What are some examples of specific tools that you have built to empower your analysts to own the full lifecycle of the data that they are working with?
Can you characterize or quantify the benefits that you have seen from training the analysts to work with the engineering tool chain?
What have been some of the most interesting, unexpected, or surprising outcomes of how you are approaching the different responsibilities and levels of ownership in your data organization?
What are some of the most interesting, unexpected, or challenging lessons that you have learned from building out the platform, tooling, and organizational structure for creating data products at Yipit?
What advice or recommendations do you have for other leaders of data teams about how to think about the organizational and technical aspects of managing the lifecycle of data projects?
Contact Info
Andrew
LinkedIn
@awgross on Twitter
Bobby
LinkedIn
@TheDooner64
Anup
LinkedIn
anup-segu on GitHub
Parting Question
From your perspective, what is the biggest gap in the tooling or technology for data management today?
Links
Yipit Data
Redshift
MySQL
Airflow
Databricks
Groupon
Living Social
Web Scraping
Podcast.__init__ Episode
Readypipe
Graphite
Podcast.init Episode
AWS Kinesis Firehose
Parquet
Papermill
Podcast Episode About Notebooks At Netflix
Fivetran
Podcast Episode
The intro and outro music is from The Hug by The Freak Fandango Orchestra / CC BY-SA
Support Data Engineering Podcast
01:04:4715/12/2020
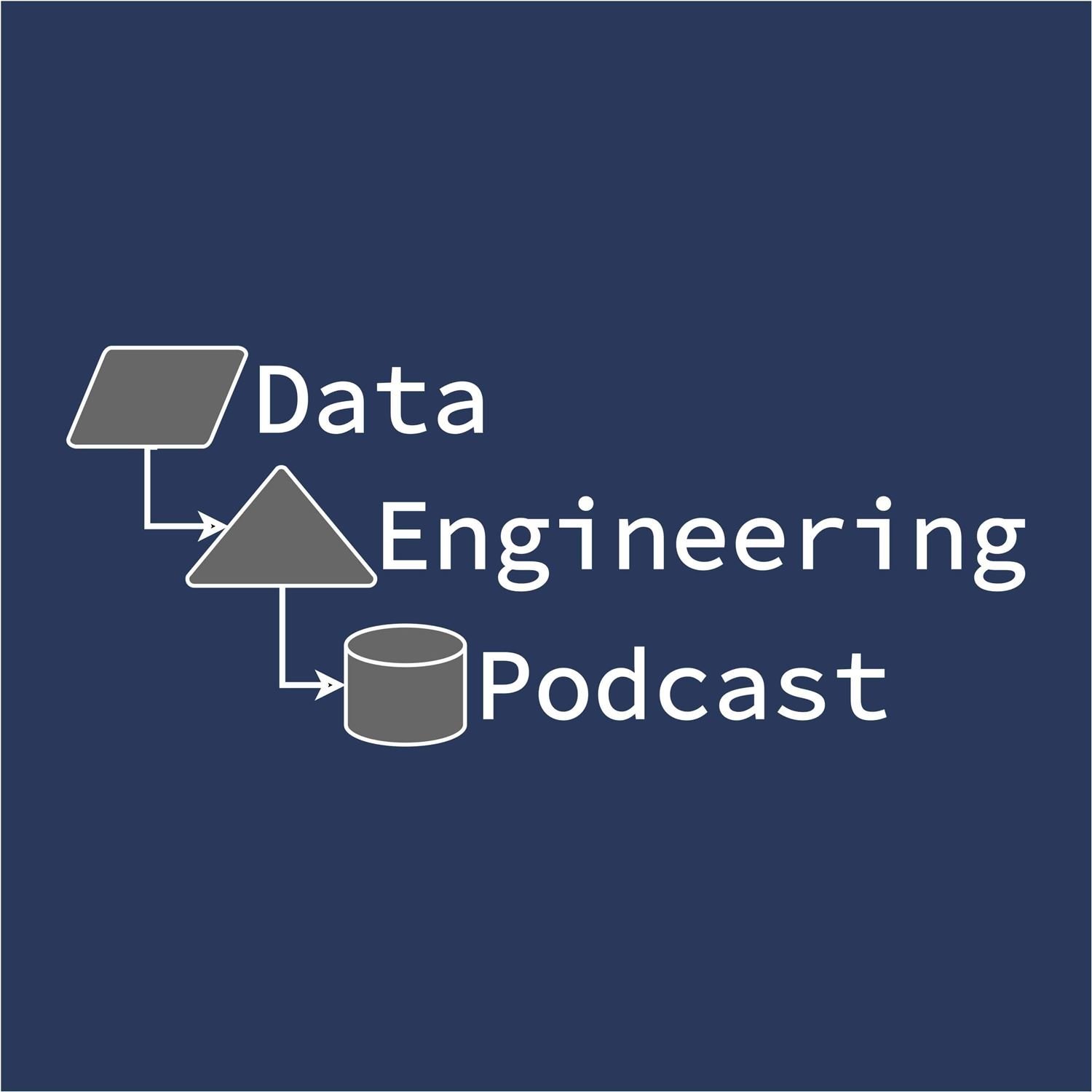
Proven Patterns For Building Successful Data Teams
Summary
Building data products are complicated by the fact that there are so many different stakeholders with competing goals and priorities. It is also challenging because of the number of roles and capabilities that are necessary to go from idea to delivery. Different organizations have tried a multitude of organizational strategies to improve the success rate of these data teams with varying levels of success. In this episode Jesse Anderson shares the lessons that he has learned while working with dozens of businesses across industries to determine the team structures and communication styles that have generated the best results. If you are struggling to deliver value from big data, or just starting down the path of building the organizational capacity to turn raw information into valuable products then this is a conversation that you don’t want to miss.
Announcements
Hello and welcome to the Data Engineering Podcast, the show about modern data management
What are the pieces of advice that you wish you had received early in your career of data engineering? If you hand a book to a new data engineer, what wisdom would you add to it? I’m working with O’Reilly on a project to collect the 97 things that every data engineer should know, and I need your help. Go to dataengineeringpodcast.com/97things to add your voice and share your hard-earned expertise.
When you’re ready to build your next pipeline, or want to test out the projects you hear about on the show, you’ll need somewhere to deploy it, so check out our friends at Linode. With their managed Kubernetes platform it’s now even easier to deploy and scale your workflows, or try out the latest Helm charts from tools like Pulsar and Pachyderm. With simple pricing, fast networking, object storage, and worldwide data centers, you’ve got everything you need to run a bulletproof data platform. Go to dataengineeringpodcast.com/linode today and get a $60 credit to try out a Kubernetes cluster of your own. And don’t forget to thank them for their continued support of this show!
Are you bogged down by having to manually manage data access controls, repeatedly move and copy data, and create audit reports to prove compliance? How much time could you save if those tasks were automated across your cloud platforms? Immuta is an automated data governance solution that enables safe and easy data analytics in the cloud. Our comprehensive data-level security, auditing and de-identification features eliminate the need for time-consuming manual processes and our focus on data and compliance team collaboration empowers you to deliver quick and valuable data analytics on the most sensitive data to unlock the full potential of your cloud data platforms. Learn how we streamline and accelerate manual processes to help you derive real results from your data at dataengineeringpodcast.com/immuta.
Today’s episode of the Data Engineering Podcast is sponsored by Datadog, a SaaS-based monitoring and analytics platform for cloud-scale infrastructure, applications, logs, and more. Datadog uses machine-learning based algorithms to detect errors and anomalies across your entire stack—which reduces the time it takes to detect and address outages and helps promote collaboration between Data Engineering, Operations, and the rest of the company. Go to dataengineeringpodcast.com/datadog today to start your free 14 day trial. If you start a trial and install Datadog’s agent, Datadog will send you a free T-shirt.
Your host is Tobias Macey and today I’m interviewing Jesse Anderson about best practices for organizing and managing data teams
Interview
Introduction
How did you get involved in the area of data management?
Can you start by giving an overview of how you view the mission and responsibilities of a data team?
What are the critical elements of a successful data team?
Beyond the core pillars of data science, data engineering, and operations, what other specialized roles do you find helpful for larger or more sophisticated teams?
For organizations that have "small data", how does that change the necessary composition of roles for successful data projects?
What are the signs and symptoms that point to the need for a dedicated team that focuses on data?
With data scientists and data engineers in particular being in such high demand, what are strategies that you have found effective for attracting new talent?
In the case where you have engineers on staff, how do you identify internal talent that can be trained into these specialized roles?
Another challenge that organizations face in dealing with data is how the team is organized. What are your thoughts on effective strategies for how to structure the communication and reporting structures of data teams? (e.g. centralized, embedded, etc.)
How do you recommend evaluating potential candidates for each of the necessary roles?
What are your thoughts on when to hire an outside consultant, vs building internal capacity?
For managers who are responsible for data teams, how much understanding of data and analytics do they need to be effective?
How do you define success or measure performance of a team focused on working with data?
What are some of the anti-patterns that you have seen in managers who oversee data professionals?
What are some of the most interesting, unexpected, or challenging lessons that you have learned in the process of helping organizations and individuals achieve success in data and analytics?
What advice or additional resources do you have for anyone who is interested in learning more about how to build and grow a successful data team?
Contact Info
Website
@jessetanderson on Twitter
Parting Question
From your perspective, what is the biggest gap in the tooling or technology for data management today?
Closing Announcements
Thank you for listening! Don’t forget to check out our other show, Podcast.__init__ to learn about the Python language, its community, and the innovative ways it is being used.
Visit the site to subscribe to the show, sign up for the mailing list, and read the show notes.
If you’ve learned something or tried out a project from the show then tell us about it! Email [email protected]) with your story.
To help other people find the show please leave a review on iTunes and tell your friends and co-workers
Join the community in the new Zulip chat workspace at dataengineeringpodcast.com/chat
Links
Data Teams Book
DBA == Database Administrator
ML Engineer
DataOps
Three Vs
The Ultimate Guide To Switching Careers To Big Data
S-1 Report
Jesse Anderson’s Youtube Channel
Video about interviewing for data teams
Uber Data Infrastructure Progression Blog Post
The intro and outro music is from The Hug by The Freak Fandango Orchestra / CC BY-SA
Support Data Engineering Podcast
01:12:3107/12/2020
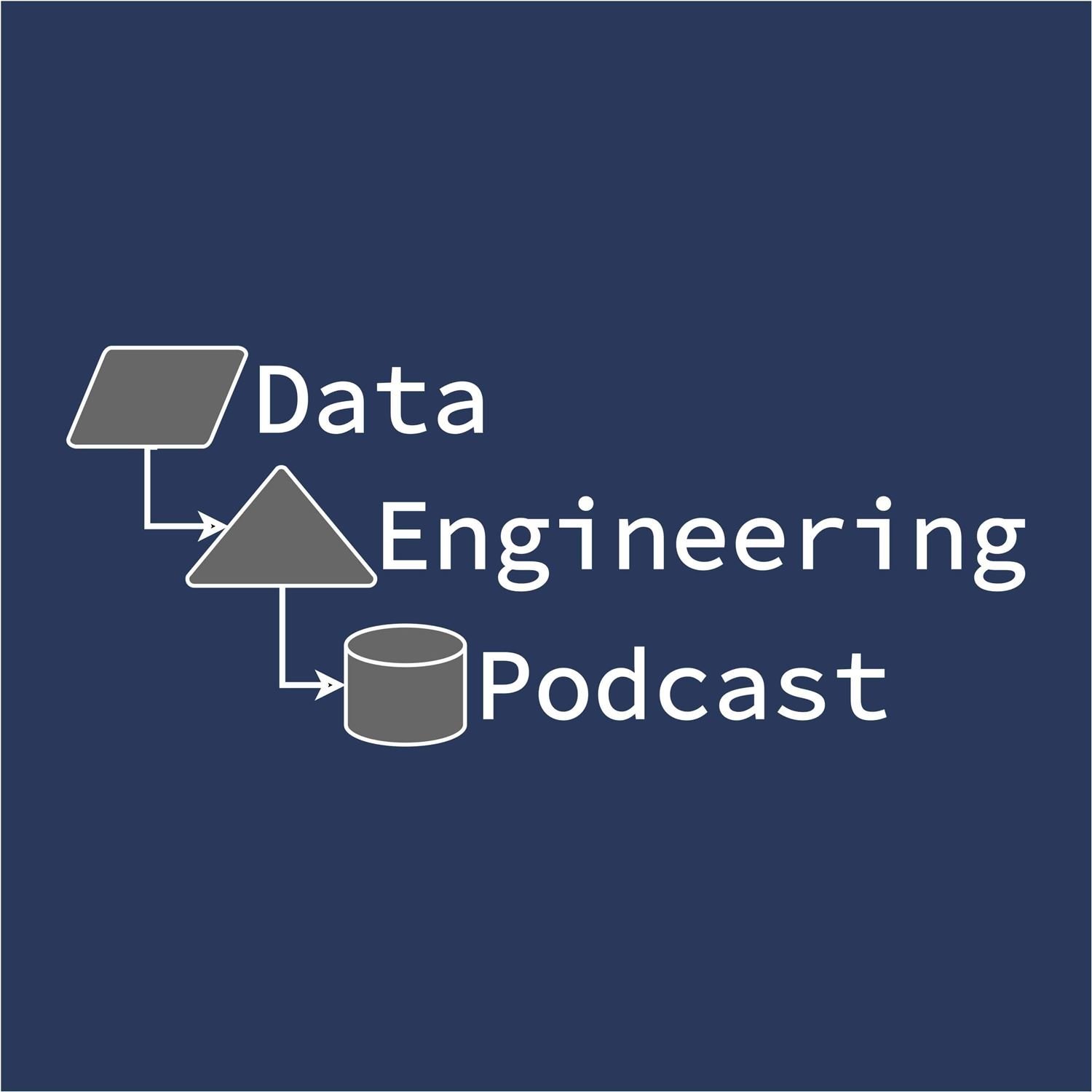
Streaming Data Integration Without The Code at Equalum
Summary
The first stage of every good pipeline is to perform data integration. With the increasing pace of change and the need for up to date analytics the need to integrate that data in near real time is growing. With the improvements and increased variety of options for streaming data engines and improved tools for change data capture it is possible for data teams to make that goal a reality. However, despite all of the tools and managed distributions of those streaming engines it is still a challenge to build a robust and reliable pipeline for streaming data integration, especially if you need to expose those capabilities to non-engineers. In this episode Ido Friedman, CTO of Equalum, explains how they have built a no-code platform to make integration of streaming data and change data capture feeds easier to manage. He discusses the challenges that are inherent in the current state of CDC technologies, how they have architected their system to integrate well with existing data platforms, and how to build an appropriate level of abstraction for such a complex problem domain. If you are struggling with streaming data integration and change data capture then this interview is definitely worth a listen.
Announcements
Hello and welcome to the Data Engineering Podcast, the show about modern data management
What are the pieces of advice that you wish you had received early in your career of data engineering? If you hand a book to a new data engineer, what wisdom would you add to it? I’m working with O’Reilly on a project to collect the 97 things that every data engineer should know, and I need your help. Go to dataengineeringpodcast.com/97things to add your voice and share your hard-earned expertise.
When you’re ready to build your next pipeline, or want to test out the projects you hear about on the show, you’ll need somewhere to deploy it, so check out our friends at Linode. With their managed Kubernetes platform it’s now even easier to deploy and scale your workflows, or try out the latest Helm charts from tools like Pulsar and Pachyderm. With simple pricing, fast networking, object storage, and worldwide data centers, you’ve got everything you need to run a bulletproof data platform. Go to dataengineeringpodcast.com/linode today and get a $60 credit to try out a Kubernetes cluster of your own. And don’t forget to thank them for their continued support of this show!
Modern Data teams are dealing with a lot of complexity in their data pipelines and analytical code. Monitoring data quality, tracing incidents, and testing changes can be daunting and often takes hours to days. Datafold helps Data teams gain visibility and confidence in the quality of their analytical data through data profiling, column-level lineage and intelligent anomaly detection. Datafold also helps automate regression testing of ETL code with its Data Diff feature that instantly shows how a change in ETL or BI code affects the produced data, both on a statistical level and down to individual rows and values. Datafold integrates with all major data warehouses as well as frameworks such as Airflow & dbt and seamlessly plugs into CI workflows. Go to dataengineeringpodcast.com/datafold today to start a 30-day trial of Datafold. Once you sign up and create an alert in Datafold for your company data, they will send you a cool water flask.
Are you bogged down by having to manually manage data access controls, repeatedly move and copy data, and create audit reports to prove compliance? How much time could you save if those tasks were automated across your cloud platforms? Immuta is an automated data governance solution that enables safe and easy data analytics in the cloud. Our comprehensive data-level security, auditing and de-identification features eliminate the need for time-consuming manual processes and our focus on data and compliance team collaboration empowers you to deliver quick and valuable data analytics on the most sensitive data to unlock the full potential of your cloud data platforms. Learn how we streamline and accelerate manual processes to help you derive real results from your data at dataengineeringpodcast.com/immuta.
Your host is Tobias Macey and today I’m interviewing Ido Friedman about Equalum, a no-code platform for streaming data integration
Interview
Introduction
How did you get involved in the area of data management?
Can you start by giving an overview of what you are building at Equalum and how it got started?
There are a number of projects and platforms on the market that target data integration. Can you give some context of how Equalum fits in that market and the differentiating factors that engineers should consider?
What components of the data ecosystem might Equalum replace, and which are you designed to integrate with?
Can you walk through the workflow for someone who is using Equalum for a simple data integration use case?
What options are available for doing in-flight transformations of data or creating customized routing rules?
How do you handle versioning and staged rollouts of changes to pipelines?
How is the Equalum platform implemented?
How has the design and architecture of Equalum evolved since it was first created?
What have you found to be the most complex or challenging aspects of building the platform?
