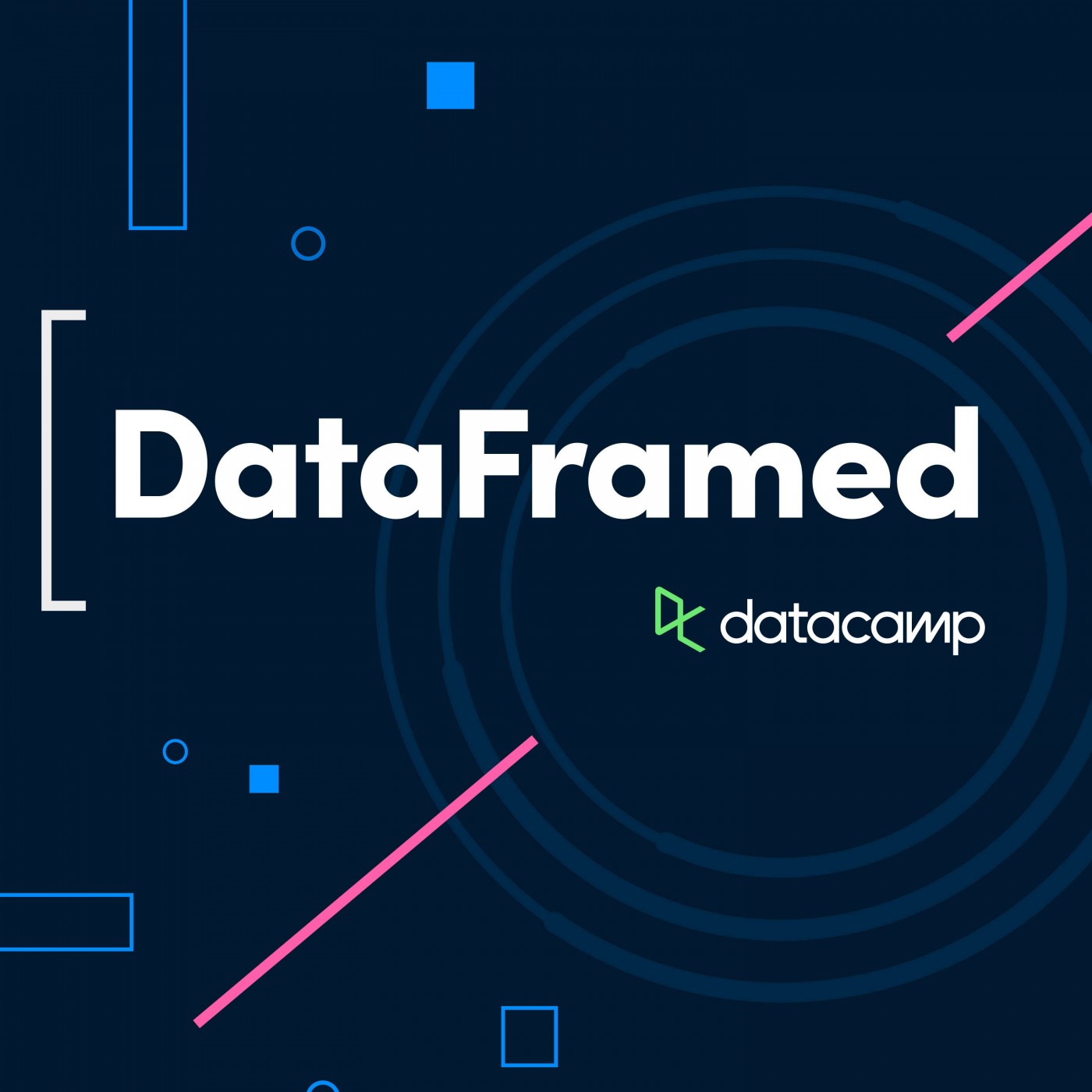
Technology
Business
DataCamp
Welcome to DataFramed, a weekly podcast exploring how artificial intelligence and data are changing the world around us. On this show, we invite data & AI leaders at the forefront of the data revolution to share their insights and experiences into how they lead the charge in this era of AI. Whether you're a beginner looking to gain insights into a career in data & AI, a practitioner needing to stay up-to-date on the latest tools and trends, or a leader looking to transform how your organization uses data & AI, there's something here for everyone.
Join co-hosts Adel Nehme and Richie Cotton as they delve into the stories and ideas that are shaping the future of data. Subscribe to the show and tune in to the latest episode on the feed below.
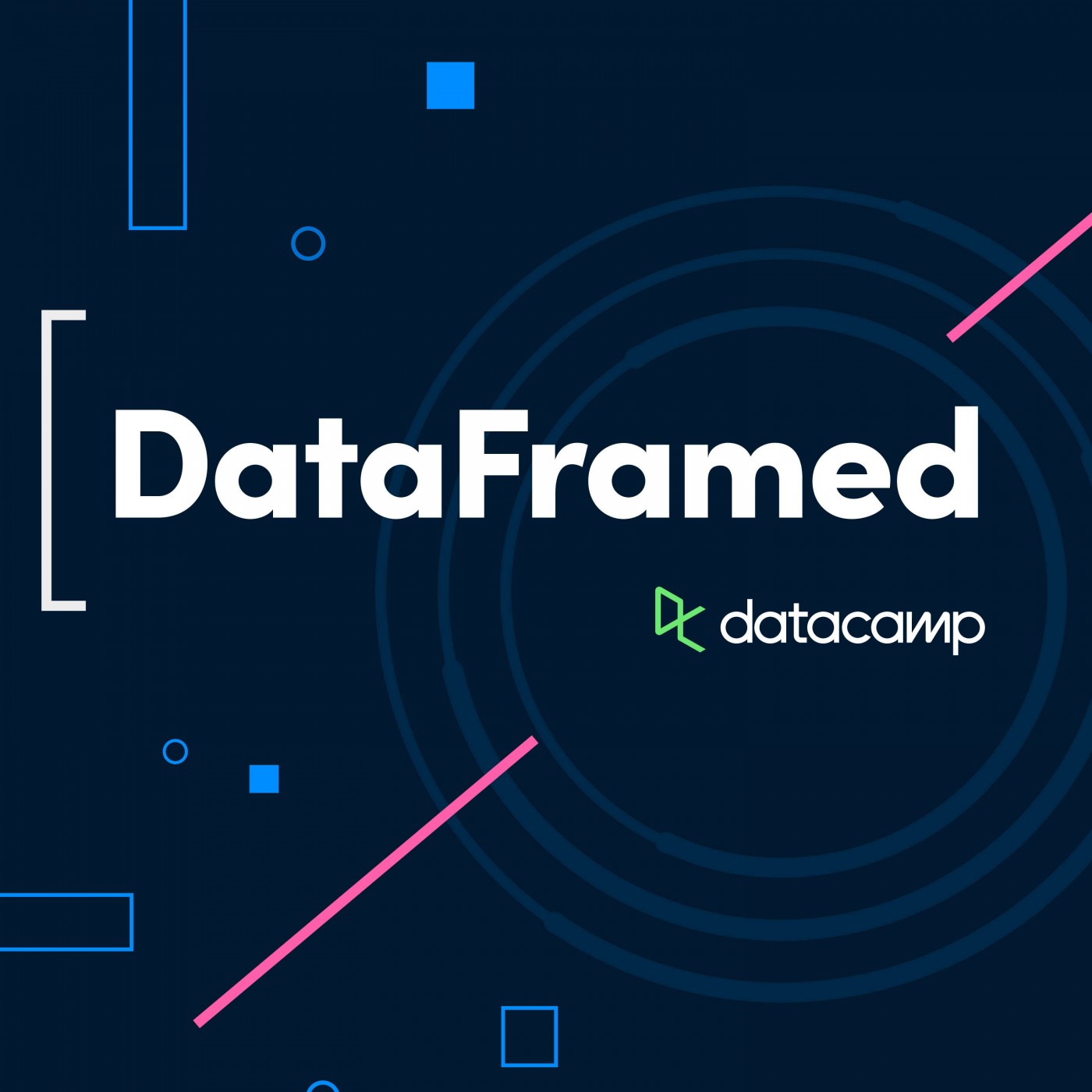
#76 Providing Financial Inclusion with Data Science, with Vishnu V Ram, VP of Data Science and Engineering at Credit Karma
In this episode of DataFramed, we speak with Vishnu V Ram, VP of Data Science and Engineering at Credit Karma about how data science is being leveraged to increase financial inclusion.
Throughout the episode, Vishnu discusses his background, Credit Karma’s mission, how data science is being used at Credit Karma to lower the barrier to entry for financial products, how he managed a data team through rapid growth, transitioning to Google Cloud, exciting trends in data science, and more.
Relevant links from the interview:
You can now learn data science with your team for free—try out DataCamp Professional with our 14-day free trial. Data roles at Credit KarmaCredit Karma’s mission
52:2929/11/2021
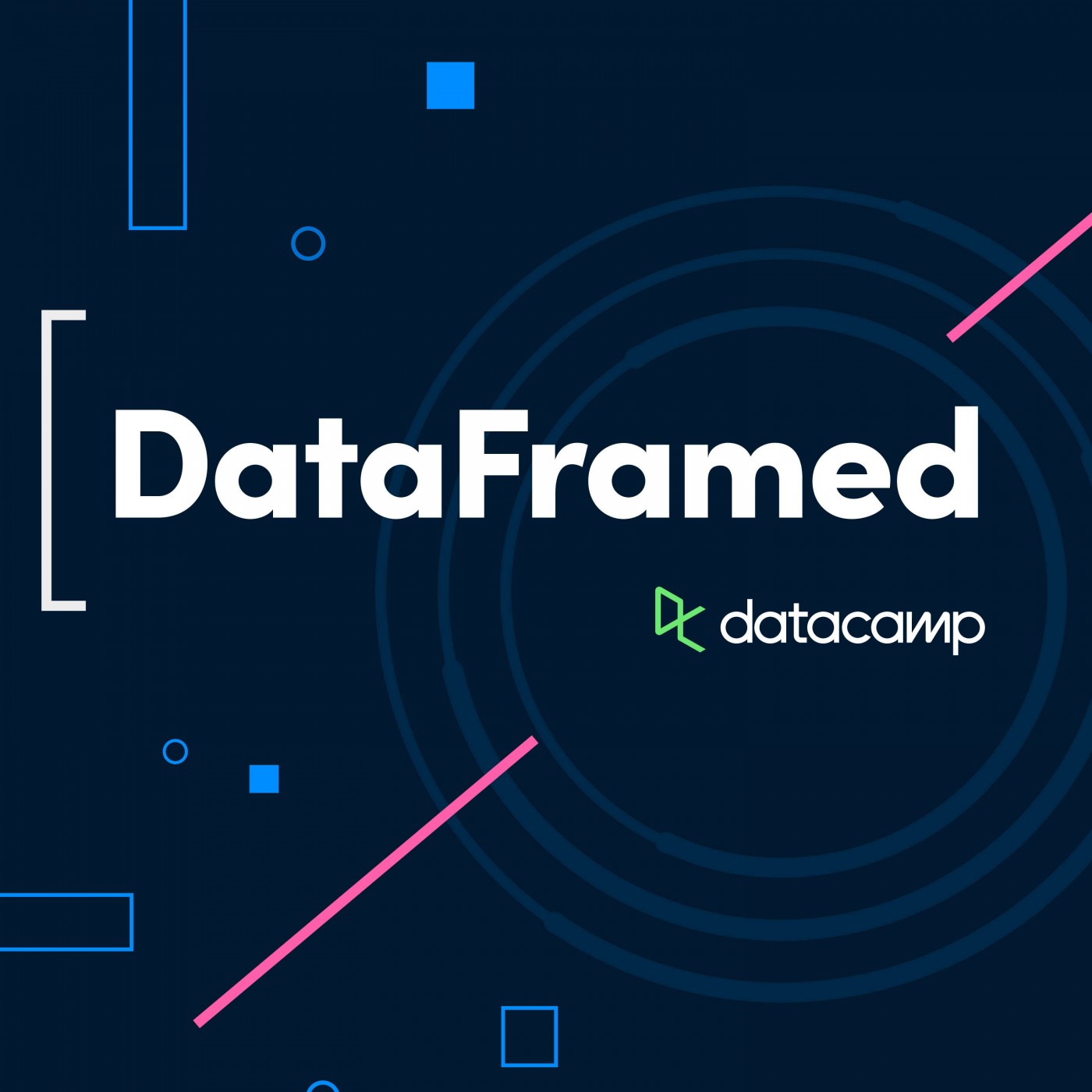
#75 The Data Storytelling Skills Data Teams Need with Andy Cotgreave, Technical Evangelist at Tableau
In this episode of DataFramed, we speak with Andy Cotgreave, Technical Evangelist at Tableau about the role of data storytelling when driving change with analytics, and the importance of the analyst role within a data-driven organization.
Throughout the episode, Andy discusses his background, the skills every analyst should know to equip organizations with better data-driven decision making, his best practices for data storytelling, how he thinks about data literacy and ways to spread it within the organization, the importance of community when creating a data-driven organization, and more.
Relevant links from the interview:
We’d love your feedback! Let us know which topics you’d like us to cover and what you think of DataFramed by answering this 30-second surveyCheck out our upcoming webinar with AndyCheck out Andy's bookBecome a Tableau expert
50:5615/11/2021
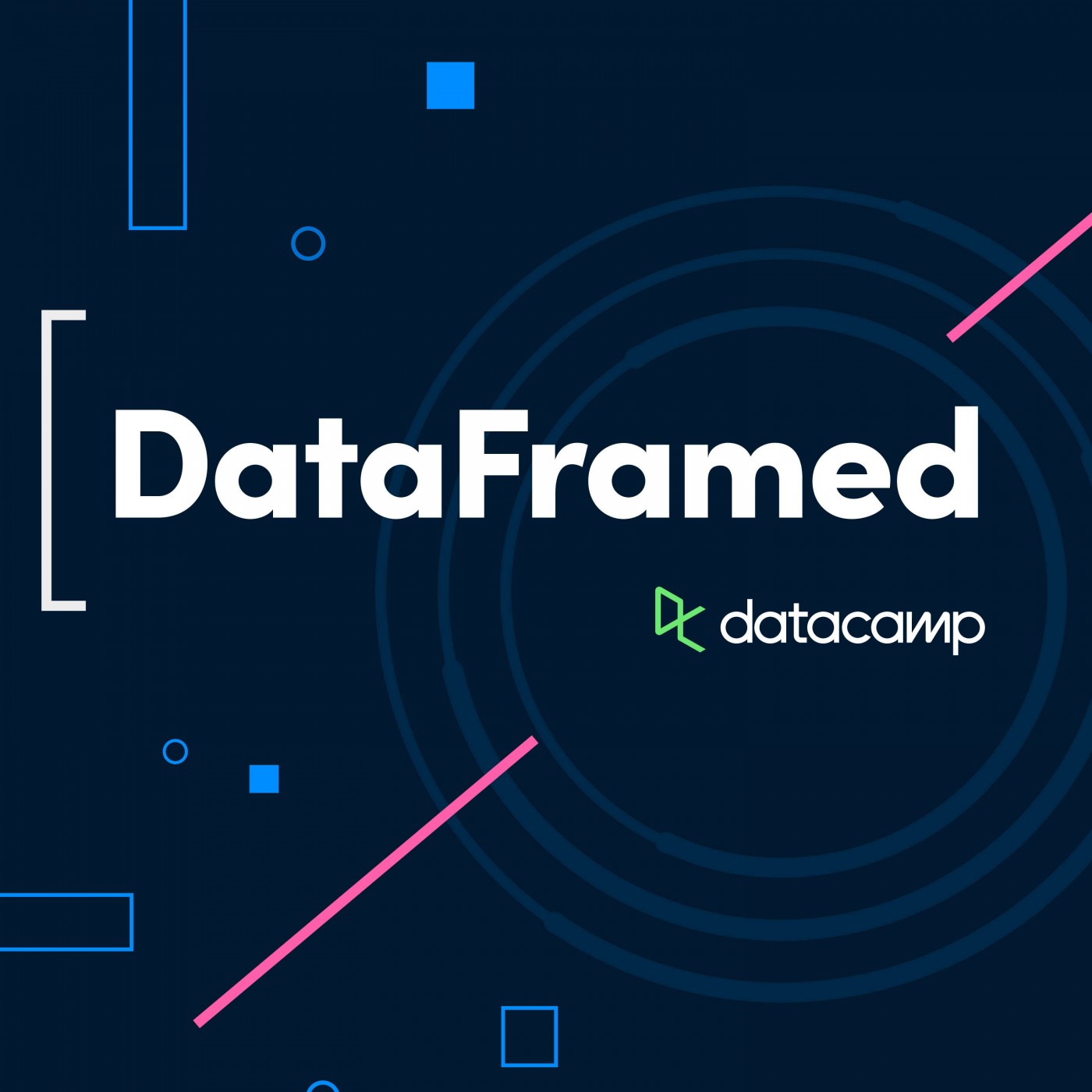
#74 Harnessing the Power of Collaboration with Engineering Manager at Lucid Software, Brian Campbell
In this episode of DataFramed, we speak with Brian Campbell, Engineering Manager at Lucid Software about managing data science projects effectively and harnessing the power of collaboration. Throughout the episode, Brian discusses his background, how data leaders can become better collaborators, data science project management best practices, the type of collaborators data teams should seek out, the latest innovations in the data engineering tooling space, and more.
Relevant links from the interview:
We’d love your feedback! Let us know which topics you’d like us to cover and what you think of DataFramed by answering this 30-second surveyLucid’s Tech Blog
33:5001/11/2021
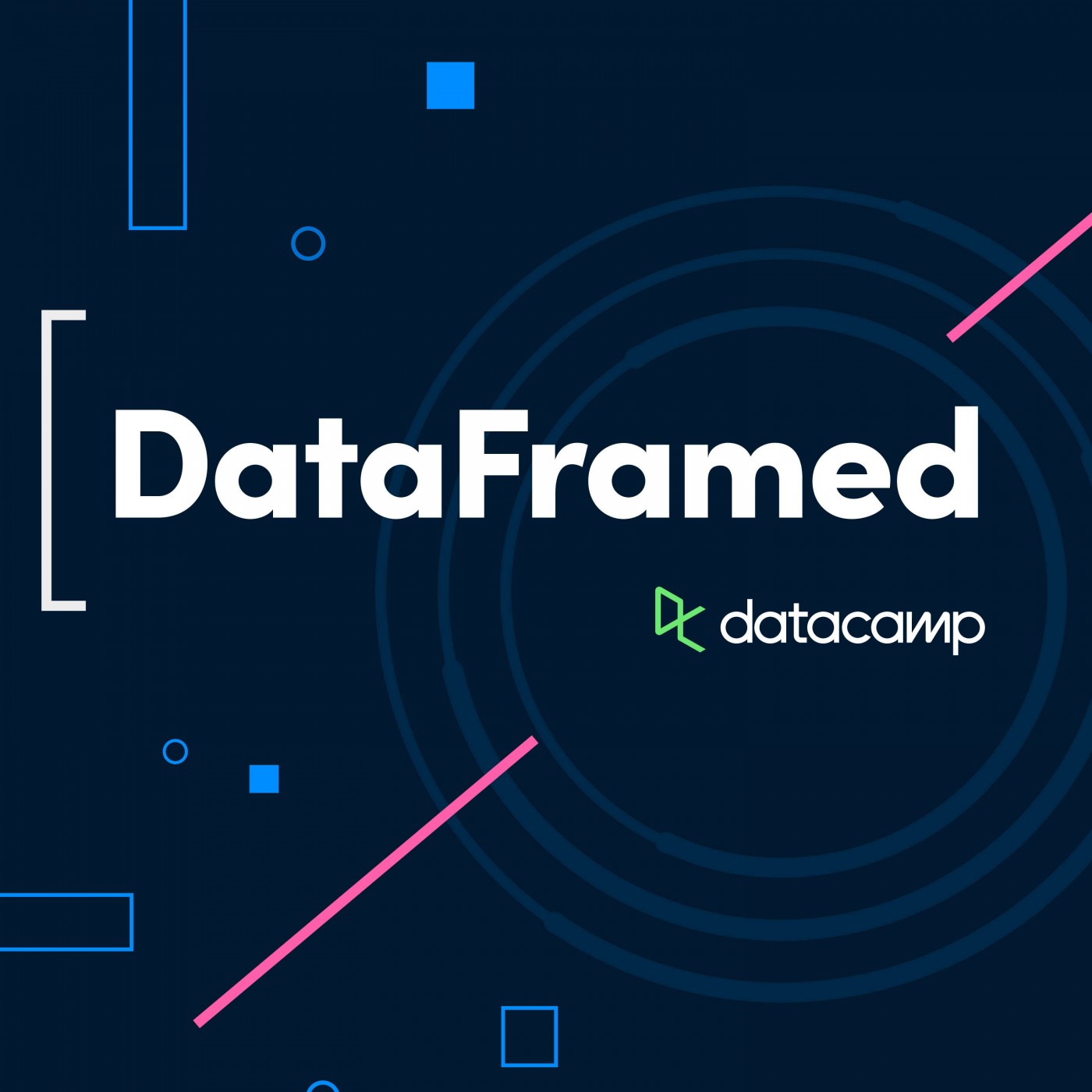
#73 Scaling AI Adoption in Financial Services with Chief Strategy Officer and Head of Financial Services at TruEra Shameek Kundu
In this episode of DataFramed, we speak with Shameek Kundu, former group CDO at Standard Chartered Bank, and Chief Strategy Officer & Head of Financial Services at TruEra Inc about Scaling AI Adoption throughout financial services.
Throughout the episode, Shameek discusses his background, the state of data transformation in financial services, the depth vs breadth of machine learning operationalization in financial services today, the challenges standing in the way of scalable AI adoption in the industry, the importance of data literacy, the trust and responsibility challenge of AI, the future of data science in financial services, and more.
Relevant links from the interview:
We’d love your feedback! Let us know which topics you’d like us to cover and what you think of DataFramed by answering this 30-second surveyCheck out TruEra in actionBank of England Report: The impact of Covid on machine learning and data science in UK BankingMIT Tech Review — Hundreds of AI tools have been built to catch covid. None of them helped
01:00:5218/10/2021
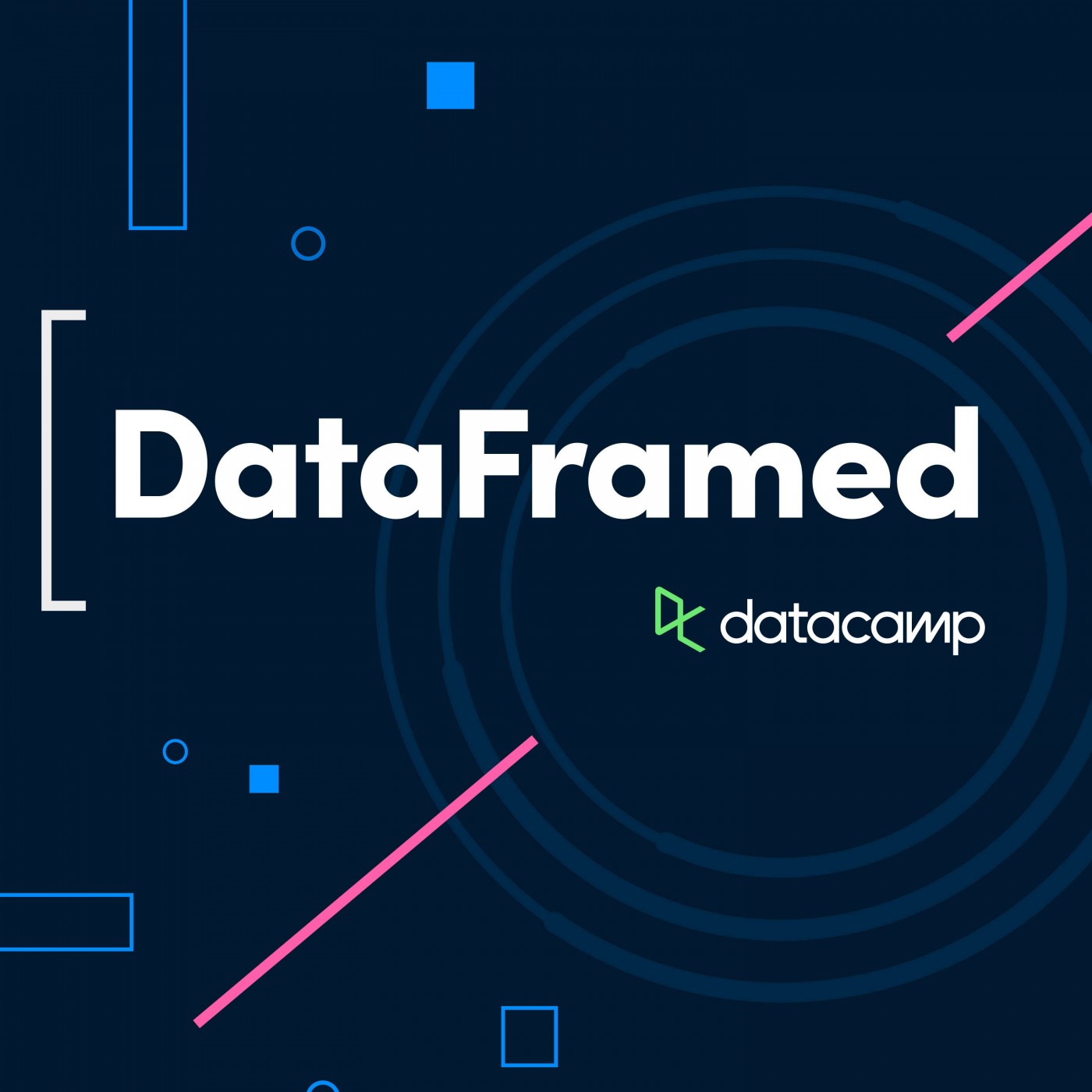
#72 Building High Performing Data Teams with Syafri Bahar, VP of Data Science at Gojek
In this episode of DataFramed, we speak with Syafri Bahar, VP of Data Science at Gojek about building high-performing data teams, and how data science is central to Gojek’s success.
Throughout the episode, Syafri discusses his background, the hallmarks of a high-performance data team, how he measures the ROI on data activities, the skills needed in every successful data team, what is the best organizational model for data mature organizations, how Covid-19 affected Gojek’s data teams, his thoughts on data literacy and governance, future trends in data science and AI, and why data scientists should sharpen their maths and machine learning skills in an age of increasing automation.
Relevant links from the interview:
We’d love your feedback! Let us know which topics you’d like us to cover and what you think of DataFramed by answering this 30-second surveyGojek’s Data Blog
50:5504/10/2021
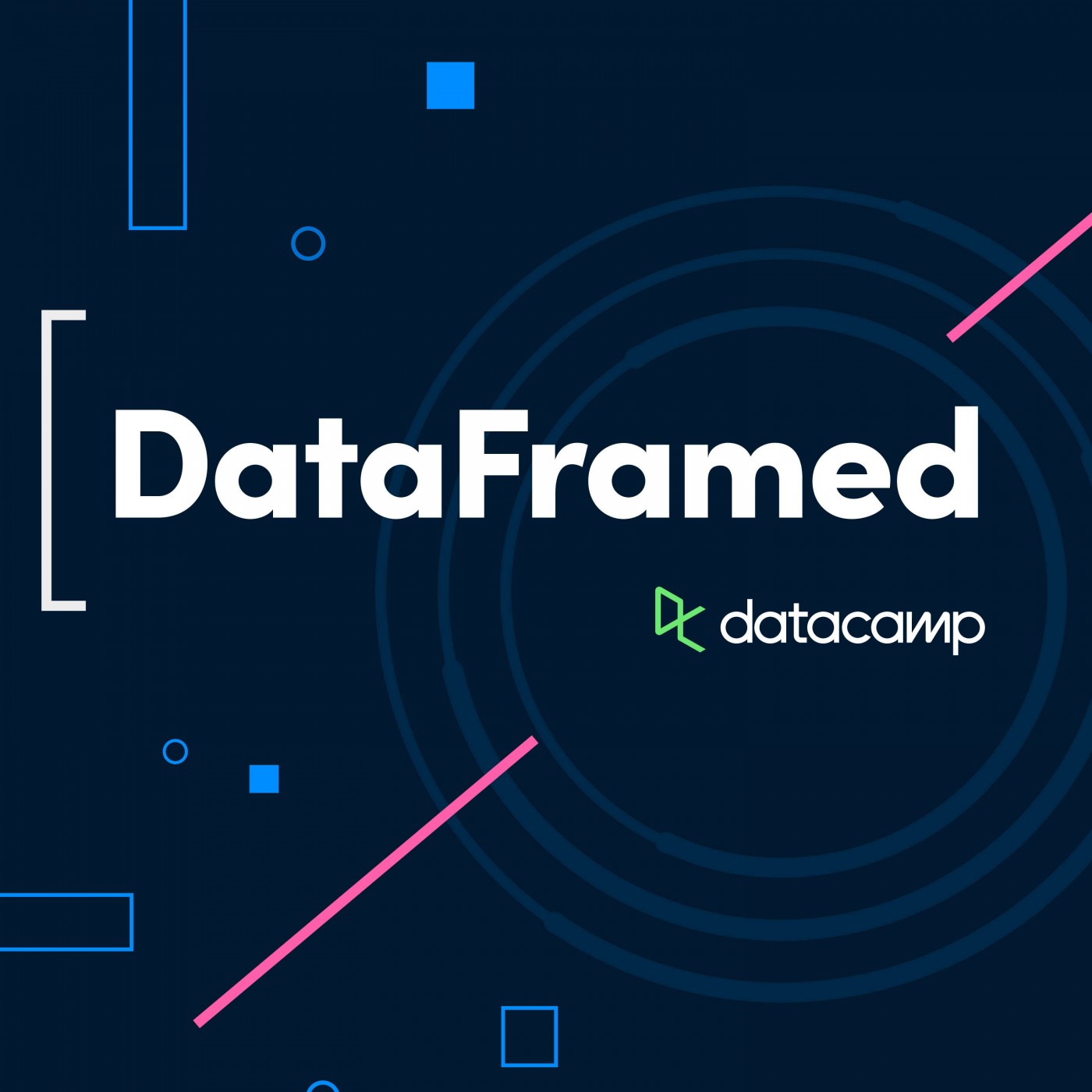
#71 Scaling Machine Learning Adoption: A Pragmatic Approach
In this episode of DataFramed, we speak with Noah Gift, founder of Pragmatic AI Labs and prolific author about operationalizing machine learning in organizations and his new book Practical MLOPs.