Change data capture is a growing area of interest, with a significant level of difficulty in implementing well. How do you handle support for the variety of different sources that customers are working with?
What are the edge cases that you typically run into when working with changes in databases?
How do you approach the user experience of the platform given its focus as a low code/no code system?
What options exist for sophisticated users to create custom operations?
How much of the underlying concerns do you surface to end users, and how much are you able to hide?
What is the process for a customer to integrate Equalum into their existing infrastructure and data systems?
What are some of the most interesting, unexpected, or innovative ways that you have seen Equalum used?
What are the most interesting, unexpected, or challenging lessons that you have learned while building and growing the Equalum platform?
When is Equalum the wrong choice?
What do you have planned for the future of Equalum?
Contact Info
LinkedIn
Parting Question
From your perspective, what is the biggest gap in the tooling or technology for data management today?
Closing Announcements
Thank you for listening! Don’t forget to check out our other show, Podcast.__init__ to learn about the Python language, its community, and the innovative ways it is being used.
Visit the site to subscribe to the show, sign up for the mailing list, and read the show notes.
If you’ve learned something or tried out a project from the show then tell us about it! Email [email protected]) with your story.
To help other people find the show please leave a review on iTunes and tell your friends and co-workers
Join the community in the new Zulip chat workspace at dataengineeringpodcast.com/chat
Links
Equalum
Change Data Capture
Debezium Podcast Episode
SQL Server
DBA == Database Administrator
Fivetran
Podcast Episode
Singer
Pentaho
EMR
Snowflake
Podcast Episode
S3
Kafka
Spark
Prometheus
Grafana
Logminer
OBLP == Oracle Binary Log Parser
Ansible
Terraform
Jupyter Notebooks
Papermill
The intro and outro music is from The Hug by The Freak Fandango Orchestra / CC BY-SA
Support Data Engineering Podcast
44:5030/11/2020
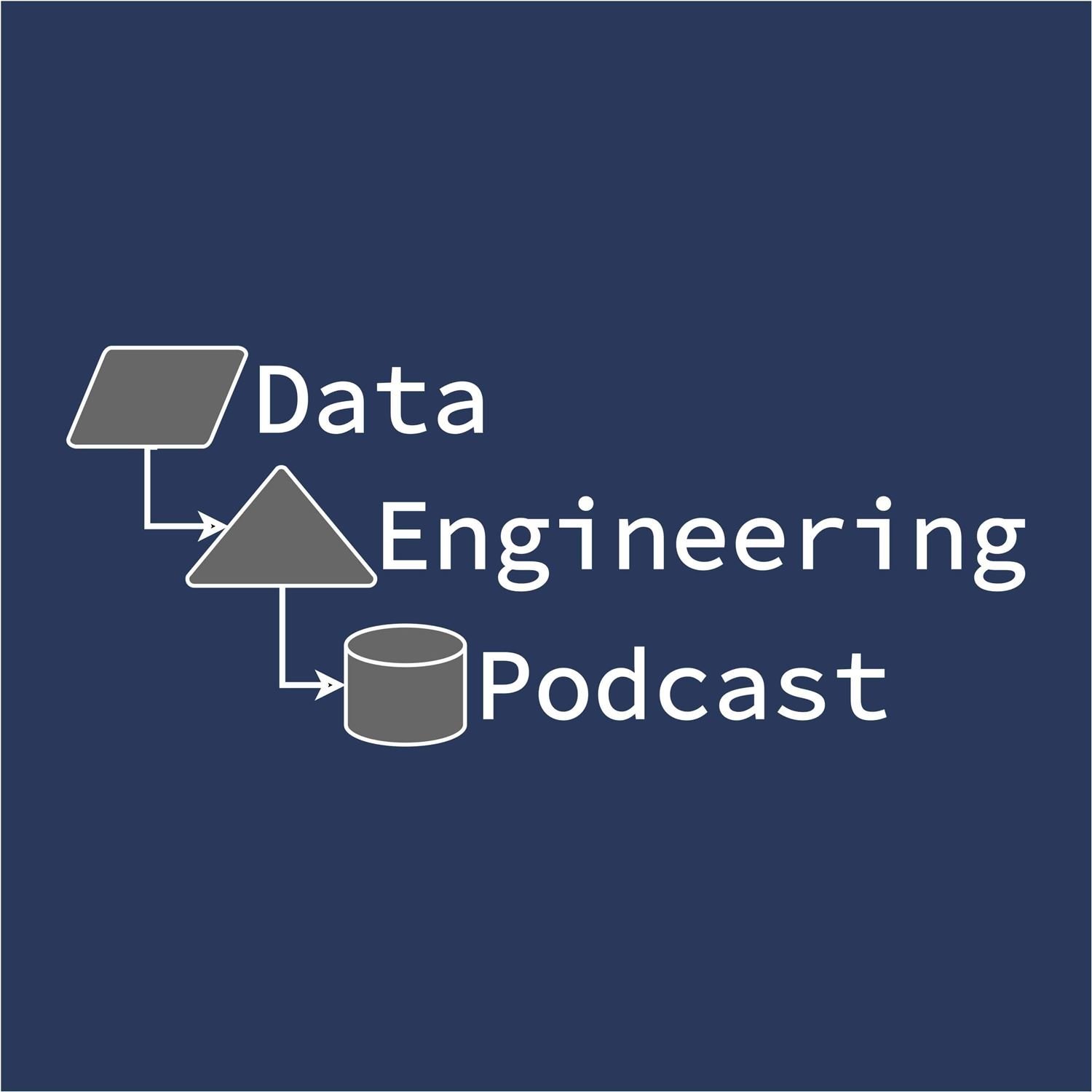
Keeping A Bigeye On The Data Quality Market
Summary
One of the oldest aphorisms about data is "garbage in, garbage out", which is why the current boom in data quality solutions is no surprise. With the growth in projects, platforms, and services that aim to help you establish and maintain control of the health and reliability of your data pipelines it can be overwhelming to stay up to date with how they all compare. In this episode Egor Gryaznov, CTO of Bigeye, joins the show to explore the landscape of data quality companies, the general strategies that they are using, and what problems they solve. He also shares how his own product is designed and the challenges that are involved in building a system to help data engineers manage the complexity of a data platform. If you are wondering how to get better control of your own pipelines and the traps to avoid then this episode is definitely worth a listen.
Announcements
Hello and welcome to the Data Engineering Podcast, the show about modern data management
What are the pieces of advice that you wish you had received early in your career of data engineering? If you hand a book to a new data engineer, what wisdom would you add to it? I’m working with O’Reilly on a project to collect the 97 things that every data engineer should know, and I need your help. Go to dataengineeringpodcast.com/97things to add your voice and share your hard-earned expertise.
When you’re ready to build your next pipeline, or want to test out the projects you hear about on the show, you’ll need somewhere to deploy it, so check out our friends at Linode. With their managed Kubernetes platform it’s now even easier to deploy and scale your workflows, or try out the latest Helm charts from tools like Pulsar and Pachyderm. With simple pricing, fast networking, object storage, and worldwide data centers, you’ve got everything you need to run a bulletproof data platform. Go to dataengineeringpodcast.com/linode today and get a $60 credit to try out a Kubernetes cluster of your own. And don’t forget to thank them for their continued support of this show!
Modern Data teams are dealing with a lot of complexity in their data pipelines and analytical code. Monitoring data quality, tracing incidents, and testing changes can be daunting and often takes hours to days. Datafold helps Data teams gain visibility and confidence in the quality of their analytical data through data profiling, column-level lineage and intelligent anomaly detection. Datafold also helps automate regression testing of ETL code with its Data Diff feature that instantly shows how a change in ETL or BI code affects the produced data, both on a statistical level and down to individual rows and values. Datafold integrates with all major data warehouses as well as frameworks such as Airflow & dbt and seamlessly plugs into CI workflows. Go to dataengineeringpodcast.com/datafold today to start a 30-day trial of Datafold. Once you sign up and create an alert in Datafold for your company data, they will send you a cool water flask.
Are you bogged down by having to manually manage data access controls, repeatedly move and copy data, and create audit reports to prove compliance? How much time could you save if those tasks were automated across your cloud platforms? Immuta is an automated data governance solution that enables safe and easy data analytics in the cloud. Our comprehensive data-level security, auditing and de-identification features eliminate the need for time-consuming manual processes and our focus on data and compliance team collaboration empowers you to deliver quick and valuable data analytics on the most sensitive data to unlock the full potential of your cloud data platforms. Learn how we streamline and accelerate manual processes to help you derive real results from your data at dataengineeringpodcast.com/immuta.
Your host is Tobias Macey and today I’m interviewing Egor Gryaznov about the state of the industry for data quality management and what he is building at Bigeye.
Interview
Introduction
How did you get involved in the area of data management?
Can you start by sharing your views on what attributes you consider when defining data quality?
You use the term "data semantics" – can you elaborate on what that means?
What are the driving factors that contribute to the presence or lack of data quality in an organization or data platform?
Why do you think now is the right time to focus on data quality as an industry?
What are you building at Bigeye and how did it get started?
How does Bigeye help teams understand and manage their data quality?
What is the difference between existing data quality approaches and data observability?
What do you see as the tradeoffs for the approach that you are taking at Bigeye?
What are the most common data quality issues that you’ve seen and what are some more interesting ones that you wouldn’t expect?
Where do you see Bigeye fitting into the data management landscape? What are alternatives to Bigeye?
What are some of the most interesting, innovative, or unexpected ways that you have seen Bigeye being used?
What are some of the most interesting homegrown approaches that you have seen?
What have you found to be the most interesting, unexpected, or challenging lessons that you have learned while building the Bigeye platform and business?
What are the biggest trends you’re following in data quality management?
When is Bigeye the wrong choice?
What do you see in store for the future of Bigeye?
Contact Info
You can email Egor about anything data
LinkedIn
Parting Question
From your perspective, what is the biggest gap in the tooling or technology for data management today?
Closing Announcements
Thank you for listening! Don’t forget to check out our other show, Podcast.__init__ to learn about the Python language, its community, and the innovative ways it is being used.
Visit the site to subscribe to the show, sign up for the mailing list, and read the show notes.
If you’ve learned something or tried out a project from the show then tell us about it! Email [email protected]) with your story.
To help other people find the show please leave a review on iTunes and tell your friends and co-workers
Join the community in the new Zulip chat workspace at dataengineeringpodcast.com/chat
Links
Bigeye
Uber
A/B Testing
Hadoop
MapReduce
Apache Impala
One King’s Lane
Vertica
Mode
Tableau
Jupyter Notebooks
Redshift
Snowflake
PyTorch
Podcast.__init__ Episode
Tensorflow
DataOps
DevOps
Data Catalog
DBT
Podcast Episode
SRE Handbook
Article About How Uber Applied SRE Principles to Data
SLA == Service Level Agreement
SLO == Service Level Objective
Dagster
Podcast Episode
Podcast.__init__ Episode
Delta Lake
Great Expectations
Podcast Episode
Podcast.__init__ Episode
Amundsen
Podcast Episode
Alation
Collibra
The intro and outro music is from The Hug by The Freak Fandango Orchestra / CC BY-SA
Support Data Engineering Podcast
49:2623/11/2020
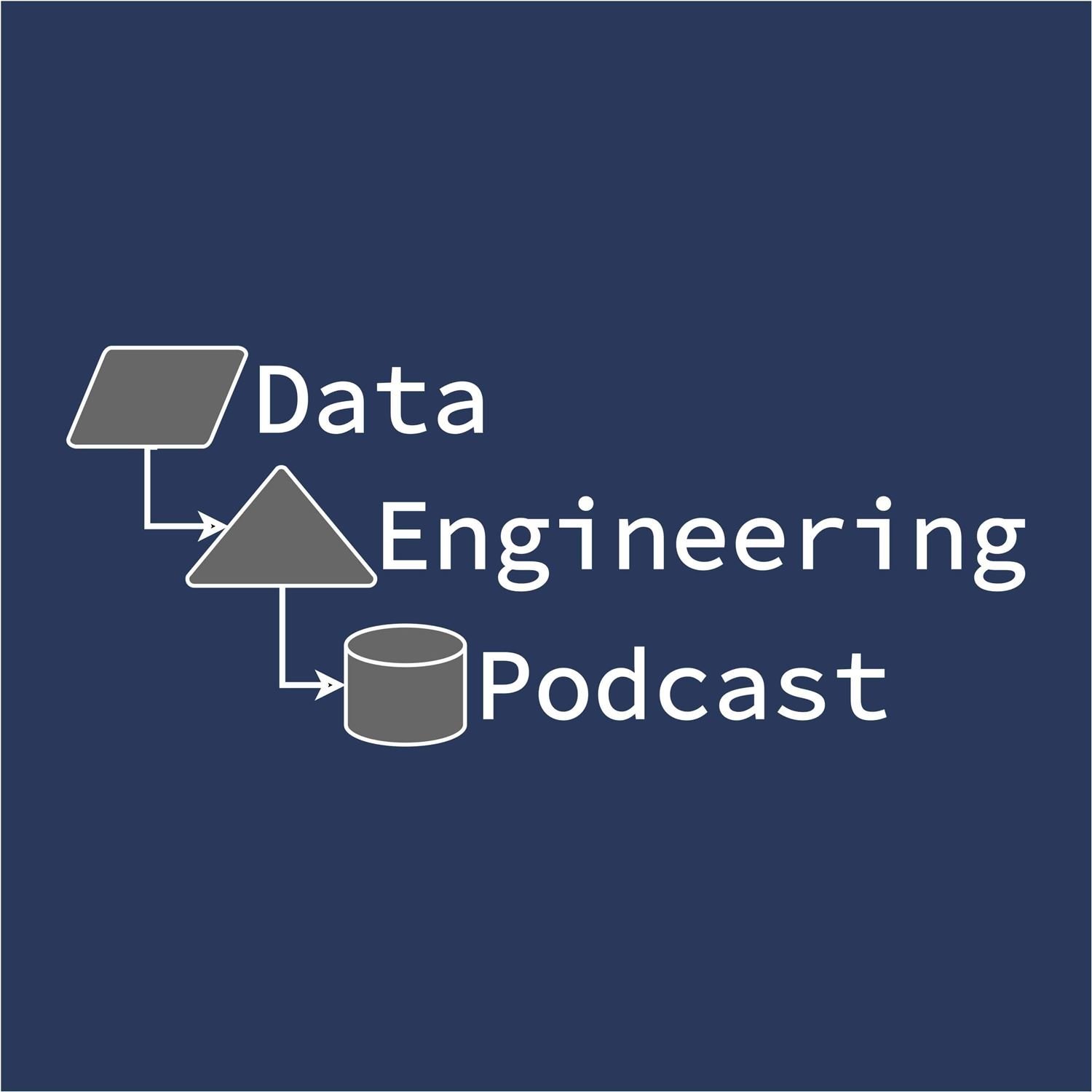
Self Service Data Management From Ingest To Insights With Isima
Summary
The core mission of data engineers is to provide the business with a way to ask and answer questions of their data. This often takes the form of business intelligence dashboards, machine learning models, or APIs on top of a cleaned and curated data set. Despite the rapid progression of impressive tools and products built to fulfill this mission, it is still an uphill battle to tie everything together into a cohesive and reliable platform. At Isima they decided to reimagine the entire ecosystem from the ground up and built a single unified platform to allow end-to-end self service workflows from data ingestion through to analysis. In this episode CEO and co-founder of Isima Darshan Rawal explains how the biOS platform is architected to enable ease of use, the challenges that were involved in building an entirely new system from scratch, and how it can integrate with the rest of your data platform to allow for incremental adoption. This was an interesting and contrarian take on the current state of the data management industry and is worth a listen to gain some additional perspective.
Announcements
Hello and welcome to the Data Engineering Podcast, the show about modern data management
What are the pieces of advice that you wish you had received early in your career of data engineering? If you hand a book to a new data engineer, what wisdom would you add to it? I’m working with O’Reilly on a project to collect the 97 things that every data engineer should know, and I need your help. Go to dataengineeringpodcast.com/97things to add your voice and share your hard-earned expertise.
When you’re ready to build your next pipeline, or want to test out the projects you hear about on the show, you’ll need somewhere to deploy it, so check out our friends at Linode. With their managed Kubernetes platform it’s now even easier to deploy and scale your workflows, or try out the latest Helm charts from tools like Pulsar and Pachyderm. With simple pricing, fast networking, object storage, and worldwide data centers, you’ve got everything you need to run a bulletproof data platform. Go to dataengineeringpodcast.com/linode today and get a $60 credit to try out a Kubernetes cluster of your own. And don’t forget to thank them for their continued support of this show!
Modern Data teams are dealing with a lot of complexity in their data pipelines and analytical code. Monitoring data quality, tracing incidents, and testing changes can be daunting and often takes hours to days. Datafold helps Data teams gain visibility and confidence in the quality of their analytical data through data profiling, column-level lineage and intelligent anomaly detection. Datafold also helps automate regression testing of ETL code with its Data Diff feature that instantly shows how a change in ETL or BI code affects the produced data, both on a statistical level and down to individual rows and values. Datafold integrates with all major data warehouses as well as frameworks such as Airflow & dbt and seamlessly plugs into CI workflows. Follow go.datafold.com/dataengineeringpodcast to start a 30-day trial of Datafold. Once you sign up and create an alert in Datafold for your company data, they will send you a cool water flask.
Are you bogged down by having to manually manage data access controls, repeatedly move and copy data, and create audit reports to prove compliance? How much time could you save if those tasks were automated across your cloud platforms? Immuta is an automated data governance solution that enables safe and easy data analytics in the cloud. Our comprehensive data-level security, auditing and de-identification features eliminate the need for time-consuming manual processes and our focus on data and compliance team collaboration empowers you to deliver quick and valuable data analytics on the most sensitive data to unlock the full potential of your cloud data platforms. Learn how we streamline and accelerate manual processes to help you derive real results from your data at dataengineeringpodcast.com/immuta.