Throughout the episode, Noah discusses his background, his philosophy around pragmatic AI, the differences between data science in academia and the real world, how data scientists can become more action-oriented by creating solutions that solve real-world problems, the importance of dev-ops, his most recent book on the practical guide to MLOps, how data science can be compared to Brazilian jiu-jitsu, what data scientists should learn to scale the amount of value they deliver, his thoughts on auto-ml and automation, and more.
Relevant links from the interview:
We’d love your feedback! Let us know which topics you’d like us to cover and what you think of DataFramed by answering this 30-second surveyUnsettled: What Climate Science Tells Us, What It Doesn't, and Why It MattersCheck out Noah's booksCheck out Noah's course on DataCampConnect with Noah on LinkedInGain access to DataCamp's full course library at a discount!
49:3020/09/2021
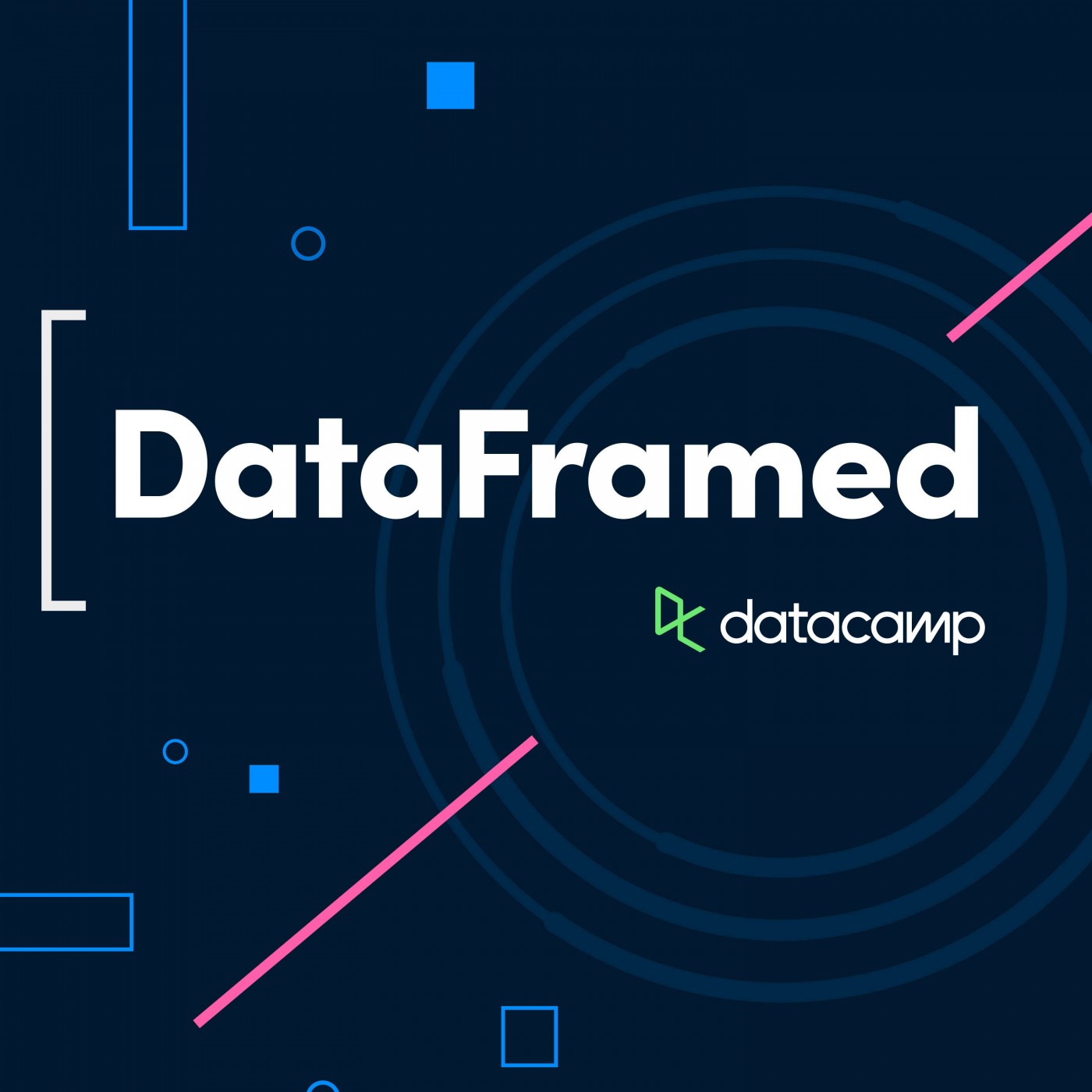
#70 Beyond the Language Wars: R & Python for the Modern Data Scientist
In this episode of DataFramed, we speak with Rick Scavetta and Boyan Angelov about their new book, Python and R for the Modern Data Scientist: The Best of Both Worlds, and how it dawns the start of a new bilingual data science community.
Throughout the episode, Rick and Boyan discuss the history of Python and R, what led them to write the book, how Python and R can be interoperable, the advantages of each language and where to use it, how beginner data scientists should think about learning programming languages, how experienced data scientists can take it to the next level by learning a language they’re not necessarily comfortable with, and more.
Relevant links from the interview:
We’d love your feedback! Let us know which topics you’d like us to cover and what you think of DataFramed by answering this 30-second surveyCheck out Rick and Boyan’s bookCheck out Rick’s courses on DataCampCheck out Boyan's other booksConnect with Rick on LinkedInConnect with Boyan on LinkedIn
55:4706/09/2021
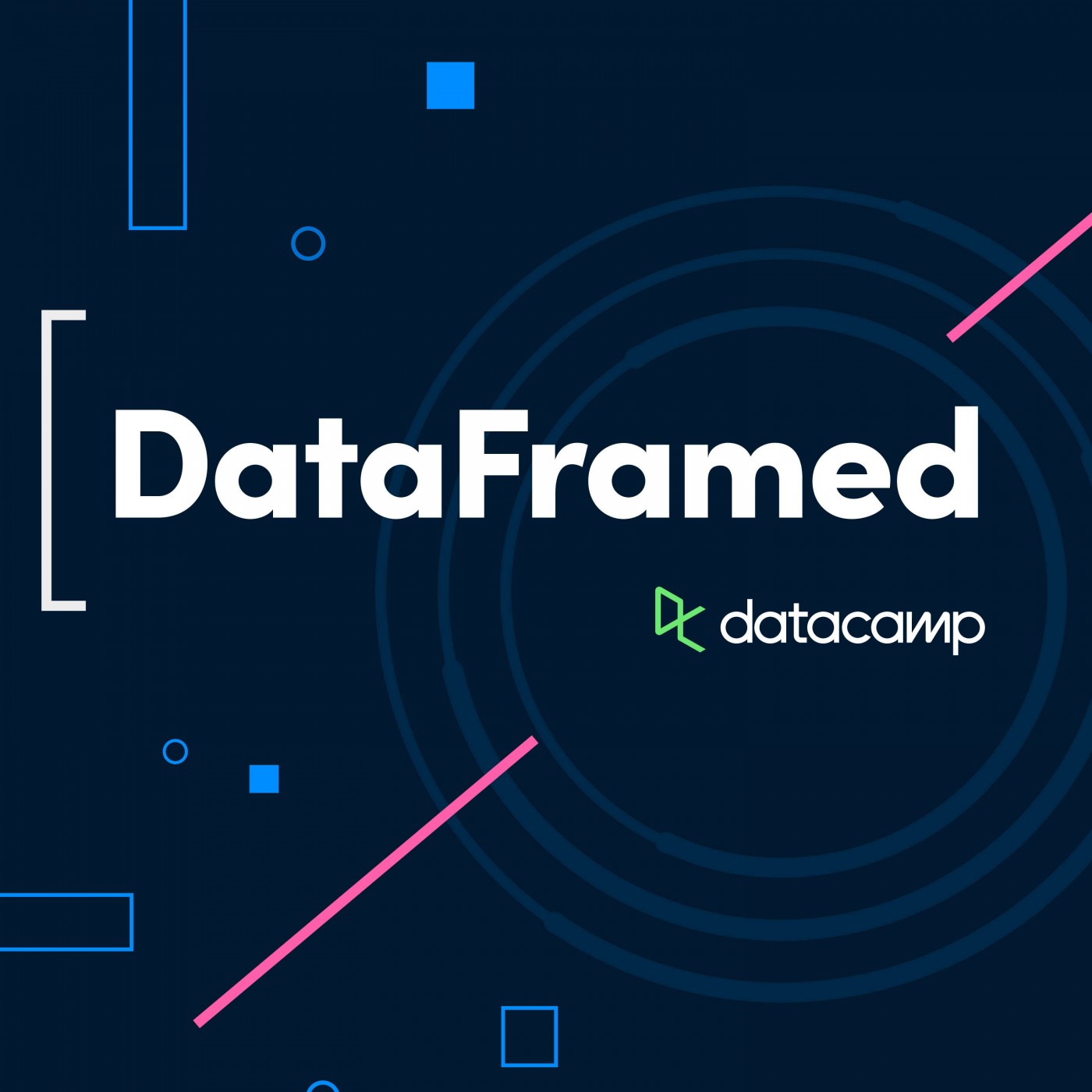
#69 Effective Data Storytelling: How to Turn Insights into Action
In this episode of DataFramed, we speak with Brent Dykes, Senior Director of Insights & Data Storytelling at Blast Analytics and author of Effective Data Storytelling: How to Turn Insights into Action on how data storytelling is shaping the analytics space.
Throughout the episode, Brent talks about his background, what made him write a book on effective data storytelling, how data storytelling is often misinterpreted and misused, the psychology of storytelling and how humans are shaped to resonate with it, the role of empathy when creating data stories, the blueprint of a successful data story, what data scientists can do to become better data storytellers, the future of augmented analytics and data storytelling, and more.
Relevant links from the interview:
Connect with Brent on LinkedInRegister for Brent's Webinar on DataCampCheck out Brent's Book
52:1723/08/2021
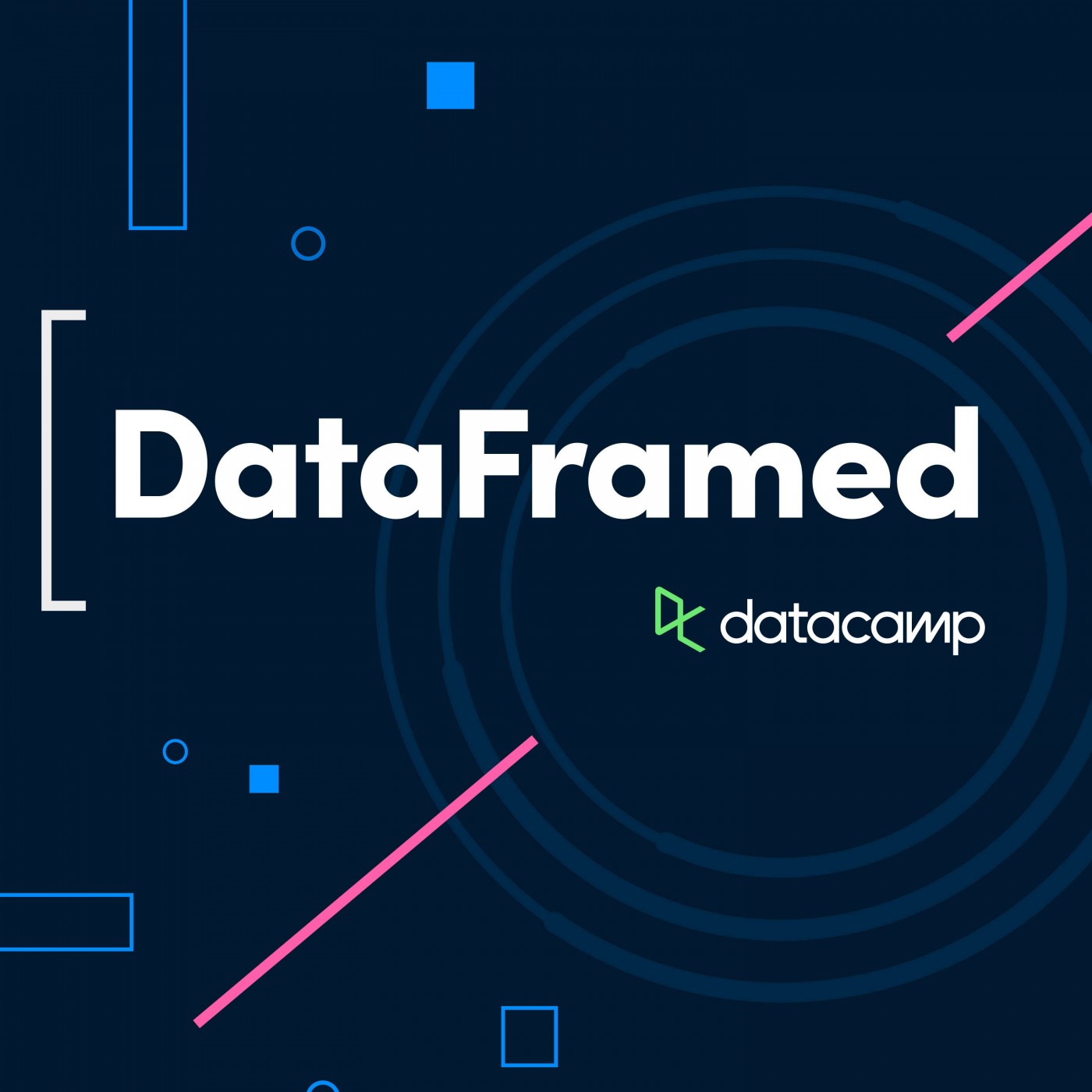
#68 The Future of Responsible AI
In this episode of DataFramed, Adel speaks with Maria Luciana Axente, Responsible AI and AI for Good Lead at PwC UK on the state and future of responsible AI.Throughout the episode, Maria talks about her background, the differences & intersections between "AI ethics" and "Responsible AI", the state of responsible AI adoption within organizations, the link between responsible AI and organizational culture, what data scientists can do today to ensure they're part of their organization's responsible AI journey, and more. Relevant links from the interview:
Connect with Maria on LinkedInKate Crawford's Atlas of AI9 Ethical AI Principles for Organizations to FollowPwC's Responsible AI ToolkitRead our Data Literacy for Responsible AI White Paper
45:0309/08/2021
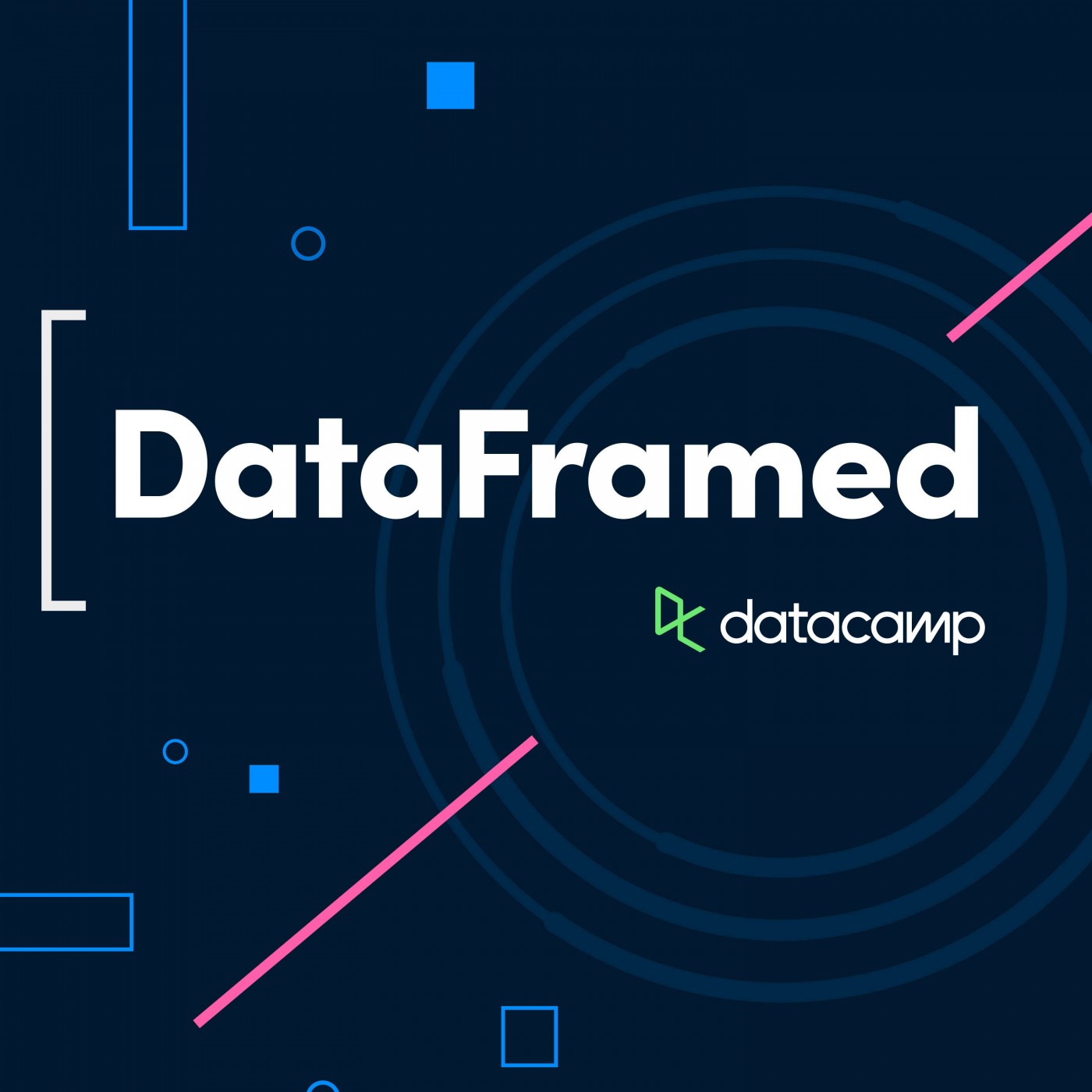
#67 Operationalizing Machine Learning with MLOps
In this episode of DataFramed, Adel speaks with Alessya Visnjic, CEO and co-founder of WhyLabs, an AI Observability company on a mission to build the interface between AI and human operators. Throughout the episode, Alessya talks about the unique challenges data teams face when operationalizing machine learning that spurred the need for MLOps, how MLOps intersects and diverges with different terms such as DataOps, ModelOps, and AIOps, how and when organizations should get started on their MLOps journey, the most important components of a successful MLOps practice, and more.
Relevant links from the interview:
Connect with Alessya on LinkedInAndrew Ng on the important of being data-centricJoe Reis on the data culture and all things datawhylogs: the standard for data logging — please send you feedback, contribute, help us build integrations into your favorite data tools and extend the concept of logging to new data types. Join the effort of building a new open standard for data logging!Try the WhyLabs platform
35:1726/07/2021
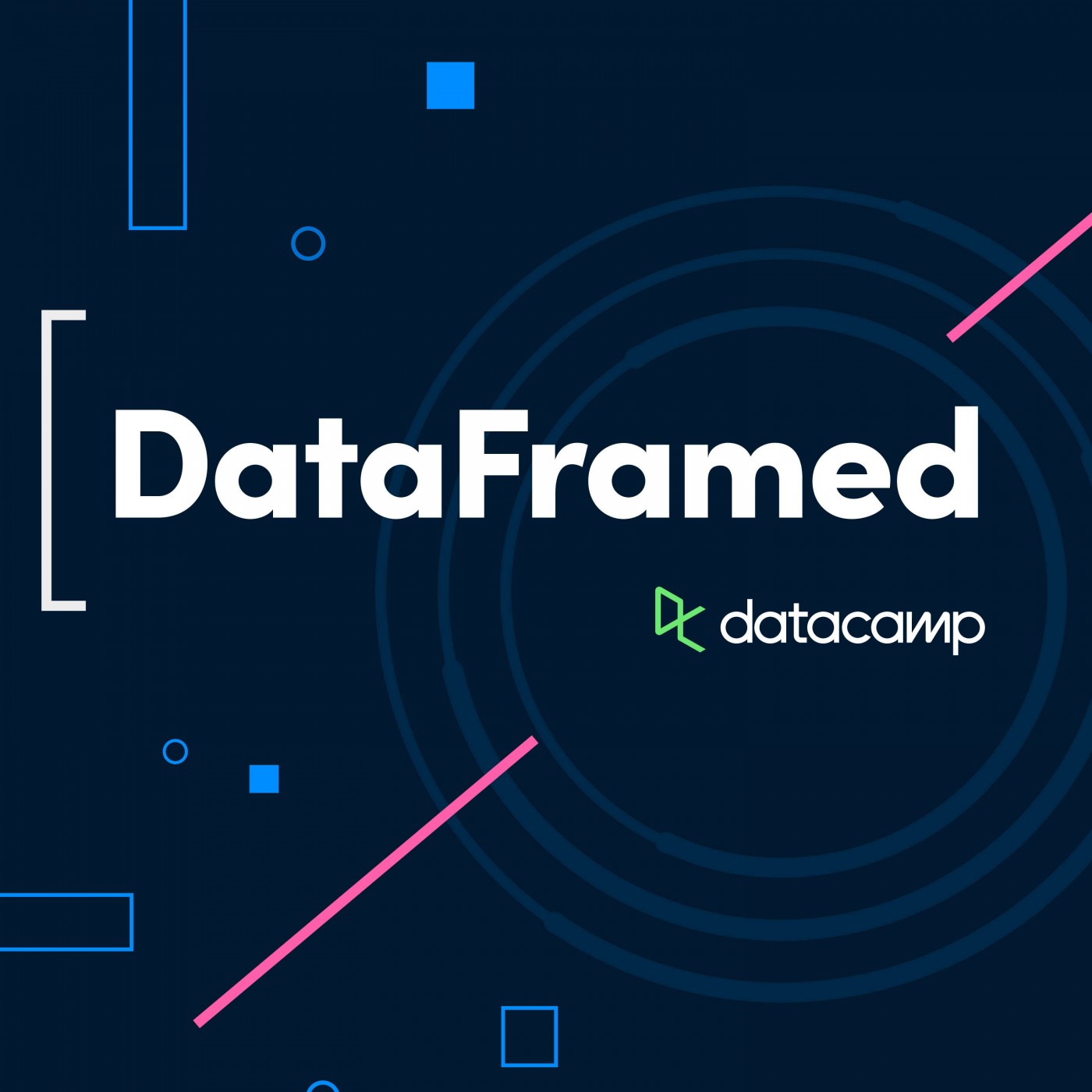
#66 The Path to Building Data Cultures
In this episode of DataFramed, Adel speaks with Sudaman Thoppan Mohanchandralal, Regional Chief Data, and Analytics Officer at Allianz Benelux, on the importance of building data cultures and his experiences operationalizing data culture transformation programs.Throughout the episode, Sudaman talks about his background, the Chief Data Officer’s mandate and how it has evolved over the years, how organizations should prioritize building data cultures, the science behind culture change, the importance of executive data literacy when scaling value from data, and more.
Relevant links from the interview:
Connect with Sudaman on LinkedInCheck out Sudaman’s Webinar on DataCampWhy Data Culture Matters
30:3512/07/2021
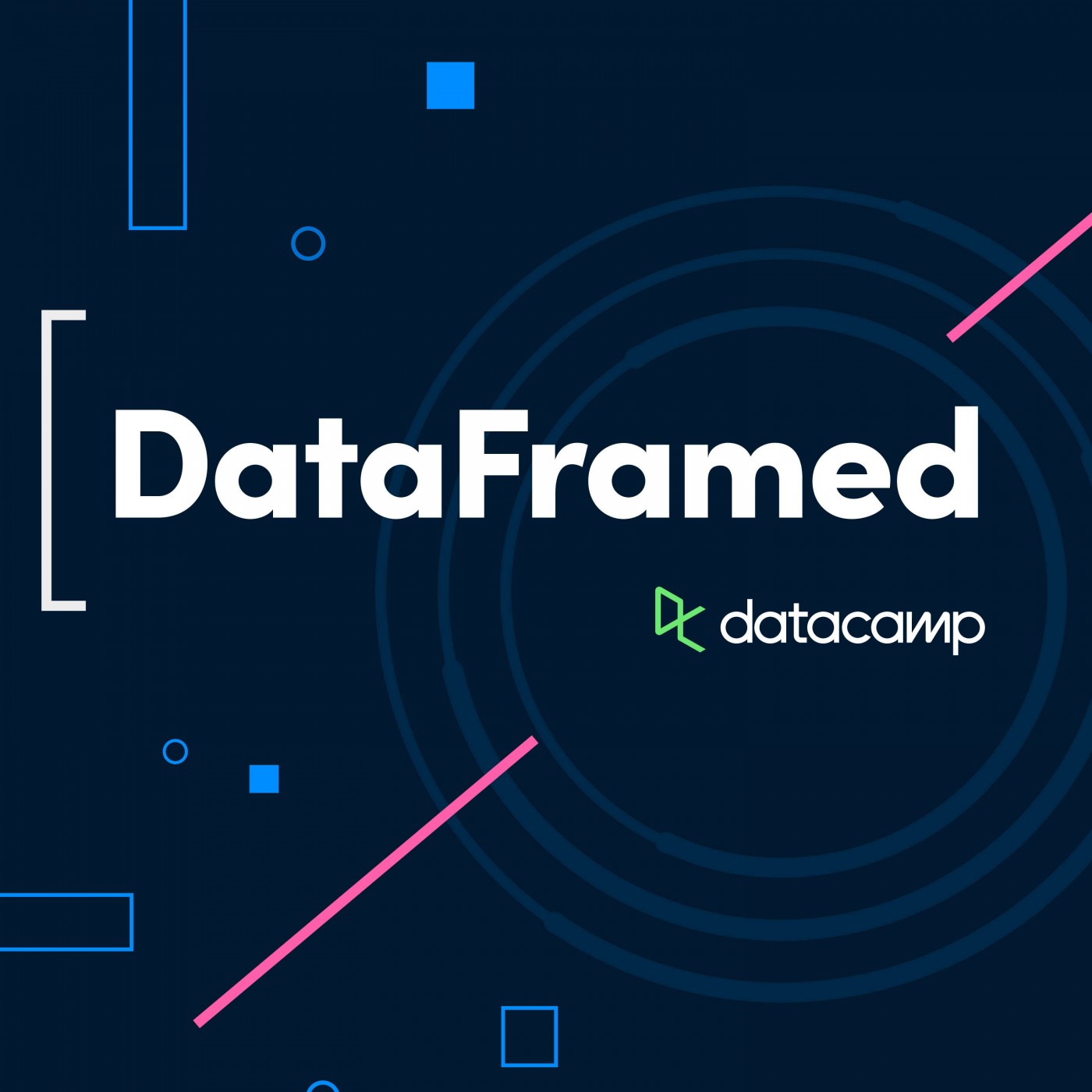
#65 Preventing Fraud in eCommerce with Data Science
In this episode of DataFramed, Adel speaks with Elad Cohen, VP of Data Science and Research at Riskified on how data science is being used to combat fraud in eCommerce.Throughout the episode, Elad talks about his background, the plethora of data science use-cases in eCommerce, how Riskified builds state-of-the-art fraud detection models, common pitfalls data teams face, his best practices gaining organizational buy-in for data projects, how data scientists should focus on value, whether they should have engineering skills, and more.
Relevant links from the interview:
Connect with Elad on LinkedInRegister for our upcoming webinarsHow Riskified chooses what to research
52:1728/06/2021
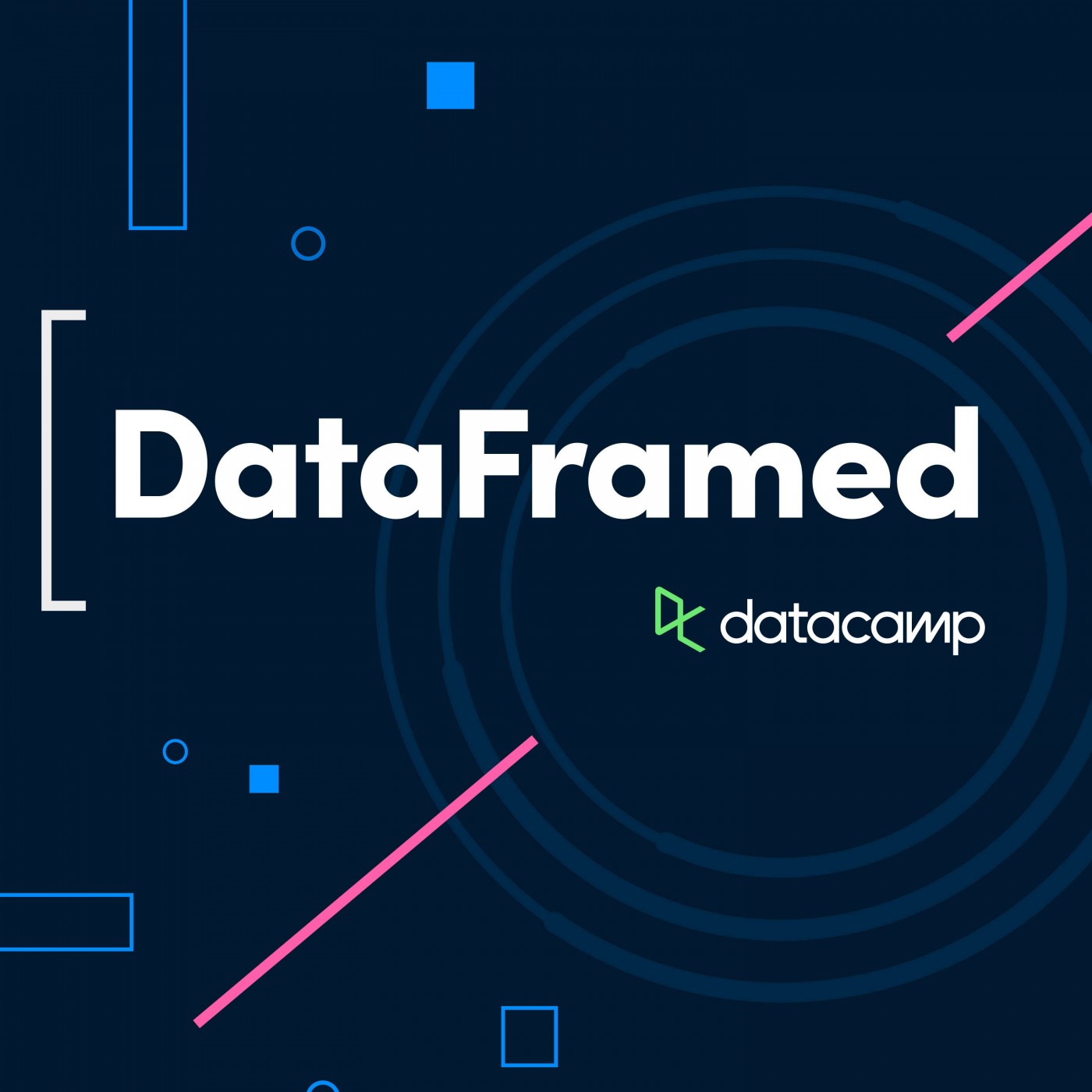
#64 Creating Trust in Data with Data Observabilty
In this episode of DataFramed, Adel speaks with Barr Moses, CEO, and co-founder of Monte Carlo on the importance of data quality and how data observability creates trust in data throughout the organization.
Throughout the episode, Barr talks about her background, the state of data-driven organizations and what it means to be data-driven, the data maturity of organizations, the importance of data quality, what data observability is, and why we’ll hear about it more often in the future. She also covers the state of data infrastructure, data meshes, and more.
Relevant links from the interview:
Connect with Barr on LinkedInLearn more about data meshesCheck out the Monte Carlo blogDataCamp's Guide to Organizational Data Maturity
43:2914/06/2021
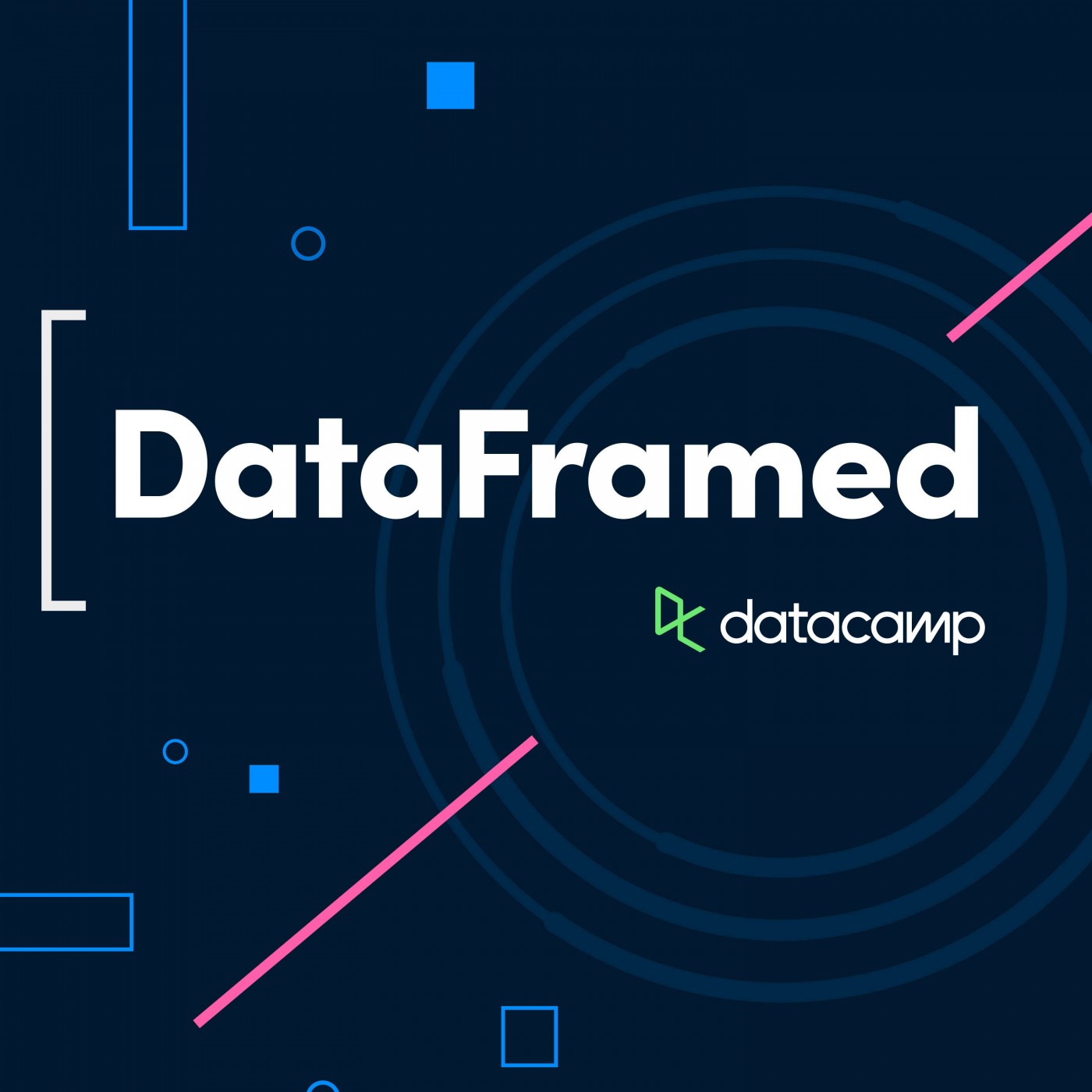
#63 The Past and Present of Data Science
In this episode of DataFramed, Adel speaks with Sergey Fogelson, Vice President of Data Science and Modeling at Viacom on how data science has evolved over the past decade, and the remaining large-scale challenges facing data teams today.
Throughout the episode, Sergey deep-dives into his background, the various projects he’s been involved with throughout his career, the most exciting advances he’s seen in the data science space, the largest challenges facing data teams today, best practices democratizing data, the importance of learning SQL, and more.
Relevant links from the interview:
Connect with Sergey on LinkedInCheck out Sergey’s course on DataCampLearn more about AirflowLearn more about PySparkLearn more about SQL
More resources from DataCamp
Upskill your team with DataCampOur Guide on Open Source Software in Data ScienceYour Organization’s Guide to Data Maturity
01:06:3031/05/2021
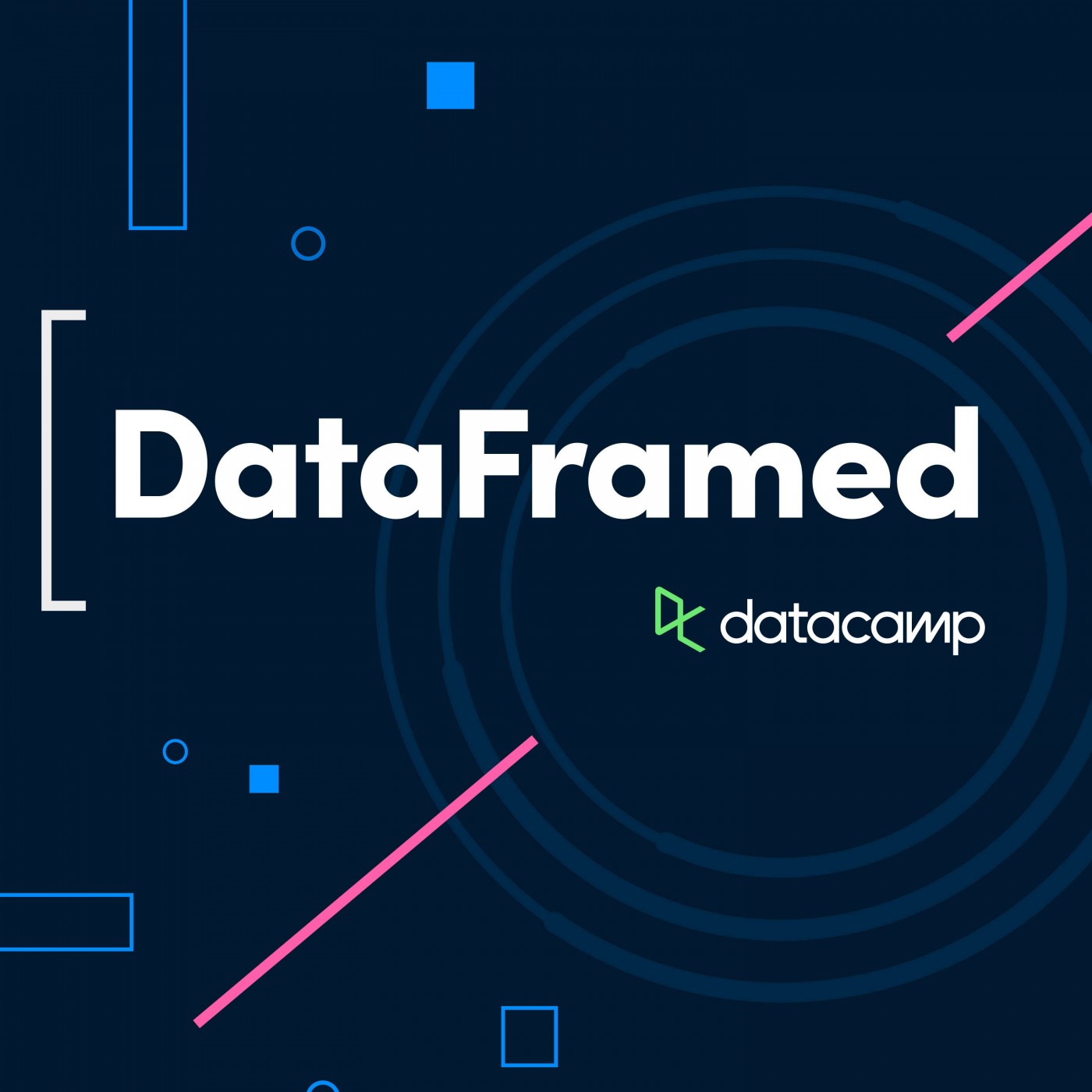
#62 From Predictions to Decisions
In this episode of DataFramed, Adel speaks with Dan Becker, CEO of decision.ai and founder of Kaggle Learn on the intersection of decision sciences and AI, and best practices when aligning machine learning to business value.
Throughout the episode, Dan deep-dives into his background, how he reached the top of a Kaggle competition, the difference between machine learning in a Kaggle competition and the real world, the role of empathy when aligning machine learning to business value, the importance of decisions sciences when maximizing the value of machine learning in production, and more.
Links:
Follow Dan on TwitterFollow Dan on LinkedInWhat 70% of data science learners do wrongCheck out Dan’s course on DataCampdecision.aiDan’s climate dashboard
52:2717/05/2021
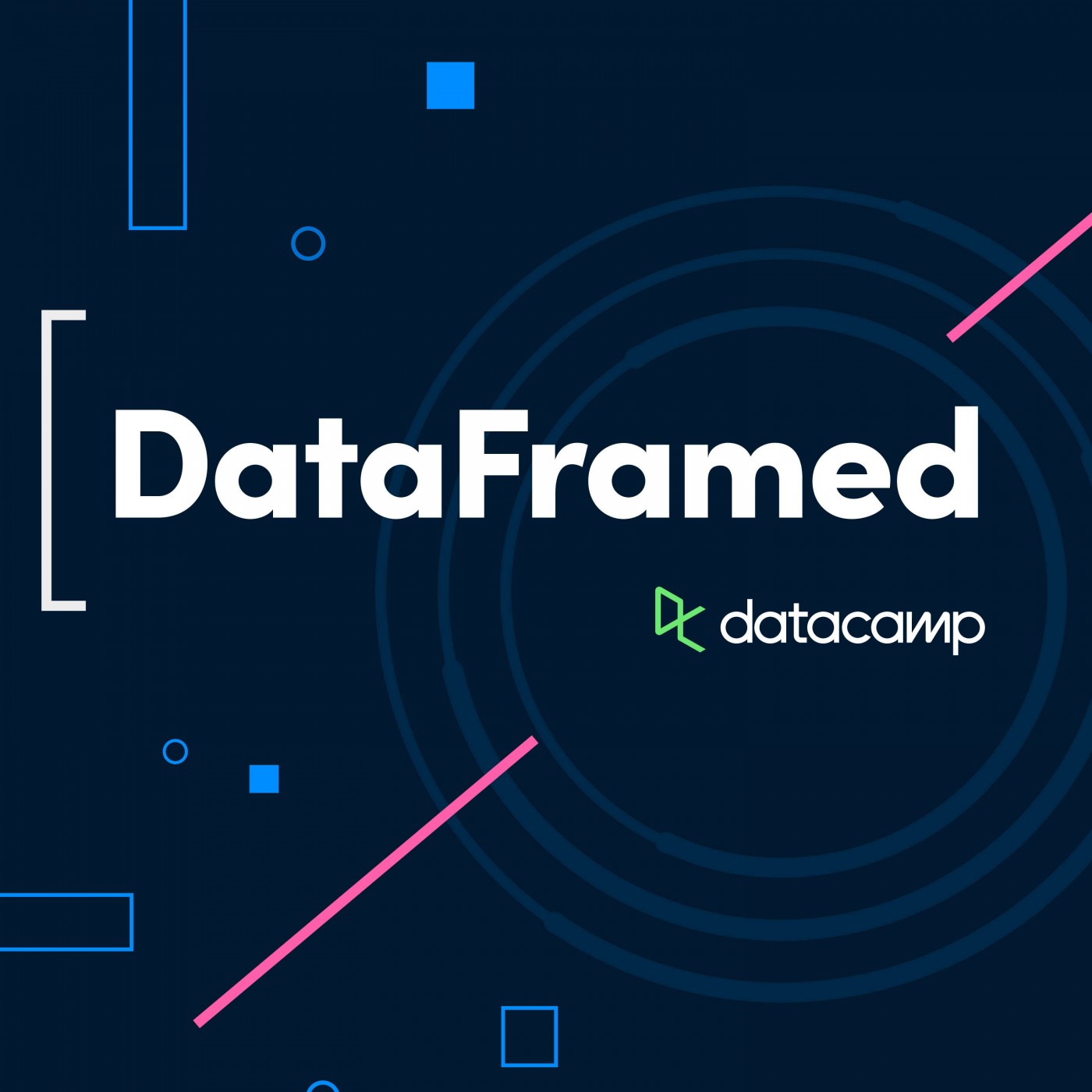
#61 Creating Smart Cities with Data Science
In this episode of DataFramed, Adel speaks with Amen Ra Mashariki, principal scientist at Nvidia and the former Chief Analytics Officer of the City of New York on how data science is done in government agencies, and how it's driving smarter cities all around us.
Throughout the episode, Amen deep-dives into the use-cases he worked on to make the city of New York smarter, how data science allows cities to become more reactive and proactive, the unique challenges of scaling data science in a government setting, the friction between providing value and data privacy and ethics, the state of data literacy in government, and more.
Links from the interview:
Follow Amen on LinkedInFollow Amen on TwitterThe New York City Business AtlasHurricane Sandy FEMA After-Action ReportData Drills
44:1703/05/2021
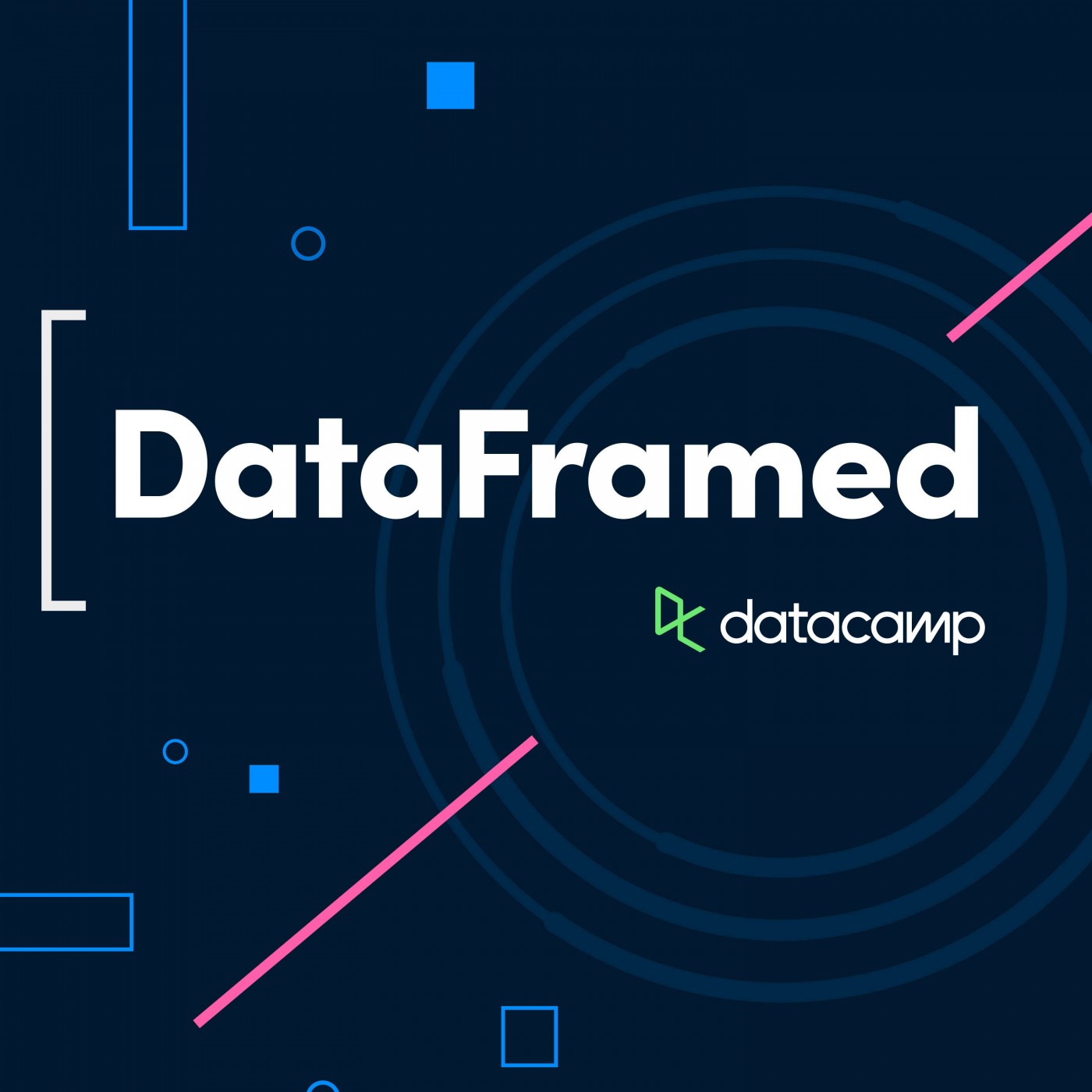
New DataFramed Episodes
We are super excited to be relaunching the DataFramed podcast. In this iteration of DataFramed, Adel Nehme, a data science educator at DataCamp, will uncover the latest thinking on all things data and how it’s impacting organizations through biweekly (once every two weeks) interviews and conversations with data experts from across the world.
Check out this snippet for a preview of what’s to come and for a short chat with DataCamp’s CEO Jonathan Cornelissen on where he thinks data science is headed and the major challenges facing data teams today.
Links:
For the rest of April, get free access to DataCamp.Get involved with DataCamp Donates
15:1326/04/2021
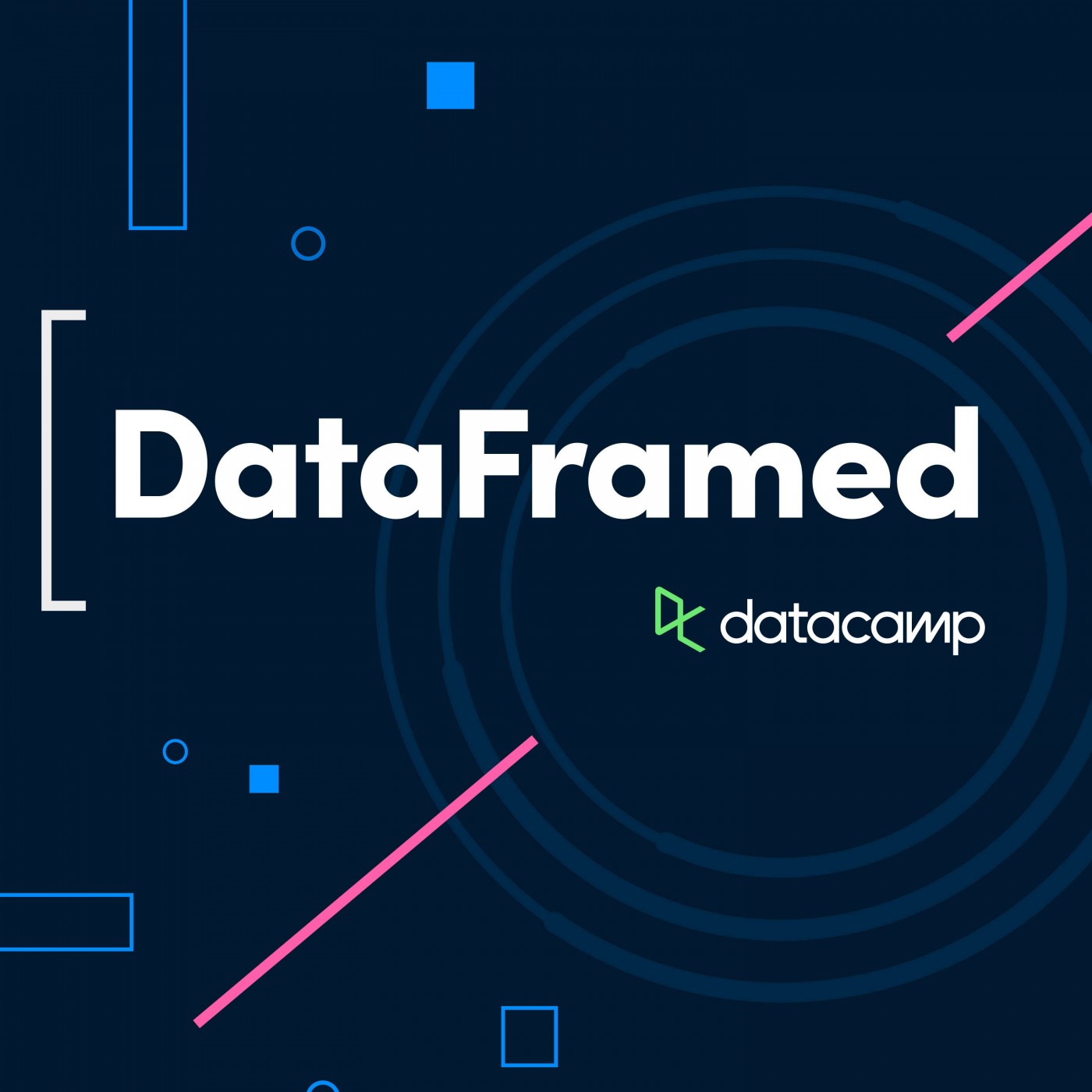
#60 Data Privacy in the Age of COVID-19
Before the COVID-19 crisis, we were already acutely aware of the need for a broader conversation around data privacy: look no further than the Snowden revelations, Cambridge Analytica, the New York Times Privacy Project, the General Data Protection Regulation (GDPR) in Europe, and the California Consumer Privacy Act (CCPA). In the age of COVID-19, these issues are far more acute. We also know that governments and businesses exploit crises to consolidate and rearrange power, claiming that citizens need to give up privacy for the sake of security. But is this tradeoff a false dichotomy? And what type of tools are being developed to help us through this crisis? In this episode, Katharine Jarmul, Head of Product at Cape Privacy, a company building systems to leverage secure, privacy-preserving machine learning and collaborative data science, will discuss all this and more, in conversation with Dr. Hugo Bowne-Anderson, data scientist and educator at DataCamp.Links from the show
FROM THE INTERVIEW
Katharine on TwitterKatharine on LinkedInContact Tracing in the Real World (By Ross Anderson)The Price of the Coronavirus Pandemic (By Nick Paumgarten)Do We Need to Give Up Privacy to Fight the Coronavirus? (By Julia Angwin)Introducing the Principles of Equitable Disaster Response (By Greg Bloom)Cybersecurity During COVID-19 ( By Bruce Schneier)
01:15:3015/05/2020
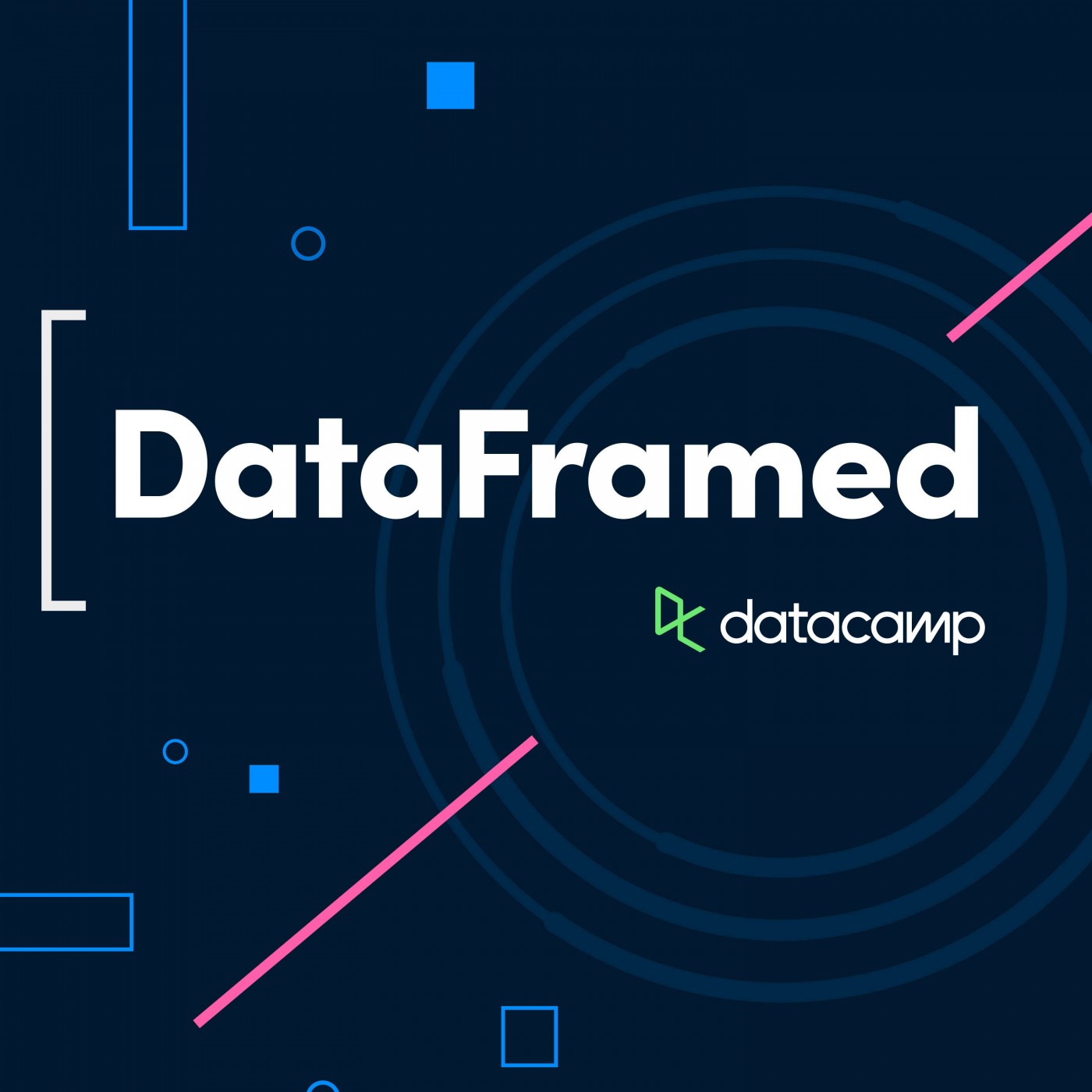
#59 Data Science R&D at TD Ameritrade
This week, Hugo speaks with Sean Law about data science research and development at TD Ameritrade. Sean’s work on the Exploration team uses cutting edge theories and tools to build proofs of concept. At TD Ameritrade they think about a wide array of questions from conversational agents that can help customers quickly get to information that they need and going beyond chatbots. They use modern time series analysis and more advanced techniques like recurrent neural networks to predict the next time a customer might call and what they might be calling about, as well as helping investors leverage alternative data sets and make more informed decisions.
What does this proof of concept work on the edge of data science look like at TD Ameritrade and how does it differ from building prototypes and products? And How does exploration differ from production? Stick around to find out.
LINKS FROM THE SHOW
DATAFRAMED GUEST SUGGESTIONS
DataFramed Guest Suggestions (who do you want to hear on DataFramed?)
FROM THE INTERVIEW
Sean on TwitterSean's WebsiteTD Ameritrade Careers PagePyData Ann Arbor MeetupPyData Ann Arbor YouTube Channel (Videos)TDA Github Account (Time Series Pattern Matching repo to be open sourced in the coming months)Aura Shows Human Fingerprint on Global Air Quality
FROM THE SEGMENTS
Guidelines for A/B Testing (with Emily Robinson ~19:20)
Guidelines for A/B Testing (By Emily Robinson)10 Guidelines for A/B Testing Slides (By Emily Robinson)
Data Science Best Practices (with Ben Skrainka ~34:50)
Debugging (By David J. Agans)Basic Debugging With GDB (By Ben Skrainka)Sneaky Bugs and How to Find Them (with git bisect) (By Wiktor Czajkowski)Good logging practice in Python (By Victor Lin)
Original music and sounds by The Sticks.