Your host is Tobias Macey and today I’m interviewing Darshan Rawal about Îsíma, a unified platform for building data applications
Interview
Introduction
How did you get involved in the area of data management?
Can you start by giving an overview of what you are building at Îsíma?
What was your motivation for creating a new platform for data applications?
What is the story behind the name?
What are the tradeoffs of a fully integrated platform vs a modular approach?
What components of the data ecosystem does Isima replace, and which does it integrate with?
What are the use cases that Isima enables which were previously impractical?
Can you describe how Isima is architected?
How has the design of the platform changed or evolved since you first began working on it?
What were your initial ideas or assumptions that have been changed or invalidated as you worked through the problem you’re addressing?
With a focus on the enterprise, how did you approach the user experience design to allow for organizational complexity?
One of the biggest areas of difficulty that many data systems face is security and scaleable access control. How do you tackle that problem in your platform?
How did you address the issue of geographical distribution of data and users?
Can you talk through the overall lifecycle of data as it traverses the bi(OS) platform from ingestion through to presentation?
What is the workflow for someone using bi(OS)?
What are some of the most interesting, innovative, or unexpected ways that you have seen bi(OS) used?
What have you found to be the most interesting, unexpected, or challenging aspects of building the bi(OS) platform?
When is it the wrong choice?
What do you have planned for the future of Isima and bi(OS)?
Contact Info
LinkedIn
Parting Question
From your perspective, what is the biggest gap in the tooling or technology for data management today?
Closing Announcements
Thank you for listening! Don’t forget to check out our other show, Podcast.__init__ to learn about the Python language, its community, and the innovative ways it is being used.
Visit the site to subscribe to the show, sign up for the mailing list, and read the show notes.
If you’ve learned something or tried out a project from the show then tell us about it! Email [email protected]) with your story.
To help other people find the show please leave a review on iTunes and tell your friends and co-workers
Join the community in the new Zulip chat workspace at dataengineeringpodcast.com/chat
Links
Îsíma
Datastax
Verizon
AT&T
Click Fraud
ESB == Enterprise Service Bus
ETL == Extract, Transform, Load
EDW == Enterprise Data Warehouse
BI == Business Intelligence
The intro and outro music is from The Hug by The Freak Fandango Orchestra / CC BY-SA
Support Data Engineering Podcast
44:0317/11/2020
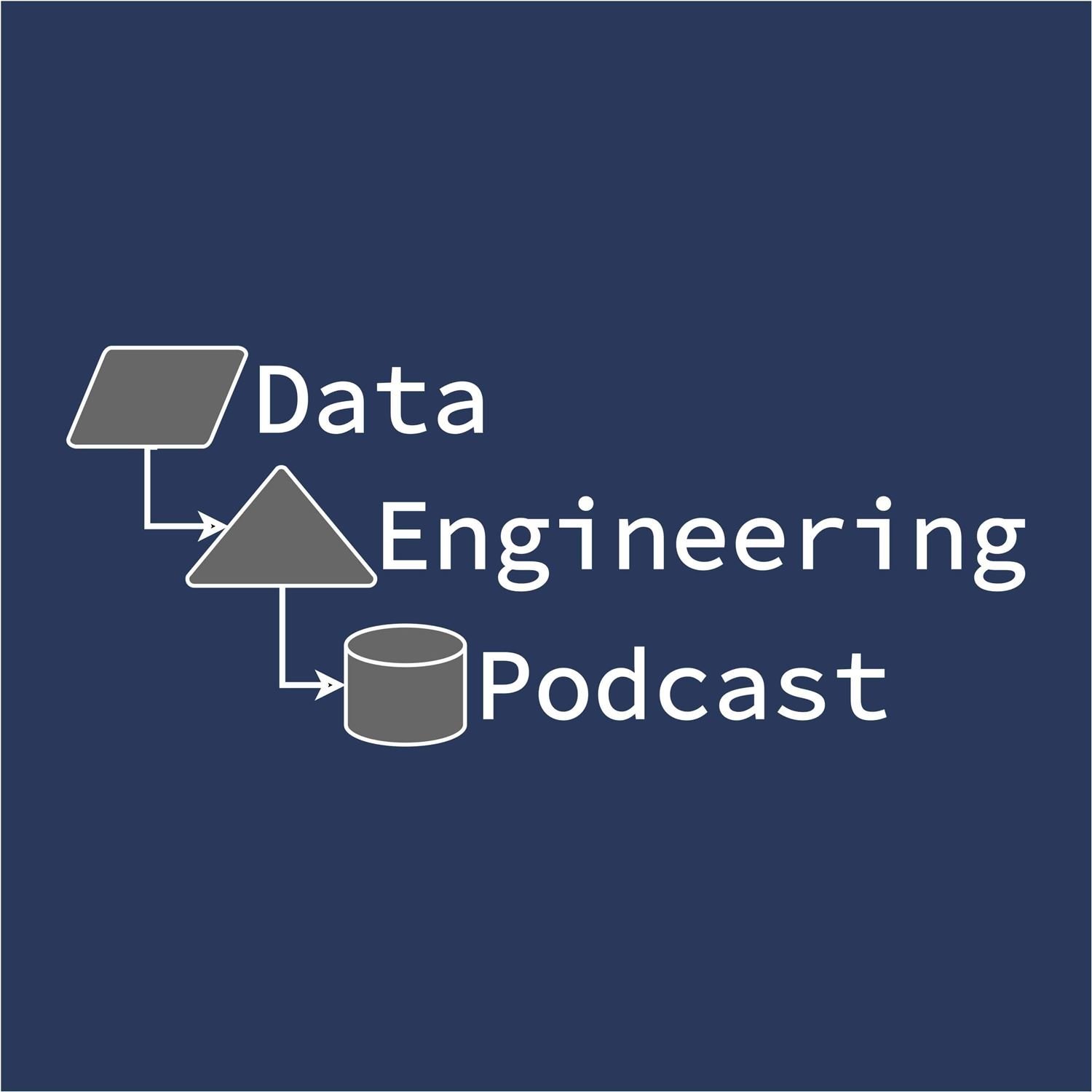
Building A Cost Effective Data Catalog With Tree Schema
Summary
A data catalog is a critical piece of infrastructure for any organization who wants to build analytics products, whether internal or external. While there are a number of platforms available for building that catalog, many of them are either difficult to deploy and integrate, or expensive to use at scale. In this episode Grant Seward explains how he built Tree Schema to be an easy to use and cost effective option for organizations to build their data catalogs. He also shares the internal architecture, how he approached the design to make it accessible and easy to use, and how it autodiscovers the schemas and metadata for your source systems.
Announcements
Hello and welcome to the Data Engineering Podcast, the show about modern data management
What are the pieces of advice that you wish you had received early in your career of data engineering? If you hand a book to a new data engineer, what wisdom would you add to it? I’m working with O’Reilly on a project to collect the 97 things that every data engineer should know, and I need your help. Go to dataengineeringpodcast.com/97things to add your voice and share your hard-earned expertise.
When you’re ready to build your next pipeline, or want to test out the projects you hear about on the show, you’ll need somewhere to deploy it, so check out our friends at Linode. With their managed Kubernetes platform it’s now even easier to deploy and scale your workflows, or try out the latest Helm charts from tools like Pulsar and Pachyderm. With simple pricing, fast networking, object storage, and worldwide data centers, you’ve got everything you need to run a bulletproof data platform. Go to dataengineeringpodcast.com/linode today and get a $60 credit to try out a Kubernetes cluster of your own. And don’t forget to thank them for their continued support of this show!
Modern Data teams are dealing with a lot of complexity in their data pipelines and analytical code. Monitoring data quality, tracing incidents, and testing changes can be daunting and often takes hours to days. Datafold helps Data teams gain visibility and confidence in the quality of their analytical data through data profiling, column-level lineage and intelligent anomaly detection. Datafold also helps automate regression testing of ETL code with its Data Diff feature that instantly shows how a change in ETL or BI code affects the produced data, both on a statistical level and down to individual rows and values. Datafold integrates with all major data warehouses as well as frameworks such as Airflow & dbt and seamlessly plugs into CI workflows. Follow go.datafold.com/dataengineeringpodcast to start a 30-day trial of Datafold. Once you sign up and create an alert in Datafold for your company data, they will send you a cool water flask.
Are you bogged down by having to manually manage data access controls, repeatedly move and copy data, and create audit reports to prove compliance? How much time could you save if those tasks were automated across your cloud platforms? Immuta is an automated data governance solution that enables safe and easy data analytics in the cloud. Our comprehensive data-level security, auditing and de-identification features eliminate the need for time-consuming manual processes and our focus on data and compliance team collaboration empowers you to deliver quick and valuable data analytics on the most sensitive data to unlock the full potential of your cloud data platforms. Learn how we streamline and accelerate manual processes to help you derive real results from your data at dataengineeringpodcast.com/immuta.
Your host is Tobias Macey and today I’m interviewing Grant Seward about Tree Schema, a human friendly data catalog
Interview
Introduction
How did you get involved in the area of data management?
Can you start by giving an overview of what you have built at Tree Schema?
What was your motivation for creating it?
At what stage of maturity should a team or organization consider a data catalog to be a necessary component in their data platform?
There are a large and growing number of projects and products designed to provide a data catalog, with each of them addressing the problem in a slightly different way. What are the necessary elements for a data catalog?
How does Tree Schema compare to the available options? (e.g. Amundsen, Company Wiki, Metacat, Metamapper, etc.)
How is the Tree Schema system implemented?
How has the design or direction of Tree Schema evolved since you first began working on it?
How did you approach the schema definitions for defining entities?
What was your guiding heuristic for determining how to design the interface and data models? – I wrote down notes that combine this with the question above
How do you handle integrating with data sources?
In addition to storing schema information you allow users to store information about the transformations being performed. How is that represented?
How can users populate information about their transformations in an automated fashion?
How do you approach evolution and versioning of schema information?
What are the scaling limitations of tree schema, whether in terms of the technical or cognitive complexity that it can handle?
What are some of the most interesting, innovative, or unexpected ways that you have seen Tree Schema being used?
What have you found to be the most interesting, unexpected, or challenging lessons learned in the process of building and promoting Tree Schema?
When is Tree Schema the wrong choice?
What do you have planned for the future of the product?
Contact Info
Email
Linkedin
Parting Question
From your perspective, what is the biggest gap in the tooling or technology for data management today?
Closing Announcements
Thank you for listening! Don’t forget to check out our other show, Podcast.__init__ to learn about the Python language, its community, and the innovative ways it is being used.
Visit the site to subscribe to the show, sign up for the mailing list, and read the show notes.
If you’ve learned something or tried out a project from the show then tell us about it! Email [email protected]) with your story.
To help other people find the show please leave a review on iTunes and tell your friends and co-workers
Join the community in the new Zulip chat workspace at dataengineeringpodcast.com/chat
Links
Tree Schema
Tree Schema – Data Lineage as Code
Capital One
Walmart Labs
Data Catalog
Data Discovery
Amundsen
Metacat
Marquez
Metamapper
Infoworks
Collibra
Faust
Podcast.__init__ Episode
Django
PostgreSQL
Redis
Celery
Amazon ECS (Elastic Container Service)
Django Storages
Dagster
Airflow
DataHub
Avro
Singer
Apache Atlas
The intro and outro music is from The Hug by The Freak Fandango Orchestra / CC BY-SA
Support Data Engineering Podcast
51:5310/11/2020
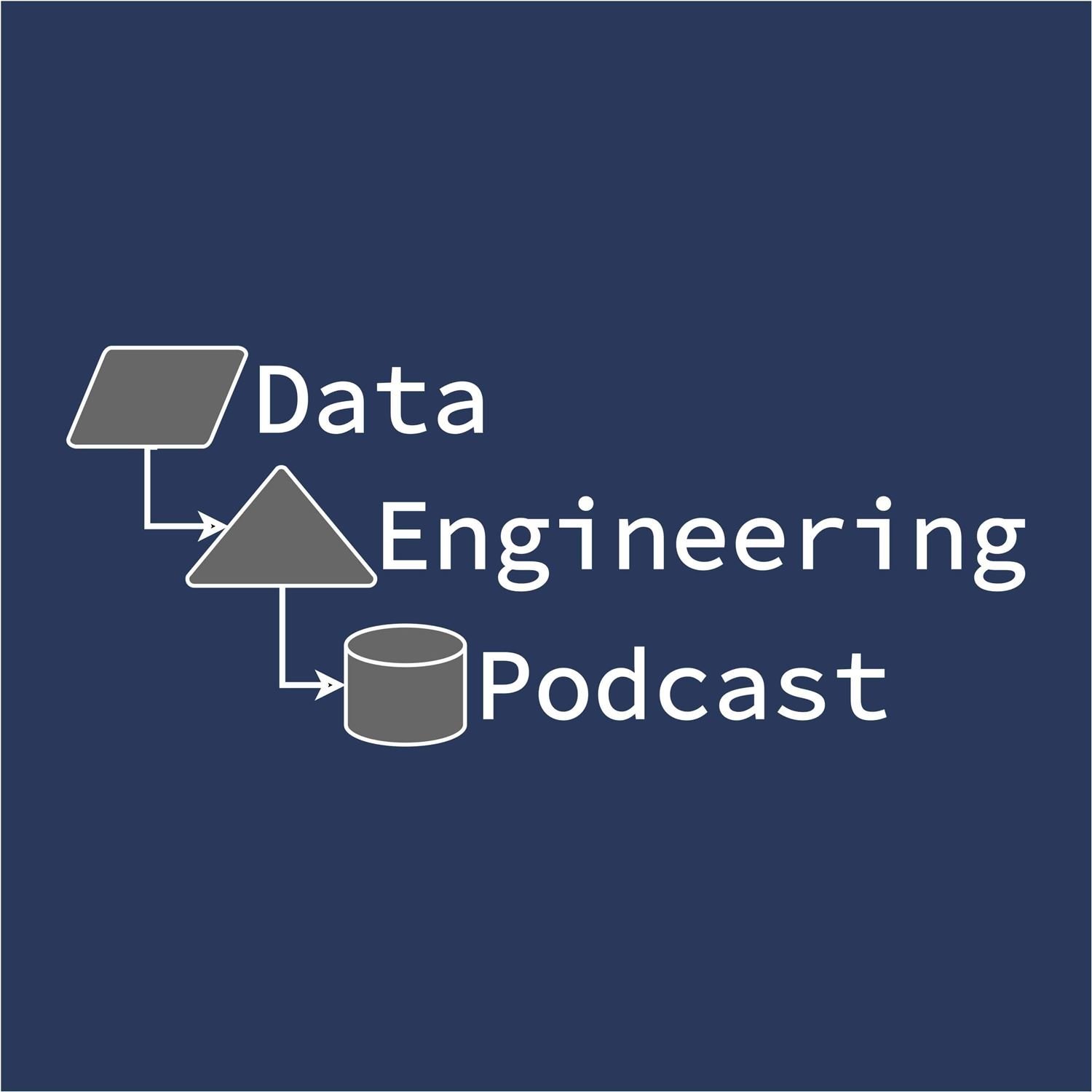
Add Version Control To Your Data Lake With LakeFS
Summary
Data lakes are gaining popularity due to their flexibility and reduced cost of storage. Along with the benefits there are some additional complexities to consider, including how to safely integrate new data sources or test out changes to existing pipelines. In order to address these challenges the team at Treeverse created LakeFS to introduce version control capabilities to your storage layer. In this episode Einat Orr and Oz Katz explain how they implemented branching and merging capabilities for object storage, best practices for how to use versioning primitives to introduce changes to your data lake, how LakeFS is architected, and how you can start using it for your own data platform.
Announcements
Hello and welcome to the Data Engineering Podcast, the show about modern data management
When you’re ready to build your next pipeline, or want to test out the projects you hear about on the show, you’ll need somewhere to deploy it, so check out our friends at Linode. With their managed Kubernetes platform it’s now even easier to deploy and scale your workflows, or try out the latest Helm charts from tools like Pulsar and Pachyderm. With simple pricing, fast networking, object storage, and worldwide data centers, you’ve got everything you need to run a bulletproof data platform. Go to dataengineeringpodcast.com/linode today and get a $60 credit to try out a Kubernetes cluster of your own. And don’t forget to thank them for their continued support of this show!
Are you bogged down by having to manually manage data access controls, repeatedly move and copy data, and create audit reports to prove compliance? How much time could you save if those tasks were automated across your cloud platforms? Immuta is an automated data governance solution that enables safe and easy data analytics in the cloud. Our comprehensive data-level security, auditing and de-identification features eliminate the need for time-consuming manual processes and our focus on data and compliance team collaboration empowers you to deliver quick and valuable data analytics on the most sensitive data to unlock the full potential of your cloud data platforms. Learn how we streamline and accelerate manual processes to help you derive real results from your data at dataengineeringpodcast.com/immuta.
Today’s episode of the Data Engineering Podcast is sponsored by Datadog, a SaaS-based monitoring and analytics platform for cloud-scale infrastructure, applications, logs, and more. Datadog uses machine-learning based algorithms to detect errors and anomalies across your entire stack—which reduces the time it takes to detect and address outages and helps promote collaboration between Data Engineering, Operations, and the rest of the company. Go to dataengineeringpodcast.com/datadog today to start your free 14 day trial. If you start a trial and install Datadog’s agent, Datadog will send you a free T-shirt.
Your host is Tobias Macey and today I’m interviewing Einat Orr and Oz Katz about their work at Treeverse on the LakeFS system for versioning your data lakes the same way you version your code.
Interview
Introduction
How did you get involved in the area of data management?
Can you start by giving an overview of what LakeFS is and why you built it?
There are a number of tools and platforms that support data virtualization and data versioning. How does LakeFS compare to the available options? (e.g. Alluxio, Denodo, Pachyderm, DVC, etc.)