51:1701/04/2019
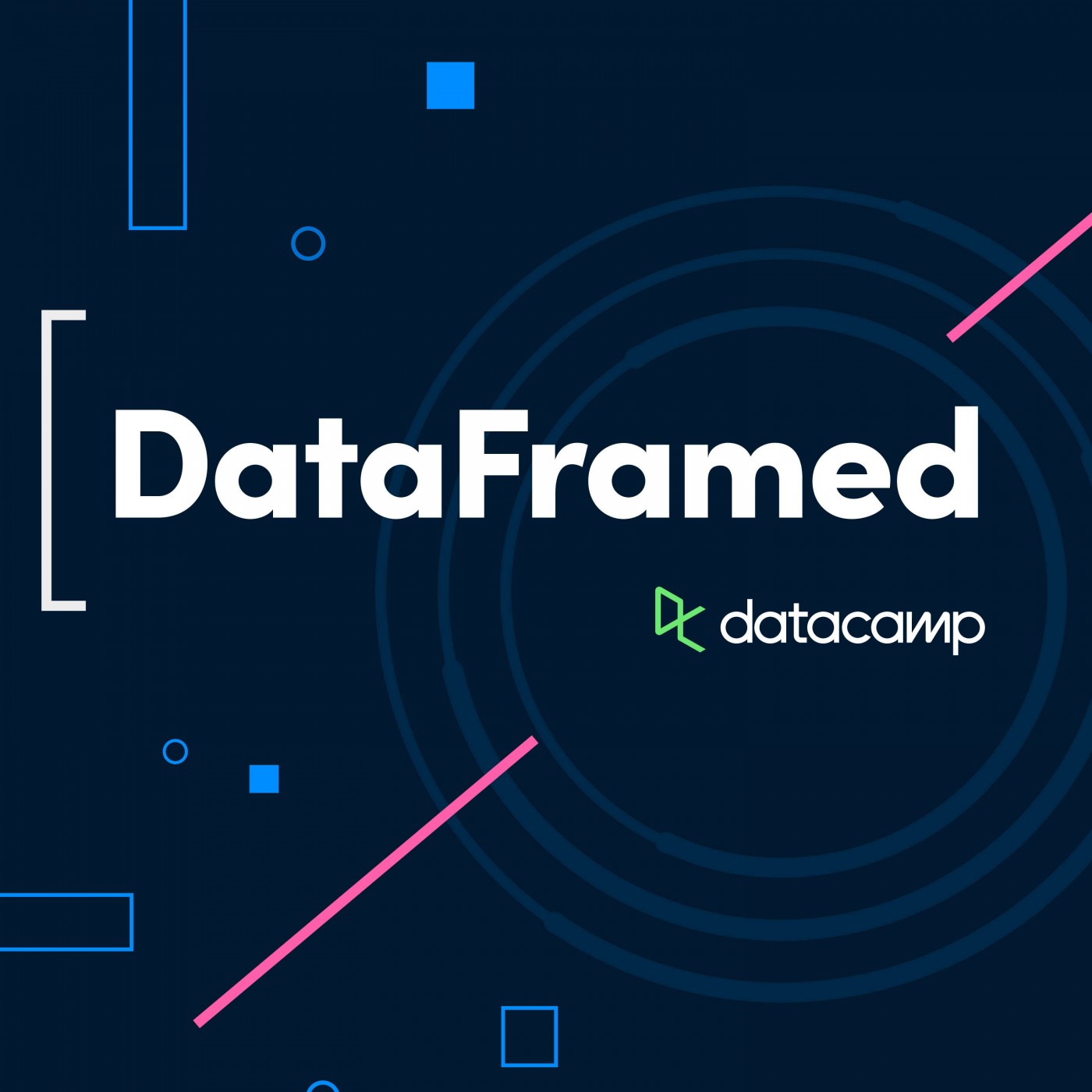
#58 Critical Thinking in Data Science
This week, Hugo speaks with Debbie Berebichez about the importance of critical thinking in data science. Debbie is a physicist, TV host and data scientist and is currently the Chief Data Scientist at Metis in NY.In a world and a professional space plagued by buzz terms like AI, big data, deep learning, and neural networks, conversations around skill sets and less than productive programming language wars, what has happened to critical thinking in data science and data thinking in general? What type of critical thinking skills are even necessary as data science, AI and machine learning become even more present in all of our lives and how spread out do they need to be across organizations and society? Listen to find out!LINKS FROM THE SHOW
DATAFRAMED GUEST SUGGESTIONS
DataFramed Guest Suggestions (who do you want to hear on DataFramed?)
FROM THE INTERVIEW
Debbie on TwitterDebbie's WebsiteDebbie Berebichez- Media Reel (Video)Deborah Berebichez' Keynote at Grace Hopper Celebration 2017 (Video)Debbie Berebichez on Perseverance and Paying it Forward (Video)Things about the Future and the Future of Things (By Debbie Berebichez, Video)
FROM THE SEGMENTS
Data Science tools for getting stuff done and giving it to the world (with Jared Lander ~21:55)
Lander Analytics WebsiteDocker Websiteplumber Website
Statistical Distributions and their Stories (with Justin Bois ~39:30)
Probability distributions and their stories (By Justin Bois)The History of Statistics (By Stephen M. Stigler)The Evolution of the Normal Distribution (By Saul Stahl)
Original music and sounds by The Sticks.
58:3525/03/2019
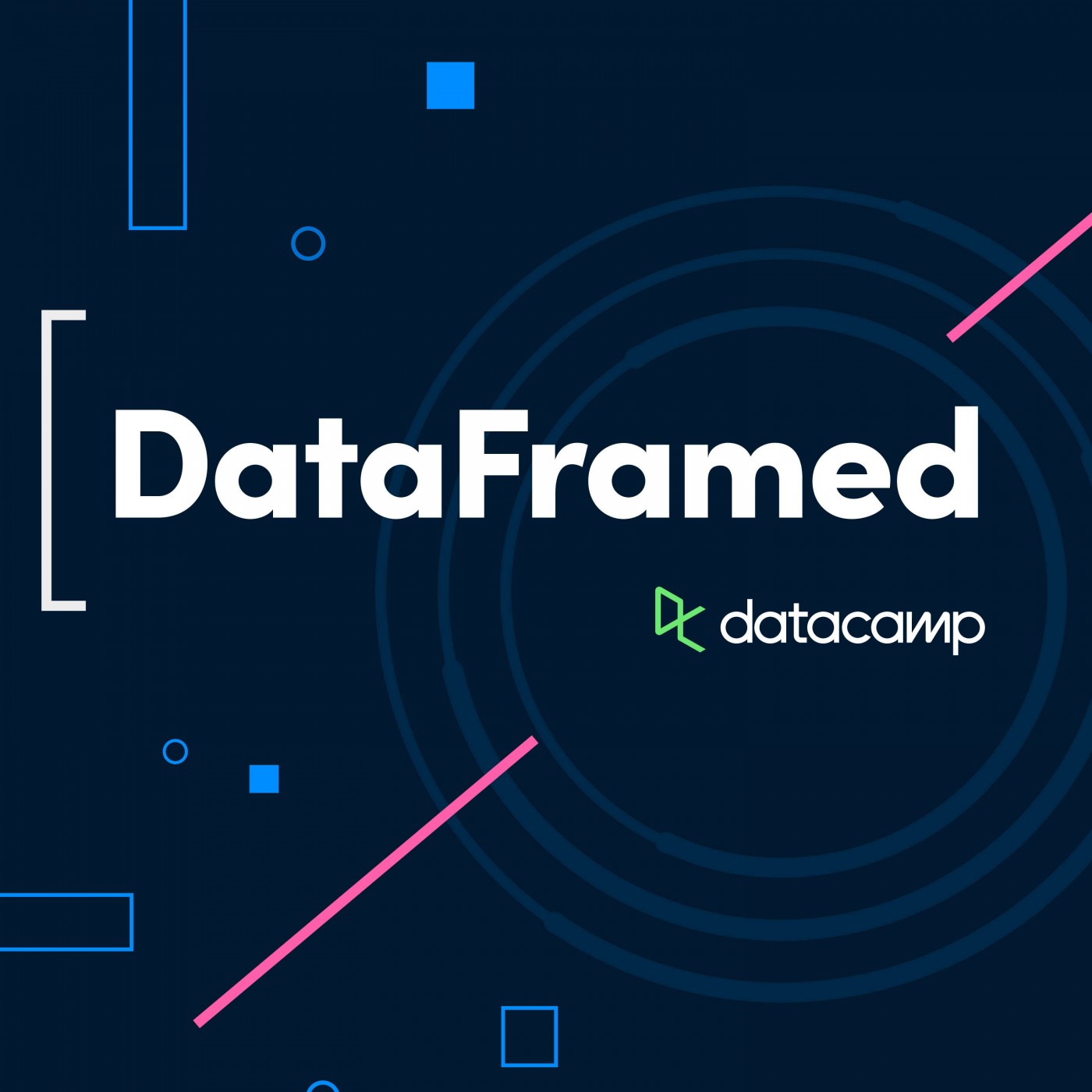
#57 The Credibility Crisis in Data Science
This week, Hugo will be speaking with Skipper Seabold about the current and looming credibility crisis in data science. Skipper is Director of Data Science at Civis Analytics, a data science technology and solutions company, and also the creator of the statsmodels package for statistical modeling and computing in python. Skipper is also a data scientist with a beard bigger than Hugo's.
They’re going to be talking about how data science is facing a credibility crisis that is manifesting itself in different ways in different industries, how and why expectations aren’t met and many stakeholders are disillusioned. You’ll see that if the crisis isn’t prevented, the data science labor market may cease to be a seller’s market and we’ll have big missed opportunities. But this isn’t an episode of Black Mirror so they’ll also discuss how to avoid the crisis, taking detours through the role of randomized control trials in data science, the rise of methods borrowed from econometrics and how to set realistic expectations around what data science can and can’t do.LINKS FROM THE SHOW
DATAFRAMED GUEST SUGGESTIONS
DataFramed Guest Suggestions (who do you want to hear on DataFramed?)
FROM THE INTERVIEW
Skipper on TwitterSkipper on GithubWhat's the Science in Data Science? (Video by Skipper Seabold)The Credibility Revolution in Empirical Economics: How Better Research Design Is Taking the Con out of Econometrics (By Joshua D. Angrist & Jörn-Steffen Pischke, American Economic Association)Project Management for the Unofficial Project Manager: A FranklinCovey Title (By Kory Kogon)Courtyard by Marriott Designing a Hotel Facility with Consumer-Based Marketing Models (Jerry Wind et al., The Institute of Management Sciences)Statsmodels's Documentation
FROM THE SEGMENTS
Guidelines for A/B Testing (with Emily Robinson ~15:48 & ~35:20)
Guidelines for A/B Testing (By Emily Robinson)10 Guidelines for A/B Testing Slides (By Emily Robinson)
Original music and sounds by The Sticks.
55:0318/03/2019
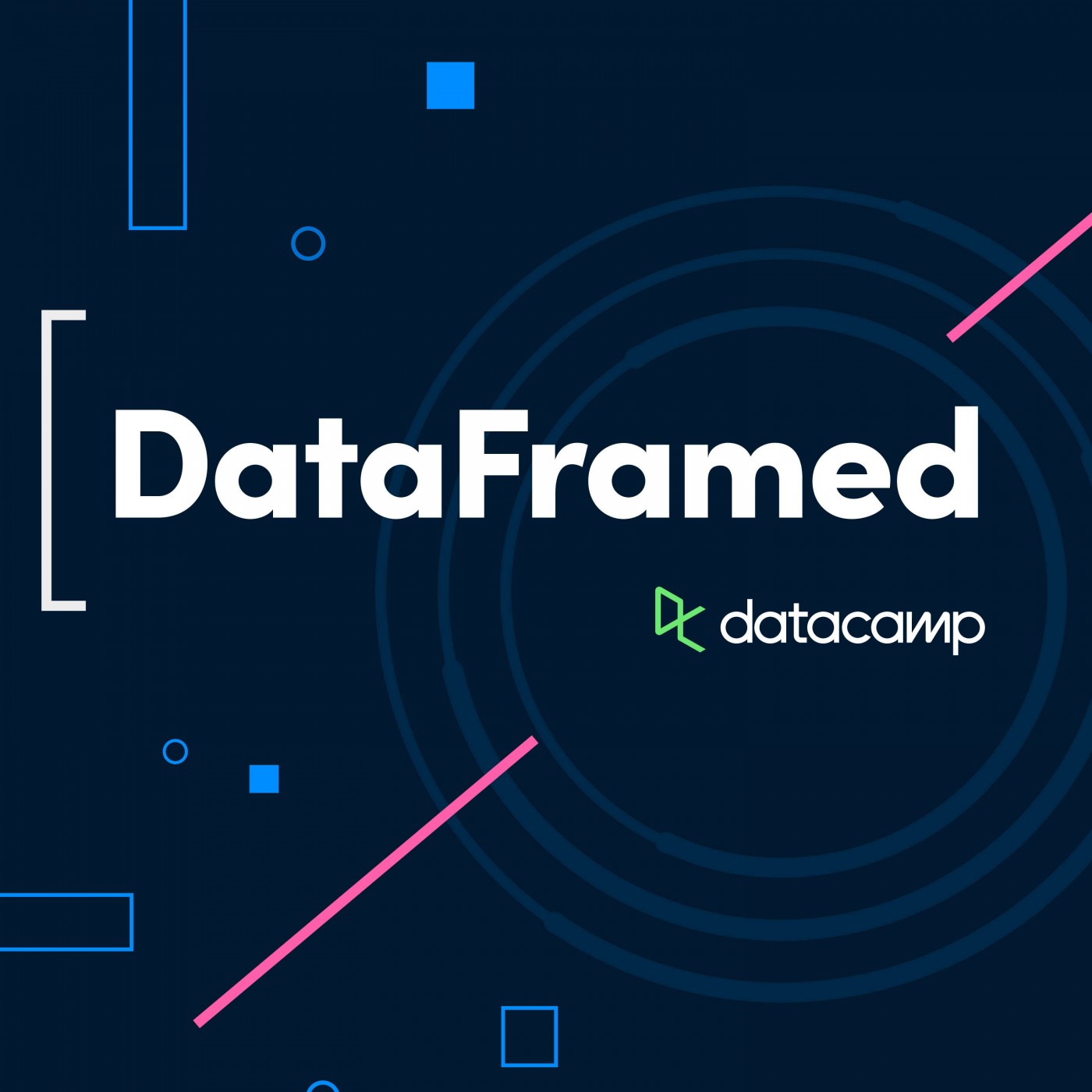
#56 Data Science at AT&T Labs Research
This week, Hugo speaks with Noemi Derzsy, a Senior Inventive Scientist at AT&T Labs within the Data Science and AI Research organization, where she does lots of science with lots of data.
They’ll be talking about her work at AT&T Labs Research, the mission of which is to look beyond today’s technology solutions to invent disruptive technologies that meet future needs. AT&T Labs works on a multitude of projects, from product development at AT&T, to how to combat bias and fairness issues in targeted advertising and creating drones for cell tower inspection research that leverages AI, ML and video analytics. They’ll be talking about some of the work Noemi does, from characterizing human mobility from cellular network data to characterizing their mobile network to analyze how its topology compares to other real social networks reported to understanding tv viewership, and how engaged people are in different shows. They’ll discuss what the future of data science looks like, whether it will even be around in 2029 and what types of skills would help you land a job in a place like AT&T Labs.LINKS FROM THE SHOW
DATAFRAMED GUEST SUGGESTIONS
DataFramed Guest Suggestions (who do you want to hear on DataFramed?)
FROM THE INTERVIEW
Noemi on TwitterNoemi's WebsiteHuman Mobility Characterization from Cellular Network Data (By Richard Becker et al., Communications of the ACM)AT&T Labs Research WebsiteNASA Datanauts WebsiteOpen NASA Website
FROM THE SEGMENTS
Guidelines for A/B Testing (with Emily Robinson ~18:23 & ~36:38)
Testing multiple statistical hypotheses resulted in spurious associations: a study of astrological signs and health (By Peter C. Austin et al., Journal of Clinical Epidemiology)From Infrastructure to Culture: A/B Testing Challenges in Large Scale Social Networks (By Ya Xu et al., LinkedIn Corp)Guidelines for A/B Testing (By Emily Robinson)10 Guidelines for A/B Testing Slides (By Emily Robinson)
Original music and sounds by The Sticks.
56:5111/03/2019
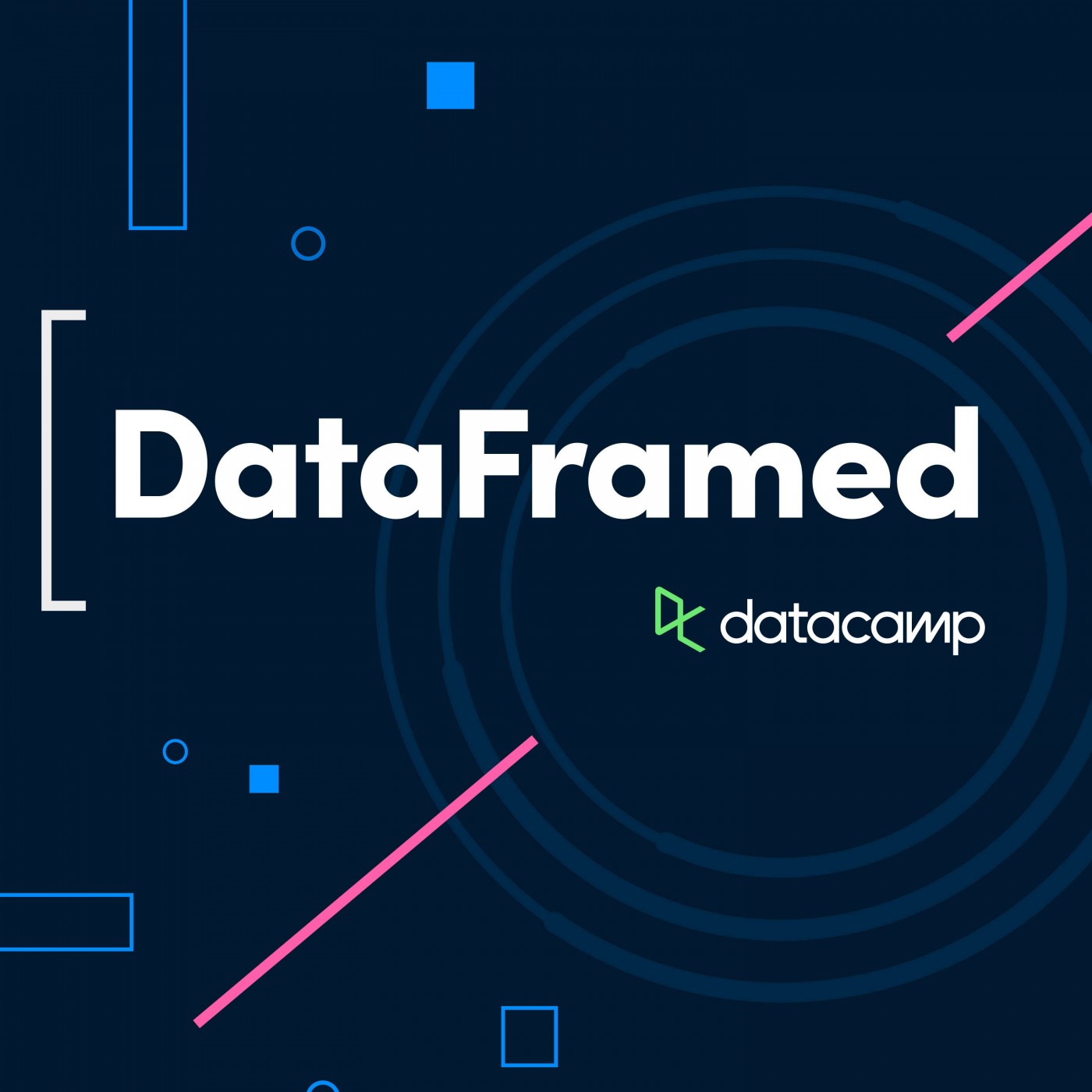
#55 Getting Your First Data Science Job
This week, Hugo speaks with Chris Albon about getting your first data science job. Chris is a Data Scientist at Devoted Health, where he uses data science and machine learning to help fix America's healthcare system. Chris is also doing a lot of hiring at Devoted and that’s why he’s so excited today to talk about how to get your first data science job. You may know Chris as co-host of the podcast Partially Derivative, from his educational resources such as his blog and machine learning flashcards or as one of the funniest data scientists on Twitter.LINKS FROM THE SHOW
DATAFRAMED GUEST SUGGESTIONS
DataFramed Guest Suggestions (who do you want to hear on DataFramed?)
FROM THE INTERVIEW
Chris on TwitterChris's WebsiteDevoted WebsiteMachine Learning Flashcards (By Chris Albon)Machine Learning with Python Cookbook (By Chris Albon)
FROM THE SEGMENTS
Guidelines for A/B Testing (with Emily Robinson ~26:50)
Guidelines for A/B Testing (By Emily Robinson)10 Guidelines for A/B Testing Slides (By Emily Robinson)
Original music and sounds by The Sticks.
01:09:2004/03/2019
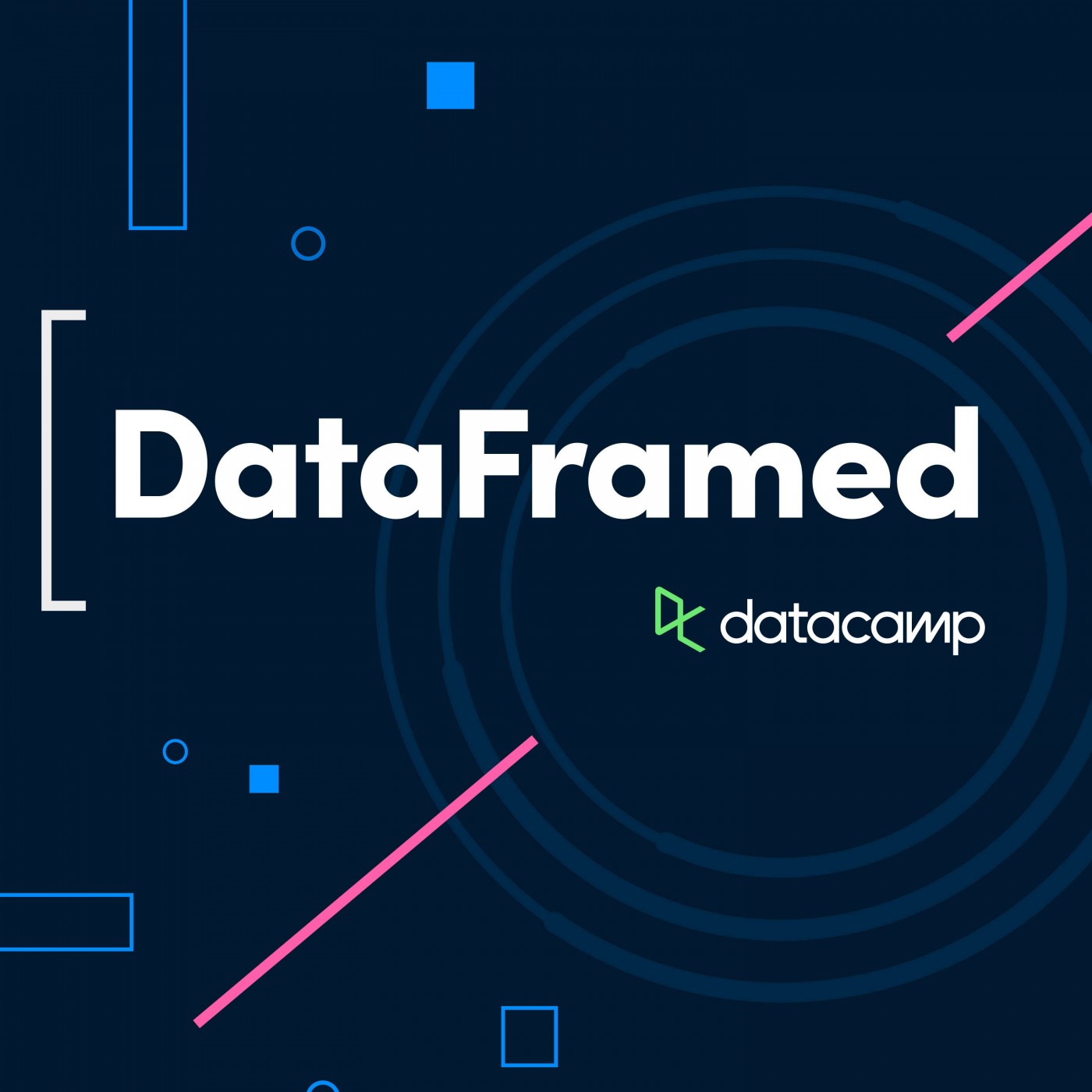
#54 Women in Data Science
This week, Hugo speaks with Reshama Shaikh, about women in machine learning and data science, inclusivity and diversity more generally and how being intentional in what you do is essential. Reshama, a freelance data scientist and statistician, is also an organizer of the meetup groups Women in Machine Learning & Data Science (otherwise known as WiMLDS) and PyLadies. She has organized WiMLDS for 4 years and is a Board Member. They’ll discuss her work at WiMLDS and what you can do to support and promote women and gender minorities in data science. They’ll also delve into why women are flourishing in the R community but lagging in Python and discuss more generally how NUMFOCUS thinks about diversity and inclusion, including their code of conduct. All this and more.LINKS FROM THE SHOW
DATAFRAMED GUEST SUGGESTIONS
DataFramed Guest Suggestions (who do you want to hear on DataFramed?)
FROM THE INTERVIEW
Reshama’s BlogReshama on TwitterList of Relevant Conferences (and Code of Conduct info)NYC PyLadies meetupCode of Conduct for NeurIPS and Other Stem OrganizationsNumFOCUS Diversity & Inclusion in Scientific Computing (DISC)NumFOCUS DISCOVER Cookbook (for inclusive events)fastai deep learning notes
WiMLDS (Women in Machine Learning and Data Science)
NYC WiMLDS meetupTo start a WiMLDS chapter: email [email protected] and more info at our starter kit.WiMLDS WebsiteGlobal List of WiMLDS Meetup ChaptersWiMLDS Paris: They run their meetups in English, so knowledge of French is not required.
FROM THE SEGMENTS
DataCamp User Stories (with David Sudolsky ~17:27 & ~31:50)
Boldr Website
Original music and sounds by The Sticks.
47:1825/02/2019
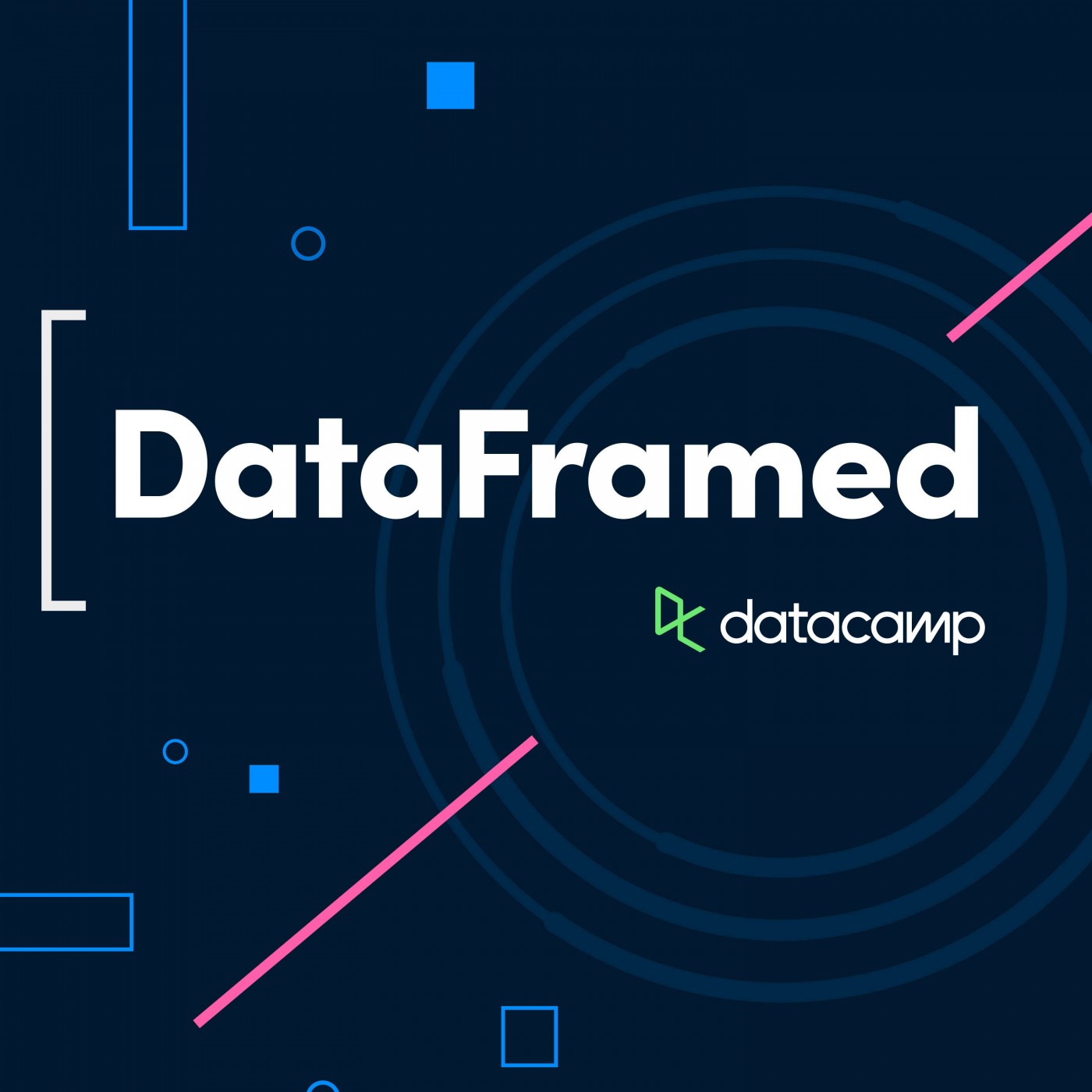
#53 Data Science, Gambling and Bookmaking
This week, Hugo speaks with Marco Blume, Trading Director at Pinnacle Sports. Marco and Hugo will talk about the role of data science in large-scale bets and bookmaking, how Marco is training an army of data scientists and much more. At Pinnacle, Marco uses tight risk-management built on cutting-edge models to provide bets not only on sports but on questions such as who will be the next pope? Who will be the world hot dog eating champion, who will land on mars first and who will be on the iron throne at the end of game of thrones. They’ll discuss the relations between risk management and uncertainty, how great forecasters are necessarily good at updating their predictions in the light of new data and evidence, how you can model this using Bayesian inference and the future of biometric sensing in sports betting. And, as always, much, much more.LINKS FROM THE SHOW
DATAFRAMED GUEST SUGGESTIONS
DataFramed Guest Suggestions (who do you want to hear on DataFramed?)
FROM THE INTERVIEW
Pinnacle WebsiteTraining an army of new data scientists (Presentation by Marco Blume)
FROM THE SEGMENTS
Data Science Best Practices (with Ben Skrainka ~16:40)
Python Debugging With Pdb (By Nathan Jennings)pdb Tutorial (Github)The Visual Python Debugger for Jupyter Notebooks You’ve Always Wanted (By David Taieb)Debugging with RStudio (By Jonathan McPherson)Basics of Debugging
Statistical Distributions and their Stories (with Justin Bois at ~36:00)
Justin's Website at CaltechProbability distributions and their stories (By Justin Bois)
Original music and sounds by The Sticks.
54:0518/02/2019
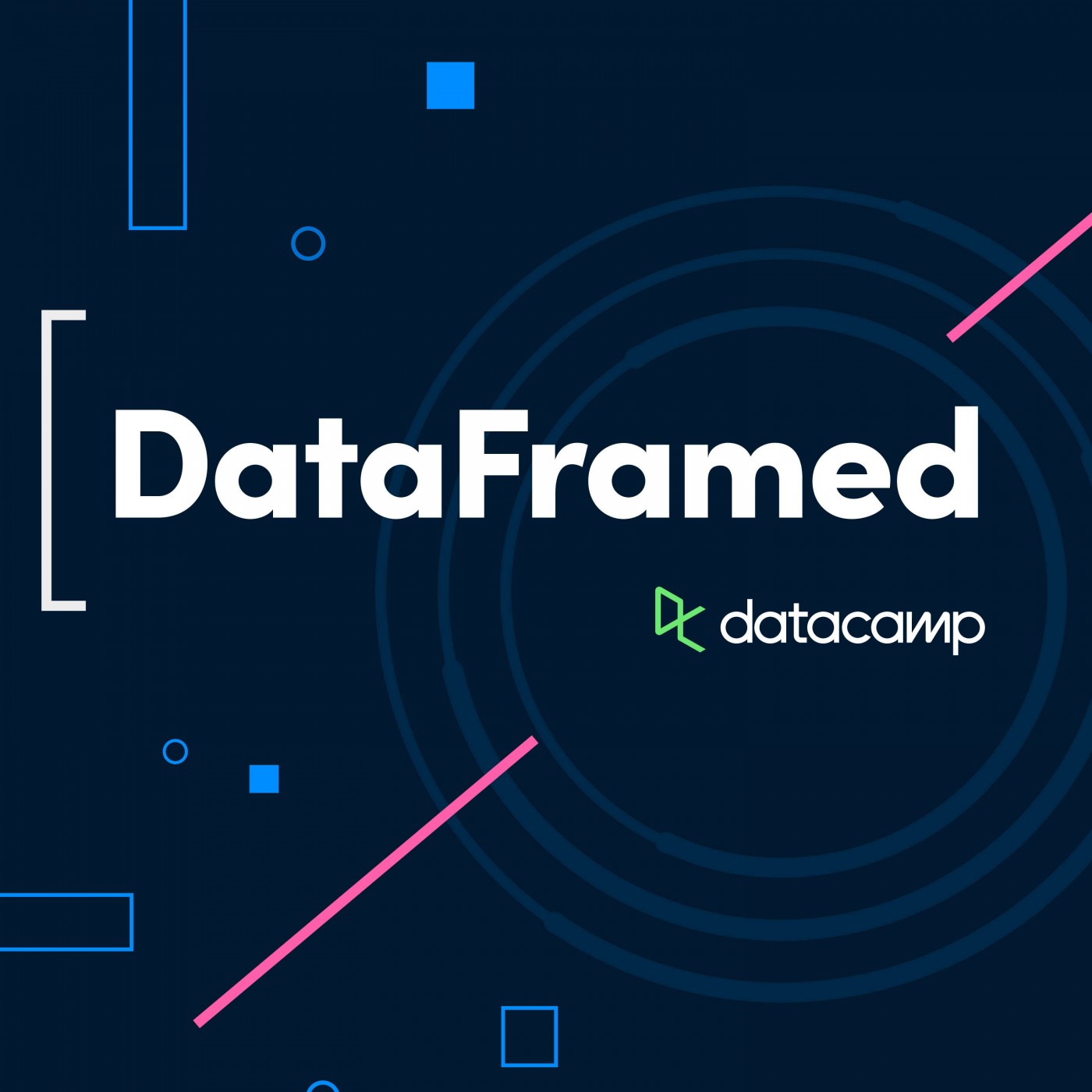
#52 Data Science at the BBC
This week on DataFramed, the DataCamp podcast, Hugo speaks with Gabriel Straub, the Head of Data Science and Architecture at the BBC, where his role is to help make the organization more data informed and to make it easier for product teams to build data and machine learning powered products. They’ll be talking about data science and machine learning at the BBC and how they can impact content discoverability, understanding content, putting the right stuff in front of people, how Gabriel and his team develop broader data science & machine learning architecture to make sure best practices are adopted and what it means to apply machine learning in a sensible way. How does the BBC think about incorporating data science into its business, which has been around since 1922 and historically been at the forefront of technological innovation such as in radio and television? Listen to find out!LINKS FROM THE SHOW
DATAFRAMED GUEST SUGGESTIONS
DataFramed Guest Suggestions (who do you want to hear on DataFramed?)
FROM THE INTERVIEW
Gabriel Straub: It's bigger on the inside (Video)BBC datalab
FROM THE SEGMENTS
DataCamp User Stories (with Krittika Patil ~16:10 & ~38:12)
Kespry (Drone Aerial Intelligence for Industry)
Original music and sounds by The Sticks.
01:01:3811/02/2019
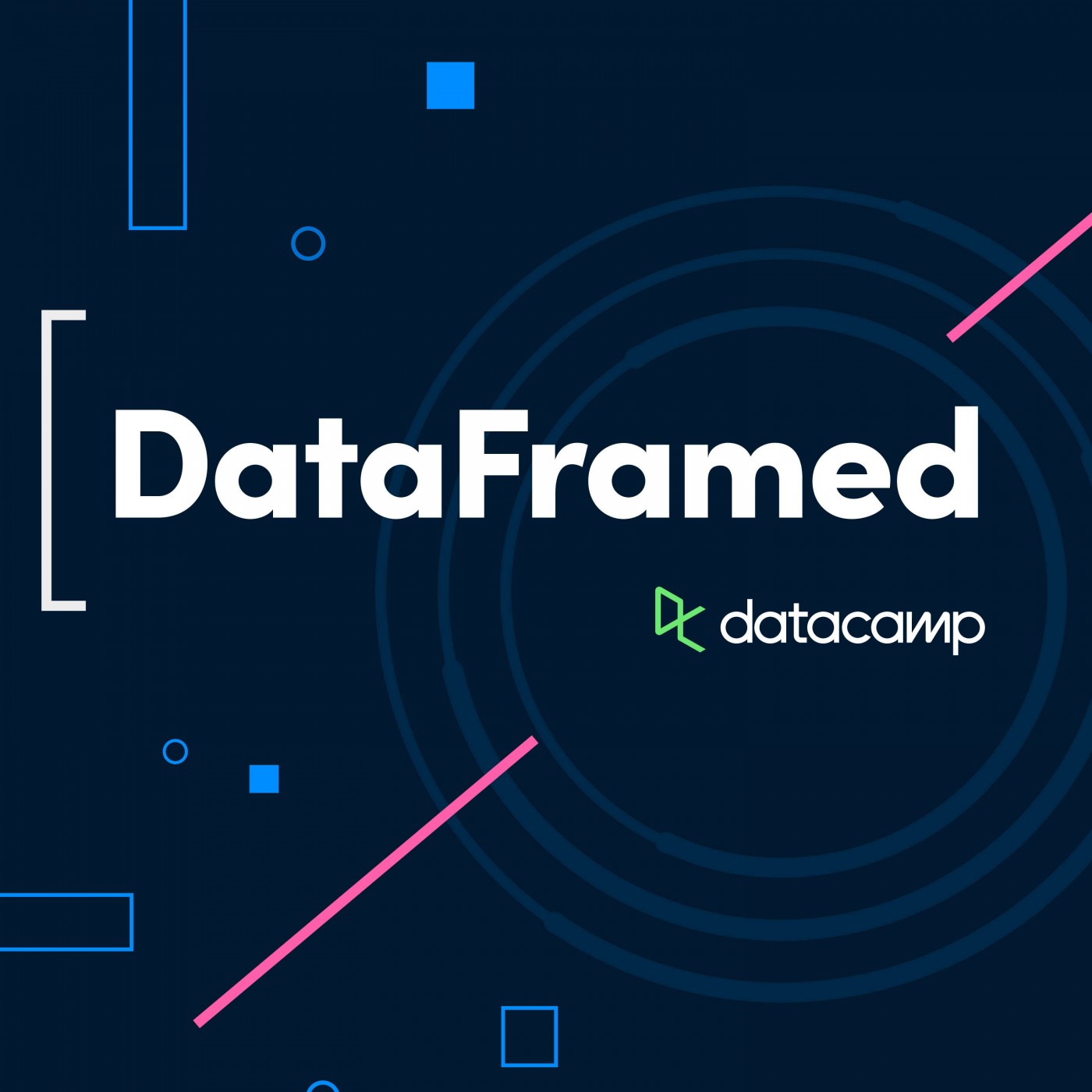
#51 Inclusivity and Data Science
This week Hugo speaks with Dr. Brandeis Marshall, about people of color and under-represented groups in data science. They’ll talk about the biggest barriers to entry for people of color, initiatives that currently exist and what we as a community can do to be as diverse and inclusive as possible.
Brandeis is an Associate Professor of Computer Science at Spelman College. Her interdisciplinary research lies in the areas of information retrieval, data science, and social media. Other research includes the BlackTwitter Project, which blends data analytics, social impact and race as a lens to understanding cultural sentiments. Brandeis is involved in a number of projects, workshops, and organizations that support data literacy and understanding, share best data practices and broaden participation in data science.
LINKS FROM THE SHOW
DATAFRAMED GUEST SUGGESTIONS
DataFramed Guest Suggestions (who do you want to hear on DataFramed?)
FROM THE INTERVIEW
Brandeis on TwitterThe BlackTwitter ProjectThe Impact of Live Tweeting on Social Movements (By Brandeis Marshall, Takeria Blunt, Tayloir Thompson)EvergreenLP: Using a social network as a learning platform (By Brandeis Marshall, Jaye Nias, Tayloir Thompson, Takeria Blunt)Journal of Computing Sciences in Colleges (By Brandeis Marshall)DSX (Data Science eXtension Faculty development and undergraduate instruction in data science) African American Women Computer Science PhDs500 Women ScientistsBlack in AIWomen in Machine Learning
FROM THE SEGMENTS
What Data Scientists Really Do (with Hugo Bowne-Anderson & Emily Robinson ~21:30 & ~41:40)
What Data Scientists Really Do, According to 35 Data Scientists (Harvard Business Review article by Hugo Bowne-Anderson)What Data Scientists Really Do, According to 50 Data Scientists (Slides from a talk by Hugo Bowne-Anderson)
Original music and sounds by The Sticks.
01:01:5204/02/2019
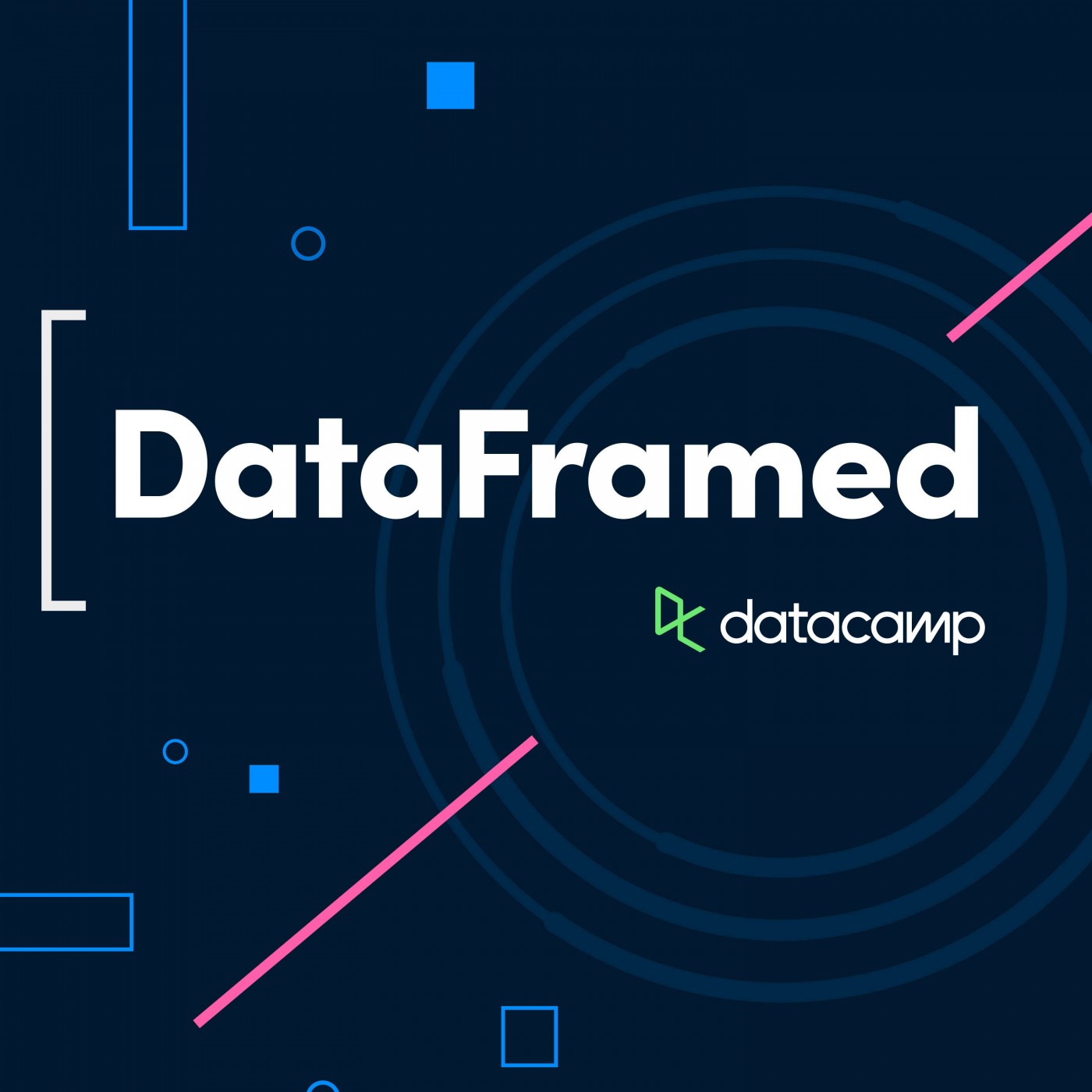
#50 Weapons of Math Destruction
In episode 50, our Season 1, 2018 finale of DataFramed, the DataCamp podcast, Hugo speaks with Cathy O’Neil, data scientist, investigative journalist, consultant, algorithmic auditor and author of the critically acclaimed book Weapons of Math Destruction. Cathy and Hugo discuss the ingredients that make up weapons of math destruction, which are algorithms and models that are important in society, secret and harmful, from models that decide whether you keep your job, a credit card or insurance to algorithms that decide how we’re policed, sentenced to prison or given parole? Cathy and Hugo discuss the current lack of fairness in artificial intelligence, how societal biases are perpetuated by algorithms and how both transparency and auditability of algorithms will be necessary for a fairer future. What does this mean in practice? Tune in to find out. As Cathy says, “Fairness is a statistical concept. It's a notion that we need to understand at an aggregate level.” And, moreover, “data science doesn't just predict the future. It causes the future.”LINKS FROM THE SHOW
DATAFRAMED SURVEY
DataFramed Survey (take it so that we can make an even better podcast for you)
DATAFRAMED GUEST SUGGESTIONS
DataFramed Guest Suggestions (who do you want to hear on Season 2?)
FROM THE INTERVIEW
Cathy on TwitterCathy's Blog MathbabeWeapons of Math Destruction: How big data increases inequality and threatens democracy by Cathy O'NeilCathy's Opinion Column, Bloomberg Doing Data Science (By Cathy O'Neil and Rachel Schutt)Cathy O'Neil & Hanna Gunn's "Ethical Matrix" paper coming soon.
FROM THE SEGMENTS
Data Science Best Practices (with Heather Nolis ~20:30)
Using docker to deploy an R plumber API (By Jonathan Nolis and Heather Nolis)Enterprise Web Services with Neural Networks Using R and TensorFlow (By Jonathan Nolis and Heather Nolis)
Data Science Best Practices (with Ben Skrainka ~39:35)
The Clean Coder Blog (By Robert C. Martin)James Shore’s blog post on Red, Green, RefactorJeff Knupp’s Python Unittesting tutorial (general unit tests in Python)John Myles White’s Intro to Unit Testing in R
Original music and sounds by The Sticks.
55:5326/11/2018
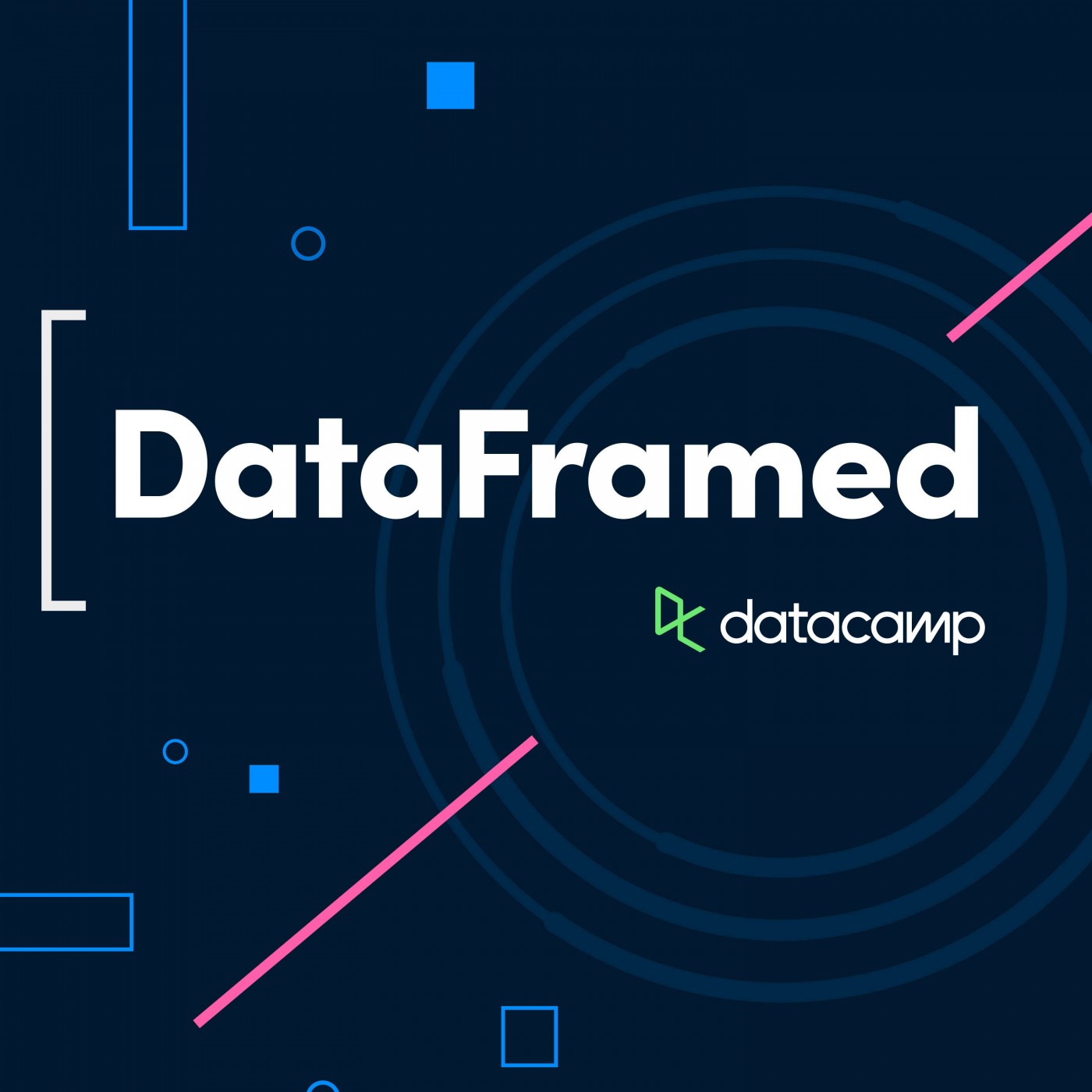
#49 Data Science Tool Building
Hugo speaks with Wes McKinney, creator of the pandas project for data analysis tools in Python and author of Python for Data Analysis, among many other things. Wes and Hugo talk about data science tool building, what it took to get pandas off the ground and how he approaches building “human interfaces to data” to make individuals more productive. On top of this, they’ll talk about the future of data science tooling, including the Apache arrow project and how it can facilitate this future, the importance of DataFrames that are portable between programming languages and building tools that facilitate data analysis work in the big data limit. Pandas initially arose from Wes noticing that people were nowhere near as productive as they could be due to lack of tooling & the projects he’s working on today, which they’ll discuss, arise from the same place and present a bold vision for the future.LINKS FROM THE SHOWDATAFRAMED SURVEY
DataFramed Survey (take it so that we can make an even better podcast for you)
DATAFRAMED GUEST SUGGESTIONS
DataFramed Guest Suggestions (who do you want to hear on Season 2?)
FROM THE INTERVIEW
Wes on TwitterRoads and Bridges: The Unseen Labor Behind Our Digital Infrastructure by Nadia Eghbalpandas, an open source, BSD-licensed library providing high-performance, easy-to-use data structures and data analysis tools for the Python programming language.Ursa Labs
FROM THE SEGMENTS
Data Science Best Practices (with Ben Skrainka ~17:10)
To Explain or To Predict? (By Galit Shmueli)Statistical Modeling: The Two Cultures (By Leo Breiman)The Book of Why (By Judea Pearl & Dana Mackenzie)
Studies in Interpretability (with Peadar Coyle at ~39:00)
Modelling Loss Curves in Insurance with RStan (By Mick Cooney)Lime: Explaining the predictions of any machine learning classifier Probabilistic Programming Primer
Original music and sounds by The Sticks.
57:4119/11/2018
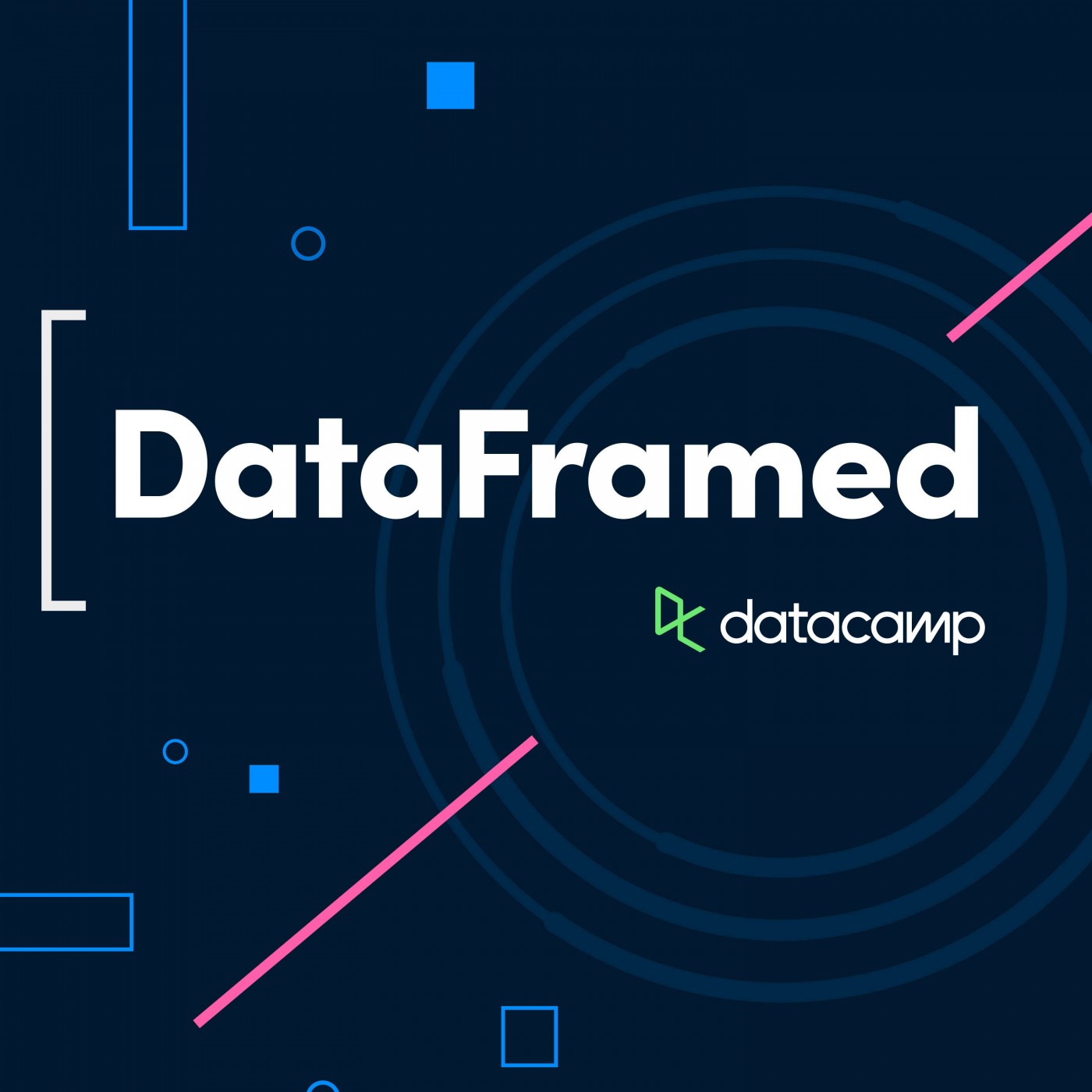
#48 Managing Data Science Teams
In this episode of DataFramed, the DataCamp podcast, Hugo speaks with Angela Bassa about managing data science teams. Angela is Director of Data Science at iRobot, where she leads the team through development of machine learning algorithms, sentiment analysis, and anomaly detection processes. iRobot are the makers of consumer robots that we all know and love, like the Roomba, and the Braava which are, respectively, a robotic vacuum cleaner and a robotic mop. Angela will talk about how to get into data science management, the most important strategies to ensure that your data science team delivers value to the organization, how to hire data scientists and key points to consider as your data science team grows over time, in addition to the types of trade-offs you need to make as a data science manager and how you make the right ones. Along the way, you’ll see why a former marine biologist has the skills and ways of thinking to be a super data scientist at a company like iRobot and you’ll also see the importance of throwing data analysis parties.LINKS FROM THE SHOW
FROM THE INTERVIEW
Angela on TwitterHBR NewslettersiRobot CareersData Science Internship
FROM THE SEGMENTS
Correcting Data Science Misconceptions (w/ Heather Nolis ~18:45)
Using docker to deploy an R plumber API (By Jonathon Nolis)Enterprise Web Services with Neural Networks Using R and TensorFlow (By Jonathan Nolis and Heather Nolis)
Project of the Month (w/ David Venturi ~38:45)
Rise and Fall of Programming Languages (R Project by David Robinson)Learn, Practice, Apply! (By Ramnath Vaidyanathan)Apply to create a DataCamp project!
Original music and sounds by The Sticks.
50:1812/11/2018
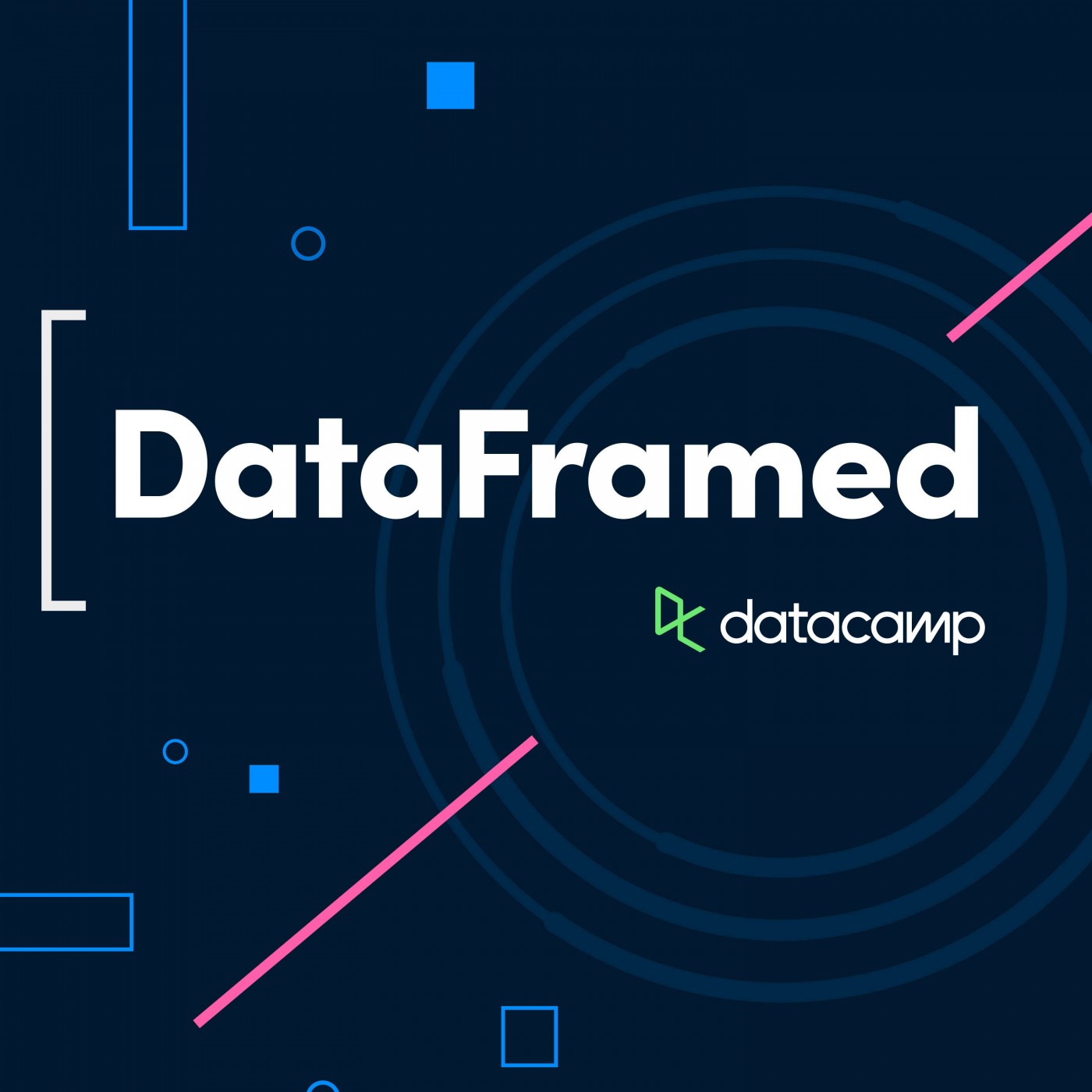
#47 Human-centered Design in Data Science
Hugo speaks with Peter Bull about the importance of human-centered design in data science. Peter is a data scientist for social good and co-founder of Driven Data, a company that brings cutting-edge practices in data science and crowdsourcing to some of the world's biggest social challenges and the organizations taking them on, including machine learning competitions for social good. They’ll speak about the practice of considering how humans interact with data and data products and how important it is to consider them while designing your data projects. They’ll see how human-centered design provides a robust and reproducible framework for involving the end-user all through the data work, illuminated by examples such as DrivenData’s work in financial services and Mobile Money in Tanzania. Along the way, they’ll discuss the role of empathy in data science, the increasingly important conversation around data ethics and much, much more.LINKS FROM THE SHOW
FROM THE INTERVIEW
Peter on TwitterDrivenDataDeon (Ethics Checklist)Cookiecutter Data ScienceIf you liked this interview, you might be interested in working with DrivenData! Currently, the team is looking for a software engineer who loves the idea of building Python applications for social impact. Apply Here!