What are the primary use cases that LakeFS enables?
For someone who wants to use LakeFS what is involved in getting it set up?
How is LakeFS implemented?
How has the design of the system changed or evolved since you began working on it?
What assumptions did you have going into it which have since been invalidated or modified?
How does the workflow for an engineer or analyst change from working directly against S3 to running against the LakeFS interface?
How do you handle merge conflicts and resolution?
What are some of the potential edge cases or foot guns that they should be aware of when there are multiple people using the same repository?
How do you approach management of the data lifecycle or garbage collection to avoid ballooning the cost of storage for a dataset that is tracking a high number of branches with diverging commits?
Given that S3 and GCS are eventually consistent storage layers, how do you handle snapshots/transactionality of the data you are working with?
What are the axes for scaling an installation of LakeFS?
What are the limitations in terms of size or geographic distribution of the datasets?
What are some of the most interesting, unexpected, or innovative ways that you have seen LakeFS being used?
What are the most interesting, unexpected, or challenging lessons that you have learned while building LakeFS?
When is LakeFS the wrong choice?
What do you have planned for the future of the project?
Contact Info
Einat Orr
Oz Katz
Parting Question
From your perspective, what is the biggest gap in the tooling or technology for data management today?
Closing Announcements
Thank you for listening! Don’t forget to check out our other show, Podcast.__init__ to learn about the Python language, its community, and the innovative ways it is being used.
Visit the site to subscribe to the show, sign up for the mailing list, and read the show notes.
If you’ve learned something or tried out a project from the show then tell us about it! Email [email protected]) with your story.
To help other people find the show please leave a review on iTunes and tell your friends and co-workers
Join the community in the new Zulip chat workspace at dataengineeringpodcast.com/chat
Links
Treeverse
LakeFS
GitHub
Documentation
lakeFS Slack Channel
SimilarWeb
Kaggle
DagsHub
Alluxio
Pachyderm
DVC
ML Ops (Machine Learning Operations)
DoltHub
Delta Lake
Podcast Episode
Hudi
Iceberg Table Format
Podcast Episode
Kubernetes
PostgreSQL
Podcast Episode
Git
Spark
Presto
CockroachDB
YugabyteDB
Citus
Hive Metastore
Iceberg Table Format
Immunai
The intro and outro music is from The Hug by The Freak Fandango Orchestra / CC BY-SA
Support Data Engineering Podcast
50:1503/11/2020
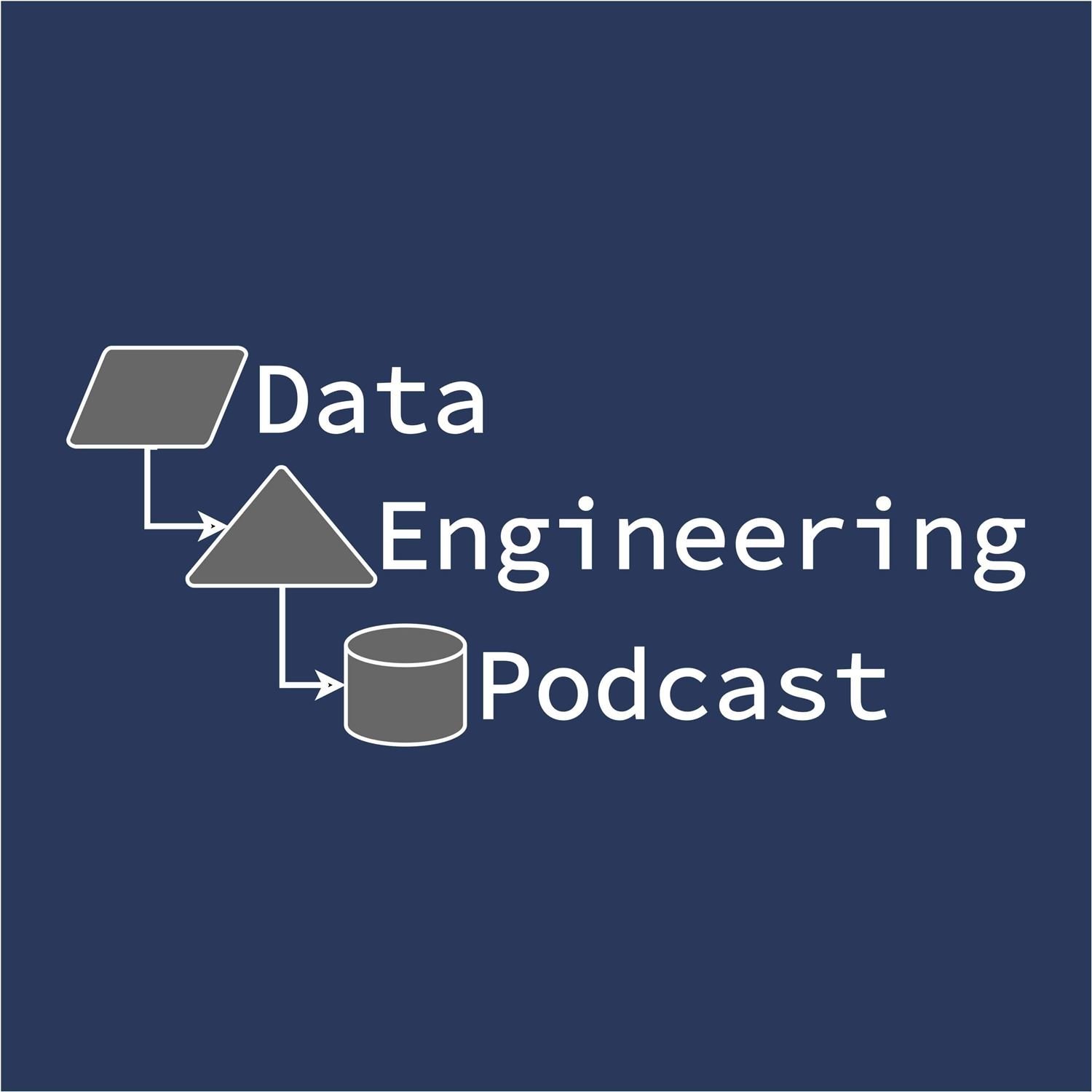
Cloud Native Data Security As Code With Cyral
Summary
One of the most challenging aspects of building a data platform has nothing to do with pipelines and transformations. If you are putting your workflows into production, then you need to consider how you are going to implement data security, including access controls and auditing. Different databases and storage systems all have their own method of restricting access, and they are not all compatible with each other. In order to simplify the process of securing your data in the Cloud Manav Mital created Cyral to provide a way of enforcing security as code. In this episode he explains how the system is architected, how it can help you enforce compliance, and what is involved in getting it integrated with your existing systems. This was a good conversation about an aspect of data management that is too often left as an afterthought.
Announcements
Hello and welcome to the Data Engineering Podcast, the show about modern data management
What are the pieces of advice that you wish you had received early in your career of data engineering? If you hand a book to a new data engineer, what wisdom would you add to it? I’m working with O’Reilly on a project to collect the 97 things that every data engineer should know, and I need your help. Go to dataengineeringpodcast.com/97things to add your voice and share your hard-earned expertise.
When you’re ready to build your next pipeline, or want to test out the projects you hear about on the show, you’ll need somewhere to deploy it, so check out our friends at Linode. With their managed Kubernetes platform it’s now even easier to deploy and scale your workflows, or try out the latest Helm charts from tools like Pulsar and Pachyderm. With simple pricing, fast networking, object storage, and worldwide data centers, you’ve got everything you need to run a bulletproof data platform. Go to dataengineeringpodcast.com/linode today and get a $60 credit to try out a Kubernetes cluster of your own. And don’t forget to thank them for their continued support of this show!
Are you bogged down by having to manually manage data access controls, repeatedly move and copy data, and create audit reports to prove compliance? How much time could you save if those tasks were automated across your cloud platforms? Immuta is an automated data governance solution that enables safe and easy data analytics in the cloud. Our comprehensive data-level security, auditing and de-identification features eliminate the need for time-consuming manual processes and our focus on data and compliance team collaboration empowers you to deliver quick and valuable data analytics on the most sensitive data to unlock the full potential of your cloud data platforms. Learn how we streamline and accelerate manual processes to help you derive real results from your data at dataengineeringpodcast.com/immuta.
Today’s episode of the Data Engineering Podcast is sponsored by Datadog, a SaaS-based monitoring and analytics platform for cloud-scale infrastructure, applications, logs, and more. Datadog uses machine-learning based algorithms to detect errors and anomalies across your entire stack—which reduces the time it takes to detect and address outages and helps promote collaboration between Data Engineering, Operations, and the rest of the company. Go to dataengineeringpodcast.com/datadog today to start your free 14 day trial. If you start a trial and install Datadog’s agent, Datadog will send you a free T-shirt.
You listen to this show to learn and stay up to date with what’s happening in databases, streaming platforms, big data, and everything else you need to know about modern data platforms. For more opportunities to stay up to date, gain new skills, and learn from your peers there are a growing number of virtual events that you can attend from the comfort and safety of your home. Go to dataengineeringpodcast.com/conferences to check out the upcoming events being offered by our partners and get registered today!
Your host is Tobias Macey and today I’m interviewing Manav Mital about the challenges involved in securing your data and the work that he is doing at Cyral to help address those problems.
Interview
Introduction
How did you get involved in the area of data management?
What is Cyral and what motivated you to build a business focused on addressing data security in the cloud?
Can you start by giving an overview of some of the common security issues that occur when working with data?
What new security challenges are introduced by building data platforms in public cloud environments?
What are the organizational roles that are typically responsible for managing security and access control to data sources and repositories?
What are the tensions, technical or organizational, that lead to a problematic or incomplete security posture?
What are the differences in security requirements and implementation complexity between software applications and data systems?
What are the data systems that Cyral integrates with?
How did you determine what platforms to prioritize?
How does Cyral integrate into the toolchains used to deploy, maintain, and upgrade an organization’s data infrastructure?
How does the Cyral platform address security and access control of data across an organization’s infrastructure?
How are schema changes handled when using Cyral to enforce access control to PII or other attributes?
How does Cyral help with reducing sprawl of data across unmonitored systems?
What are some of the most interesting, unexpected, or challenging lessons that you learned while building Cyral?
When is Cyral the wrong choice?
What do you have planned for the future of the Cyral platform?
Contact Info
LinkedIn
@manavrm on Twitter
Parting Question
From your perspective, what is the biggest gap in the tooling or technology for data management today?
Links
Cyral
Snowflake
Podcast Episode
BigQuery
Object Storage
MongoDB
The intro and outro music is from The Hug by The Freak Fandango Orchestra / CC BY-SA
Support Data Engineering Podcast
48:3326/10/2020
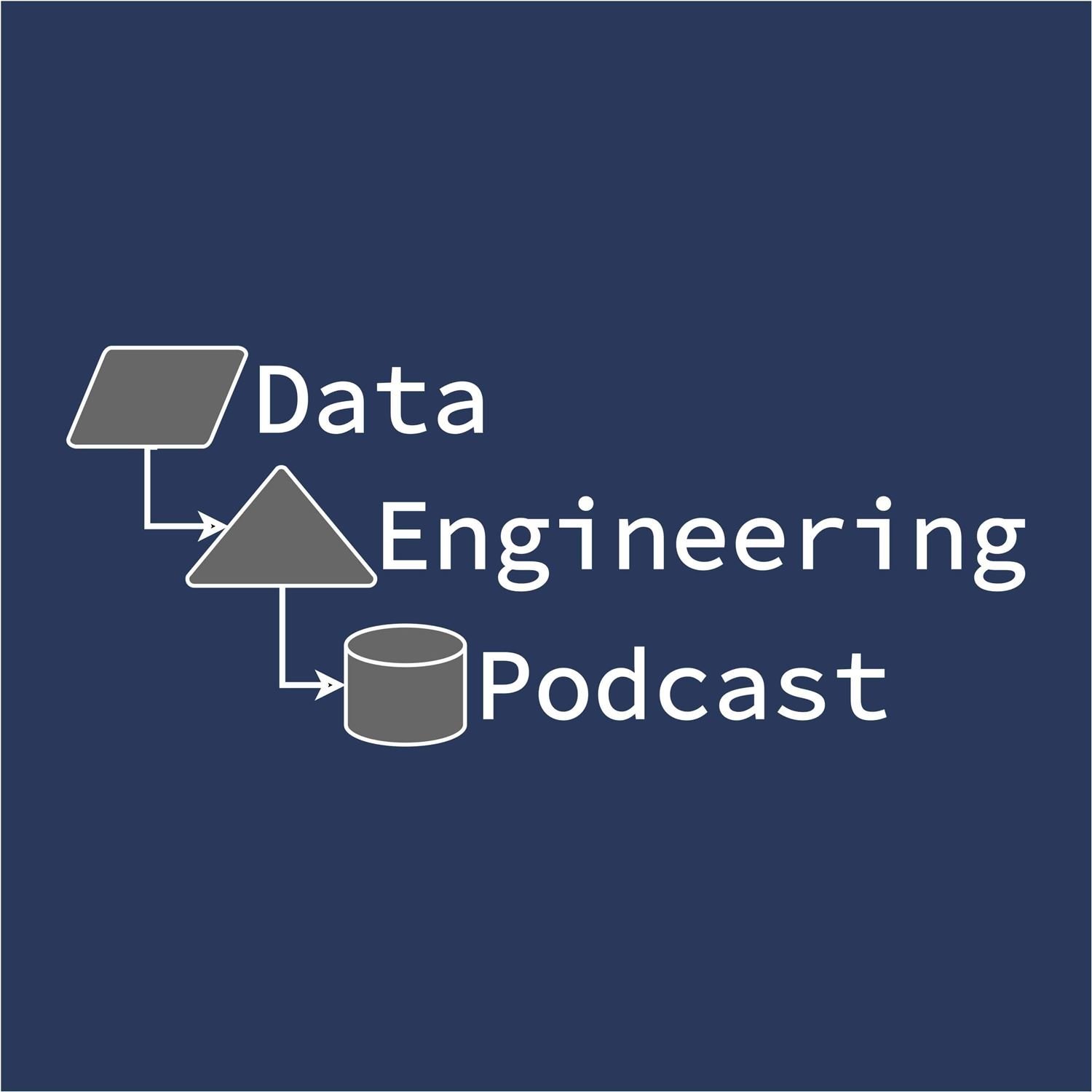
Better Data Quality Through Observability With Monte Carlo
Summary
In order for analytics and machine learning projects to be useful, they require a high degree of data quality. To ensure that your pipelines are healthy you need a way to make them observable. In this episode Barr Moses and Lior Gavish, co-founders of Monte Carlo, share the leading causes of what they refer to as data downtime and how it manifests. They also discuss methods for gaining visibility into the flow of data through your infrastructure, how to diagnose and prevent potential problems, and what they are building at Monte Carlo to help you maintain your data’s uptime.
Announcements
Hello and welcome to the Data Engineering Podcast, the show about modern data management
What are the pieces of advice that you wish you had received early in your career of data engineering? If you hand a book to a new data engineer, what wisdom would you add to it? I’m working with O’Reilly on a project to collect the 97 things that every data engineer should know, and I need your help. Go to dataengineeringpodcast.com/97things to add your voice and share your hard-earned expertise.
When you’re ready to build your next pipeline, or want to test out the projects you hear about on the show, you’ll need somewhere to deploy it, so check out our friends at Linode. With their managed Kubernetes platform it’s now even easier to deploy and scale your workflows, or try out the latest Helm charts from tools like Pulsar and Pachyderm. With simple pricing, fast networking, object storage, and worldwide data centers, you’ve got everything you need to run a bulletproof data platform. Go to dataengineeringpodcast.com/linode today and get a $60 credit to try out a Kubernetes cluster of your own. And don’t forget to thank them for their continued support of this show!
Are you bogged down by having to manually manage data access controls, repeatedly move and copy data, and create audit reports to prove compliance? How much time could you save if those tasks were automated across your cloud platforms? Immuta is an automated data governance solution that enables safe and easy data analytics in the cloud. Our comprehensive data-level security, auditing and de-identification features eliminate the need for time-consuming manual processes and our focus on data and compliance team collaboration empowers you to deliver quick and valuable data analytics on the most sensitive data to unlock the full potential of your cloud data platforms. Learn how we streamline and accelerate manual processes to help you derive real results from your data at dataengineeringpodcast.com/immuta.
Today’s episode of the Data Engineering Podcast is sponsored by Datadog, a SaaS-based monitoring and analytics platform for cloud-scale infrastructure, applications, logs, and more. Datadog uses machine-learning based algorithms to detect errors and anomalies across your entire stack—which reduces the time it takes to detect and address outages and helps promote collaboration between Data Engineering, Operations, and the rest of the company. Go to dataengineeringpodcast.com/datadog today to start your free 14 day trial. If you start a trial and install Datadog’s agent, Datadog will send you a free T-shirt.
You listen to this show to learn and stay up to date with what’s happening in databases, streaming platforms, big data, and everything else you need to know about modern data platforms. For more opportunities to stay up to date, gain new skills, and learn from your peers there are a growing number of virtual events that you can attend from the comfort and safety of your home. Go to dataengineeringpodcast.com/conferences to check out the upcoming events being offered by our partners and get registered today!
Your host is Tobias Macey and today I’m interviewing Barr Moses and Lior Gavish about observability for your data pipelines and how they are addressing it at Monte Carlo.
Interview
Introduction
How did you get involved in the area of data management?
How did you come up with the idea to found Monte Carlo?
What is "data downtime"?
Can you start by giving your definition of observability in the context of data workflows?
What are some of the contributing factors that lead to poor data quality at the different stages of the lifecycle?
Monitoring and observability of infrastructure and software applications is a well understood problem. In what ways does observability of data applications differ from "traditional" software systems?