FROM THE SEGMENTS
Probability Distributions and their Stories (with Justin Bois at ~24:00)
Justin's Website at CaltechProbability distributions and their stories (By Justin Bois)
Studies in Interpretability (with Peadar Coyle at ~38:10)
Interpretable ML SymposiumHow will the GDPR impact machine learning? (By Andrew Burt)How to use Bayesian Stats in your daily job (Gates, Perry, Zorn (2002))Fairness in Machine Learning (By Moritz Hardt)
Original music and sounds by The Sticks.
01:02:3105/11/2018
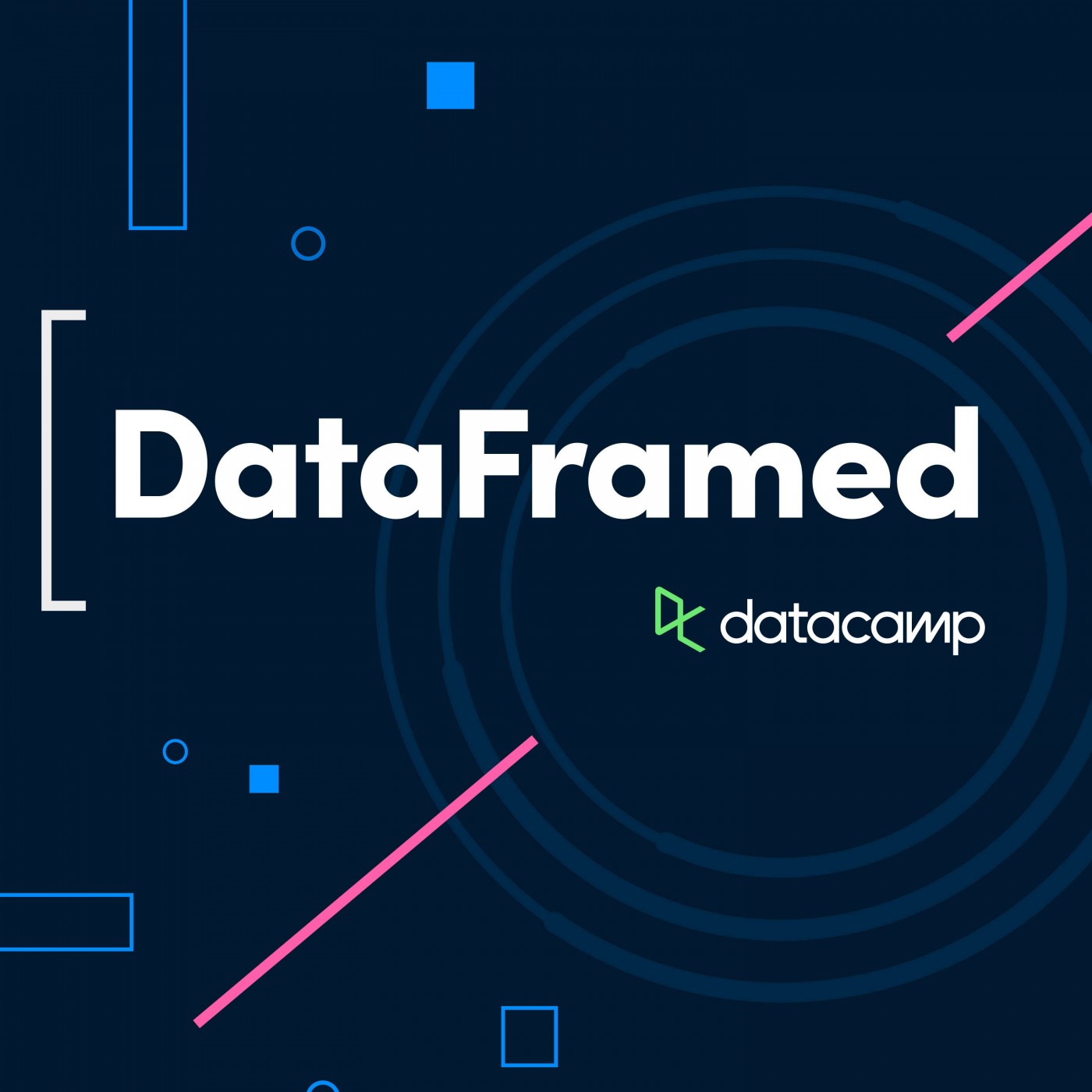
#46 AI in Healthcare, an Insider's Account
In this episode of DataFramed, a DataCamp podcast, Hugo speaks with Arnaub Chatterjee. Arnaub is a Senior Expert and Associate Partner in the Pharmaceutical and Medical Products group at McKinsey & Company. They’ll discuss cutting through the hype about artificial intelligence (AI) and machine learning (ML) in healthcare by looking at practical applications and how McKinsey & Company is helping the industry evolve.
Tune in for an insider’s account into what has worked in healthcare, from ML models being used to predict nearly everything in clinical settings, to imaging analytics for disease diagnosis, to wound therapeutics. Will robots and AI replace disciplines such as radiology, ophthalmology, and dermatology? How have the moving parts of data science work evolved in healthcare? What does the future of data science, ML and AI in healthcare hold? Stick around to find out.
LINKS FROM THE SHOW
FROM THE INTERVIEW
McKinsey Analytics on TwitterHot off the press article for HBR’s Future of Healthcare online forum (By Arnaub Chatterjee)Our latest piece on the promise & challenge of AI (By James Manyika and Jacques Bughin)Are robots coming for our jobs? (mckinsey.com)Analytics Careers page (mckinsey.com)How we help clients in healthcare analytics (mckinsey.com)AI analysis of 400+ use cases, including ones in healthcare (By Michael Chui et al. mckinsey.com)
FROM THE SEGMENTS
Machines that Multi-task (with Manny Moss)
Part 1 at ~21:05
Responsible AI in Consumer EnterpriseHilary Mason, DJ Patil and Mike Loukides on Data EthicsEthicalOS Tookit
Part 2 at ~40:00
21 Definitions of Fairness Tutorial from FAT* (Arvind Naranayan)Kate Crawford's keynote address "The Trouble with Bias" from NIPS 2017The (im)possibility of Fairness (Sorelle et al. arXiv.org)Learning from disparate data sources (Li Y et al. PubMed.gov)Distributed Multi-task Learning (Liyang Xie et al. KDD.org)The Cost of Fairness in Binary Classification (Aditya Krishna Menon et al. proceedings.mlr.press)
Original music and sounds by The Sticks.
01:02:2729/10/2018
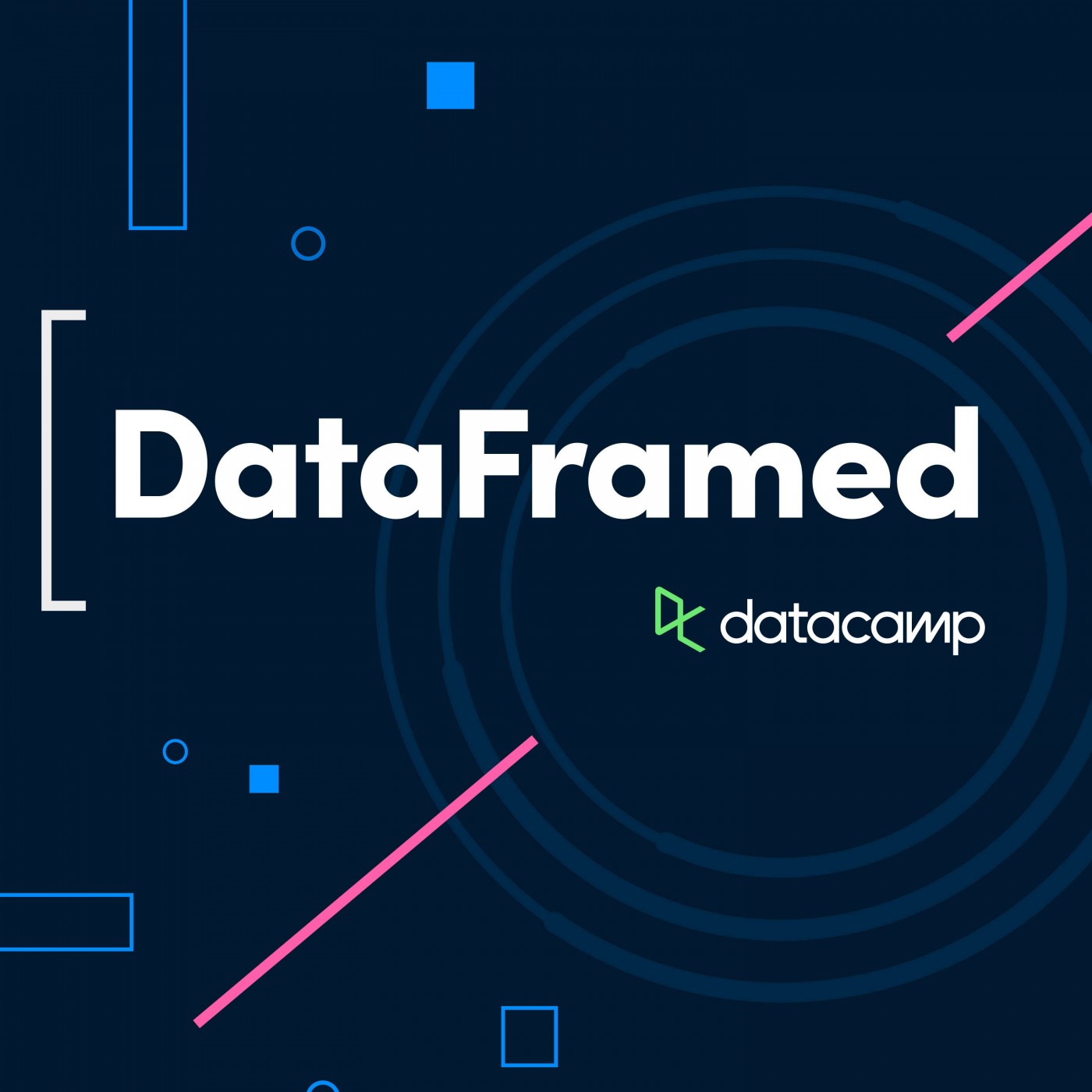
#45 Decision Intelligence and Data Science
In this episode of DataFramed, Hugo speaks with Cassie Kozyrkov, Chief Decision Scientist at Google Cloud. Cassie and Hugo will be talking about data science, decision making and decision intelligence, which Cassie thinks of as data science plus plus, augmented with the social and managerial sciences. They’ll talk about the different and evolving models for how the fruits of data science work can be used to inform robust decision making, along with pros and cons of all the models for embedding data scientists in organizations relative to the decision function. They’ll tackle head on why so many organizations fail at using data to robustly inform decision making, along with best practices for working with data, such as not verifying your results on the data that inspired your models. As Cassie says, “Split your damn data”.Links from the show
FROM THE INTERVIEW
Cassie on Twitter Is data science a bubble? (By Cassie Kozyrkov, Hackernoon)Incompetence, delegation, and population (By Cassie Kozyrkov, Hackernoon)Populations — You’re doing it wrong (By Cassie Kozyrkov, Hackernoon)What on earth is data science? (By Cassie Kozyrkov, Hackernoon)
FROM THE SEGMENTS
Probability Distributions and their Stories (with Justin Bois at ~19:45)
Justin's Website at CaltechProbability distributions and their stories (By Justin Bois)
Machines that Multi-Task (with Friederike Schüür of Fast Forward Labs ~43:45)
Sebastian’s Ruder’s Overview of Multi-Task Learning in Deep Neural NetworksMulti-Task Learning for NLP, also by Sebastian RuderGANs for Fake Celebrity Images (Karras et al, Nvidia)Adversarial Multi-Task Learning for Text Classification (Liu et al., arXiv.org)
Original music and sounds by The Sticks.
01:05:4122/10/2018
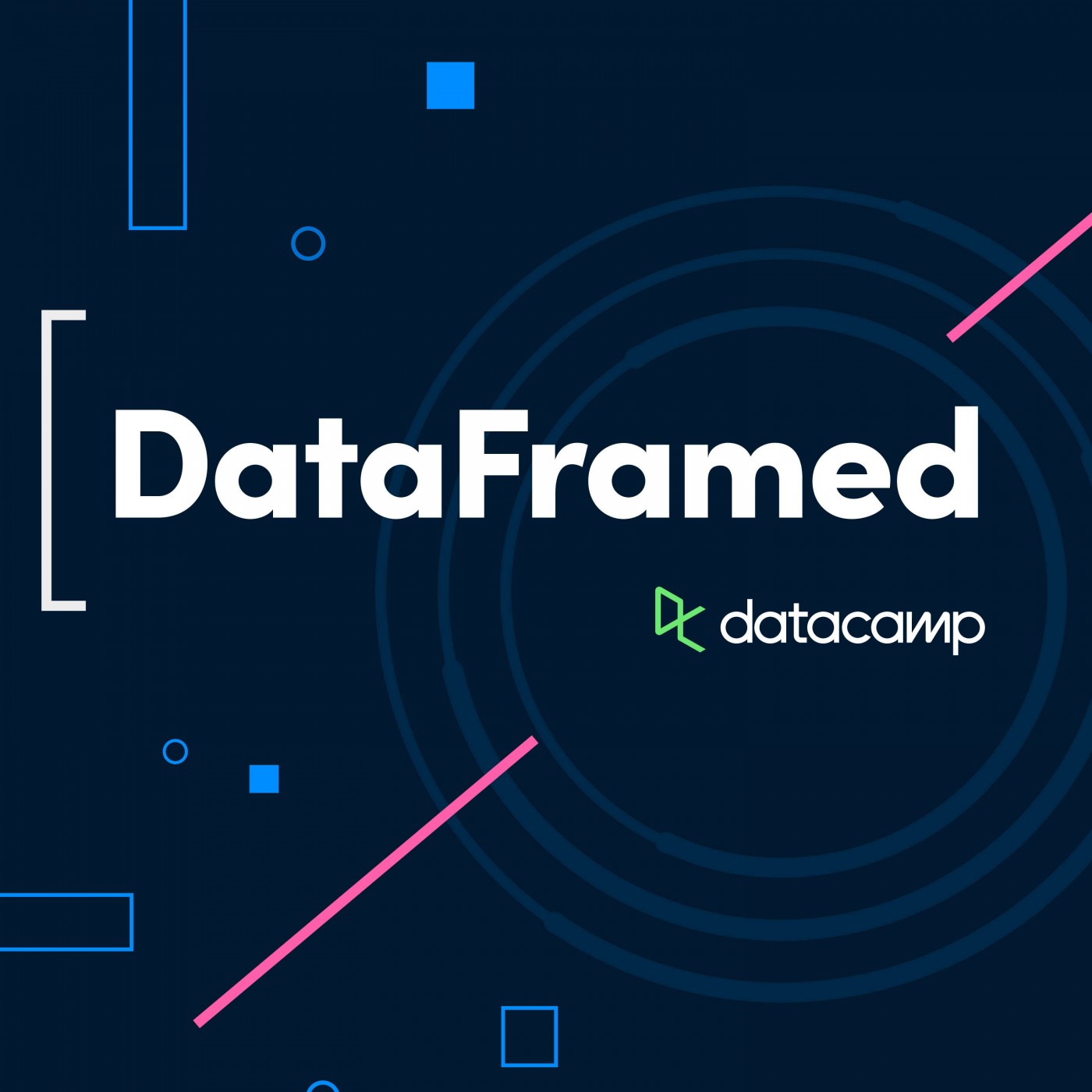
#44 Project Jupyter and Interactive Computing
In this episode of DataFramed, Hugo speaks with Brian Granger, co-founder and co-lead of Project Jupyter, physicist and co-creator of the Altair package for statistical visualization in Python.
They’ll speak about data science, interactive computing, open source software and Project Jupyter. With over 2.5 million public Jupyter notebooks on github alone, Project Jupyter is a force to be reckoned with. What is interactive computing and why is it important for data science work? What are all the the moving parts of the Jupyter ecosystem, from notebooks to JupyterLab to JupyterHub and binder and why are they so relevant as more and more institutions adopt open source software for interactive computing and data science? From Netflix running around 100,000 Jupyter notebook batch jobs a day to LIGO’s Nobel prize winning discovery of gravitational waves publishing all their results reproducibly using Notebooks, Project Jupyter is everywhere.
Links from the show
FROM THE INTERVIEW
Brian on Twitter Project JupyterBeyond Interactive: Notebook Innovation at Netflix (Ufford, Pacer, Seal, Kelley, Netflix Tech Blog)Gravitational Wave Open Science Center (Tutorials)JupyterCon YouTube Playlistjupyterstream Github Repository
FROM THE SEGMENTS
Machines that Multi-Task (with Friederike Schüür of Fast Forward Labs)Part 1 at ~24:40
Brief Introduction to Multi-Task Learning (By Friederike Schüür)Overview of Multi-Task Learning Use Cases (By Manny Moss)Multi-Task Learning for the Segmentation of Building Footprints (Bischke et al., arXiv.org)Multi-Task as Question Answering (McCann et al., arXiv.org)The Salesforce Natural Language Decathlon: A Multitask Challenge for NLP
Part 2 at ~44:00
Rich Caruana’s Awesome Overview of Multi-Task Learning and Why It WorksSebastian’s Ruder’s Overview of Multi-Task Learning in Deep Neural NetworksMassively Multi-Task Network for Drug Discovery, 259 Tasks (!) (Ramsundar et al. arXiv.org)Brief Overview of Multi-Task Learning with Video of Newsie, the Prototype (By Friederike Schüür)
Original music and sounds by The Sticks.
01:05:1115/10/2018
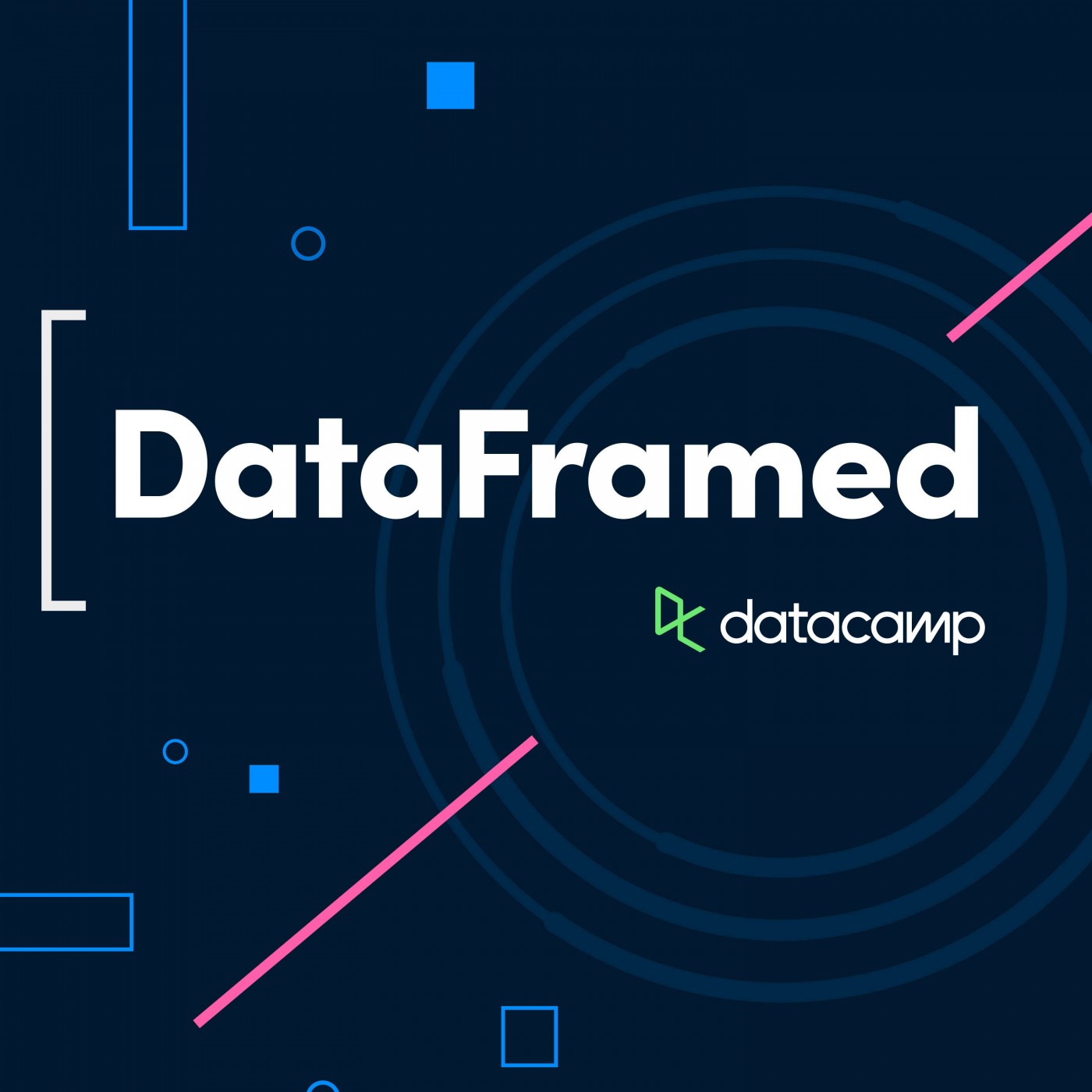
#43 Election Forecasting and Polling
Hugo speaks with Andrew Gelman about statistics, data science, polling, and election forecasting. Andy is a professor of statistics and political science and director of the Applied Statistics Center at Columbia University and this week we’ll be talking the ins and outs of general polling and election forecasting, the biggest challenges in gauging public opinion, the ever-present challenge of getting representative samples in order to model the world and the types of corrections statisticians can and do perform. "Chatting with Andy was an absolute delight and I cannot wait to share it with you!"-Hugo
Links from the show
FROM THE INTERVIEW
Andrew's Blog Andrew on Twitter We Need to Move Beyond Election-Focused Polling (Gelman and Rothschild, Slate)We Gave Four Good Pollsters the Same Raw Data. They Had Four Different Results (Cohn, The New York Times).19 things we learned from the 2016 election (Gelman and Azari, Science, 2017)The best books on How Americans Vote (Gelman, Five Books)The best books on Statistics (Gelman, Five Books)Andrew's Research
FROM THE SEGMENTS
Statistical Lesson of the Week (with Emily Robinson at ~13:30)
The five Cs (Loukides, Mason, and Patil, O'Reilly)
Data Science Best Practices (with Ben Skrainka~40:40)
Oberkampf & Roy’s Verification and Validation in Scientific Computing provides a thorough yet very readable treatment A comprehensive framework for verification, validation, and uncertainty quantification in scientific computing (Roy and Oberkampf, Science Direct)
Original music and sounds by The Sticks.
01:05:1508/10/2018
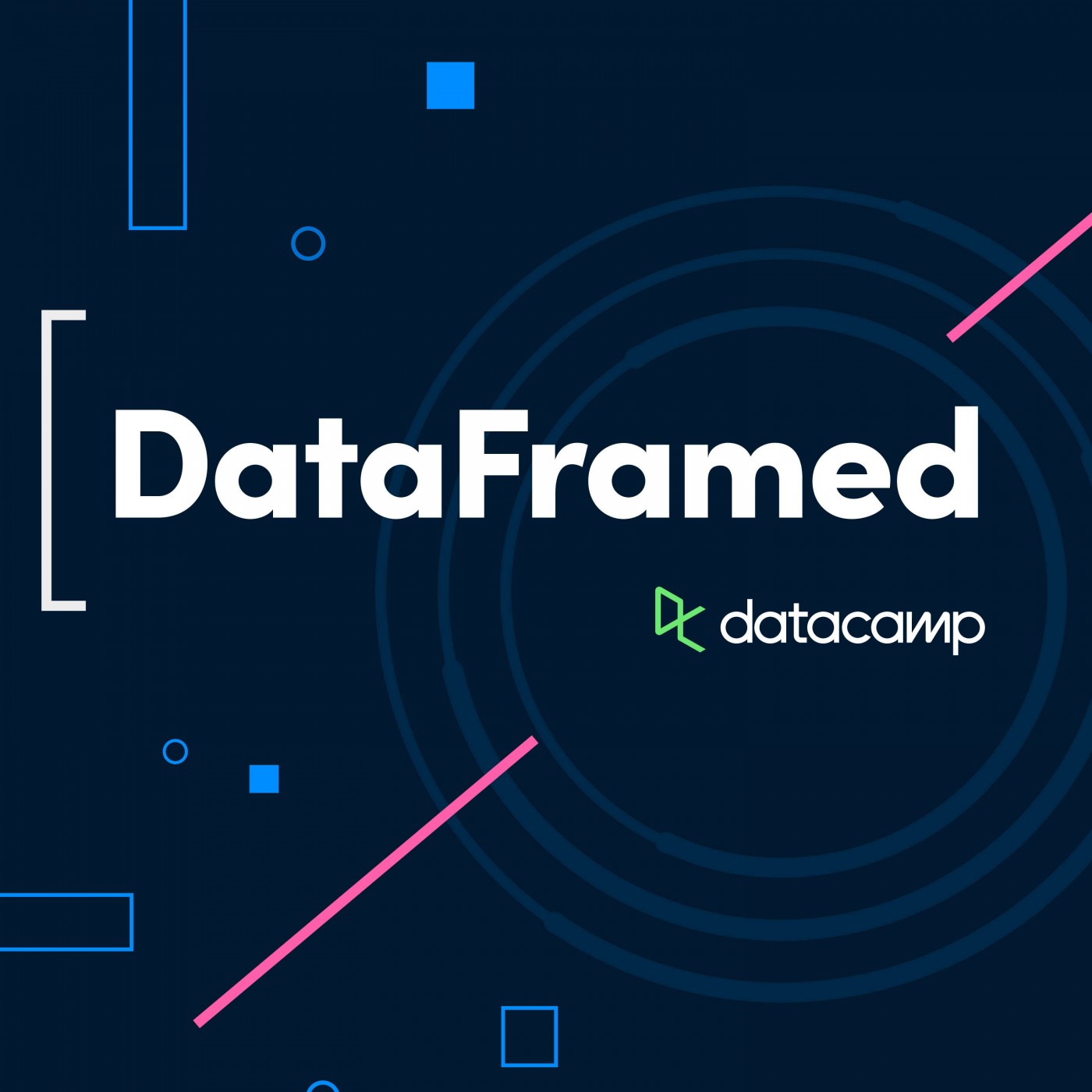
#42 Full Stack Data Science
Hugo speaks with Vicki Boykis about what full-stack end-to-end data science actually is, how it works in a consulting setting across various industries and why it’s so important in developing modern data-driven solutions to business problems. Vicki is a full-stack data scientist and senior manager at CapTech Consulting, working on projects in machine learning and data engineering. They'll also discuss the increasing adoption of data science in the cloud technologies and associated pitfalls, along with how to equip businesses with the skills to maintain the data products you developed for them. All this and more: Hugo is pumped!
Links from the show
FROM THE INTERVIEW
Vicki's Tech Blog
Vicki on Twitter
CapTech Consulting
Vicki's Tweet about Programming
Building a Twitter art bot with Python, AWS, and socialist realism art
FROM THE SEGMENTS
Data Science Best Practices (with Ben Skrainka~15:00)
Cross-industry standard process for data mining
Fundamentals of Machine Learning for Predictive Data Analytics
Statistical Lesson of the Week (with Emily Robinson at ~32:05)
Sex Bias in Graduate Admissions: Data from Berkeley (Bickel et al., Science, 1975)
Time Series Analysis Tutorial with Python
Original music and sounds by The Sticks.