What are some of the metrics or signals that we should be looking at to identify problems in our data applications?
Why is this the year that so many companies are working to address the issue of data quality and observability?
How are you addressing the challenge of bringing observability to data platforms at Monte Carlo?
What are the areas of integration that you are targeting and how did you identify where to prioritize your efforts?
For someone who is using Monte Carlo, how does the platform help them to identify and resolve issues in their data?
What stage of the data lifecycle have you found to be the biggest contributor to downtime and quality issues?
What are the most challenging systems, platforms, or tool chains to gain visibility into?
What are some of the most interesting, innovative, or unexpected ways that you have seen teams address their observability needs?
What are the most interesting, unexpected, or challenging lessons that you have learned while building the business and technology of Monte Carlo?
What are the alternatives to Monte Carlo?
What do you have planned for the future of the platform?
Contact Info
Visit www.montecarlodata.com?utm_source=rss&utm_medium=rss to lean more about our data reliability platform;
Or reach out directly to [email protected] — happy to chat about all things data!
Parting Question
From your perspective, what is the biggest gap in the tooling or technology for data management today?
Links
Monte Carlo
Monte Carlo Platform
Observability
Gainsight
Barracuda Networks
DevOps
New Relic
Datadog
Netflix RAD Outlier Detection
The intro and outro music is from The Hug by The Freak Fandango Orchestra / CC BY-SA
Support Data Engineering Podcast
55:5319/10/2020
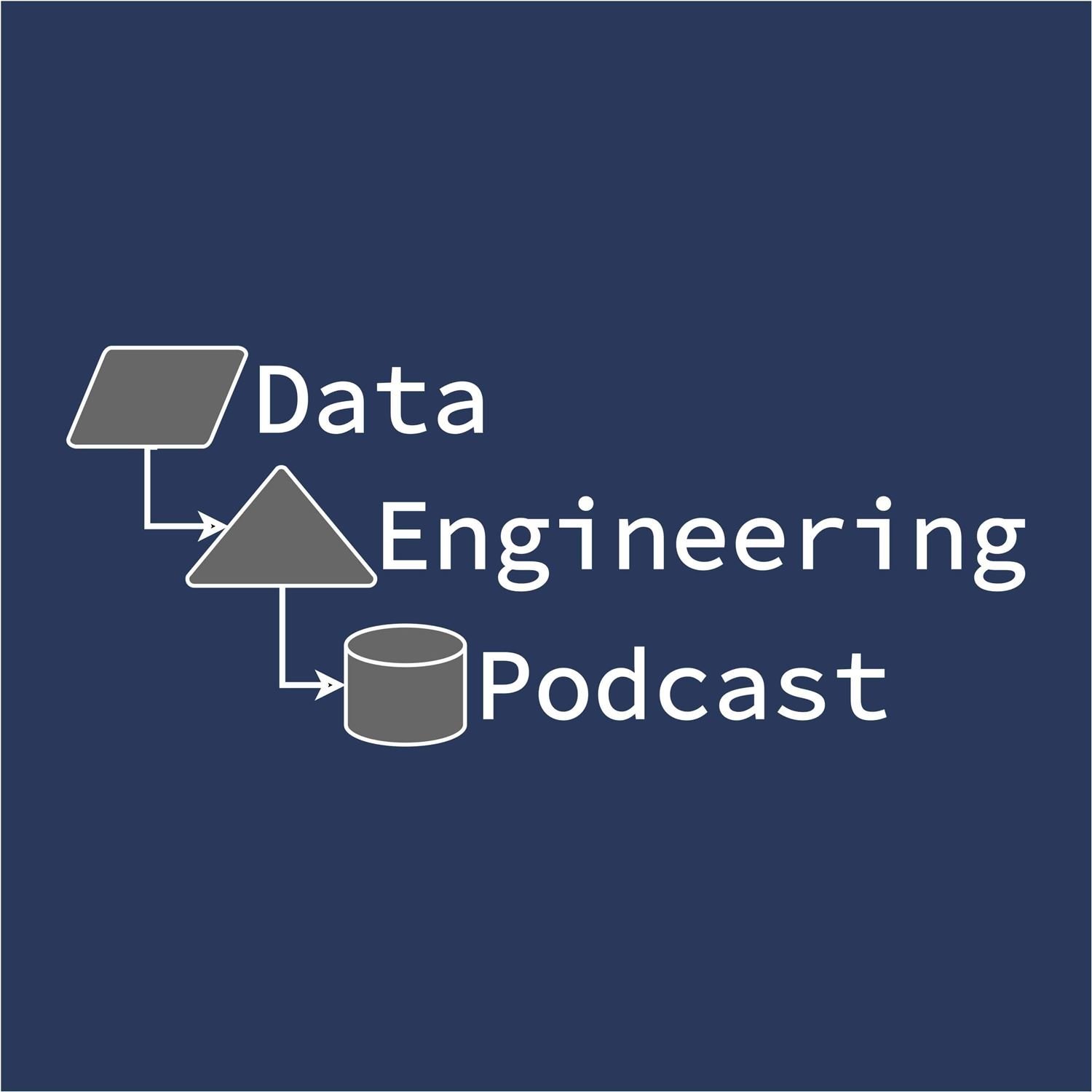
Rapid Delivery Of Business Intelligence Using Power BI
Summary
Business intelligence efforts are only as useful as the outcomes that they inform. Power BI aims to reduce the time and effort required to go from information to action by providing an interface that encourages rapid iteration. In this episode Rob Collie shares his enthusiasm for the Power BI platform and how it stands out from other options. He explains how he helped to build the platform during his time at Microsoft, and how he continues to support users through his work at Power Pivot Pro. Rob shares some useful insights gained through his consulting work, and why he considers Power BI to be the best option on the market today for business analytics.
Announcements
Hello and welcome to the Data Engineering Podcast, the show about modern data management
What are the pieces of advice that you wish you had received early in your career of data engineering? If you hand a book to a new data engineer, what wisdom would you add to it? I’m working with O’Reilly on a project to collect the 97 things that every data engineer should know, and I need your help. Go to dataengineeringpodcast.com/97things to add your voice and share your hard-earned expertise.
When you’re ready to build your next pipeline, or want to test out the projects you hear about on the show, you’ll need somewhere to deploy it, so check out our friends at Linode. With their managed Kubernetes platform it’s now even easier to deploy and scale your workflows, or try out the latest Helm charts from tools like Pulsar and Pachyderm. With simple pricing, fast networking, object storage, and worldwide data centers, you’ve got everything you need to run a bulletproof data platform. Go to dataengineeringpodcast.com/linode today and get a $60 credit to try out a Kubernetes cluster of your own. And don’t forget to thank them for their continued support of this show!
Are you bogged down by having to manually manage data access controls, repeatedly move and copy data, and create audit reports to prove compliance? How much time could you save if those tasks were automated across your cloud platforms? Immuta is an automated data governance solution that enables safe and easy data analytics in the cloud. Our comprehensive data-level security, auditing and de-identification features eliminate the need for time-consuming manual processes and our focus on data and compliance team collaboration empowers you to deliver quick and valuable data analytics on the most sensitive data to unlock the full potential of your cloud data platforms. Learn how we streamline and accelerate manual processes to help you derive real results from your data at dataengineeringpodcast.com/immuta.
Equalum’s end to end data ingestion platform is relied upon by enterprises across industries to seamlessly stream data to operational, real-time analytics and machine learning environments. Equalum combines streaming Change Data Capture, replication, complex transformations, batch processing and full data management using a no-code UI. Equalum also leverages open source data frameworks by orchestrating Apache Spark, Kafka and others under the hood. Tool consolidation and linear scalability without the legacy platform price tag. Go to dataengineeringpodcast.com/equalum today to start a free 2 week test run of their platform, and don’t forget to tell them that we sent you.
You listen to this show to learn and stay up to date with what’s happening in databases, streaming platforms, big data, and everything else you need to know about modern data platforms. For more opportunities to stay up to date, gain new skills, and learn from your peers there are a growing number of virtual events that you can attend from the comfort and safety of your home. Go to dataengineeringpodcast.com/conferences to check out the upcoming events being offered by our partners and get registered today!
Your host is Tobias Macey and today I’m interviewing Rob Collie about Microsoft’s Power BI platform and his work at Power Pivot Pro to help users employ it effectively.
Interview
Introduction
How did you get involved in the area of data management?
Can you start by giving an overview of what Power BI is?
The business intelligence market is fairly crowded. What are the features of Power BI that make it stand out?
Who are the target users of Power BI?
How does the design of the platform reflect those priorities?
Can you talk through the workflow for someone to build a report or dashboard in Power BI?
What is the broader ecosystem of data tools and platforms that Power BI sits within?
What are the available integration and extension points for Power BI?
In addition to your work at Microsoft building Power BI you now run a consulting company dedicated to helping people adopt that platform. What are some of the common challenges that users face in employing Power BI effectively?
In your experience working with clients, what are some of the core principles of data processing and visualization that apply across industries?
What are some of the modeling or presentation methods that are specific to a given industry?
One of the perennial challenges of business intelligence is to make reports discoverable. What facilities does Power BI have to aid in surfacing useful information to end users?
What capabilities does Power BI have for exposing elements of data quality?
What are some of the most challenging aspects of building and maintaining a business intelligence effort in an organization?
What are some of the most interesting, unexpected, or innovative uses of Power BI that you have seen, or projects that you have worked on?
What are some of the most interesting, unexpected, or challenging lessons that you have learned in your work building Power BI and building a business to support its users?
When is Power BI the wrong choice?
What trends in business intelligence are you most excited by?
Contact Info
LinkedIn
@robocolli3 on Twitter
Parting Question
From your perspective, what is the biggest gap in the tooling or technology for data management today?
Links
P3
Power BI
Microsoft Excel
Fantasy Football
Excel Functions
Lisp
Business Intelligence
VLOOKUP
Looker
Podcast Episode
SQL Server Reporting Services
SQL Server Analysis Services
Tableau
Master Data Management
ERP == Enterprise Resoure Planning
M Language
Power Query
DAX
The intro and outro music is from The Hug by The Freak Fandango Orchestra / CC BY-SA
Support Data Engineering Podcast
01:02:5512/10/2020
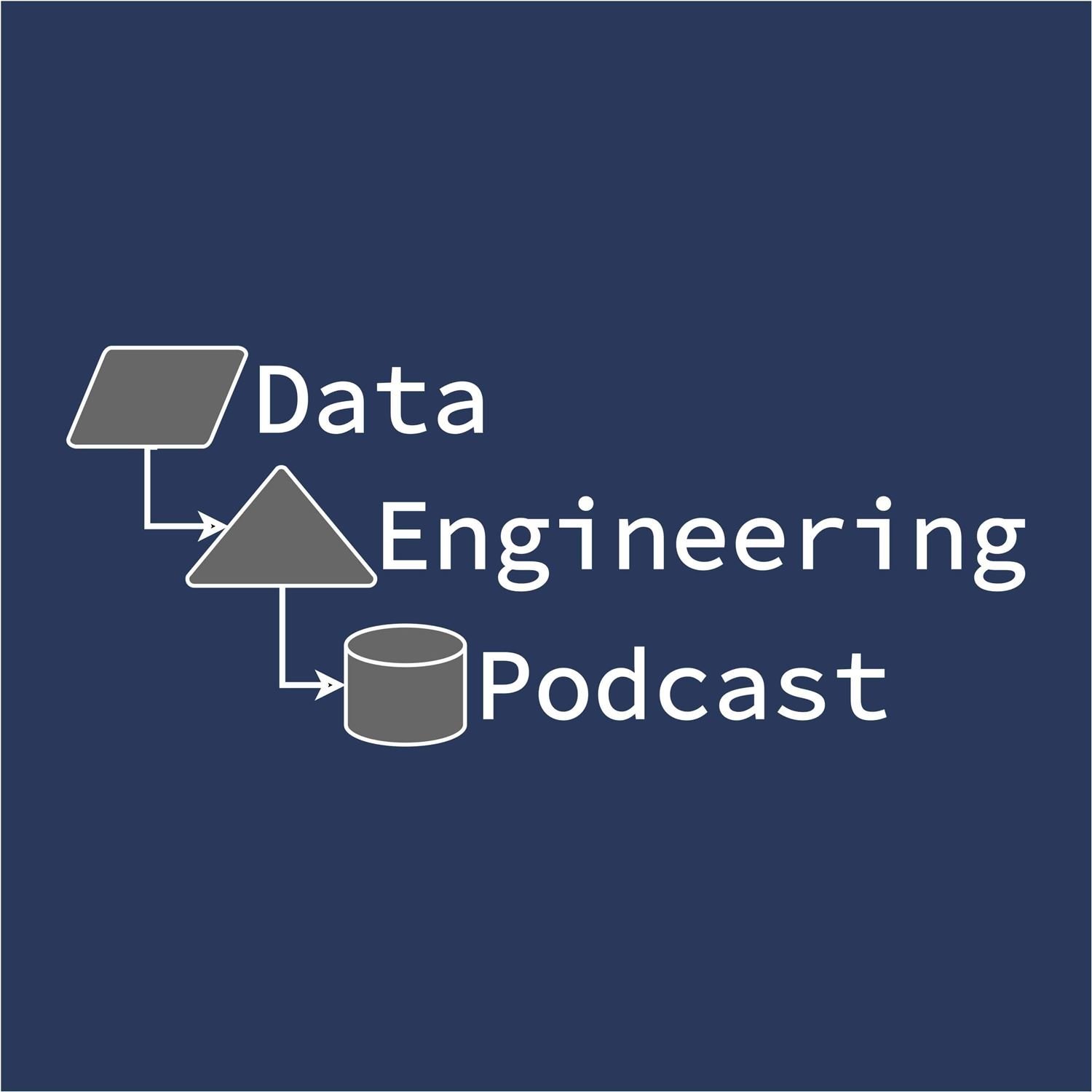
Self Service Real Time Data Integration Without The Headaches With Meroxa
Summary
Analytical workloads require a well engineered and well maintained data integration process to ensure that your information is reliable and up to date. Building a real-time pipeline for your data lakes and data warehouses is a non-trivial effort, requiring a substantial investment of time and energy. Meroxa is a new platform that aims to automate the heavy lifting of change data capture, monitoring, and data loading. In this episode founders DeVaris Brown and Ali Hamidi explain how their tenure at Heroku informed their approach to making data integration self service, how the platform is architected, and how they have designed their system to adapt to the continued evolution of the data ecosystem.
Announcements
Hello and welcome to the Data Engineering Podcast, the show about modern data management
What are the pieces of advice that you wish you had received early in your career of data engineering? If you hand a book to a new data engineer, what wisdom would you add to it? I’m working with O’Reilly on a project to collect the 97 things that every data engineer should know, and I need your help. Go to dataengineeringpodcast.com/97things to add your voice and share your hard-earned expertise.
When you’re ready to build your next pipeline, or want to test out the projects you hear about on the show, you’ll need somewhere to deploy it, so check out our friends at Linode. With their managed Kubernetes platform it’s now even easier to deploy and scale your workflows, or try out the latest Helm charts from tools like Pulsar and Pachyderm. With simple pricing, fast networking, object storage, and worldwide data centers, you’ve got everything you need to run a bulletproof data platform. Go to dataengineeringpodcast.com/linode today and get a $60 credit to try out a Kubernetes cluster of your own. And don’t forget to thank them for their continued support of this show!
Are you bogged down by having to manually manage data access controls, repeatedly move and copy data, and create audit reports to prove compliance? How much time could you save if those tasks were automated across your cloud platforms? Immuta is an automated data governance solution that enables safe and easy data analytics in the cloud. Our comprehensive data-level security, auditing and de-identification features eliminate the need for time-consuming manual processes and our focus on data and compliance team collaboration empowers you to deliver quick and valuable data analytics on the most sensitive data to unlock the full potential of your cloud data platforms. Learn how we streamline and accelerate manual processes to help you derive real results from your data at dataengineeringpodcast.com/immuta.
Today’s episode of the Data Engineering Podcast is sponsored by Datadog, a SaaS-based monitoring and analytics platform for cloud-scale infrastructure, applications, logs, and more. Datadog uses machine-learning based algorithms to detect errors and anomalies across your entire stack—which reduces the time it takes to detect and address outages and helps promote collaboration between Data Engineering, Operations, and the rest of the company. Go to dataengineeringpodcast.com/datadog today to start your free 14 day trial. If you start a trial and install Datadog’s agent, Datadog will send you a free T-shirt.
You listen to this show to learn and stay up to date with what’s happening in databases, streaming platforms, big data, and everything else you need to know about modern data platforms. For more opportunities to stay up to date, gain new skills, and learn from your peers there are a growing number of virtual events that you can attend from the comfort and safety of your home. Go to dataengineeringpodcast.com/conferences to check out the upcoming events being offered by our partners and get registered today!
Your host is Tobias Macey and today I’m interviewing DeVaris Brown and Ali Hamidi about Meroxa, a new platform as a service for data integration
Interview
Introduction
How did you get involved in the area of data management?
Can you start by describing what you are building at Meroxa and what motivated you to turn it into a business?
What are the lessons that you learned from your time at Heroku which you are applying to your work on Meroxa?
Who are your target users and what are your guiding principles for designing the platform interface?
What are the common difficulties that engineers face in building and maintaining data infrastructure?
There are a variety of platforms that offer solutions for managing data integration, or powering end-to-end analytics, or building machine learning pipelines. What are the shortcomings of those existing options that might lead someone to choose Meroxa?
How is the Meroxa platform architected?
What are some of the initial assumptions that you had which have been challenged as you proceed with implementation?
What new capabilities does Meroxa bring to someone who uses it for integrating their application data?
What are the growth options for organizations that get started with Meroxa?
What are the core principles that you are focused on to allow for evolving your platform over the long run as the surrounding ecosystem continues to mature?
When is Meroxa the wrong choice?
What do you have planned for the future?