50:5001/10/2018
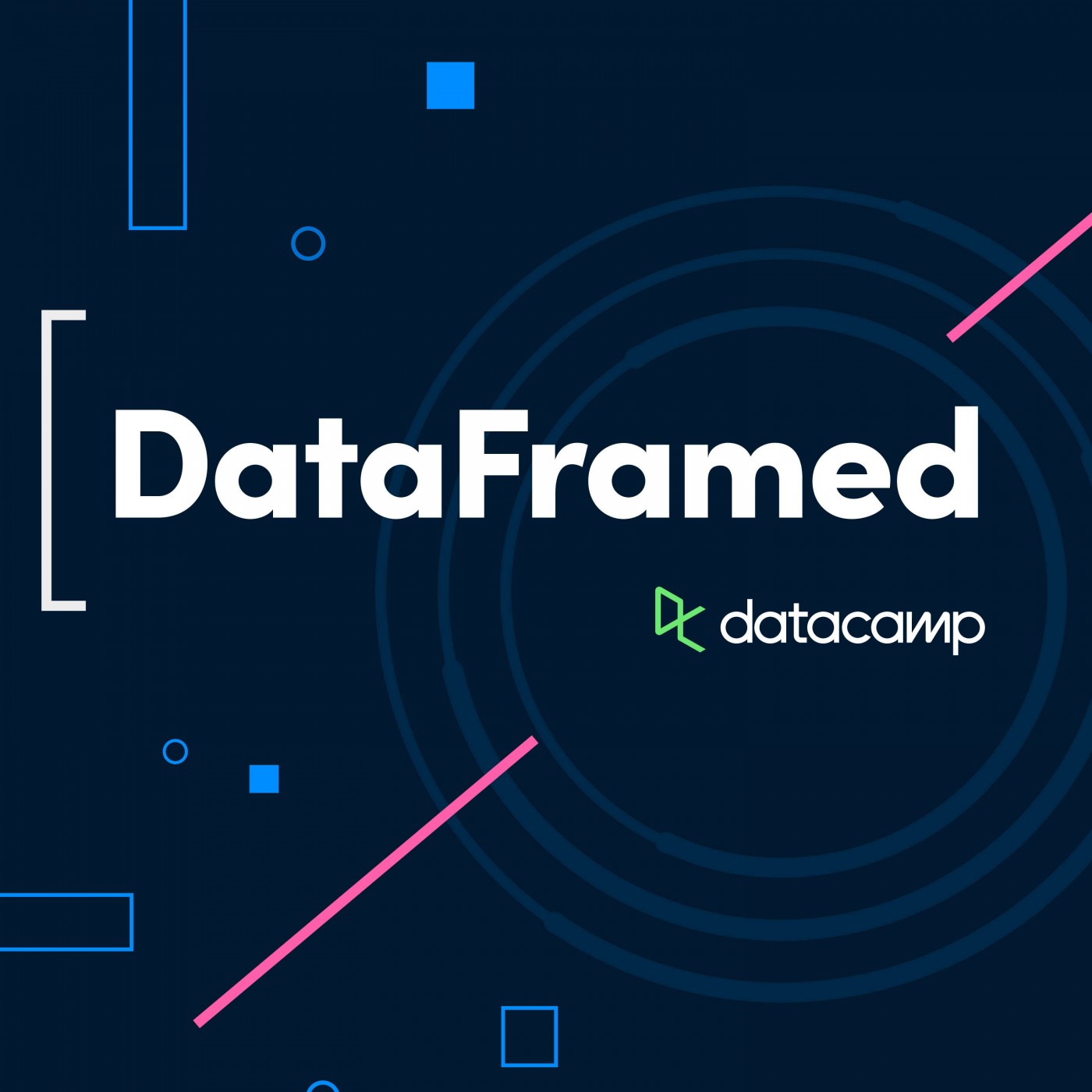
#41 Uncertainty in Data Science
Hugo speaks with Allen Downey about uncertainty in data science. Allen is a professor of Computer Science at Olin College and the author of a series of free, open-source textbooks related to software and data science. Allen and Hugo speak about uncertainty in data science and how we, as humans, are not always good at thinking about uncertainty, which we need be to in such an uncertain world. Should we have been surprised at the outcome of the 2016 election? What approaches can we, as a data reporting community, take to communicate around uncertainty better in the future? From election forecasting to health and safety, thinking about uncertainty and using data & data-oriented tools to communicate around uncertainty are essential.
Links from the show
FROM THE INTERVIEW
Data Science Data Optimism
Allen's Twitter
List of cognitive biases
Why are we so surprised? (Allen's Blog)
Probably Overthinking It (Allen Downey's Blog)
Think Stats (Allen's Book)
There is only one test! (Allen's Blog)
FROM THE SEGMENT
Statistical Distributions and their Stories (with Justin Bois at ~27:00)
Justin's Website at Caltech
Probability distributions and their stories
LeBron James Field Goals
Original music and sounds by The Sticks.
58:3824/09/2018
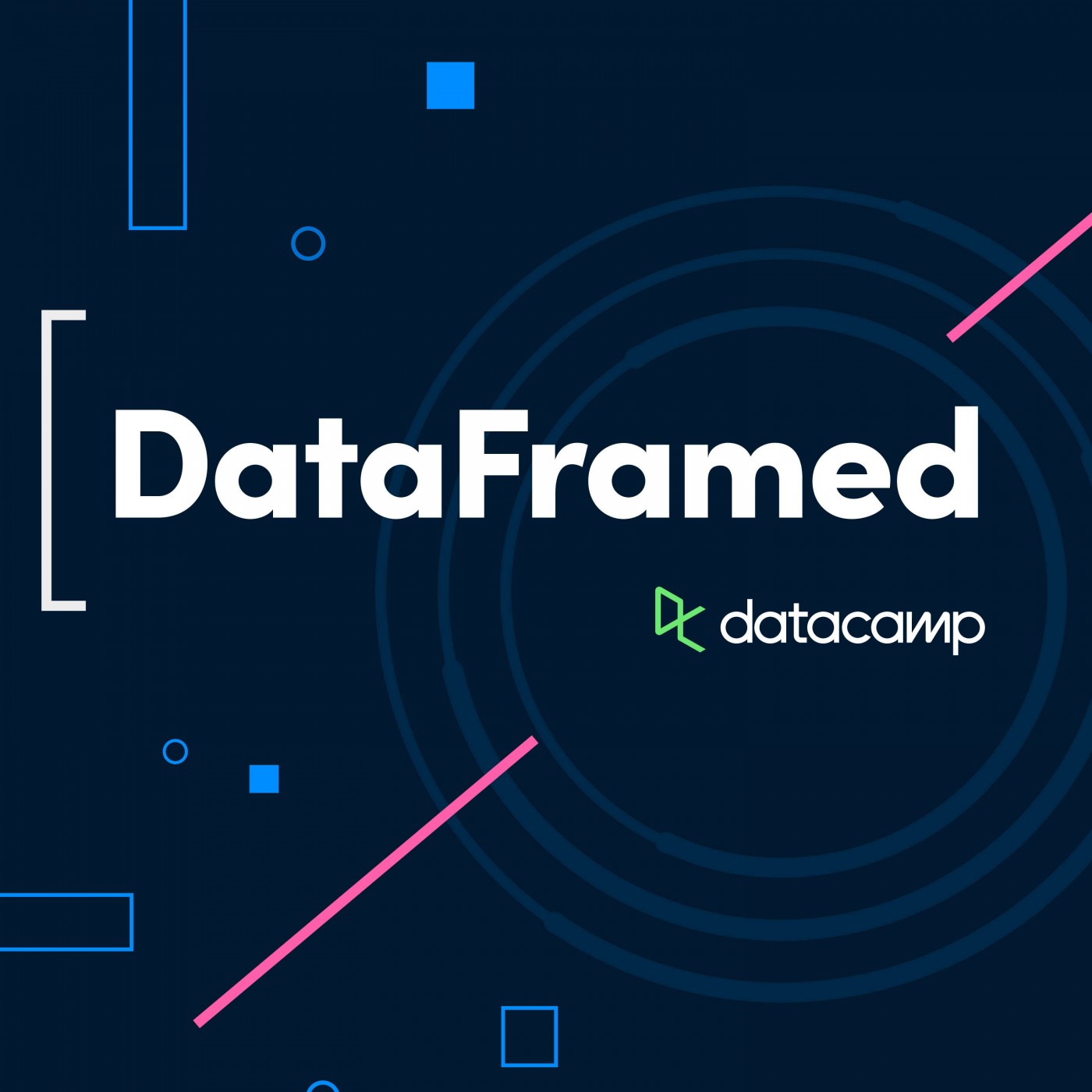
#40 Becoming a Data Scientist
Hugo speaks with Renee Teate about the many paths to becoming a data scientist. Renee is a Data Scientist at higher ed analytics start-up HelioCampus, and creator and host of the Becoming a Data Scientist Podcast. In addition to discussing the many possible ways to become becoming a data scientist, they will discuss the common data scientist profiles and how to figure out which ones may be a fit for you. They’ll also dive into the fact that you need to figure out both where you are in terms of skills and knowledge and where you want to go in terms of your career. Renee has a bunch of great suggestions for aspiring data scientists and also flags several important pitfalls and warnings. On top of this, they'll dive into how much statistics, linear algebra and calculus you need to know in order to become an effective data scientist and/or data analyst.
Links from the show
FROM THE INTERVIEW
Becoming a Data Scientist (Renée's Blog)
Renée's Twitter
Data Sci Guide (Data Science Learning Directory)
FROM THE SEGMENTS
Statistical Distributions and their Stories (with Justin Bois at ~19:20)
Justin's Website at Caltech
Probability distributions and their stories
Programming Topic of the Week (with Emily Robinson at ~43:20)
Categorical Data in the Tidyverse, a DataCamp Course taught by Emily Robinson.
R for Data Science Book by Hadley Wickham (Factors Chapter)
Inference for Categorical Data, a DataCamp Course taught by Andrew Bray.
stringsAsFactors: An unauthorized biography (Roger Peng, July 24, 2015)
Wrangling categorical data in R (Amelia McNamara & Nicholas J Horton, August 30, 2017)
Original music and sounds by The Sticks.
01:01:0217/09/2018
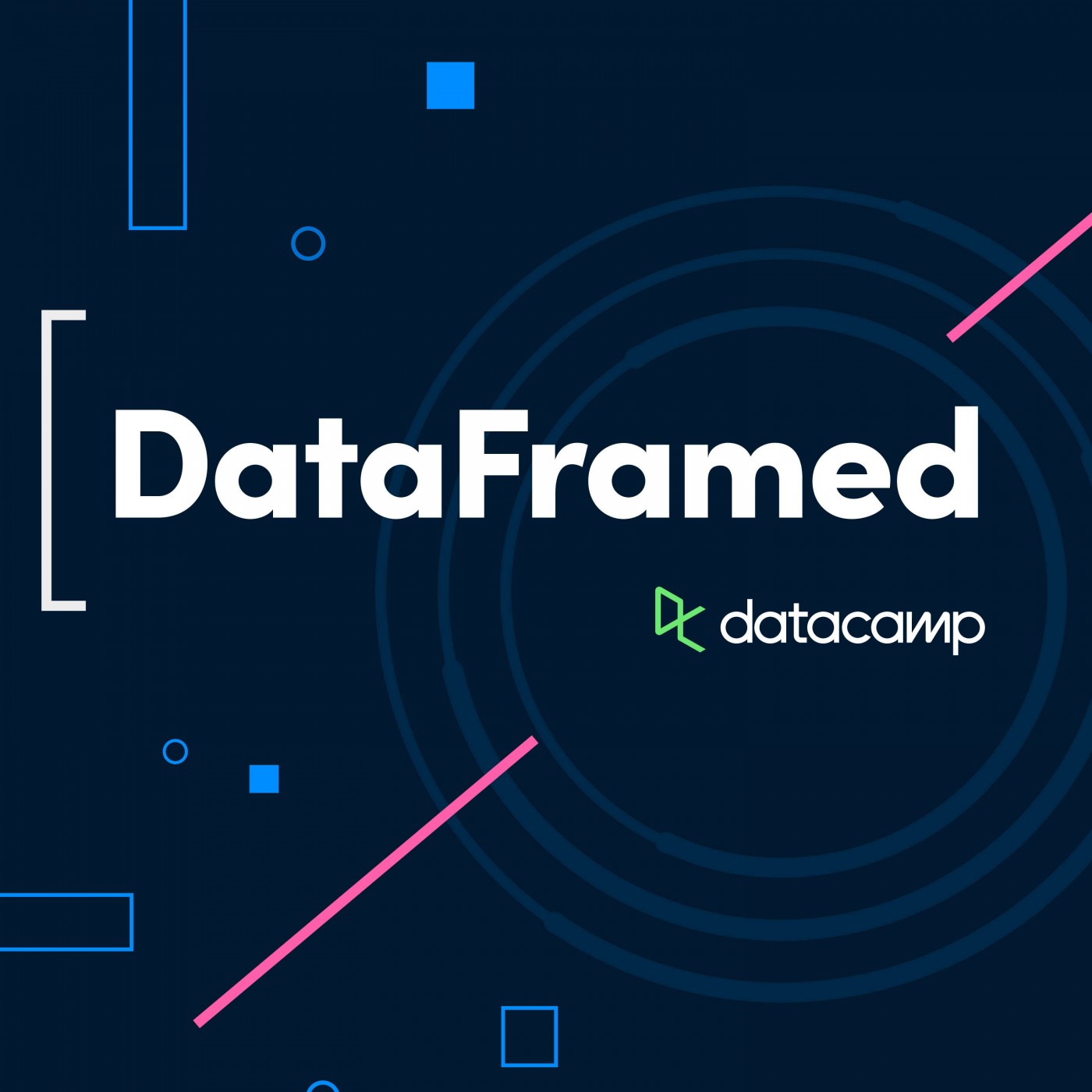
#39 Data Science at Stitch Fix
Hugo speaks with Eric Colson, Chief Algorithms Officer at Stitch Fix, an online personal styling service reinventing the shopping experience by delivering one-to-one personalization to their clients through the combination of data science and human judgment. Eric is responsible for the creation of dozens of algorithms at Stitch Fix that are pervasive to nearly every function of the company, from merchandise, inventory, and marketing to forecasting and demand, operations, and the styling recommender system. Join for all of this and more.
Links from the show
FROM THE INTERVIEW
Stitch Fix Algorithm Tour
Warehouse Maps, Movie Recommendation, Structural Biology
Advice for Data Scientists on where to work
More Human Humans: how our work-life can be improved by ceding tasks to machines.
Learning from Textual Feedback (natural Language processing)
Deep Style: Teaching machines about style from images
Hybrid Designs
You Can’t Make this stuff up … or can you? The Blissful Ignorance of the Narrative Fallacy
FROM THE SEGMENTS
Blog Post of the Week (with Emily Robinson)
Doing Good Data Science by Mike Loukides, Hilary Mason and DJ Patil
Original music and sounds by The Sticks.
59:3610/09/2018
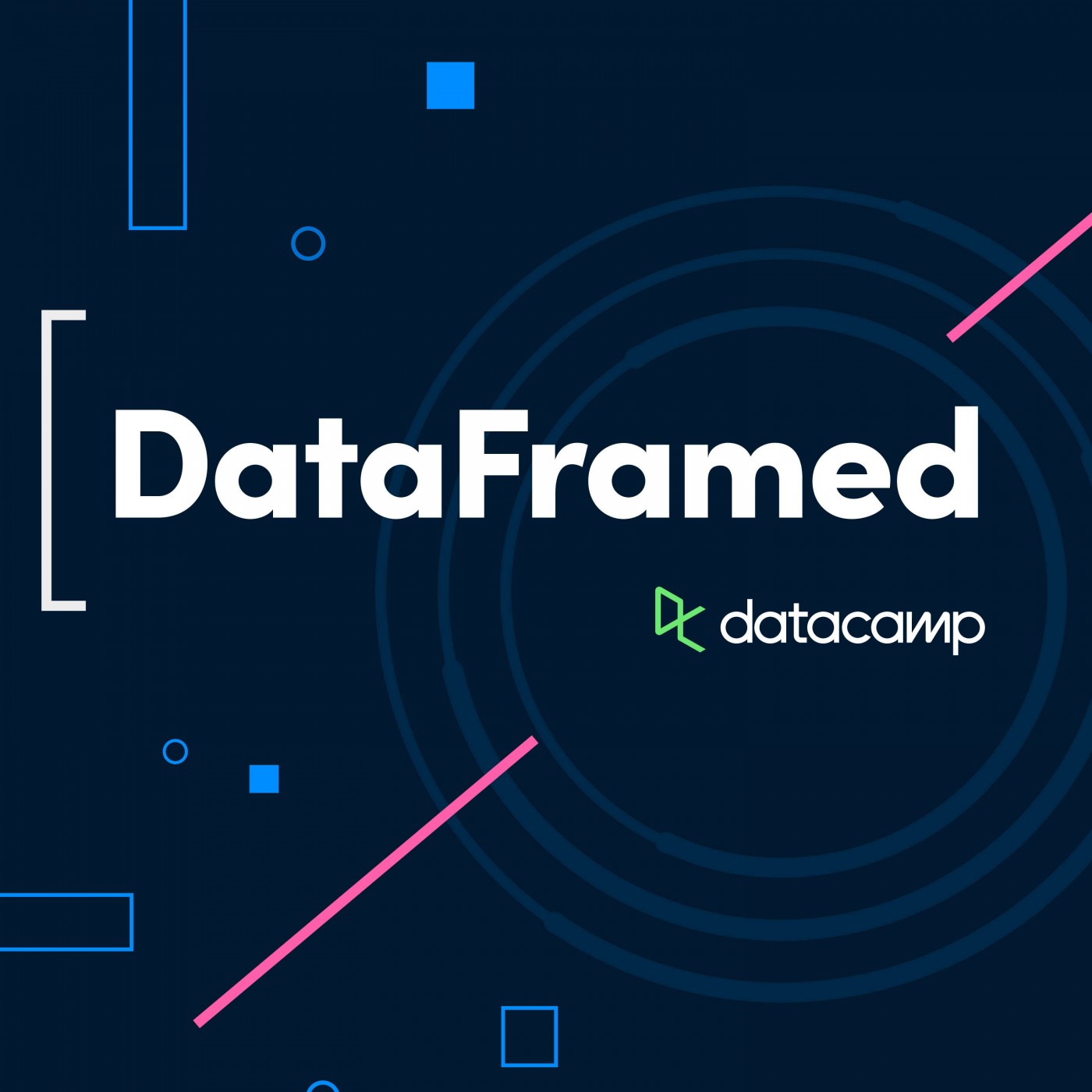
#38 Data Products, Dashboards and Rapid Prototyping
Meet Tanya Cashorali, a founding partner of TCB Analytics, a Boston-based data consultancy. Tanya started her career in bioinformatics and has applied her experience to other industries such as healthcare, finance, retail, and sports. We’ll be talking about what it means to be a data consultant, the wide range of industries that Tanya works in, the impact of data products in her work and the importance of rapid prototyping and getting MVPs or minimum viable products out the door. How does Tanya balance the trade-off between rapid prototyping and building fully mature data products? How does this play out in particular cases in the healthcare and telecommunications spaces? How has her ability to do this evolved as a function of open source software development? We’ll also dive into how general data literacy has evolved, how it can help decision making in business more generally, the data science skills gap and how many data science hiring processes are broken and how to fix them.
51:2903/09/2018
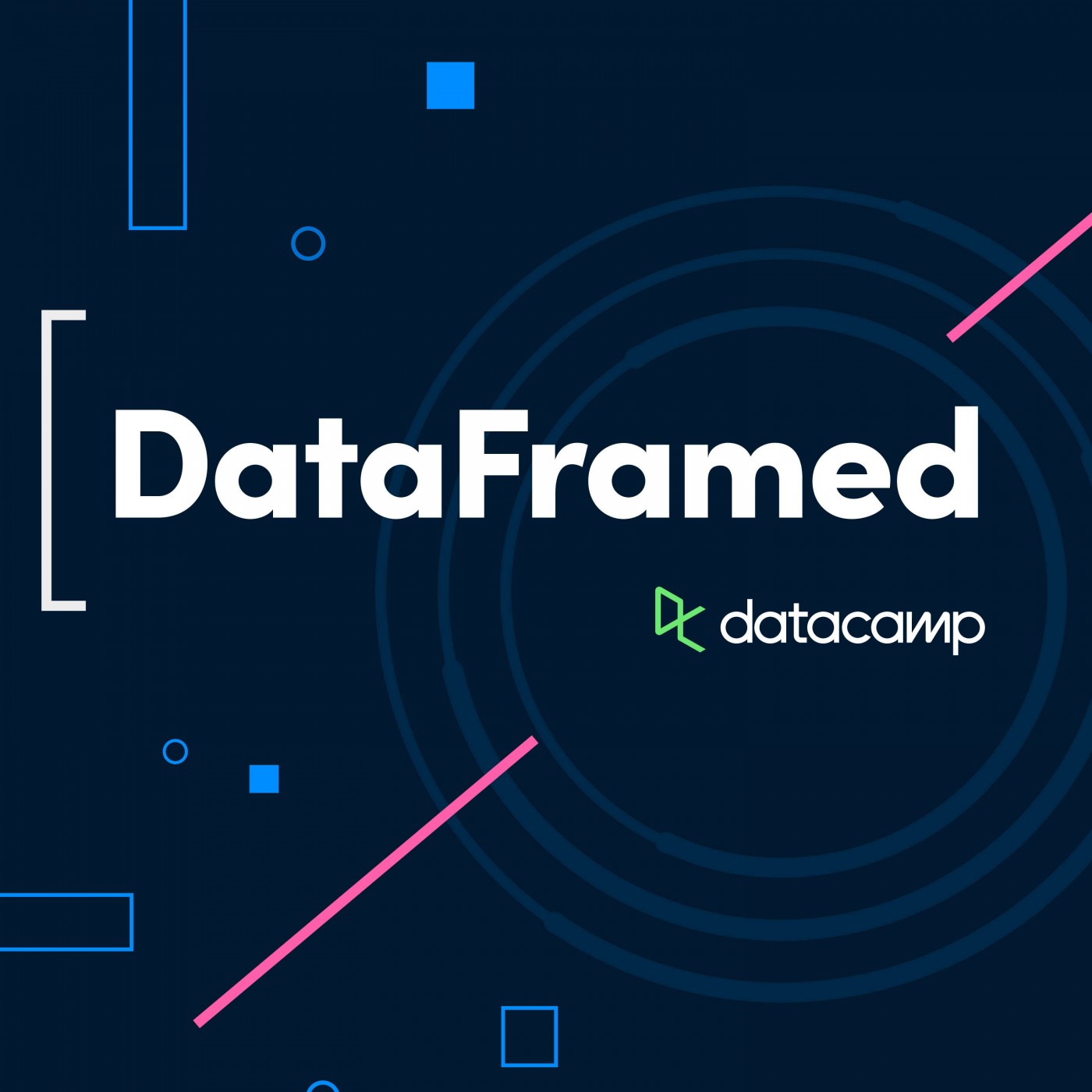
#37 Data Science and Insurance
Hugo speaks with JD Long, VP of risk management for Renaissance reinsurance, about applications of data science techniques to the omnipresent worlds of insurance, reinsurance, risk management and uncertainty. What are the biggest challenges in insurance and reinsurance that data science can impact? How does JD go about building risk representations of every deal? How can thinking in a distributed fashion allow us to think about risk and uncertainty? What is the role of empathy in data science?
59:3427/08/2018
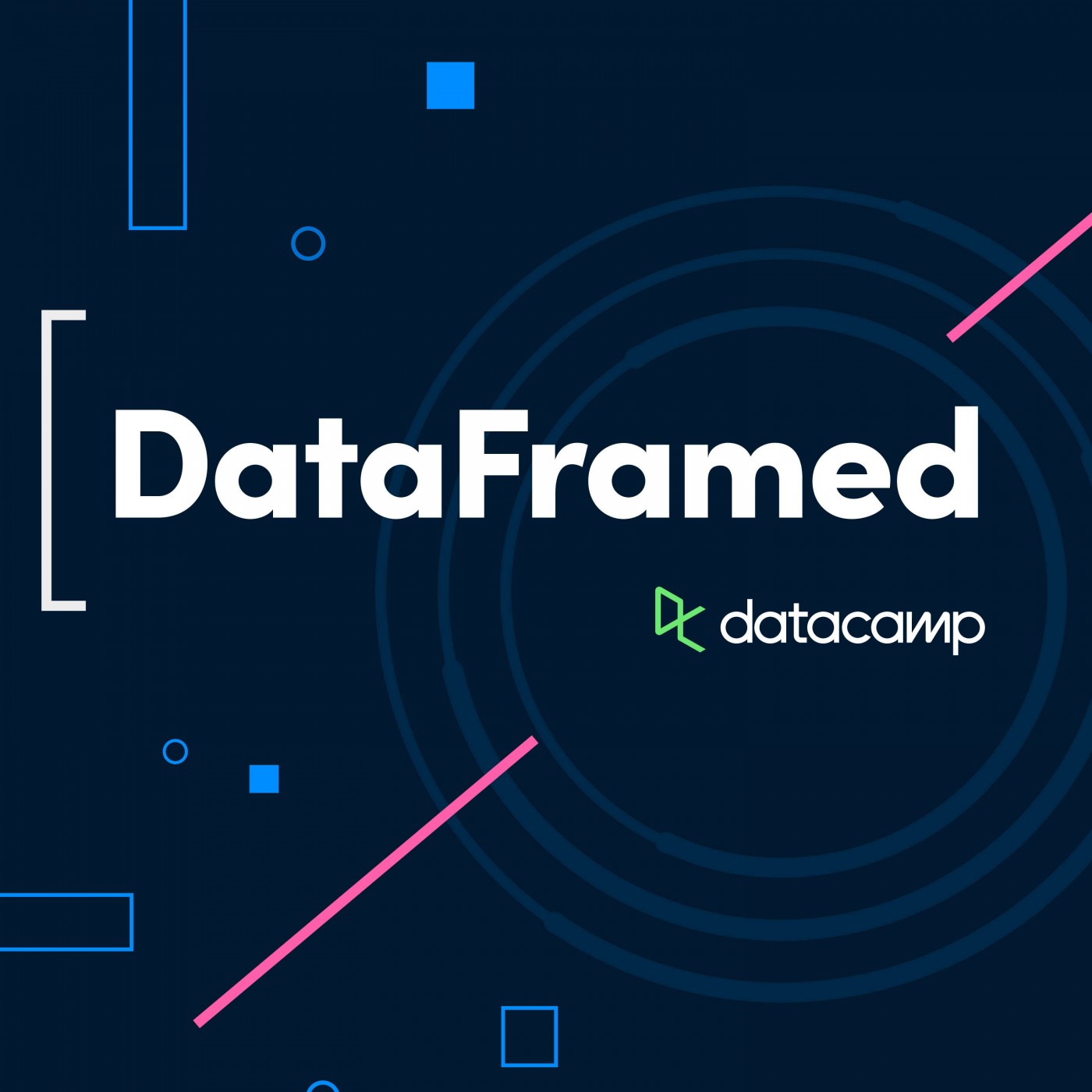
#36 Data Science and Ecology
Hugo speaks with Christie Bahlai, Assistant Professor at Kent State University, about data science, ecology, and the adoption of techniques such as machine learning in academic research. What are the biggest challenges in ecology that data science can help to solve? What does the intersection of open science and data science look like? In scientific research, what is happening at the interface between data science & machine learning methods, which are pattern-based, and traditional research methods, which are classically hypothesis driven? Is there a paradigm shift occurring here? Listen to find out!
Links from the show
The Bahlai Lab of applied quantitative ecology
Christie Bahlai on twitter
Hugo's article on What Data Scientists Really Do in Harvard Business Review
Hugo's webinar on What Managers Need To Know About Machine Learning
55:3420/08/2018
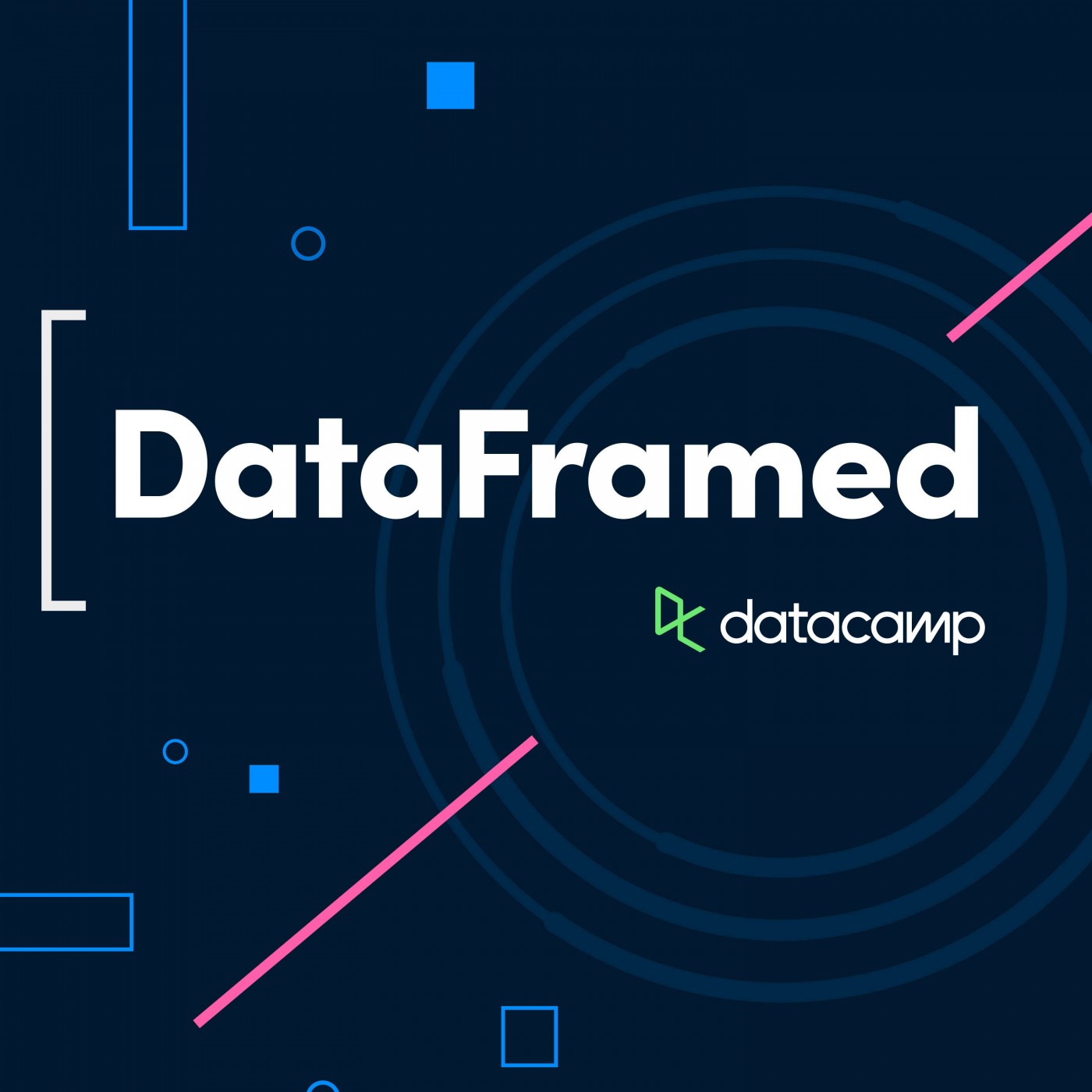
#35 Data Science in Finance
Hugo speaks with Yves Hilpisch about how data science is disrupting finance. Yves’ name is synonymous with Python for Finance and he is founder and managing partner of The Python Quants, a group focusing on the use of open source technologies for financial data science, artificial intelligence, algorithmic trading and computational finance. Why are banks such as Bank of America & JP Morgan adopting the open source data science ecosystem? What are the major sub-disciplines of Finance that data science is and can have a large impact in? How has the rise of data science changed the financial world and how the work is done and thought about? Stick around to find out.