Contact Info
DeVaris Brown
Ali Hamidi
Parting Question
From your perspective, what is the biggest gap in the tooling or technology for data management today?
Closing Announcements
Thank you for listening! Don’t forget to check out our other show, Podcast.__init__ to learn about the Python language, its community, and the innovative ways it is being used.
Visit the site to subscribe to the show, sign up for the mailing list, and read the show notes.
If you’ve learned something or tried out a project from the show then tell us about it! Email [email protected]) with your story.
To help other people find the show please leave a review on iTunes and tell your friends and co-workers
Join the community in the new Zulip chat workspace at dataengineeringpodcast.com/chat
Links
Meroxa
Heroku
Heroku Kafka
Ascend
StreamSets
Nexus
Kafka Connect
Airflow
Podcast.__init__ Episode
Spark
Data Engineering Episode
Change Data Capture
Segment
Podcast Episode
Rudderstack
MParticle
Debezium
Podcast Episode
DBT
Podcast Episode
Materialize
Podcast Episode
Stitch Data
Fivetran
Podcast Episode
Elasticsearch
Podcast Episode
gRPC
GraphQL
REST == REpresentational State Transfer
Dagster/Elementl
Data Engineering Podcast Episode
Podcast.__init__ Episode
Prefect
Podcast Episode
The intro and outro music is from The Hug by The Freak Fandango Orchestra / CC BY-SA
Support Data Engineering Podcast
01:00:5605/10/2020
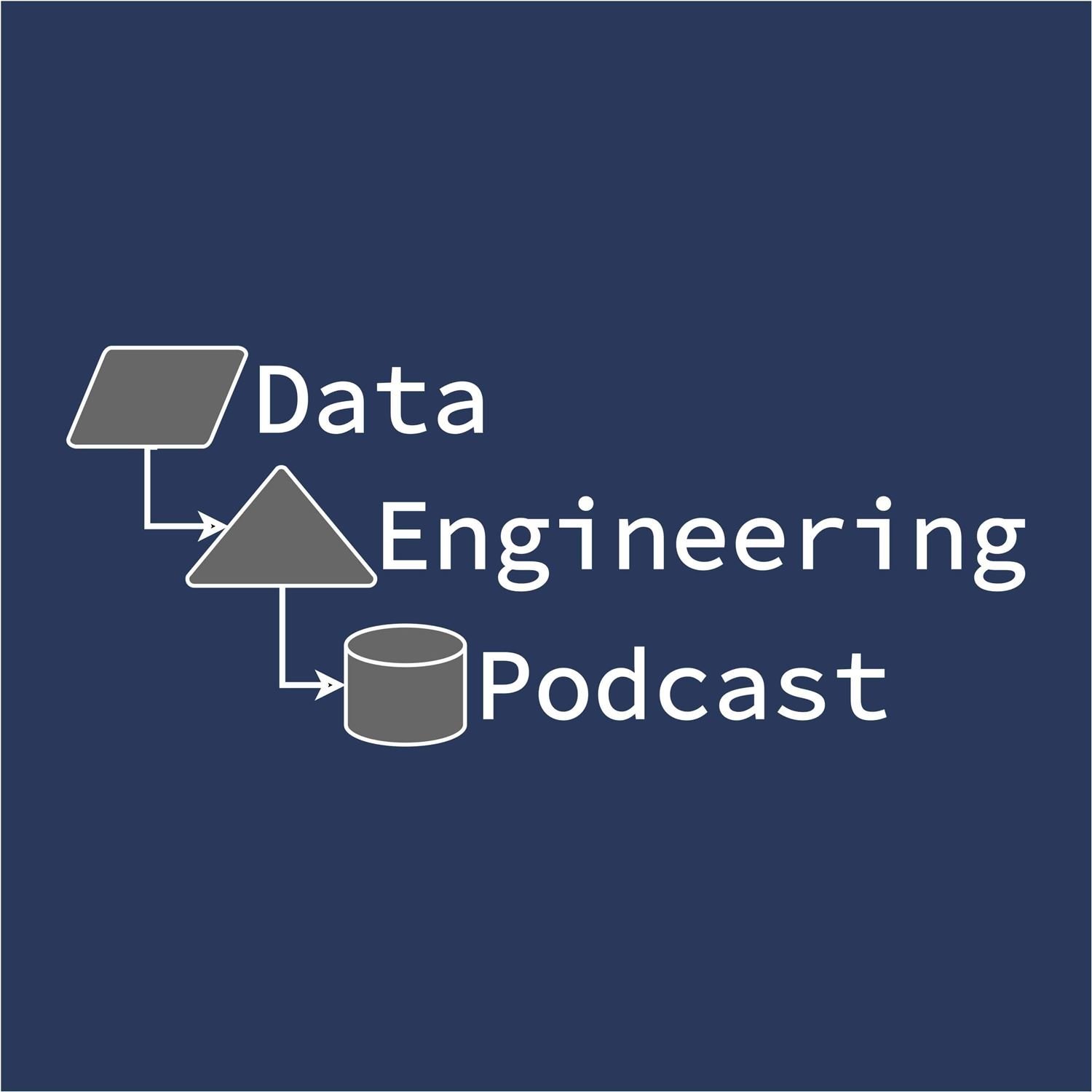
Speed Up And Simplify Your Streaming Data Workloads With Red Panda
Summary
Kafka has become a de facto standard interface for building decoupled systems and working with streaming data. Despite its widespread popularity, there are numerous accounts of the difficulty that operators face in keeping it reliable and performant, or trying to scale an installation. To make the benefits of the Kafka ecosystem more accessible and reduce the operational burden, Alexander Gallego and his team at Vectorized created the Red Panda engine. In this episode he explains how they engineered a drop-in replacement for Kafka, replicating the numerous APIs, that can scale more easily and deliver consistently low latencies with a much lower hardware footprint. He also shares some of the areas of innovation that they have found to help foster the next wave of streaming applications while working within the constraints of the existing Kafka interfaces. This was a fascinating conversation with an energetic and enthusiastic engineer and founder about the challenges and opportunities in the realm of streaming data.
Announcements
Hello and welcome to the Data Engineering Podcast, the show about modern data management
What are the pieces of advice that you wish you had received early in your career of data engineering? If you hand a book to a new data engineer, what wisdom would you add to it? I’m working with O’Reilly on a project to collect the 97 things that every data engineer should know, and I need your help. Go to dataengineeringpodcast.com/97things to add your voice and share your hard-earned expertise.
When you’re ready to build your next pipeline, or want to test out the projects you hear about on the show, you’ll need somewhere to deploy it, so check out our friends at Linode. With their managed Kubernetes platform it’s now even easier to deploy and scale your workflows, or try out the latest Helm charts from tools like Pulsar and Pachyderm. With simple pricing, fast networking, object storage, and worldwide data centers, you’ve got everything you need to run a bulletproof data platform. Go to dataengineeringpodcast.com/linode today and get a $60 credit to try out a Kubernetes cluster of your own. And don’t forget to thank them for their continued support of this show!
Are you bogged down by having to manually manage data access controls, repeatedly move and copy data, and create audit reports to prove compliance? How much time could you save if those tasks were automated across your cloud platforms? Immuta is an automated data governance solution that enables safe and easy data analytics in the cloud. Our comprehensive data-level security, auditing and de-identification features eliminate the need for time-consuming manual processes and our focus on data and compliance team collaboration empowers you to deliver quick and valuable data analytics on the most sensitive data to unlock the full potential of your cloud data platforms. Learn how we streamline and accelerate manual processes to help you derive real results from your data at dataengineeringpodcast.com/immuta.
If you’re looking for a way to optimize your data engineering pipeline – with instant query performance – look no further than Qubz. Qubz is next-generation OLAP technology built for the scale of Big Data from UST Global, a renowned digital services provider. Qubz lets users and enterprises analyze data on the cloud and on-premise, with blazing speed, while eliminating the complex engineering required to operationalize analytics at scale. With an emphasis on visual data engineering, connectors for all major BI tools and data sources, Qubz allow users to query OLAP cubes with sub-second response times on hundreds of billions of rows. To learn more, and sign up for a free demo, visit dataengineeringpodcast.com/qubz.
You listen to this show to learn and stay up to date with what’s happening in databases, streaming platforms, big data, and everything else you need to know about modern data platforms. For more opportunities to stay up to date, gain new skills, and learn from your peers there are a growing number of virtual events that you can attend from the comfort and safety of your home. Go to dataengineeringpodcast.com/conferences to check out the upcoming events being offered by our partners and get registered today!
Your host is Tobias Macey and today I’m interviewing Alexander Gallego about his work at Vectorized building Red Panda as a performance optimized, drop-in replacement for Kafka
Interview
Introduction
How did you get involved in the area of data management?
Can you start by describing what Red Panda is and what motivated you to create it?
What are the limitations of Kafka that make something like Red Panda necessary?
What are the current strengths of the Kafka ecosystem that make it a reasonable implementation target for Red Panda?
How is Red Panda architected?
How has the design or direction changed or evolved since you first began working on it?
What are the challenges that you face in automatically optimizing the runtime to take advantage of the hardware that it is deployed on?
How do cloud environments contribute to that complexity?
How are you handling the compatibility layer for the Kafka API?
What is your approach for managing versioning and ensuring that you maintain bug compatibility?
Beyond performance, what other areas of innovation or improvement in the capabilities and experience do you see while adhering to the Kafka protocol?
What are the opportunities for innovation in the streaming space that aren’t being explored yet?
What are some of the most interesting, innovative, or unexpected ways that you have seen Redpanda being used?
What are the most interesting, unexpected, or challenging lessons that you have learned while building Red Panda and Vectorized?
When is Red Panda the wrong choice?
What do you have planned for the future of the product and business?
What is your Hack The Planet diversity scholarship?
Contact Info
@emaxerrno on Twitter
LinkedIn
Parting Question
From your perspective, what is the biggest gap in the tooling or technology for data management today?
Closing Announcements
Thank you for listening! Don’t forget to check out our other show, Podcast.__init__ to learn about the Python language, its community, and the innovative ways it is being used.
Visit the site to subscribe to the show, sign up for the mailing list, and read the show notes.
If you’ve learned something or tried out a project from the show then tell us about it! Email [email protected]) with your story.
To help other people find the show please leave a review on iTunes and tell your friends and co-workers
Join the community in the new Zulip chat workspace at dataengineeringpodcast.com/chat
Links
Vectorized
Free Download Trial
@vectorizedio Company Twitter Accn’t
Community Slack
Concord alternative to Flink
Apache Flink
Podcast Episode
FAANG == Facebook, Apple, Amazon, Netflix, and Google
Blackblaze
Raft
NATS
Pulsar
Podcast Episode
StreamNative Podcast Episode
Open Messaging Specification
ScyllaDB
CockroachDB
MemSQL
WASM == Web Assembly
Debezium
Podcast Episode
The intro and outro music is from The Hug by The Freak Fandango Orchestra / CC BY-SA
Support Data Engineering Podcast
59:4129/09/2020
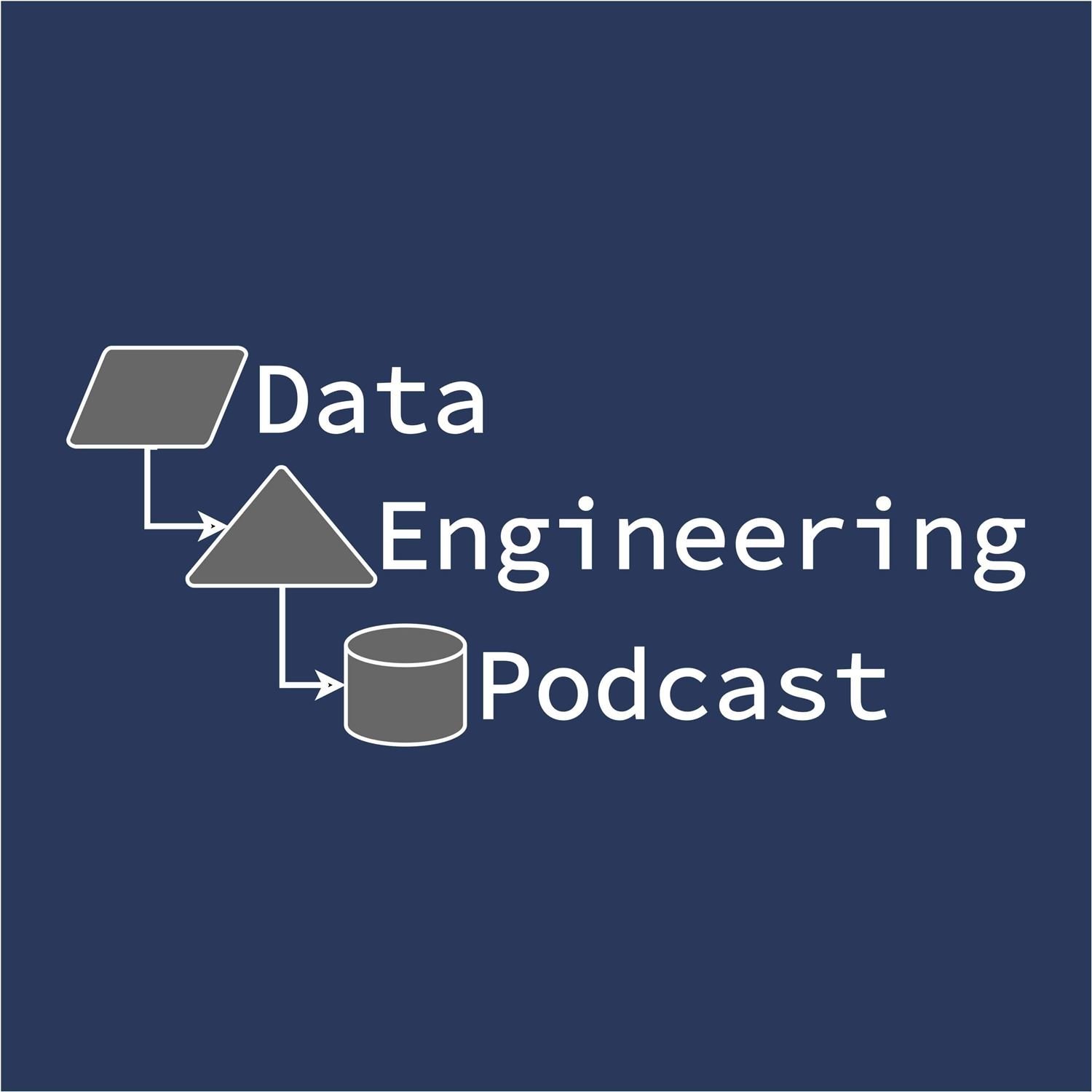
Cutting Through The Noise And Focusing On The Fundamentals Of Data Engineering With The Data Janitor
Summary
Data engineering is a constantly growing and evolving discipline. There are always new tools, systems, and design patterns to learn, which leads to a great deal of confusion for newcomers. Daniel Molnar has dedicated his time to helping data professionals get back to basics through presentations at conferences and meetups, and with his most recent endeavor of building the Pipeline Data Engineering Academy. In this episode he shares advice on how to cut through the noise, which principles are foundational to building a successful career as a data engineer, and his approach to educating the next generation of data practitioners. This was a useful conversation for anyone working with data who has found themselves spending too much time chasing the latest trends and wishes to develop a more focused approach to their work.
Announcements
Hello and welcome to the Data Engineering Podcast, the show about modern data management
What are the pieces of advice that you wish you had received early in your career of data engineering? If you hand a book to a new data engineer, what wisdom would you add to it? I’m working with O’Reilly on a project to collect the 97 things that every data engineer should know, and I need your help. Go to dataengineeringpodcast.com/97things to add your voice and share your hard-earned expertise.
When you’re ready to build your next pipeline, or want to test out the projects you hear about on the show, you’ll need somewhere to deploy it, so check out our friends at Linode. With their managed Kubernetes platform it’s now even easier to deploy and scale your workflows, or try out the latest Helm charts from tools like Pulsar and Pachyderm. With simple pricing, fast networking, object storage, and worldwide data centers, you’ve got everything you need to run a bulletproof data platform. Go to dataengineeringpodcast.com/linode today and get a $60 credit to try out a Kubernetes cluster of your own. And don’t forget to thank them for their continued support of this show!
Are you bogged down by having to manually manage data access controls, repeatedly move and copy data, and create audit reports to prove compliance? How much time could you save if those tasks were automated across your cloud platforms? Immuta is an automated data governance solution that enables safe and easy data analytics in the cloud. Our comprehensive data-level security, auditing and de-identification features eliminate the need for time-consuming manual processes and our focus on data and compliance team collaboration empowers you to deliver quick and valuable data analytics on the most sensitive data to unlock the full potential of your cloud data platforms. Learn how we streamline and accelerate manual processes to help you derive real results from your data at dataengineeringpodcast.com/immuta.
You listen to this show to learn and stay up to date with what’s happening in databases, streaming platforms, big data, and everything else you need to know about modern data platforms. For more opportunities to stay up to date, gain new skills, and learn from your peers there are a growing number of virtual events that you can attend from the comfort and safety of your home. Go to dataengineeringpodcast.com/conferences to check out the upcoming events being offered by our partners and get registered today!
Your host is Tobias Macey and today I’m interviewing Daniel Molnar about being a data janitor and how to cut through the hype to understand what to learn for the long run
Interview
Introduction
How did you get involved in the area of data management?
Can you start by describing your thoughts on the current state of the data management industry?
What is your strategy for being effective in the face of so much complexity and conflicting needs for data?
What are some of the common difficulties that you see data engineers contend with, whether technical or social/organizational?
What are the core fundamentals that you think are necessary for data engineers to be effective?
What are the gaps in knowledge or experience that you have seen data engineers contend with?
You recently started down the path of building a bootcamp for training data engineers. What was your motivation for embarking on that journey?
How would you characterize your particular approach?
What are some of the reasons that your applicants have for wanting to become versed in data engineering?
What is the baseline of capabilities that you expect of your target audience?
What level of proficiency do you aim for when someone has completed your training program?
Who do you think would not be a good fit for your academy?
As a hiring manager, what are the core capabilities that you look for in a data engineering candidate?
What are some of the methods that you use to assess competence?
What are the overall trends in the data management space that you are worried by?
Which ones are you happy about?
What are your plans and overall goals for the pipeline academy?
Contact Info
LinkedIn
@soobrosa on Twitter
Website
Parting Question
From your perspective, what is the biggest gap in the tooling or technology for data management today?
Closing Announcements
Thank you for listening! Don’t forget to check out our other show, Podcast.__init__ to learn about the Python language, its community, and the innovative ways it is being used.
Visit the site to subscribe to the show, sign up for the mailing list, and read the show notes.
If you’ve learned something or tried out a project from the show then tell us about it! Email [email protected]) with your story.
To help other people find the show please leave a review on iTunes and tell your friends and co-workers
Join the community in the new Zulip chat workspace at dataengineeringpodcast.com/chat
Links
Pipeline Data Engineering Academy
Data Janitor 101
The Data Janitor Returns
Berlin, Germany
Hungary
Urchin google analytics precursor
AWS Redshift
Nassim Nicholas Taleb
Black Swans (affiliate link)
KISS == Keep It Simple Stupid
Dan McKinley
Ralph Kimball Data Warehousing design
Falsehoods Programmers Believe
Apache Kafka
AWS Kinesis
ETL/ELT
CI/CD
Telemetry
Dêpeche Mode
Designing Data Intensive Applications (affiliate link)
Stop Hiring DevOps Engineers and Start Growing Them
T Shaped Engineer
Pipeline Data Engineering Academy Curriculum
MPP == Massively Parallel Processing
Apache Flink
Podcast Episode
Flask web framework
YAGNI == You Ain’t Gonna Need It
Pair Programming
Clojure
The intro and outro music is from The Hug by The Freak Fandango Orchestra / CC BY-SA
Support Data Engineering Podcast
47:4022/09/2020
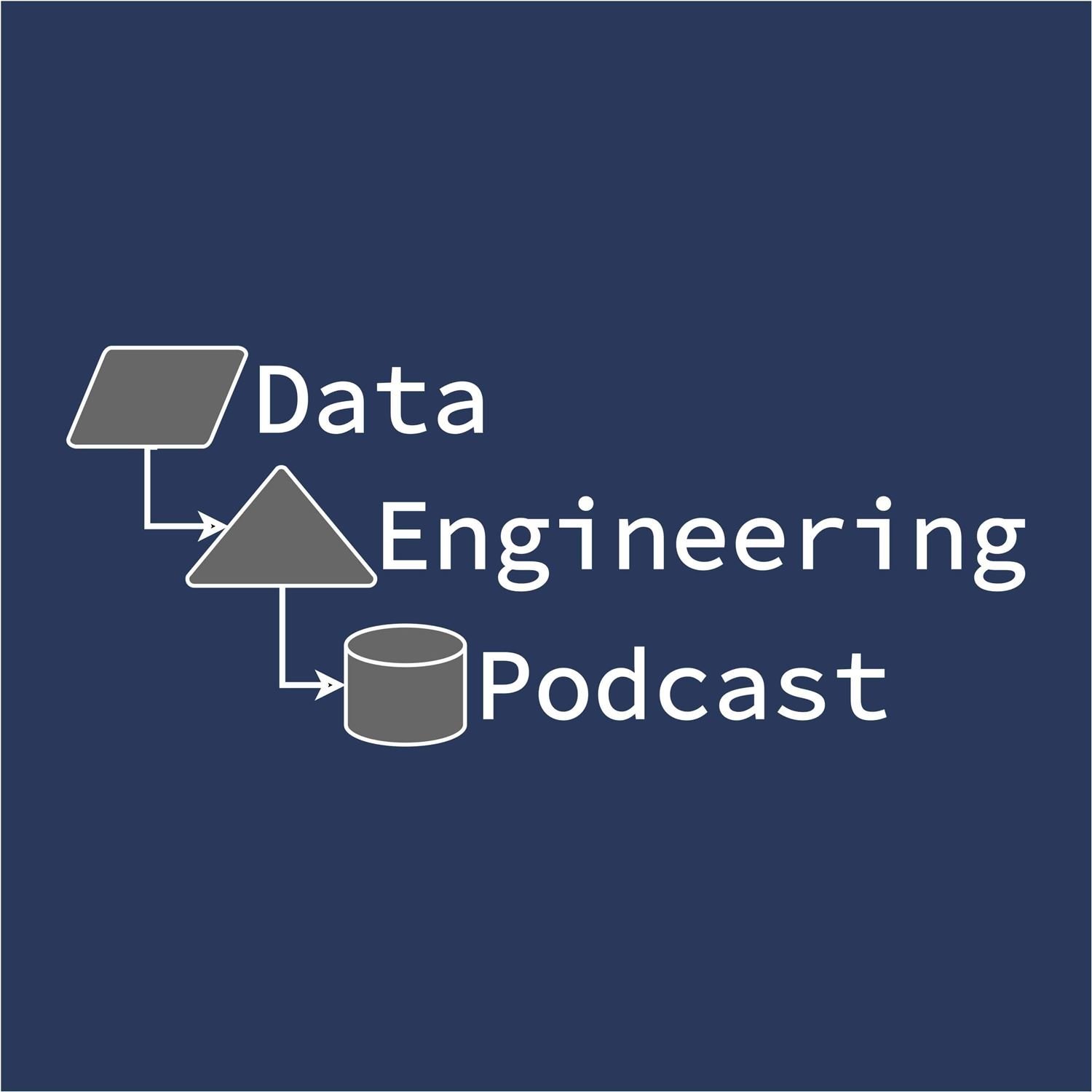
Distributed In Memory Processing And Streaming With Hazelcast
Summary
In memory computing provides significant performance benefits, but brings along challenges for managing failures and scaling up. Hazelcast is a platform for managing stateful in-memory storage and computation across a distributed cluster of commodity hardware. On top of this foundation, the Hazelcast team has also built a streaming platform for reliable high throughput data transmission. In this episode Dale Kim shares how Hazelcast is implemented, the use cases that it enables, and how it complements on-disk data management systems.
Announcements
Hello and welcome to the Data Engineering Podcast, the show about modern data management
What are the pieces of advice that you wish you had received early in your career of data engineering? If you hand a book to a new data engineer, what wisdom would you add to it? I’m working with O’Reilly on a project to collect the 97 things that every data engineer should know, and I need your help. Go to dataengineeringpodcast.com/97things to add your voice and share your hard-earned expertise.
When you’re ready to build your next pipeline, or want to test out the projects you hear about on the show, you’ll need somewhere to deploy it, so check out our friends at Linode. With their managed Kubernetes platform it’s now even easier to deploy and scale your workflows, or try out the latest Helm charts from tools like Pulsar and Pachyderm. With simple pricing, fast networking, object storage, and worldwide data centers, you’ve got everything you need to run a bulletproof data platform. Go to dataengineeringpodcast.com/linode today and get a $60 credit to try out a Kubernetes cluster of your own. And don’t forget to thank them for their continued support of this show!
Tree Schema is a data catalog that is making metadata management accessible to everyone. With Tree Schema you can create your data catalog and have it fully populated in under five minutes when using one of the many automated adapters that can connect directly to your data stores. Tree Schema includes essential cataloging features such as first class support for both tabular and unstructured data, data lineage, rich text documentation, asset tagging and more. Built from the ground up with a focus on the intersection of people and data, your entire team will find it easier to foster collaboration around your data. With the most transparent pricing in the industry – $99/mo for your entire company – and a money-back guarantee for excellent service, you’ll love Tree Schema as much as you love your data. Go to dataengineeringpodcast.com/treeschema today to get your first month free, and mention this podcast to get %50 off your first three months after the trial.
You listen to this show to learn and stay up to date with what’s happening in databases, streaming platforms, big data, and everything else you need to know about modern data platforms. For more opportunities to stay up to date, gain new skills, and learn from your peers there are a growing number of virtual events that you can attend from the comfort and safety of your home. Go to dataengineeringpodcast.com/conferences to check out the upcoming events being offered by our partners and get registered today!
Your host is Tobias Macey and today I’m interviewing Dale Kim about Hazelcast, a distributed in-memory computing platform for data intensive applications
Interview
Introduction
How did you get involved in the area of data management?
Can you start by describing what Hazelcast is and its origins?
What are the benefits and tradeoffs of in-memory computation for data-intensive workloads?
What are some of the common use cases for the Hazelcast in memory grid?
How is Hazelcast implemented?
How has the architecture evolved since it was first created?
How is the Jet streaming framework architected?
What was the motivation for building it?
How do the capabilities of Jet compare to systems such as Flink or Spark Streaming?
How has the introduction of hardware capabilities such as NVMe drives influenced the market for in-memory systems?
How is the governance of the open source grid and Jet projects handled?
What is the guiding heuristic for which capabilities or features to include in the open source projects vs. the commercial offerings?
What is involved in building an application or workflow on top of Hazelcast?
What are the common patterns for engineers who are building on top of Hazelcast?
What is involved in deploying and maintaining an installation of the Hazelcast grid or Jet streaming?
What are the scaling factors for Hazelcast?
What are the edge cases that users should be aware of?
What are some of the most interesting, innovative, or unexpected ways that you have seen Hazelcast used?
When is Hazelcast Grid or Jet the wrong choice?
What is in store for the future of Hazelcast?
Contact Info
LinkedIn
Parting Question
From your perspective, what is the biggest gap in the tooling or technology for data management today?
Closing Announcements
Thank you for listening! Don’t forget to check out our other show, Podcast.__init__ to learn about the Python language, its community, and the innovative ways it is being used.
Visit the site to subscribe to the show, sign up for the mailing list, and read the show notes.
If you’ve learned something or tried out a project from the show then tell us about it! Email [email protected]) with your story.
To help other people find the show please leave a review on iTunes and tell your friends and co-workers
Join the community in the new Zulip chat workspace at dataengineeringpodcast.com/chat
Links
HazelCast
Istanbul
Apache Spark
OrientDB
CAP Theorem
NVMe
Memristors
Intel Optane Persistent Memory
Hazelcast Jet
Kappa Architecture
IBM Cloud Paks
Digital Integration Hub (Gartner)
The intro and outro music is from The Hug by The Freak Fandango Orchestra / CC BY-SA
Support Data Engineering Podcast
44:0715/09/2020
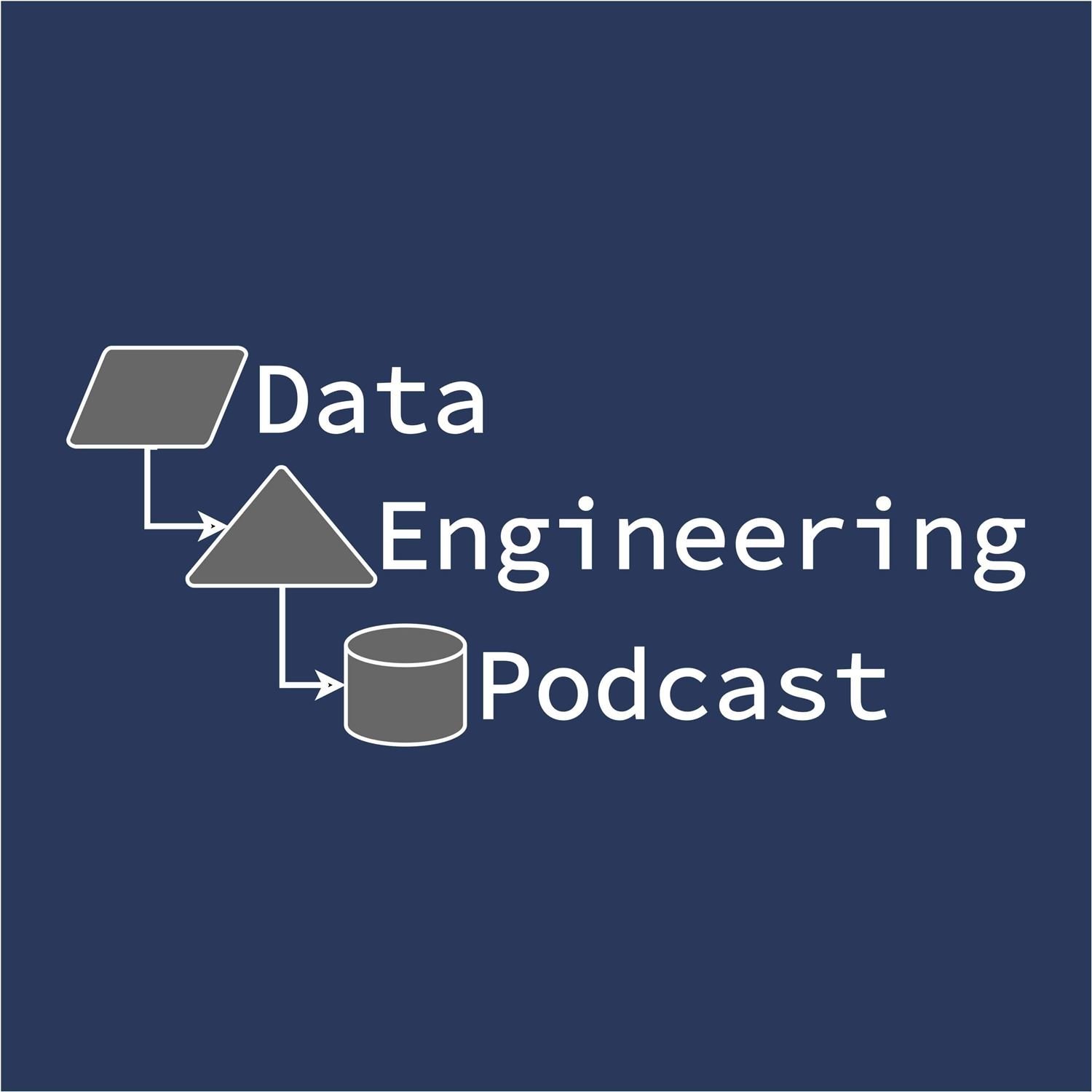
Simplify Your Data Architecture With The Presto Distributed SQL Engine
Summary
Databases are limited in scope to the information that they directly contain. For analytical use cases you often want to combine data across multiple sources and storage locations. This frequently requires cumbersome and time-consuming data integration. To address this problem Martin Traverso and his colleagues at Facebook built the Presto distributed query engine. In this episode he explains how it is designed to allow for querying and combining data where it resides, the use cases that such an architecture unlocks, and the innovative ways that it is being employed at companies across the world. If you need to work with data in your cloud data lake, your on-premise database, or a collection of flat files, then give this episode a listen and then try out Presto today.
Announcements
Hello and welcome to the Data Engineering Podcast, the show about modern data management
What are the pieces of advice that you wish you had received early in your career of data engineering? If you hand a book to a new data engineer, what wisdom would you add to it? I’m working with O’Reilly on a project to collect the 97 things that every data engineer should know, and I need your help. Go to dataengineeringpodcast.com/97things to add your voice and share your hard-earned expertise.
When you’re ready to build your next pipeline, or want to test out the projects you hear about on the show, you’ll need somewhere to deploy it, so check out our friends at Linode. With their managed Kubernetes platform it’s now even easier to deploy and scale your workflows, or try out the latest Helm charts from tools like Pulsar and Pachyderm. With simple pricing, fast networking, object storage, and worldwide data centers, you’ve got everything you need to run a bulletproof data platform. Go to dataengineeringpodcast.com/linode today and get a $60 credit to try out a Kubernetes cluster of your own. And don’t forget to thank them for their continued support of this show!
You listen to this show to learn and stay up to date with what’s happening in databases, streaming platforms, big data, and everything else you need to know about modern data platforms. For more opportunities to stay up to date, gain new skills, and learn from your peers there are a growing number of virtual events that you can attend from the comfort and safety of your home. Go to dataengineeringpodcast.com/conferences to check out the upcoming events being offered by our partners and get registered today!
Your host is Tobias Macey and today I’m interviewing Martin Traverso about PrestoSQL, a distributed SQL engine that queries data in place
Interview
Introduction
How did you get involved in the area of data management?
Can you start by giving an overview of what Presto is and its origin story?
What was the motivation for releasing Presto as open source?
For someone who is responsible for architecting their organization’s data platform, what are some of the signals that Presto will be a good fit for them?
What are the primary ways that Presto is being used?
I interviewed your colleague at Starburst, Kamil 2 years ago. How has Presto changed or evolved in that time, both technically and in terms of community and ecosystem growth?
What are some of the deployment and scaling considerations that operators of Presto should be aware of?
What are the best practices that have been established for working with data through Presto in terms of centralizing in a data lake vs. federating across disparate storage locations?
What are the tradeoffs of using Presto on top of a data lake vs a vertically integrated warehouse solution?
When designing the layout of a data lake that will be interacted with via Presto, what are some of the data modeling considerations that can improve the odds of success?
What are some of the most interesting, unexpected, or innovative ways that you have seen Presto used?
What are the most interesting, unexpected, or challenging lessons that you have learned while building, growing, and supporting the Presto project?
When is Presto the wrong choice?
What is in store for the future of the Presto project and community?
Contact Info
LinkedIn
@mtraverso on Twitter
martint on GitHub
Parting Question
From your perspective, what is the biggest gap in the tooling or technology for data management today?
Closing Announcements
Thank you for listening! Don’t forget to check out our other show, Podcast.__init__ to learn about the Python language, its community, and the innovative ways it is being used.
Visit the site to subscribe to the show, sign up for the mailing list, and read the show notes.
If you’ve learned something or tried out a project from the show then tell us about it! Email [email protected]) with your story.