59:1313/08/2018
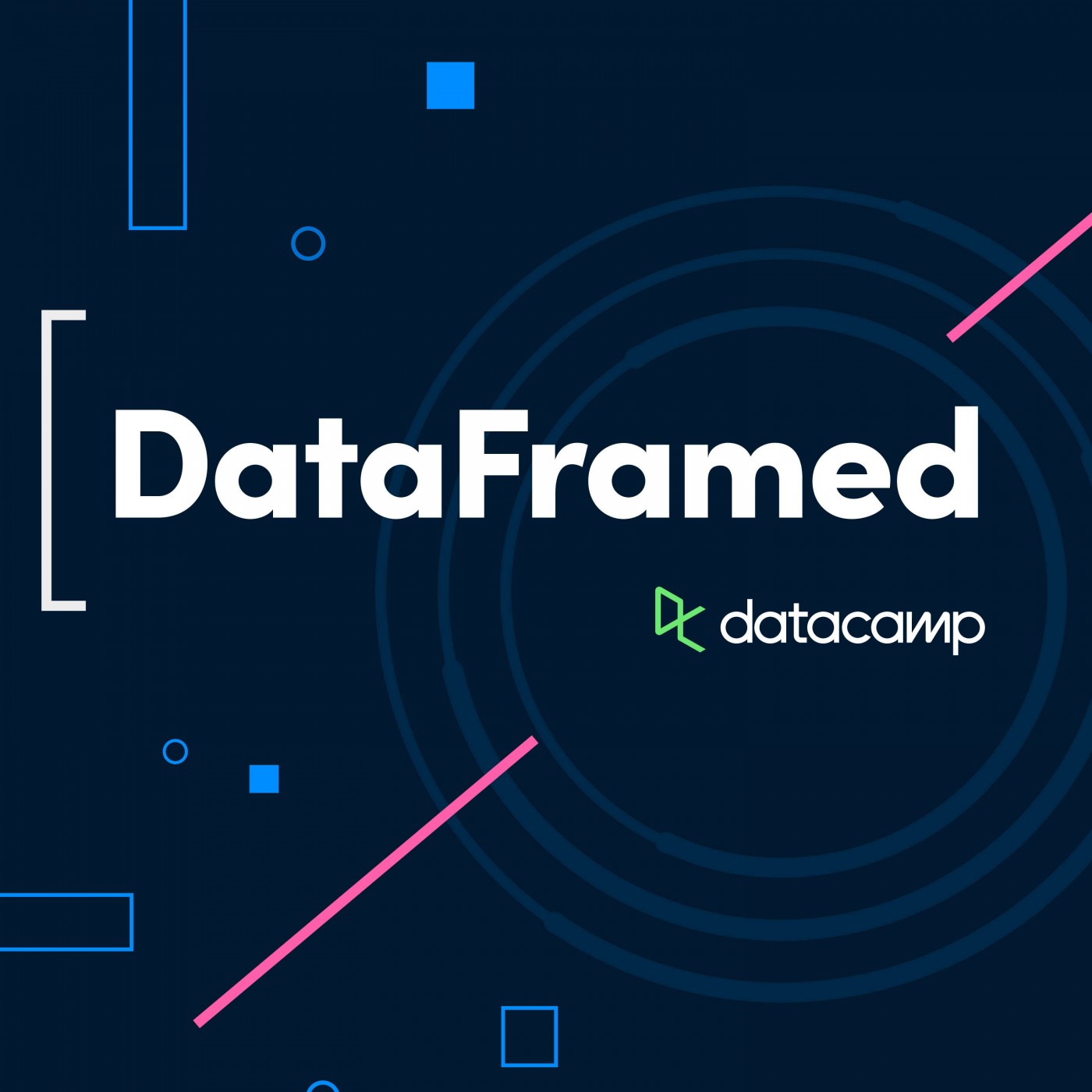
#34 Data Journalism & Interactive Visualization
Hugo speaks with Amber Thomas about data journalism, interactive visualization and data storytelling. Amber is a journalist-engineer at The Pudding, which is a collection of data-driven, visual essays. We’ll discuss the ins and outs of what it takes to tell interactive journalistic stories using data visualization and, in the process, we’ll find out what it takes to be successful at data journalism, the trade-off between being being a generalist and specialist and much more. We’ll explore these issues by focusing on several case studies, including a piece that Amber worked on late last year called “How far is too far? An analysis of driving times to abortion clinics in the US.”
56:2506/08/2018
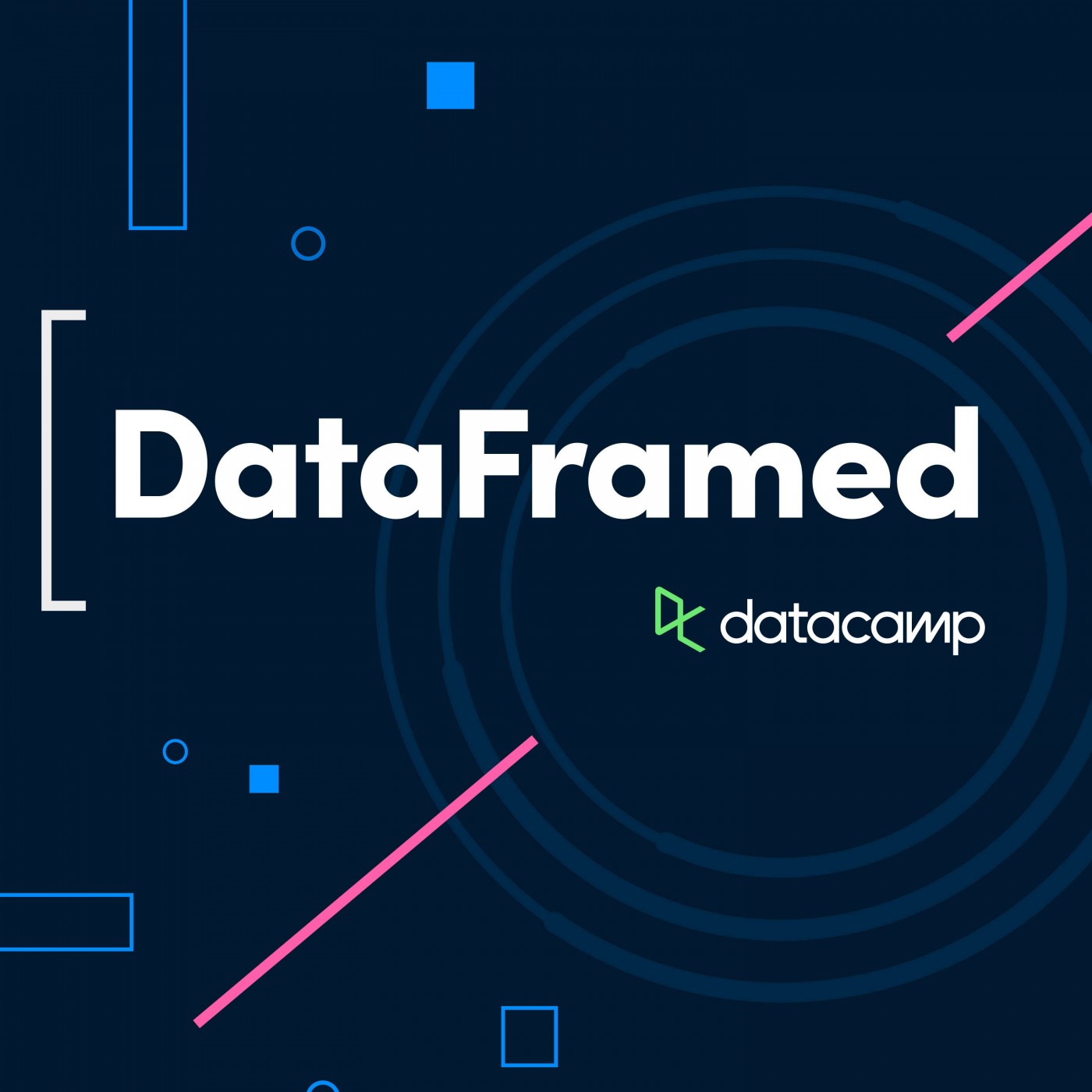
#33 Pharmaceuticals and Data Science
What are the biggest challenges in Pharmaceuticals that data science can help to solve? How are data science and statistics generally embedded in organizations such as Pfizer? What aspects of the pharmaceutical business run the gamut of nonclinical statistics? Hugo speaks with Max Kuhn, a software engineer at RStudio who was previously Senior Director of Nonclinical Statistics at Pfizer Global R&D. Max was applying models in the pharmaceutical and diagnostic industries for over 18 years.
59:5630/07/2018
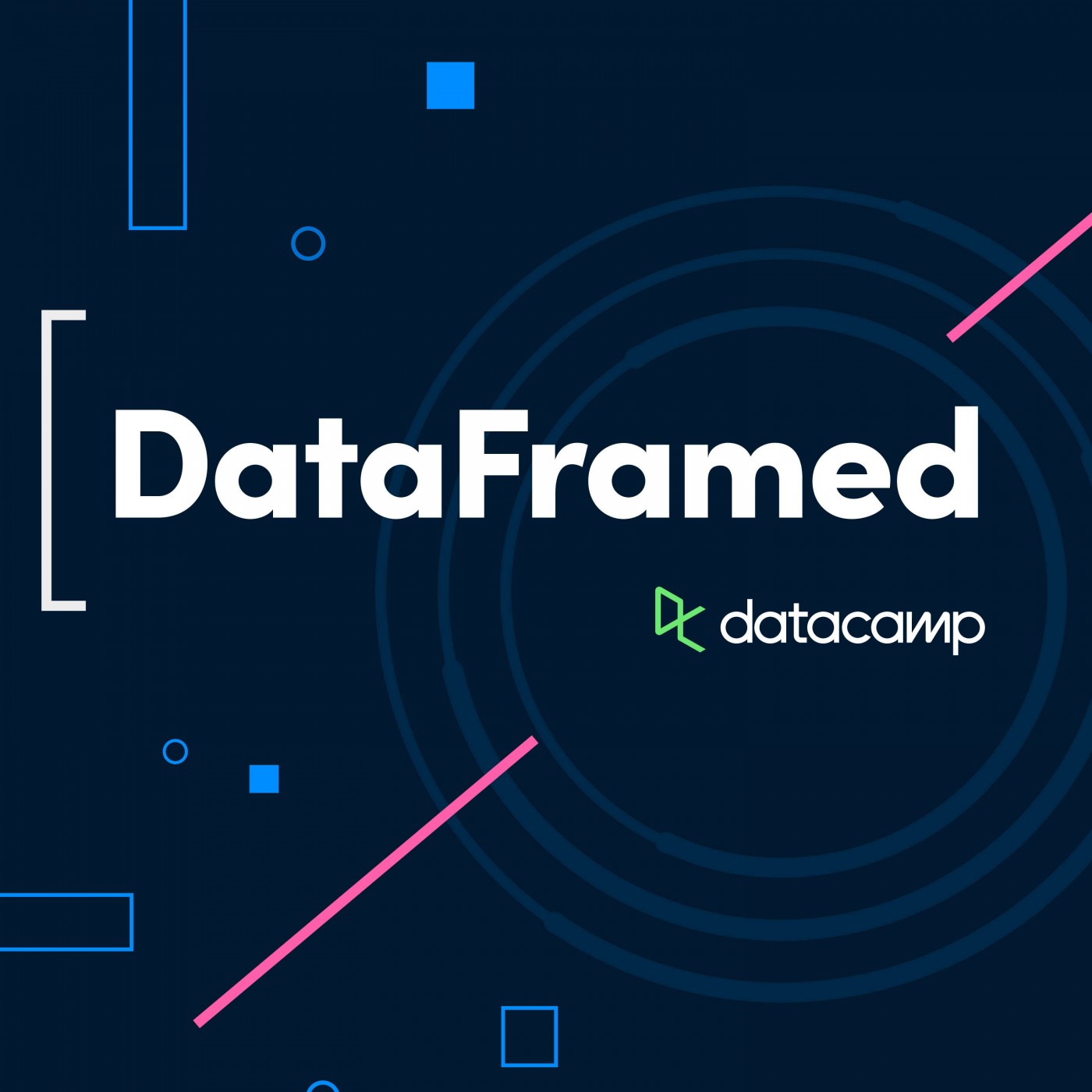
#32 Data Science at Doctors without Borders
Hugo speaks with Derek Johnson, an epidemiologist with Doctors without Borders. Derek leverages statistical methods, experimental design and data scientific techniques to investigate the barriers impeding people from accessing health care in Lahe Township, Myanmar. If you thought data science was all machine learning, SQL databases and convolutional neural nets, this is gonna be a wild ride as to get the data for their baseline health assessments, Derek and his team ride motorcycles into villages in northern Myanmar for weeks on end to perform in person surveys, equipped with translators and pens and paper because they can’t be guaranteed of electricity. Derek also researches the factors associated with the transmission of hepatitis C between family members and has helped to conduct studies in Uganda, Nepal, and India. All this and more.
54:5423/07/2018
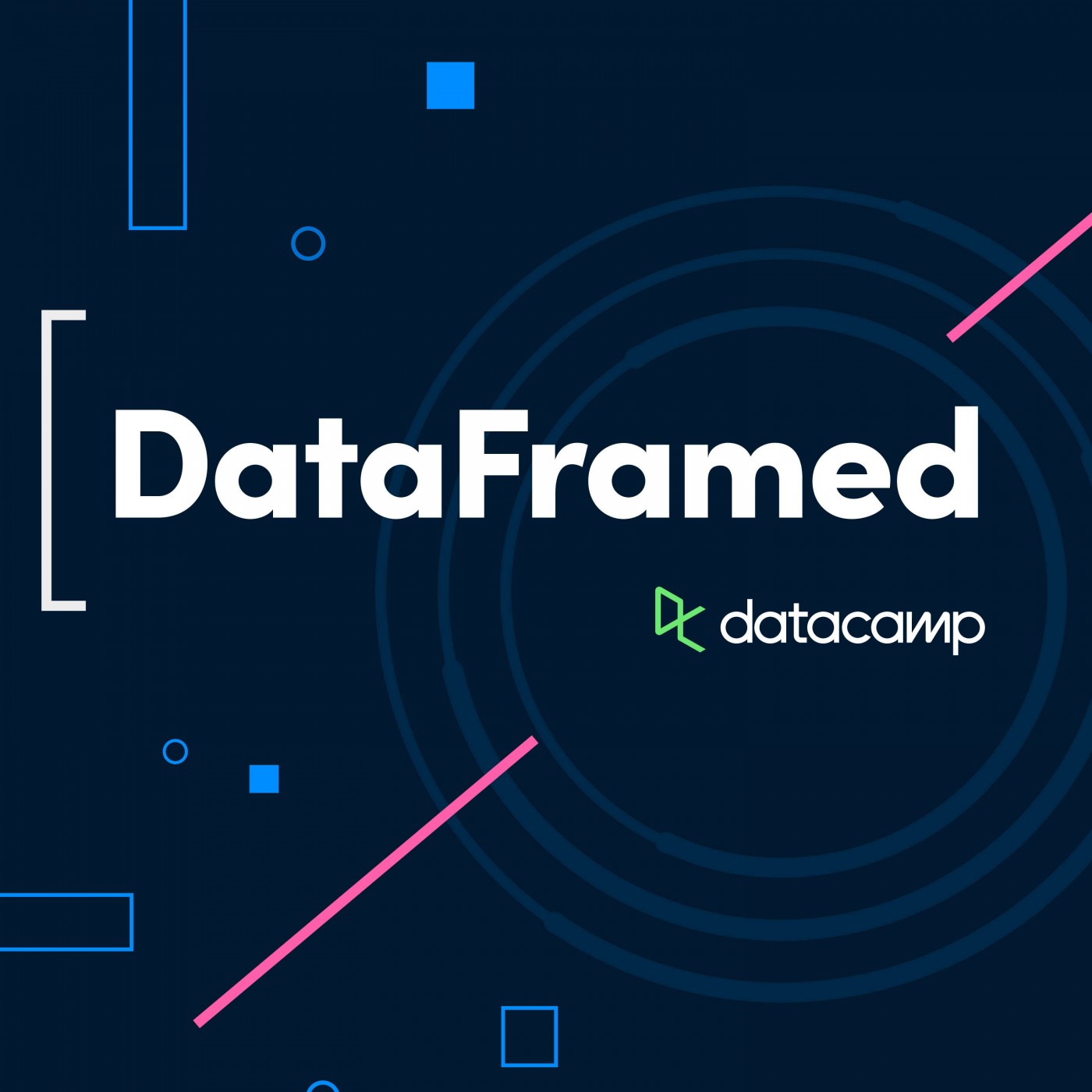
#31 Chatbots, Conversational Software & Data Science
Hugo speaks with Alan Nichol about chatbots, conversational software and data science. Alan is co-founder and CTO of Rasa, who build open source machine learning tools for developers and product teams to expand bots beyond answering simple questions. Which verticals are conversational software currently having the biggest impact on? What are the biggest challenges facing the fields of chatbots and conversational software? What misapprehensions do we as a society have about these technologies that experts such as Alan would like to correct? And how can we all build chatbots and conversational software ourselves?
57:0716/07/2018
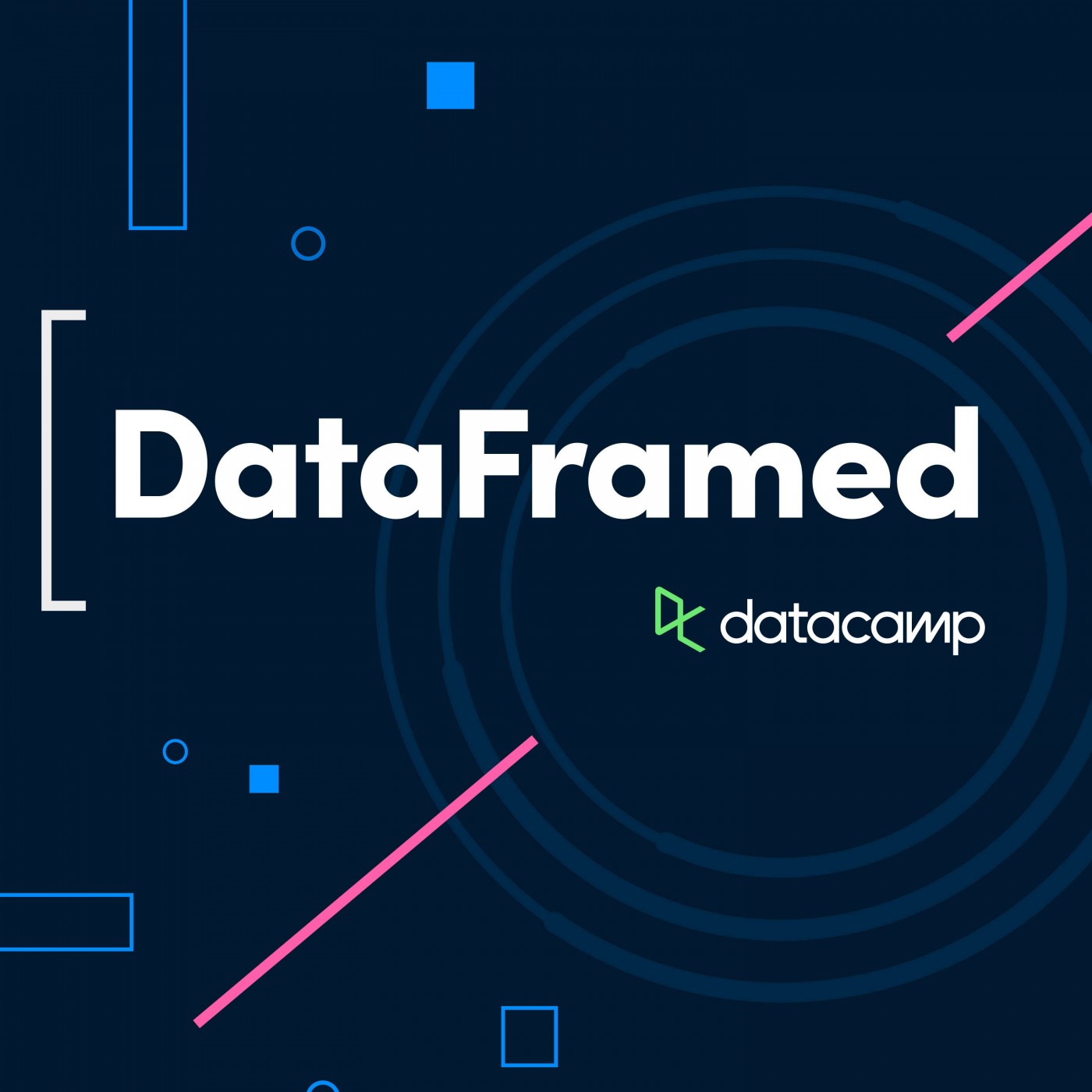
#30 Data Science at McKinsey
Hugo speaks with Taras Gorishnyy, a Senior Analytics Manager at McKinsey and Head of Data Science at QuantumBlack, a McKinsey company. They discuss
the role of data science in management consulting,
what it takes to change organizations through data science,
how the different moving parts of data science have evolved over the past decade and in which direction they’re heading.
You’ll see the impact that data science can have not only in tech, but also in such various verticals as retail, agriculture and the penal system. Taras will also take us through the 5 steps required to change organizations through data science, all of which are necessary. Can you guess what they are?
We're really excited to have Taras on the show as DataCamp has had a long relationship with McKinsey, including that McKinsey uses DataCamp for training.
56:5509/07/2018
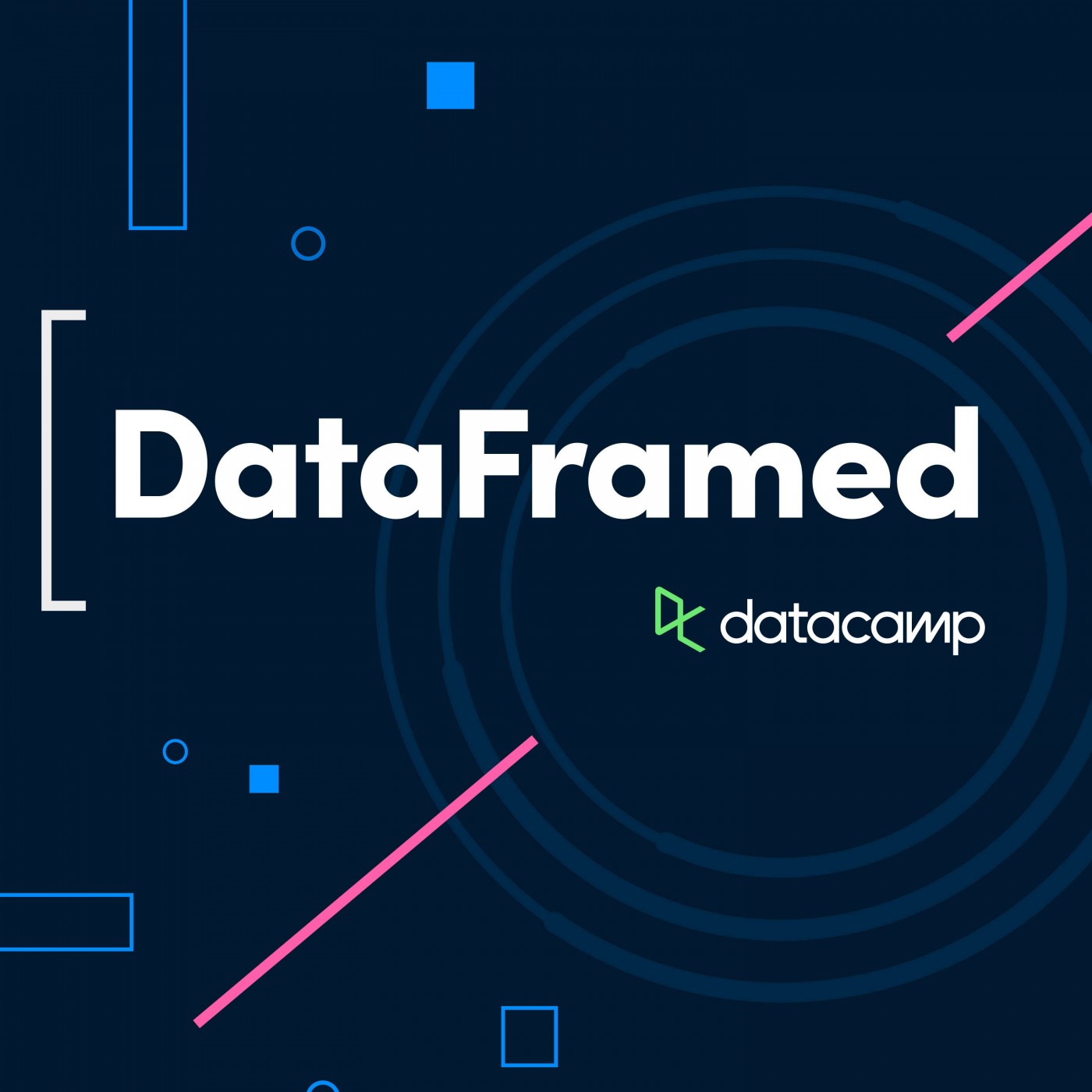
#29 Machine Learning & Data Science at Github
Omoju Miller, a Senior Machine Learning Data Scientist with Github, speaks with Hugo about the role of data science in product development at github, what it means to “use computation to build products to solve real-life decision making, practical challenges” and what building data products at github actually looks like.
Machine learning has the power to automate so much of the drudgery around data science & software engineering, from automated code review to flagging security vulnerabilities in code, and from recommending repositories to contributors to matching issues with maintainers and contributors and identifying duplicate issues.
And just in case that’s not enough, they'll discuss github as a platform for work, not just technical, and, as Omoju has called it, “a collaborative work environment centered around humans.”
58:4602/07/2018
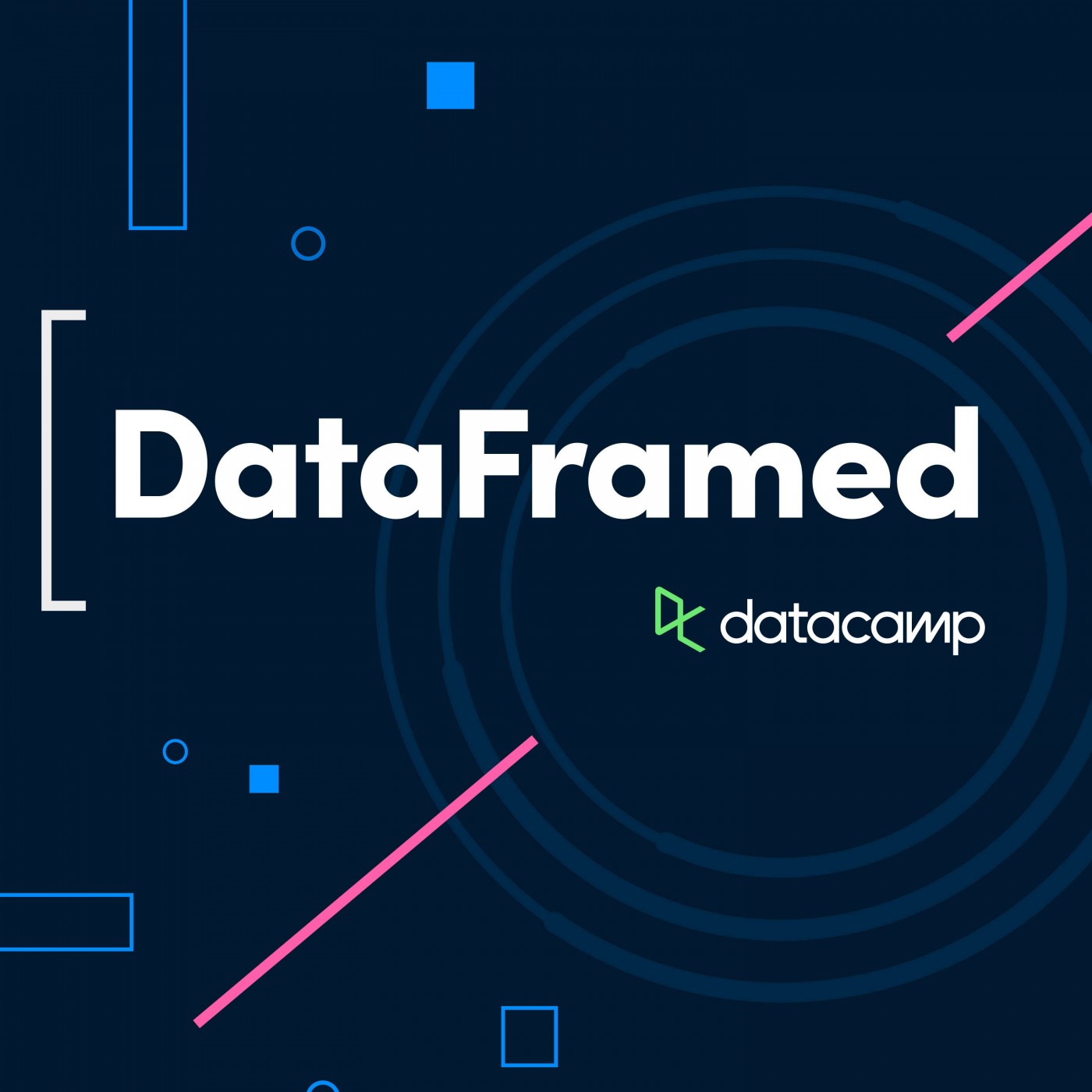
#28 Organizing Data Science Teams
What are best practices for organizing data science teams? Having data scientists distributed through companies or having a Centre of Excellence? What are the most important skills for data scientists? Is the ability to use the most sophisticated deep learning models more important than being able to make good powerpoint slides? Find out in this conversation with Jacqueline Nolis, a data science leader in the Seattle area with over a decade of experience. Jacqueline is currently running a consulting firm helping Fortune 500 companies with data science, machine learning, and AI. This interview is with Jacqueline Nolis, but at the time of recording, she went by Jonathan Nolis.
Links from the show
Jacqueline Nolis' website You're relying on data too much: making decisions worse, not better, by Jacqueline Nolis Hiring data scientists (part 1): what to look for in a candidate, by Jacqueline Nolis Jacqueline on Twitter For more, see our page here
59:0625/06/2018