To help other people find the show please leave a review on iTunes and tell your friends and co-workers
Join the community in the new Zulip chat workspace at dataengineeringpodcast.com/chat
Links
Presto
Starburst Data
Podcast Episode
Hadoop
Hive
Glue Metastore
BigQuery
Kinesis
Apache Pinot
Elasticsearch
ORC
Parquet
AWS Redshift
Avro
Podcast Episode
LZ4
Zstandard
KafkaSQL
Flink
Podcast Episode
PyTorch
Podcast.__init__ Episode
Tensorflow
Spark
The intro and outro music is from The Hug by The Freak Fandango Orchestra / CC BY-SA
Support Data Engineering Podcast
53:5907/09/2020
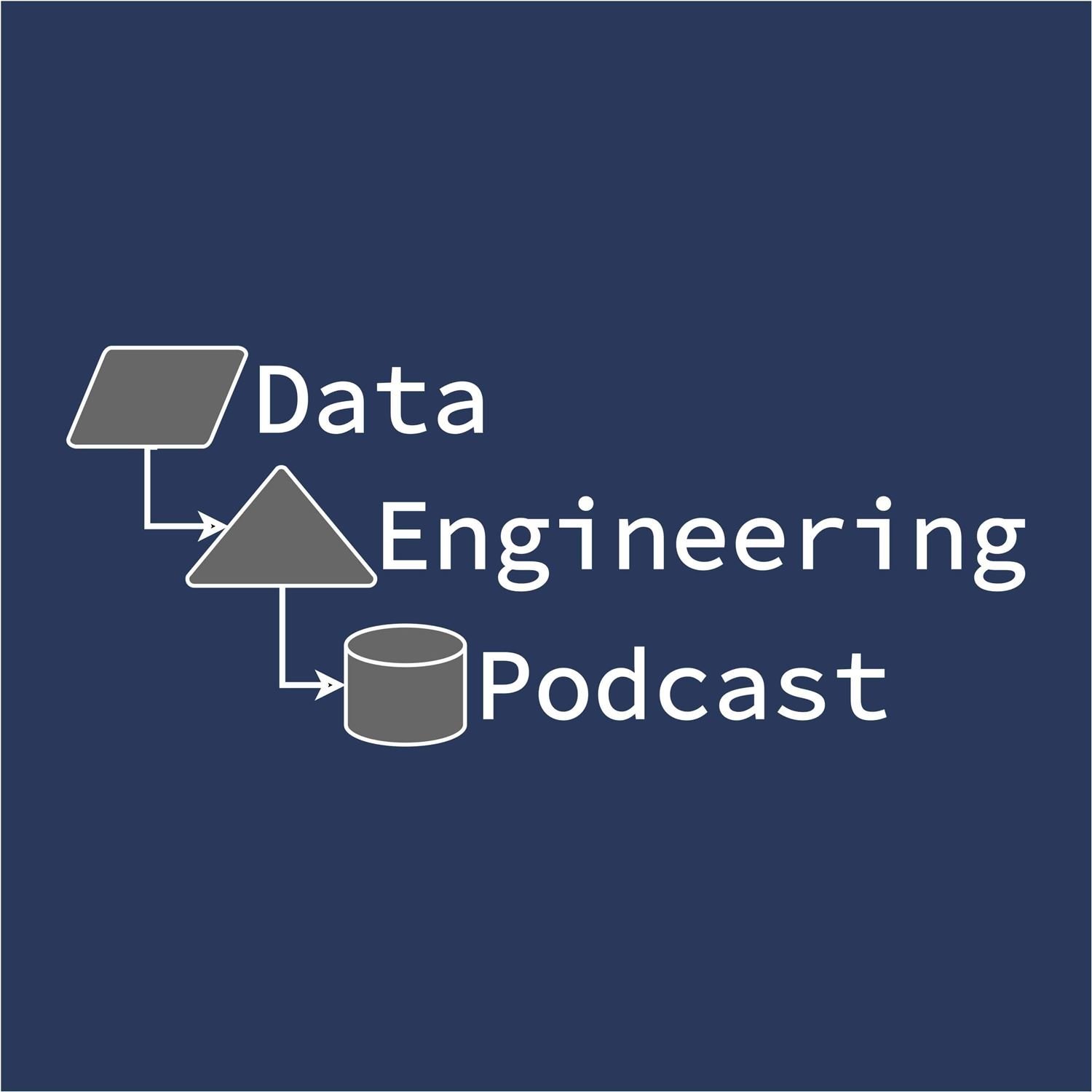
Building A Better Data Warehouse For The Cloud At Firebolt
Summary
Data warehouse technology has been around for decades and has gone through several generational shifts in that time. The current trends in data warehousing are oriented around cloud native architectures that take advantage of dynamic scaling and the separation of compute and storage. Firebolt is taking that a step further with a core focus on speed and interactivity. In this episode CEO and founder Eldad Farkash explains how the Firebolt platform is architected for high throughput, their simple and transparent pricing model to encourage widespread use, and the use cases that it unlocks through interactive query speeds.
Announcements
Hello and welcome to the Data Engineering Podcast, the show about modern data management
What are the pieces of advice that you wish you had received early in your career of data engineering? If you hand a book to a new data engineer, what wisdom would you add to it? I’m working with O’Reilly on a project to collect the 97 things that every data engineer should know, and I need your help. Go to dataengineeringpodcast.com/97things to add your voice and share your hard-earned expertise.
When you’re ready to build your next pipeline, or want to test out the projects you hear about on the show, you’ll need somewhere to deploy it, so check out our friends at Linode. With their managed Kubernetes platform it’s now even easier to deploy and scale your workflows, or try out the latest Helm charts from tools like Pulsar and Pachyderm. With simple pricing, fast networking, object storage, and worldwide data centers, you’ve got everything you need to run a bulletproof data platform. Go to dataengineeringpodcast.com/linode today and get a $60 credit to try out a Kubernetes cluster of your own. And don’t forget to thank them for their continued support of this show!
Today’s episode of the Data Engineering Podcast is sponsored by Datadog, a SaaS-based monitoring and analytics platform for cloud-scale infrastructure, applications, logs, and more. Datadog uses machine-learning based algorithms to detect errors and anomalies across your entire stack—which reduces the time it takes to detect and address outages and helps promote collaboration between Data Engineering, Operations, and the rest of the company.
Go to dataengineeringpodcast.com/datadog today to start your free 14 day trial. If you start a trial and install Datadog’s agent, Datadog will send you a free T-shirt.
You listen to this show to learn and stay up to date with what’s happening in databases, streaming platforms, big data, and everything else you need to know about modern data platforms. For more opportunities to stay up to date, gain new skills, and learn from your peers there are a growing number of virtual events that you can attend from the comfort and safety of your home. Go to dataengineeringpodcast.com/conferences to check out the upcoming events being offered by our partners and get registered today!
Your host is Tobias Macey and today I’m interviewing Eldad Farkash about Firebolt, a cloud data warehouse optimized for speed and elasticity on structured and semi-structured data
Interview
Introduction
How did you get involved in the area of data management?
Can you start by describing what Firebolt is and your motivation for building it?
How does Firebolt compare to other data warehouse technologies what unique features does it provide?
The lines between a data warehouse and a data lake have been blurring in recent years. Where on that continuum does Firebolt lie?
What are the unique use cases that Firebolt allows for?
How do the performance characteristics of Firebolt change the ways that an engineer should think about data modeling?
What technologies might someone replace with Firebolt?
How is Firebolt architected and how has the design evolved since you first began working on it?
What are some of the most challenging aspects of building a data warehouse platform that is optimized for speed?
How do you handle support for nested and semi-structured data?
In what ways have you found it necessary/useful to extend SQL?
Due to the immutability of object storage, for data lakes the update or delete process involves reprocessing a potentially large amount of data. How do you approach that in Firebolt with your F3 format?
What have you found to be the most interesting, unexpected, or challenging lessons while building and scaling the Firebolt platform and business?
When is Firebolt the wrong choice?
What do you have planned for the future of Firebolt?
Contact Info
LinkedIn
Parting Question
From your perspective, what is the biggest gap in the tooling or technology for data management today?
Closing Announcements
Thank you for listening! Don’t forget to check out our other show, Podcast.__init__ to learn about the Python language, its community, and the innovative ways it is being used.
Visit the site to subscribe to the show, sign up for the mailing list, and read the show notes.
If you’ve learned something or tried out a project from the show then tell us about it! Email [email protected]) with your story.
To help other people find the show please leave a review on iTunes and tell your friends and co-workers
Join the community in the new Zulip chat workspace at dataengineeringpodcast.com/chat
Links
Firebolt
Sisense
SnowflakeDB
Podcast Episode
Redshift
Spark
Podcast Episode
Parquet
Podcast Episode
Hadoop
HDFS
S3
AWS Athena
BigQuery
Data Vault
Podcast Episode
Star Schema
Dimensional Modeling
Slowly Changing Dimensions
JDBC
TPC Benchmarks
DBT
Podcast Episode
Tableau
Looker
Podcast Episode
PrestoSQL
Podcast Episode
PostgreSQL
Podcast Episode
FoundationDB
Podcast Episode
The intro and outro music is from The Hug by The Freak Fandango Orchestra / CC BY-SA
Support Data Engineering Podcast
01:05:5101/09/2020
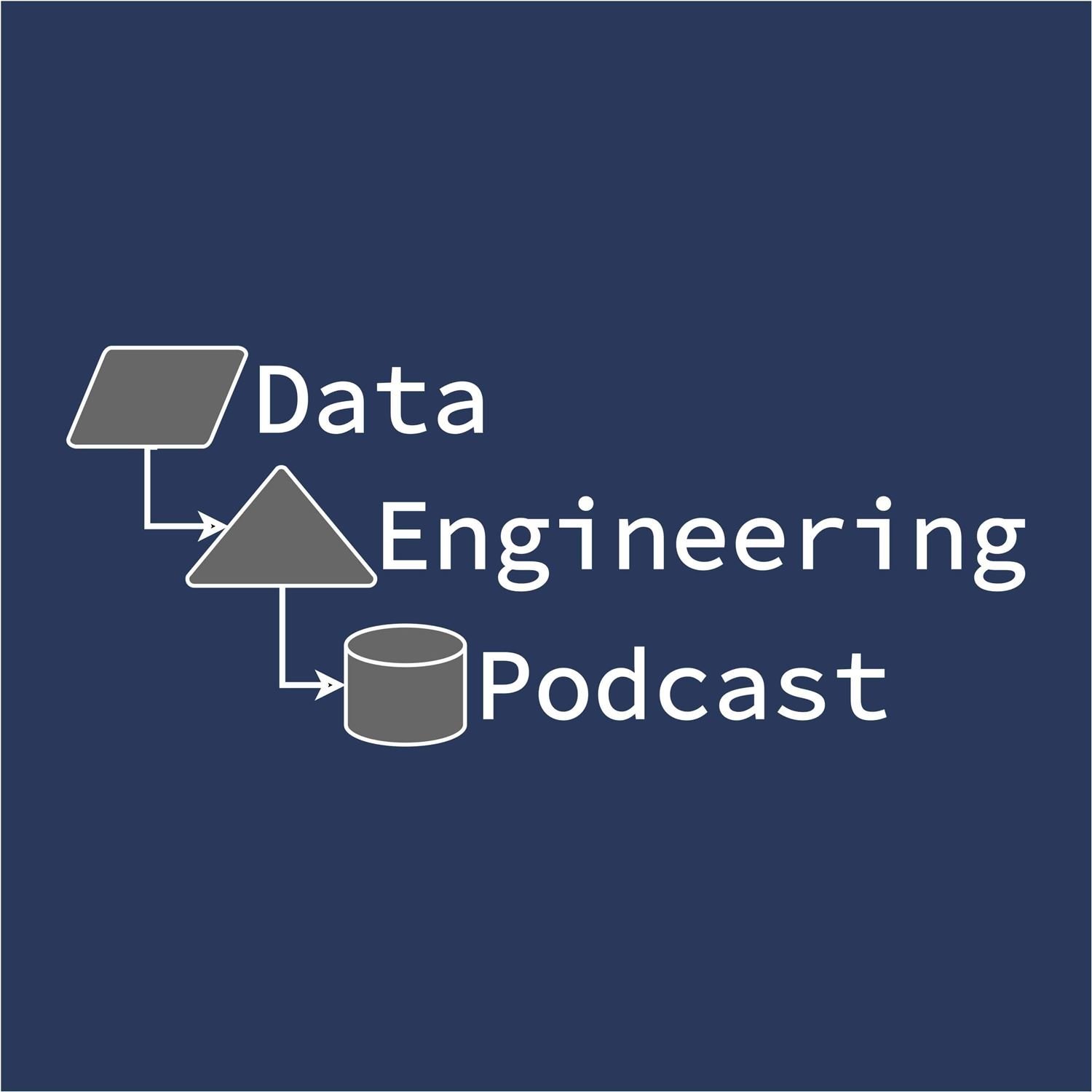
Metadata Management And Integration At LinkedIn With DataHub
Summary
In order to scale the use of data across an organization there are a number of challenges related to discovery, governance, and integration that need to be solved. The key to those solutions is a robust and flexible metadata management system. LinkedIn has gone through several iterations on the most maintainable and scalable approach to metadata, leading them to their current work on DataHub. In this episode Mars Lan and Pardhu Gunnam explain how they designed the platform, how it integrates into their data platforms, and how it is being used to power data discovery and analytics at LinkedIn.
Announcements
Hello and welcome to the Data Engineering Podcast, the show about modern data management
What are the pieces of advice that you wish you had received early in your career of data engineering? If you hand a book to a new data engineer, what wisdom would you add to it? I’m working with O’Reilly on a project to collect the 97 things that every data engineer should know, and I need your help. Go to dataengineeringpodcast.com/97things to add your voice and share your hard-earned expertise.
When you’re ready to build your next pipeline, or want to test out the projects you hear about on the show, you’ll need somewhere to deploy it, so check out our friends at Linode. With their managed Kubernetes platform it’s now even easier to deploy and scale your workflows, or try out the latest Helm charts from tools like Pulsar and Pachyderm. With simple pricing, fast networking, object storage, and worldwide data centers, you’ve got everything you need to run a bulletproof data platform. Go to dataengineeringpodcast.com/linode today and get a $60 credit to try out a Kubernetes cluster of your own. And don’t forget to thank them for their continued support of this show!
If you’ve been exploring scalable, cost-effective and secure ways to collect and route data across your organization, RudderStack is the only solution that helps you turn your own warehouse into a state of the art customer data platform. Their mission is to empower data engineers to fully own their customer data infrastructure and easily push value to other parts of the organization, like marketing and product management. With their open-source foundation, fixed pricing, and unlimited volume, they are enterprise ready, but accessible to everyone. Go to dataengineeringpodcast.com/rudder to request a demo and get one free month of access to the hosted platform along with a free t-shirt.
You listen to this show to learn and stay up to date with what’s happening in databases, streaming platforms, big data, and everything else you need to know about modern data platforms. For more opportunities to stay up to date, gain new skills, and learn from your peers there are a growing number of virtual events that you can attend from the comfort and safety of your home. Go to dataengineeringpodcast.com/conferences to check out the upcoming events being offered by our partners and get registered today!
Your host is Tobias Macey and today I’m interviewing Pardhu Gunnam and Mars Lan about DataHub, LinkedIn’s metadata management and data catalog platform
Interview
Introduction
How did you get involved in the area of data management?
Can you start by giving an overview of what DataHub is and some of its back story?
What were you using at LinkedIn for metadata management prior to the introduction of DataHub?
What was lacking in the previous solutions that motivated you to create a new platform?
There are a large number of other systems available for building data catalogs and tracking metadata, both open source and proprietary. What are the features of DataHub that would lead someone to use it in place of the other options?
Who is the target audience for DataHub?
How do the needs of those end users influence or constrain your approach to the design and interfaces provided by DataHub?
Can you describe how DataHub is architected?
How has it evolved since you first began working on it?
What was your motivation for releasing DataHub as an open source project?
What have been the benefits of that decision?
What are the challenges that you face in maintaining changes between the public repository and your internally deployed instance?
What is the workflow for populating metadata into DataHub?
What are the challenges that you see in managing the format of metadata and establishing consistent models for the information being stored?
How do you handle discovery of data assets for users of DataHub?
What are the integration and extension points of the platform?
What is involved in deploying and maintaining and instance of the DataHub platform?
What are some of the most interesting or unexpected ways that you have seen DataHub used inside or outside of LinkedIn?
What are some of the most interesting, unexpected, or challenging lessons that you learned while building and working with DataHub?
When is DataHub the wrong choice?
What do you have planned for the future of the project?
Contact Info
Mars
LinkedIn
mars-lan on GitHub
Pardhu
LinkedIn
Parting Question
From your perspective, what is the biggest gap in the tooling or technology for data management today?
Closing Announcements
Thank you for listening! Don’t forget to check out our other show, Podcast.__init__ to learn about the Python language, its community, and the innovative ways it is being used.
Visit the site to subscribe to the show, sign up for the mailing list, and read the show notes.
If you’ve learned something or tried out a project from the show then tell us about it! Email [email protected]) with your story.
To help other people find the show please leave a review on iTunes and tell your friends and co-workers
Join the community in the new Zulip chat workspace at dataengineeringpodcast.com/chat
Links
DataHub
Map/Reduce
Apache Flume
LinkedIn Blog Post introducing DataHub
WhereHows
Hive Metastore
Kafka
CDC == Change Data Capture
Podcast Episode
PDL LinkedIn language
GraphQL
Elasticsearch
Neo4J
Apache Pinot
Apache Gobblin
Apache Samza
Open Sourcing DataHub Blog Post
The intro and outro music is from The Hug by The Freak Fandango Orchestra / CC BY-SA
Support Data Engineering Podcast
51:0425/08/2020